- 1Division of Biomedical Engineering for Health and Welfare, Tohoku University Graduate School of Biomedical Engineering, Sendai, Japan
- 2Faculty of Mechanical Engineering, Department of Mechatronics, Optics and Mechanical Engineering Informatics, Budapest University of Technology and Economics, Budapest, Hungary
- 3Biomedical Engineering Department, Khalifa University, Abu Dhabi, United Arab Emirates
- 4Department of Kinesiology, Hungarian University of Sports Science, Budapest, Hungary
- 5School of Biomedical Engineering, The University of Sydney, Sydney, NSW, Australia
- 6Center for Human Movement Sciences, University of Groningen, University Medical Center Groningen, Groningen, Netherlands
- 7Somogy County Kaposi Mór Teaching Hospital, Kaposvár, Hungary
- 8Department of Sport Biology, Institute of Sport Sciences and Physical Education, University of Pécs, Pécs, Hungary
- 9Faculty of Information Technology, Pázmány Péter Catholic University, Budapest, Hungary
- 10Department of Medicine and Science in Sports and Exercise, Tohoku University Graduate School of Medicine, Sendai, Japan
We examined the effects of side-dominance on the laterality of standing stability using ground reaction force, motion capture (MoCap), and EMG data in healthy young adults. We recruited participants with strong right (n = 15) and left (n = 9) hand and leg dominance (side-dominance). They stood on one or two legs on a pair of synchronized force platforms for 50 s with 60 s rest between three randomized stance trials. In addition to 23 CoP-related variables, we also computed six MoCap variables representing each lower-limb joint motion time series. Moreover, 39 time- and frequency-domain features of EMG data from five muscles in three muscle groups were analyzed. Data from the multitude of biosignals converged and revealed concordant patterns: no differences occurred between left- and right-side dominant participants in kinetic, kinematic, or EMG outcomes during bipedal stance. Regarding single leg stance, larger knee but lower ankle joint kinematic values appeared in left vs right-sided participants during non-dominant stance. Left-vs right-sided participants also had lower medial gastrocnemius EMG activation during non-dominant stance. While right-side dominant participants always produced larger values for kinematic data of ankle joint and medial gastrocnemius EMG activation during non-dominant vs dominant unilateral stance, this pattern was the opposite for left-sided participants, showing larger values when standing on their dominant vs non-dominant leg, i.e., participants had a more stable balance when standing on their right leg. Our results suggest that side-dominance affects biomechanical and neuromuscular control strategies during unilateral standing.
Introduction
Functions such as language, speech, or face recognition show localization to one side of the brain. This phenomenon is called hemispheric lateralization. Due to the evolutionary specialization of the left hemisphere for skilled motor activities (Goodale, 1988; Gonzalez and Goodale, 2009; Stone et al., 2013), 90% of healthy adults are right-hand dominant and perform fundamental manual motor tasks with the right hand (Perelle and Ehrman, 2005; Vuoksimaa et al., 2009; Sartarelli, 2016). This behavioural asymmetry is known as “right-handedness” or “right-hand dominance”. The nature of hand dominance is also a consequence of brain lateralization through complex motor control processes (for reviews, see (Hatta, 2007; Sainburg, 2014)). Specifically, left-handedness is a marker of atypical cerebral lateralization. Unlike the upper limbs, the determination of leg dominance is more complicated because leg vs hand dominance is much less lateralized and seems to be task-dependent (Gabbard and Hart, 1996). Most of the leg dominance tasks are performed under bilateral conditions so that one leg stabilizes the system while the other leg moves as in kicking a ball, stepping up on a chair, or high jumping. The consensus suggests the mobilizing limb is the dominant leg in lower extremity tasks requiring two legs, an idea also supported by neurodevelopmental studies (Pompeiano, 1985; Previc, 1991). These studies suggest that the left leg subserves postural tasks while the right leg concurrently generates voluntary movements. Such functional organization is due to the asymmetric prenatal development of the vestibular function on the left side. Consequently, it may be not surprising that the left leg is suggested to be the preferred limb for tasks of unipedal stability (Maki, 1991). Overall, it seems that right-hand dominant people tend to be right-legged.
Feedback from lower extremity proprioceptors shapes postural stability in standing (Allum et al., 1998). Joint position sense (JPS) measurements reveal proprioceptive function at the knee (Barrett et al., 1991) and ankle (Konradsen, 2002) joints. Strongly right-side dominant individuals consistently sense movements more accurately in both upper and lower extremity joints of the non-dominant left vs the right-dominant side (Roy and MacKenzie, 1978; Kurian et al., 1989; Nishizawa, 1991; Goble et al., 2006; Goble and Brown, 2007, 2008; Han et al., 2013; Negyesi et al., 2019). We also found that left-sided participants performed a target-matching task more accurately with their dominant left vs right knee joint (Galamb et al., 2018). These data suggest that right-hemisphere specialization may underlie proprioceptive feedback (Naito et al., 2005; Goble and Brown, 2007, 2008), regardless of hand and leg dominance (side dominance). This idea is supported by clinical data demonstrating postural impairments including the side ipsilateral to a right-hemisphere stroke (Bohannon et al., 1986; Perennou et al., 2008; Duclos et al., 2015). It thus seems that central and peripheral functional asymmetry may differ between left-vs right-side dominant individuals. Therefore, further research is needed to resolve the differences in co-lateralization of postural stability between left- and right-side dominant healthy adults.
Balance is a fundamental motor skill that underlies gait and posture through the activation of muscular synergies (Torres-Oviedo and Ting, 2007; Chvatal and Ting, 2012) generated by the central nervous system (CNS) (Winter 1995; Winter et al., 2003). Proprioceptive feedback from the lower extremity joints forms an important element of this control. Static balance is the maintenance of the equilibrium under unperturbed conditions such as during quiet standing (Papegaaij and Hortobágyi, 2017). Standing balance stability can be assessed by multiple ways, including kinetics, kinematics, and neural control via electromyography (EMG). During quiet standing, the base of support is fixed and balance is maintained by countering torques produced by the gravitational force around the center of mass (CoM). As a result, the center of pressure (CoP) shifts within the base of support. Because balance is controlled by keeping the position of the CoM between the weight-bearing limbs (Gray, 1944), kinetic data of CoP movements provide information on how the CNS controls standing stability through the involvement of three synergistic muscle groups (Krishnamoorthy et al., 2004). Short CoP paths are associated with high standing stability (Lepers et al., 1997). Whether or not leggedness affects bi- or unipedal standing stability is unclear. CoP path does not seem to differ between dominant vs non-dominant leg during unilateral (Matsuda et al., 2008; Lopez-Fernandez et al., 2020) and bipedal (Haddad et al., 2011) stance. Perhaps CoP path data are not sensitive enough for detecting leg-differences in standing stability. Especially, because these studies only recruited right-side dominant participants and limited their analysis only to CoP data. Such data form a resultant outcome that condenses information on the whole-body posture and information on postural accelerations into one two-dimensional (2D) variable (Federolf, 2016). Nevertheless, examining a variety of traditional CoP metrics including sway, velocity, and area of ellipse (Prieto et al., 1996; Rocchi et al., 2004; Sun et al., 2018) is fundamental and important for the assessment of standing stability.
Because athletes often encounter situations in which the CoM is controlled while standing on one leg, CoP paths and velocity are complementary to CoM outcomes in indexing standing stability (Vuillerme et al., 2001; Paillard et al., 2006). A complimentary approach to assessing balance stability is kinematics, using motion capture (MoCap). Specifically, by affixing reflective markers over anatomical landmarks, we can measure body kinematics by a motion capture system that determines the positions of the markers (Alradwan et al., 2015). Briefly, several camera recordings are used by the system to calculate trajectories of the markers or the position and orientation of the rigid bodies, and to estimate the motions of the underlying bones to produce joint kinematics data (Cappozzo et al., 2005). Lower limb joint angular kinematics via MoCap is widely used in the last few decades to analyze postural control (reviewed in (Roggio et al., 2021)). While CoP provides information only about foot-related events, MoCap data informs us about CoM displacement and other descriptive aspects of standing balance stability. With respect to stability, measuring a set of variables representing change and deviation for each lower extremity joint motion time series, e.g., range, total movement, velocity, or acceleration would shed light on the laterality effects on standing stability.
Ultimately, the neural command controls kinetic and kinematic features by activating muscle groups and muscle synergy modules. Specifically, earlier studies using principal component analysis (PCA) identified that muscles are not independently controlled but work in groups in various tasks requiring shifts of the CoP (Krishnamoorthy et al., 2003a; Krishnamoorthy et al., 2003b). Therefore, EMG data analyses from five muscles in these three synergistic muscle groups (anterior leg muscles, posterior leg muscles, trunk muscles) responsible for controlling posture (Krishnamoorthy et al., 2004) can provide insights into the neural control of stability while standing (Danna-Dos-Santos et al., 2015). The relationship between standing and walking balance abilities and muscle activation is well described (Papegaaij and Hortobágyi, 2017; Cinthuja et al., 2021). The literature (Phinyomark et al., 2012a; b; Phinyomark et al., 2014; Karthick and Ramakrishnan, 2016; Khushaba et al., 2017; Samuel et al., 2018; Waris and Kamavuako, 2018; Too et al., 2019; Verma and Gupta, 2019; Toledo-Pérez et al., 2020) also reports a wide spectrum of time- and frequency-domain features of the EMG signal that could help to determine if the synergistic muscles’ activity contributes to the potential differences, if any, between left- and right-side dominant individuals during bi- and unipedal stance.
It is unclear if the aforementioned three methods produce complementary results when used in combination, a measurement scheme rarely used. Therefore, the primary objective of the present study was to examine whether side-dominance affects the laterality of bi- and unilateral postural stability during quiet standing in healthy younger adults using a multitude of biosignal processing methods: CoP, MoCap, and EMG data. Based on the preponderance of data from previous studies suggesting right-hemisphere specialization for standing stability, we hypothesize right-side dominant participants to have more stable standing balance in their non-dominant left vs right-dominant leg. However, left-sided participants may have more stable standing balance in their dominant left as compared to their non-dominant right leg. Consequently, during non-dominant unilateral stance, we expect more stable standing balance in right-compared to left-sided participants but vice versa during dominant leg stance. We also hypothesize that these between-group and within-group differences will be concordantly reflected across measures of kinetics, kinematics, and muscle activation. Our study fits under the current research efforts towards understanding the effects of side-dominance on postural control.
Methods
Participants
Sample size calculations (G*Power 3.1.7 (Faul et al., 2007)) based on a previous study (Danna-Dos-Santos et al., 2015) that aimed to determine the effects of visual information on multi-muscle control during quiet stance, revealed that a total sample size of 16 would be appropriate to detect significant differences between the groups, assuming type I error of 0.05 and power of 0.80.
A large number of potential participants were asked to fill out two inventories to clarify their hand and leg dominance. Hand dominance was determined using the Edinburgh Handedness Inventory (Oldfield, 1971), a scale that is used to measure the degree of hand laterality in daily activities such as writing, drawing, throwing, using scissors, brushing teeth, opening a box, striking a match and using a pair of scissors, a knife, a spoon, and a broom. Leg dominance was determined by one- or two-foot item skill tests such as kicking a ball or stepping up on a chair (Spry et al., 1993). Laterality index (LI) for both hand and leg dominance was calculated by summing the number of tasks performed with the right limb (R) and the number of tasks performed with the left limb (L) as follows (R - L)/(R + L). The required level of laterality for both hand and leg dominance was LI ≥ 0.9.
We finally recruited nine left-side dominant (age = 27.9 ± 5.8 years; height = 179.2 ± 7.6 cm; mass = 76.3 ± 8.2 kg; three females) and 15 right-side dominant (age = 28.2 ± 5.5 years; height = 173 ± 8.2 cm; mass = 67.9 ± 13.3 kg; six females) participants who met our laterality conditions with no reported neurological deficit or sensorimotor impairment. Laterality index for both hand and leg dominance was ≥0.9 in right-side dominant, and ≥ -0.9 in left-side dominant participants, showing strong right- or left-side dominance, respectively. After giving both verbal and written explanations of the experimental protocol, participants signed the informed consent document in accordance with the declaration of Helsinki. The study was carried out in accordance with the recommendations of the University Ethical Committee (Approval No. TE-KEB:2:2021).
Experimental design
Each participant performed barefoot bi- and unilateral (with both the dominant and non-dominant leg) quiet standing trials on either two or one force plate, respectively, both with eyes-open in a randomized order. Participants were asked to stand as still as possible during each trial. Each trial lasted for 50 s with a rest period of 60 s between trials (Garcia-Masso et al., 2016). Each participant adopted the same foot placement and posture during the tasks: 1.) during bipedal stance, the participants’ heels were separated by the width of their shoulders and their toes pointed forward, while 2.) during unilateral stance, participants were asked to raise their heel by flexing their non-supporting leg’s knee joint at 90° to ensure the absence of contact between the foot and the floor during the trial. Only successful trials were considered, therefore, if the participants bent their trunk or arms, or touched down with the non-supporting leg, the trial was repeated after a recovery period of 60 s. Nevertheless, the frequency of failures was very low and did not differ between right and left-side dominant participants neither during dominant nor during non-dominant unilateral stance. During each trial, participants were instructed to look at a point of reference (5 cm in diameter) placed in front of them at eye level at a distance of 2 m and keep their arms down by their side. The CoP, MoCap, and EMG recordings were synchronized in time by starting the data analyses from a trigger signal that appeared in all channels.
Experimental procedures
CoP-related kinetics
Ground reaction force (GRF) was measured by a pair of synchronized force platforms (P-6000, BTS Bioengineering SnA., Garbagnate Milanese MI, Italy) at a sampling rate of 1 kHz. The two platforms recorded the GRF in a common reference frame that coincided with the MoCap system’s reference frame. During bipedal standing, one foot was placed on each platform. The platforms are able to measure the CoP (point of attack of the GRF), the magnitude of the GRF in three dimensions, and the torque around the vertical axis that goes through the CoP.
Kinematics
In addition, participants’ movements were captured via an optical-based MoCap system (OptiTrack, 18 pieces of Flex13 cameras, NaturalPoint Inc., Oregon, United States ) at a 100 fps sampling rate using 16 skin-attached retro-reflective markers placed at anatomical locations (Figure 1A). The 3D avatar of a representative participant during bi- and unilateral stances is shown in Figures 1B,C, respectively.
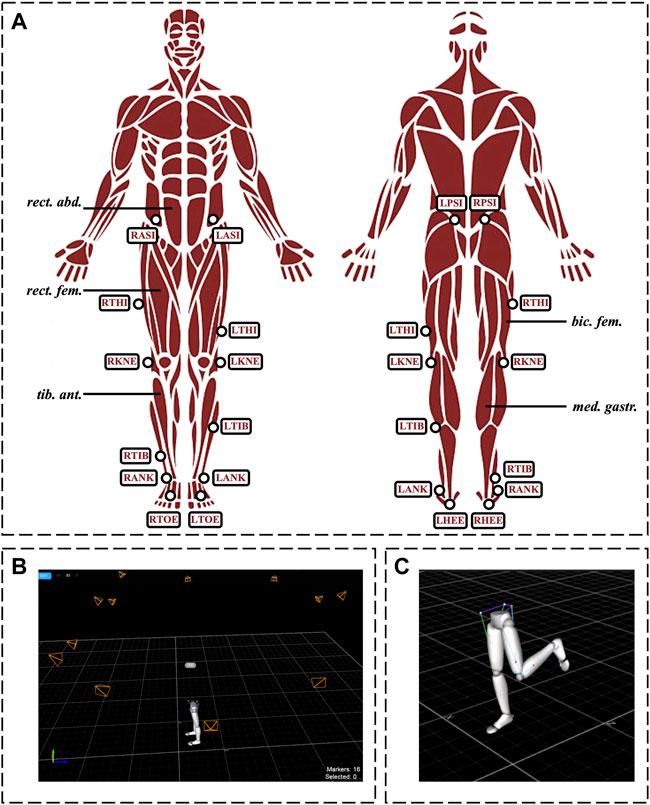
FIGURE 1. Experimental setup. Panel (A): Schematic illustration of the measured muscles for EMG analysis, and the placement of the reflective markers for motion capture measurements. Panel (B) and (C): 3D avatar of a representative subject’s lower limbs during bi- and unilateral stances, respectively. Muscles: bic. fem., biceps femoris; med. gastr., medial gastrocnemius; rect. abd., rectus abdominis; rect. fem., rectus femoris; tib. ant., tibialis anterior. Markers: LANK/RANK, left/right lateral malleolus; LASI/RASI, left/right anterior superior iliac spine; LHEE/RHEE, left/right heel (bisection of the distal aspect of the posterior calcaneum); LKNE/RKNE, left/right knee (lateral epicondyle of the femur); LPSI/RPSI, left/right posterior superior iliac spine; LTHI/RTHI, left/right thigh (not an exact location, only to aid with sides); LTIB/RTIB, left/right shank (not an exact location, only to aid with sides); LTOE/RTOE, left/right toes (between the distal ends of the 1st and 2nd metatarsi).
Electromyography (EMG)
To record EMG signals, a Cometa Wave Plus wireless EMG system with Mini Wave Waterproof units and the EMGandMotionTools 7.0.10.0 software (Cometa S. r.l., Bareggio MI, Italy) were used. EMG signals were recorded on both sides of the body using surface bipolar electrodes. The measured muscles and muscle groups were the following: 1. Posterior muscles: medial gastrocnemius and biceps femoris, 2. Anterior muscles: tibialis anterior and rectus femoris, 3. rectus abdominis (Figure 1A). Electrodes were placed according to the recommendations of the SENIAM project (seniam.org) on the muscular belly with an inter-electrode distance of approximately 20 mm. The sampling frequency was 2000 Hz.
Data analyses
CoP-related kinetic data
The CoP-sway time-series collected were jointly defined by anterior-posterior (AP) and medio-lateral (ML) direction signals. Considering that the analysis of phase lag or time-related signal features was unnecessary in the present study, the AP and ML time-series were first filtered at 5 Hz using a 4th order Butterworth low pass filter (Prieto et al., 1996; Sun et al., 2018), then referenced to the mean CoP. Furthermore, the resultant distance time series was calculated to quantify the CoP signal in the combined AP and ML directions. The first second of data was excluded from the analysis to eliminate the filter’s transient response. To analyze the CoP-sway and assess standing stability, a total of twenty-three variables (Table 1) were computed from both the independent AP and ML time-series as well as the resultant distance time-series using MATLAB® (MATLAB R2021b, MathWorks, Natick, MA, United States ). A variety of traditional CoP metrics often used in literature (Prieto et al., 1996; Rocchi et al., 2004; Sun et al., 2018) were determined from the CoP data, including: the mean distance (mdist), standard deviation (SD), root mean square distance (RMS_dist), range (range), path length (pathlength), mean velocity (mvelo), 95% confidence ellipse area (area_ce), fractal dimension (fd), sway area (area_sw) and mean frequency (mfreq). Moreover, the sample entropy (SampEn) was evaluated to analyze the regularity and complexity of the CoP-sway signal. Briefly, SampEn is the negative logarithm of the conditional probability that a dataset having repeated itself for m samples within a tolerance r will also repeat itself for m+1 samples, excluding self-matches. The SampEn algorithm was obtained from Richman et al. (Richman and Moorman, 2000). A range of m values from 2 to 9 and r values from 0.01 to 0.9 were examined and the parameters m = 2 and r = 0.01 were found to be appropriate for our data. The signal length was fixed at N = 24,000 samples for all the SampEn computations and the total sample entropy was determined as the average of SampEn results for consecutive signal intervals of size N starting from the end of the signal. Table 1 presents and defines all the CoP-based variables computed in this study. To control for weight distribution during bipedal stance, the symmetry index (Rougier and Genthon, 2009; Hendrickson et al., 2014) was calculated using the total GRF measured under each foot from the left and right force platforms.
The symmetry index can range between 0 and 1. A symmetry index of 0.5 indicates that the values for the dominant and non-dominant limbs are equal, i.e., represents perfect symmetry. If the index is greater than 0.5, the dominant vs. non-dominant limb has a greater value of weight distribution during bipedal stance.
Kinematic data acquired from MoCap analysis
Experimental marker placement was replicated on a lower limb model in OpenSim 4.3 (Delp et al., 2007; Seth et al., 2018), a software for biomechanical modeling, simulation and analysis of movement. The model was scaled for each subject and inverse kinematics were solved to determine the joint angles describing the motion in each trial. The rotational movements calculated were the pelvic list, tilt and rotation, hip flexion, rotation and adduction, and knee and ankle flexion. Pelvic translational motion was also included in the analysis. The joint motion time series were filtered using a 4rth order Butterworth low pass filter with a cut-off frequency of 5 Hz (Schurr et al., 2017). Moreover, the first second of all signals was excluded to eliminate the filter’s transient response. To evaluate standing stability, a set of variables representing change and deviation for each joint motion time series were computed in MATLAB® (MATLAB R2021b, MathWorks, Natick, MA, United States ), including: the standard deviation (sd), range (range), total movement (totmov), root mean square velocity (rvelo), root mean square acceleration (raccel), and mean percentage index (mpi). The motion capture analysis variables used in this study are summarized and defined in Table 2.
EMG data
The EMG data from all 10 channels was obtained from five different muscles of both the left and right lower limbs during each standing condition. First, wavelet filters were applied using MATLAB® (Misiti et al., 1996) to remove any unwanted artifacts in the signal obtained. In this study, we used wavelet filters between 30 and 500 Hz to reduce the effects of high-frequency noise and low-frequency artifacts in the EMG signals. In MATLAB ‘wfilters ( )’ returns the four lowpass and highpass, decomposition, and reconstruction filters associated with the orthogonal or biorthogonal wavelet. In order to set the cut-off frequencies to make the custom wavelet filter, we decomposed the EMG signals into 13 levels and reconstructed from levels 2 to 5 of decomposed signals with symlet mother wavelet (Sym5). More details can be found elsewhere (Vidakovic and Mueller, 1994). Following that, 39 different time- and frequency-domain features were extracted from each channel of the filtered signal. A brief description of the examined EMG features, their abbreviations, the corresponding equations, and the relevant references are shown in Table 3.
Statistical analyses
Statistical analyses were performed using SPSS Statistics Package (version 22.0, SPSS Inc., Chicago, IL, United States ). Variables were normally distributed, measured by Shapiro–Wilk’s test of normality and visual inspection of their histograms. Separate multivariate analysis of variance (MANOVA) was applied to comprehensively assess the interaction and/or main effect of 1) CoP-related kinetic (dimensionless parameters, parameters for AP direction, parameters for ML direction), 2) kinematic (hip adduction, hip flexion, hip rotation, knee, ankle), and 3) EMG (medial gastrocnemius, biceps femoris, tibialis anterior, rectus femoris, rectus abdominis) variables for the group (side dominance: right-side dominant [R], left-side dominant [L]) by the laterality (dominant leg, non-dominant leg) during bi- and unilateral standing. In case of significant main effect or interaction, a series of group × laterality mixed ANOVA and planned post-hoc tests with Bonferroni correction for multiple comparisons were performed in each condition (bipedal stance, single leg stance) for each dependent measures (CoP, EMG, MoCap) to statistically investigate the effect of side-dominance on the laterality of bi- and unilateral standing stability. The Greenhouse–Geisser correction was used when data violated the assumption of sphericity. Complementary post-hoc analyses (paired-samples t-tests) were used when indicated. Cohen’s effect size (d) was also computed as appropriate. Additionally, effect sizes of repetition factors were expressed using partial eta squared (ηp2) (Peat et al., 2008). In order to determine if between- or within-group differences in CoP, EMG, and MoCap data were associated with each other, Pearson’s correlations were computed. Statistical significance was set at p < 0.05.
Results
Bipedal stance
Table 4, Table 5, Table 6 summarize the results of kinetic, kinematic, and EMG data, respectively. The symmetry index analyses showed that none of the participants stood symmetrically during bipedal stance. Specifically, 87% of L had greater weight-bearing on their dominant left leg (symmetry index >0.5), while 87% of R had greater weight-bearing on their non-dominant left leg (symmetry index <0.5). In other words, 62.5% of participants had greater weight-bearing on their left leg, regardless of side-dominance. Multivariate ANOVA (MANOVA) demonstrated that there was no significant group and laterality main effects or their interactions (all p > 0.05) on CoP-related kinetic parameters during bipedal stance, regardless of the dimension of the parameters (dimensionless, AP direction, ML direction) suggesting that CoP-related kinetic data of L and R was similar both in their dominant and non-dominant leg (Table 4). Furthermore, no group and laterality main effects or their interactions occurred in the kinematic data or EMG activity of the measured muscles during bipedal stance (all p > 0.05).
Single leg stance
No group and laterality main effects or their interactions was detected by MANOVA in any of the kinetic data during single leg stance suggesting that standing stability detected by a variety of CoP-related parameters did not differ between R and L (Table 4).
There was however a group by laterality interaction in knee and ankle flexion (each Pillai’s trace = 0.286, F6,39 = 2.6, p = 0.032, ηp2 = 0.29) revealed by MANOVA. Additional mixed ANOVAs in single kinematic parameters of the knee joint detected group × laterality interaction on totmov (F1,22 = 6.6, p = 0.018, ηp2 = 0.23) (Figure 2) and range (F1,22 = 8.9, p = 0.007, ηp2 = 0.29) of knee flexion with the post-hoc analysis showing larger values in L vs R (each p ≤ 0.022) (Table 5) during dominant leg stance. In addition, this pattern was also found in rvelo (L: 3.45 ± 1.62°/sec vs R: 2.09 ± 1.08°/sec, p = 0.027, d = 1.04) and sd (L: 1.75 ± 0.90° vs R: 0.84 ± 0.63°, p = 0.005, d = 1.39) of knee flexion during dominant leg stance (Figure 2; Table 5). Moreover, L showed larger values in each measured variable of knee flexion when standing on their dominant vs non-dominant leg (each p ≤ 0.013). Regarding the kinematic data of ankle flexion, group by laterality interactions were found on totmov (F1,22 = 13.6, p = 0.001, ηp2 = 0.38), range (F1,22 = 7.0, p = 0.015, ηp2 = 0.24), rvelo (F1,22 = 11.9, p = 0.002, ηp2 = 0.35), and raccel (F1,22 = 17.3, p < 0.001, ηp2 = 0.44) of ankle joint angle during non-dominant leg stance with the post-hoc analysis showing larger values in R as compared to L during non-dominant leg stance (each p ≤ 0.05) (Figure 2; Table 5). Moreover, R always produced larger ankle joint angle values during non-dominant vs dominant unilateral stance (each p ≤ 0.043), however, this pattern was the opposite in case of L showing larger values when standing on their dominant vs non-dominant leg (each p ≤ 0.033) (Figure 2; Table 5).
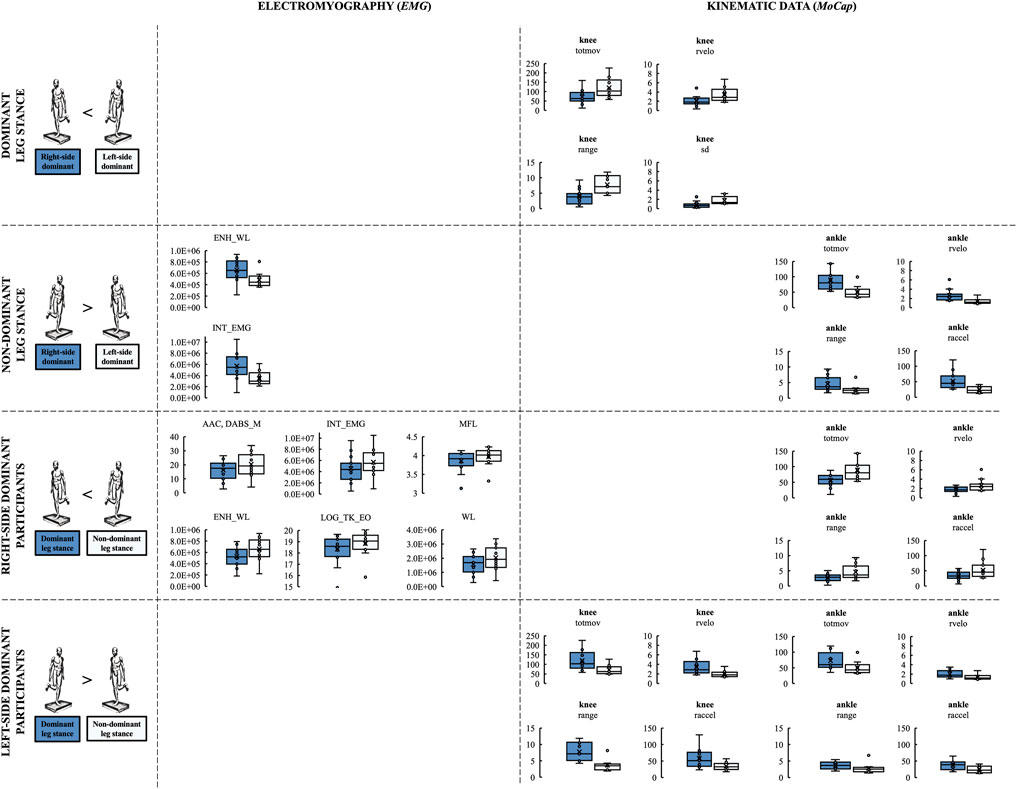
FIGURE 2. Between-group and within-group differences in CoP, EMG, and MoCap outcomes during unilateral stance. EMG outcomes: AAC, Average Amplitude Change; DABS_M, Difference Absolute Mean Value; ENH_WL, Enhanced Wavelength; INT_EMG, Integrated EMG; LOG_TK_EO, Log Teager Kaiser Energy Opertor; MFL, Maximum Fractal Length; WL, Waveform Length. Kinematic outcomes: raccel, RMS acceleration; range, Range; rvelo, RMS velocity; sd, Standard deviation; totmov, Total movement.
There was a group main effect (Pillai’s trace = 0.810, F1,22 = 2.4, p = 0.038, ηp2 = 0.81) on medial gastrocnemius EMG activity revealed by MANOVA. Additional statistical analyses based on group × laterality interaction and their post-hoc analyses revealed that ENH_WL and INT_EMG values of medial gastrocnemius were higher in R vs L when standing on their non-dominant leg (p = 0.045, d = 0.93; p = 0.047, d = 0.94; respectively) (Figure 2; Table 6). In addition, R had larger values in seven EMG features of medial gastrocnemius when standing on the non-dominant vs dominant leg (each p ≤ 0.05) (Figure 2; Table 6).
Association between dependent variables
Strong association was found only among MoCap variables that showed between-group differences during non-dominant unilateral standing (each r ≥ 0.778 and p < 0.001) but no association was found with medial gastrocnemius EMG activity. Similarly, no associations were found between those MoCap and EMG variables that showed larger values for R when they were standing on their non-dominant vs dominant leg (all p ≥ 0.05).
Discussion
We examined the effects of side-dominance on the laterality of bi- and unilateral standing stability in healthy adults by using a multitude of biosignal processing methods. While no differences occurred between left- and right-side dominant participants in kinetic, kinematic, or EMG outcomes during bipedal stance, the symmetry index revealed that 87% of right- but only 13% of left-side dominant participants had greater weight-bearing on their non-dominant leg. Regarding single leg stance, larger knee but lower ankle joint kinematic values appeared in left vs right-sided participants during non-dominant stance. Left-vs right-sided participants also had lower medial gastrocnemius EMG activation during non-dominant stance. While right-side dominant participants always produced larger values for kinematic data of ankle joint and medial gastrocnemius EMG activation during non-dominant vs dominant unilateral stance, this pattern was the opposite for left-sided participants showing larger values when standing on their dominant vs non-dominant leg, i.e., participants had a more stable balance when standing on their right leg.
Less stable standing balance of right-side dominant participants during non-dominant leg stance
In the present study, participants performed both bi- and unilateral stances, however, data acquired during unilateral stance might be a better marker of postural control because standing on one vs two legs poses a greater challenge for the neural command to organize kinematics and kinetics of stability and could thus be more informative about the role of proprioception in standing stability. Furthermore, unilateral vs bilateral stance challenges the postural control system and is more often performed during daily and sports activities (Vuillerme et al., 2001; Paillard et al., 2006).
Previous studies (Dietz et al., 1989; Goldie et al., 1989; Geurts et al., 1993; Hoffman et al., 1998) have demonstrated no significant differences in postural control measures between healthy participants’ right and left limbs during unilateral stance. However, only one of these studies (Hoffman et al., 1998) determined the functionally dominant and non-dominant lower limb of participants. In the present study, we recruited healthy participants without a history of orthopedic or neuromuscular injuries. Although some previous studies (Freeman et al., 1965; Hoffman et al., 1998) suggested an acute or chronic injury when asymmetry is present in unilateral balance testing, neurodevelopmental studies (Pompeiano, 1985; Previc, 1991) suggest laterality effects on standing posture, i.e., the left leg subserves postural tasks while the right leg performs tasks involving voluntary movement. Differences in the movement characteristics between the right and left limbs are also supported by the association found between lower limb laterality and different activation characteristics in the primary sensorimotor cortex and the basal ganglia (Kapreli et al., 2006). Consequently, it may be not surprising that the left leg is suggested to be the preferred limb for tasks of unipedal stability (Maki, 1991).
Considering that feedback from lower extremity proprioceptors shapes postural strategy while standing (Allum et al., 1998), results from previous studies using JPS measurements for the determination of proprioceptive acuity may also serve important information about the laterality effects on standing posture. The existing literature suggests that right-side dominant individuals consistently sense movements more accurately in both upper and lower extremity joints of the non-dominant left vs the right-dominant side (Roy and MacKenzie, 1978; Kurian et al., 1989; Nishizawa, 1991; Goble et al., 2006; Goble and Brown, 2007, 2008; Han et al., 2013; Negyesi et al., 2019). Therefore, we hypothesized that right-side dominant participants would have more stable standing balance when they stand on their non-dominant left vs right-dominant leg. We also expected right vs left-sided participants to have more stable standing balance during non-dominant leg stance.
Numerous parameters can characterize postural performance (Yamamoto et al., 2015). Traditional parameters are based on the motion and velocity of the CoP, usually decomposed along the AP and ML directions. Therefore, we chose to analyze a wide variety of CoP data (Table 1). However, interpretations of these parameters differ. Although high postural sway, computed as the total length of the CoP path, is often correlated with low postural stability (Paillard and Noé, 2015), reduced sway and tightening of the motion may also constitute a mechanism to handle fear of falling (Adkin et al., 2002). Average CoP velocity has been proposed as a better indicator of postural stability than CoP displacement since joint velocities are directly used by the body as postural feedback (Masani et al., 2003). Non-linear approaches, such as fractal analyses, can highlight subtle changes in the postural strategy that are not detected by the traditional linear analyses of sway and CoP velocity (Doyle et al., 2004; Noda and Demura, 2006). Notably, these non-linear analyses can assess the adaptability of a system, i.e. its capability to efficiently react to external perturbations, however, they have been scarcely applied to quantify the effects of footwear on postural control (Hausselle et al., 2021). Nevertheless, in the present study, none of the CoP measures differed significantly between left- and right-side dominant participants’ dominant and non-dominant leg during bipedal or unipedal stance. Overall, the predictive power of CoP metrics is questionable considering that perhaps CoP path data are not sensitive enough for detecting leg differences in standing stability according to previous studies using unilateral (Matsuda et al., 2008; Lopez-Fernandez et al., 2020) and bipedal (Haddad et al., 2011) stance conditions.
Kinematic data acquired by MoCap analysis revealed larger knee but lower ankle joint kinematic values for left vs right-sided participants during non-dominant stance (Table 5). This finding suggests that left and right-side dominant participants use a different balancing strategy while standing on their non-dominant leg. The lower ankle joint angular velocity, acceleration, range, and total movement converged and revealed concordant patterns with the EMG activity of their medial gastrocnemius (Table 6). These data are in line with the literature considering that the medial gastrocnemius muscle contributes to maintaining balance during unilateral stance (Tokuno et al., 2007; Hodson-Tole et al., 2013; Lima et al., 2014) and is recruited during low-intensity tasks, i.e., quiet standing (Jacono et al., 2004). Overall, our results suggest that left vs right-sided participants had more stable standing balance while standing on the non-dominant leg.
The larger medial gastrocnemius muscle activation and kinematic values of the ankle joint angle were also present when right-side dominant participants were standing on their non-dominant left vs right-dominant leg. These data suggest worse postural stability of right-as compared to left-side dominant participants during non-dominant leg stance and also compared to their own biosignal data acquired when they stood on their dominant leg. On the other hand, the lack of association between EMG data of medial gastrocnemius and the kinematic data of the ankle suggests that the larger activation of medial gastrocnemius muscle was not present due to the larger rotational movements in the ankle joint. In the present study, the activity of triceps surae was measured by the medial gastrocnemius muscle of each leg because this muscle plays a crucial role in controlling posture (Krishnamoorthy et al., 2004). However, because the soleus is also activated to control upright standing, it might have been insightful to determine its activation in left- and right-side dominant participants’ dominant and non-dominant legs. The human soleus and gastrocnemius muscles differ in many respects. The soleus is monoarticular and the gastrocnemius is biarticular. The soleus consists of ∼88% of slow-twitch muscle fibers and the gastrocnemius has ∼52% of fast-twitch muscle fibers (Johnson et al., 1973; Buchthal and Schmalbruch, 1980). Moreover, the control of soleus and gastrocnemius during gait tasks seems to belong to different task groups (Duysens et al., 1991). Thus, it was suggested that despite sharing a common distal tendon, these two muscles may have distinct functional roles (Héroux et al., 2014). Therefore, it is possible that each could affect the knee and/or ankle joint function differently in left-vs right-side dominant participants. Future studies should clarify this idea.
Less stable standing balance of left-side dominant participants during dominant leg stance
Because neurodevelopmental studies (Pompeiano, 1985; Previc, 1991) indicate left-side dominance for postural control, we expected that right hemisphere specialization may underlie proprioceptive feedback (Naito et al., 2005; Goble and Brown, 2007, 2008) not only in right but also in left-side dominant participants. This idea was also supported by findings of our previous study (Galamb et al., 2018), i.e., left-sided participants performed a target-matching task more accurately with their dominant left vs right knee joint. Therefore, we hypothesized that left-sided participants may have more stable standing balance in their dominant as compared to their non-dominant leg and also as compared to right-side dominant participants during dominant-leg stance. However, our results indicated left vs right-sided participants to have worse markers of balance i.e., larger range and total movement of knee joint (L: 7.76 ± 2.92°, R: 3.91 ± 2.38°; L: 120.64 ± 53.94°, R: 71.8 ± 36.98°; respectively) during dominant leg stance. Furthermore, larger values of kinematic data for knee joint angles were present when they stood on their dominant vs non-dominant leg consistently suggesting a more stable standing balance of left-side dominant participants during non-dominant leg stance (Table 5). However, considering that no differences in CoP or EMG data were found, the practical significance of the kinematic data is questionable especially due to the relatively low sample size. Future studies should recruit more strongly left-side dominant participants to increase the statistical power and to reveal whether between- and within-group differences could also be found in CoP and EMG data, or in line with the results of previous studies (Tokuno et al., 2007; Hodson-Tole et al., 2013; Lima et al., 2014), no differences can be found between the dominant and non-dominant limb of left-side dominant participants during unilateral stance.
Laterality effects on EMG and kinematic data during bipedal stance
Although unilateral stance is considered to be a better marker of postural control than bipedal upright standing (Vuillerme et al., 2001; Paillard et al., 2006), we also examined left- and right-side dominant participants’ balance stability during bipedal stance. It might be possible that during bipedal stance, the non-dominant leg unknowingly bears a greater portion of body weight hence determines standing stability which in turn would result in a larger CoP path, higher muscle activity, or greater range of motion of lower limb joints. However, no differences between left- and right-side dominant participants’ kinetic, kinematic, or EMG data was observed during bipedal stance. On the other hand, our results indicate that this hypothesis may apply to right-side dominant participants only considering that 87% of R but only 13% of L had greater weight-bearing on their non-dominant leg. Nevertheless, we found no differences in any outcomes between participants’ dominant and non-dominant legs during bipedal stance, regardless of group.
Limitations and future perspectives
One limitation of the present and all other studies considering laterality is the difficulty in the determination of side-dominance due to the contradictory results of functional laterality. For example, we recruited only strongly left- and strongly right-side dominant participants with a laterality index >0.9 according to the results of the Edinburgh Handedness Inventory (Oldfield, 1971) and a questionnaire for determining leg dominance (Spry et al., 1993), still, we expected right-sided participants to have more stable standing balance during non-dominant unilateral stance considering the proposed hemispheric lateralization of proprioception shown in previous neurodevelopmental (Pompeiano, 1985; Previc, 1991), neuroanatomic (Bohannon et al., 1986; Perennou et al., 2008; Duclos et al., 2015) and JPS (Roy and MacKenzie, 1978; Kurian et al., 1989; Nishizawa, 1991; Goble et al., 2006; Goble and Brown, 2007, 2008; Han et al., 2013; Negyesi et al., 2019) studies. This leads to the conclusion that hand and/or leg dominance could be defined based on given tasks of questionnaires but we expect this determination not to be universal across tasks. Future studies should consider whether they continue to determine side-dominance based on available questionnaires or rather define the participants’ dominant limb before the main experiment based on their performance in the task of interest. Also, researchers should be careful with the direct interpretation of JPS results on proprioception considering that perceptual judgments may not accurately reflect how proprioceptive signals are processed and interpreted (Brown et al., 2003; Filimon et al., 2013) or how they are linked to function (Héroux et al., 2022).
Second, we placed the bipolar EMG electrodes over each muscle belly which might not be the most sufficient method to describe overall muscle activity accurately. Future studies should provide a more comprehensive overview of muscle activity using high-density surface EMG (HD-EMG) (Drost et al., 2006) to reveal regional distribution (e.g., proximal vs distal) of muscle activity during bi- and unilateral stances. Future studies should also consider analyzing biosignal data not only in a conventional way but also using automated, intelligent, and flexible AI-based analytical procedures that solve the difficulty of discovering patterns that do not conform to the expected structure (Chandola et al., 2009). For example, anomaly detection of time series data is widely used in biomedical analyses to detect abnormal ECG (Soga et al., 2021) or EEG (Takahashi et al., 2020). We could not perform such an analysis due to our experimental setup considering that healthy participants were instructed to stand quietly and only successful trials were taken into the analyses. Future studies recruiting both healthy participants and patients with orthopedic or neuromuscular disorders should gather long-lasting time-series data with unexpected events, i.e., falls, balance problems when standing up, sitting down, or even losses of balance during walking to label the data for efficient anomaly detection. Detecting the underlying mechanism of the interaction between side-dominance and posture could serve as the basis for developing more efficient rehabilitation strategies after an injury or even after left- or right-hemisphere damage.
Overall, our results suggest that side-dominance affects biomechanical and neuromuscular control strategies during unilateral standing, which may have implications for the understanding of mechanisms for rehabilitation.
Data availability statement
The datasets generated during and analyzed during the current study are not publicly available due to the large amount of ground reaction force, EMG, and motion capture data with associated excel files but are available from the corresponding author on reasonable request.
Ethics statement
After giving both verbal and written explanations of the experimental protocol, participants signed the informed consent document in accordance with the declaration of Helsinki. The study was carried out in accordance with the recommendations of the University Ethical Committee (Approval No. TE-KEB:2:2021).
Author contributions
JN, AK, and RN designed the research. JN, BP, PK, KR and ZP performed the research. JN, BP, AK, ZW, AA, and DS analyzed the data. JN performed the statistical analyses. JN and BP directed the research. LG and RK provided the facilities. JN, TH, MV and RN wrote the manuscript and all the authors have read and approved the final version.
Funding
This work was supported by JSPS KAKENHI Grant Number 21K17600.
Acknowledgments
The authors thank all the participants for their contribution to the research. The authors also thank the Department of Mechatronics, Optics and Mechanical Engineering Informatics, Budapest University of Technology and Economics, Budapest, Hungary for providing the facilities for the experiment.
Conflict of interest
The authors declare that the research was conducted in the absence of any commercial or financial relationships that could be construed as a potential conflict of interest.
Publisher’s note
All claims expressed in this article are solely those of the authors and do not necessarily represent those of their affiliated organizations, or those of the publisher, the editors and the reviewers. Any product that may be evaluated in this article, or claim that may be made by its manufacturer, is not guaranteed or endorsed by the publisher.
Abbreviations
ANOVA, analysis of variance; AP, anterior-posterior; area_ce, 95% confidence ellipse area; area_sw, sway area; CoP, center of pressure; CNS, central nervous system; EMG, electromyography; FD, fractal dimension; GRF, ground reaction force; JPS, joint position sense; mdist, mean distance; mfreq, mean frequency; mvelo, mean velocity; ML, mediolateral; MoCap, motion capture; mpi, mean percentage index; rms, root mean square; raccel, root mean square acceleration; rvelo, root mean square velocity; SampEn, sample entropy; sd, standard deviation of MoCap data; SD, standard deviation of CoP; totmov, total movement.
References
Adkin A. L., Frank J. S., Carpenter M. G., Peysar G. W. (2002). Fear of falling modifies anticipatory postural control. Exp. Brain Res. 143 (2), 160–170. doi:10.1007/s00221-001-0974-8
Allum J. H. J., Bloem B. R., Carpenter M. G., Hulliger M., Hadders-Algra M. (1998). Proprioceptive control of posture: A review of new concepts. Gait Posture 8 (3), 214–242. doi:10.1016/S0966-6362(98)00027-7
Alradwan H., Khan M., Grassby M. H., Bedi A., Philippon M. J., Ayeni O. R. (2015). Gait and lower extremity kinematic analysis as an outcome measure after femoroacetabular impingement surgery. Arthroscopy 31 (2), 339–344. doi:10.1016/j.arthro.2014.06.016
Barrett D. S., Cobb A. G., Bentley G. (1991). Joint proprioception in normal, osteoarthritic and replaced knees. J. Bone Jt. Surg. Br. 73-B (1), 53–56. doi:10.1302/0301-620X.73B1.1991775
Bohannon R. W., Smith M. B., Larkin P. A. (1986). Relationship between independent sitting balance and side of hemiparesis. Phys. Ther. 66(6), 944–945. doi:10.1093/ptj/66.6.944
Brown L. E., Rosenbaum D. A., Sainburg R. L. (2003). Limb position drift: Implications for control of posture and movement. J. Neurophysiol. 90 (5), 3105–3118. doi:10.1152/jn.00013.2003
Buchthal F., Schmalbruch H. (1980). Motor unit of mammalian muscle. Physiol. Rev. 60 (1), 90–142. doi:10.1152/physrev.1980.60.1.90
Cappozzo A., Della Croce U., Leardini A., Chiari L. (2005). Human movement analysis using stereophotogrammetry. Part 1: Theoretical background. Gait Posture 21 (2), 186–196. doi:10.1016/j.gaitpost.2004.01.010
Chandola V., Banerjee A., Kumar V. (2009). Anomaly detection: A survey. ACM Comput. Surv. 41 (3), 1–58. Article 15. doi:10.1145/1541880.1541882
Chvatal S. A., Ting L. H. (2012). Voluntary and reactive recruitment of locomotor muscle synergies during perturbed walking. J. Neurosci. 32 (35), 12237–12250. doi:10.1523/jneurosci.6344-11.2012
Cinthuja P., Arnold G., Abboud R. J., Wang W. (2021). Analysis of the relationships between balance ability and walking in terms of muscle activities and lower limb kinematics and kinetics. Biomechanics 1 (2), 190–201. doi:10.3390/biomechanics1020016
Danna-Dos-Santos A., Degani A. M., Boonstra T. W., Mochizuki L., Harney A. M., Schmeckpeper M. M., et al. (2015). The influence of visual information on multi-muscle control during quiet stance: A spectral analysis approach. Exp. Brain Res. 233 (2), 657–669. doi:10.1007/s00221-014-4145-0
Delp S. L., Anderson F. C., Arnold A. S., Loan P., Habib A., John C. T., et al. (2007). OpenSim: Open-Source software to create and analyze dynamic simulations of movement. IEEE Trans. Biomed. Eng. 54 (11), 1940–1950. doi:10.1109/TBME.2007.901024
Dietz V., Horstmann G. A., Berger W. (1989). Significance of proprioceptive mechanisms in the regulation of stance. Prog. Brain Res. 80, 419–423. discussion 395-417. doi:10.1016/s0079-6123(08)62238-4
Doyle T. L., Dugan E. L., Humphries B., Newton R. U. (2004). Discriminating between elderly and young using a fractal dimension analysis of centre of pressure. Int. J. Med. Sci. 1 (1), 11–20. doi:10.7150/ijms.1.11
Drost G., Stegeman D. F., van Engelen B. G., Zwarts M. J. (2006). Clinical applications of high-density surface EMG: A systematic review. J. Electromyogr. Kinesiol. 16 (6), 586–602. doi:10.1016/j.jelekin.2006.09.005
Duclos N. C., Maynard L., Abbas D., Mesure S. (2015). Hemispheric specificity for proprioception: Postural control of standing following right or left hemisphere damage during ankle tendon vibration. Brain Res. 1625, 159–170. doi:10.1016/j.brainres.2015.08.043
Duysens J., Tax A., Van der Doelen B., Trippel M., Dietz V. (1991). Selective activation of human soleus or gastrocnemius in reflex responses during walking and running. Exp. Brain Res. 87 (1), 193–204. doi:10.1007/BF00228520
Faul F., Erdfelder E., Lang A. G., Buchner A. (2007). G*Power 3: A flexible statistical power analysis program for the social, behavioral, and biomedical sciences. Behav. Res. Methods 39 (2), 175–191. doi:10.3758/bf03193146
Federolf P. A. (2016). A novel approach to study human posture control: "Principal movements" obtained from a principal component analysis of kinematic marker data. J. Biomech. 49 (3), 364–370. doi:10.1016/j.jbiomech.2015.12.030
Filimon F., Philiastides M. G., Nelson J. D., Kloosterman N. A., Heekeren H. R. (2013). How embodied is perceptual decision making? Evidence for separate processing of perceptual and motor decisions. J. Neurosci. 33 (5), 2121–2136. doi:10.1523/jneurosci.2334-12.2013
Freeman M. A., Dean M. R., Hanham I. W. (1965). The etiology and prevention of functional instability of the foot. J. Bone Jt. Surg. Br. volume 47(4), 678–685. doi:10.1302/0301-620x.47b4.678
Gabbard C., Hart S. (1996). A question of foot dominance. J. Gen. Psychol. 123 (4), 289–296. doi:10.1080/00221309.1996.9921281
Galamb K., Szilagyi B., Magyar O. M., Hortobagyi T., Nagatomi R., Vaczi M., et al. (2018). Effects of side-dominance on knee joint proprioceptive target-matching asymmetries. Physiol. Int. 105 (3), 257–265. doi:10.1556/2060.105.2018.3.22
Garcia-Masso X., Pellicer-Chenoll M., Gonzalez L. M., Toca-Herrera J. L. (2016). The difficulty of the postural control task affects multi-muscle control during quiet standing. Exp. Brain Res. 234 (7), 1977–1986. doi:10.1007/s00221-016-4602-z
Geurts A. C., Nienhuis B., Mulder T. W. (1993). Intrasubject variability of selected force-platform parameters in the quantification of postural control. Arch. Phys. Med. Rehabil. 74 (11), 1144–1150.
Goble D. J., Brown S. H. (2007). Task-dependent asymmetries in the utilization of proprioceptive feedback for goal-directed movement. Exp. Brain Res. 180 (4), 693–704. doi:10.1007/s00221-007-0890-7
Goble D. J., Brown S. H. (2008). Upper limb asymmetries in the matching of proprioceptive versus visual targets. J. Neurophysiol. 99 (6), 3063–3074. doi:10.1152/jn.90259.2008
Goble D. J., Lewis C. A., Brown S. H. (2006). Upper limb asymmetries in the utilization of proprioceptive feedback. Exp. Brain Res. 168 (1-2), 307–311. doi:10.1007/s00221-005-0280-y
Goldie P. A., Bach T. M., Evans O. M. (1989). Force platform measures for evaluating postural control: Reliability and validity. Arch. Phys. Med. Rehabil. 70 (7), 510–517.
Gonzalez C. L., Goodale M. A. (2009). Hand preference for precision grasping predicts language lateralization. Neuropsychologia 47 (14), 3182–3189. doi:10.1016/j.neuropsychologia.2009.07.019
Goodale M. A. (1988). Hemispheric differences in motor control. Behav. Brain Res. 30 (2), 203–214. doi:10.1016/0166-4328(88)90149-0
Gray J. (1944). Studies in the mechanics of the tetrapod skeleton. J. Exp. Biol. 20 (2), 88–116. doi:10.1242/jeb.20.2.88
Haddad J. M., Rietdyk S., Ryu J. H., Seaman J. M., Silver T. A., Kalish J. A., et al. (2011). Postural asymmetries in response to holding evenly and unevenly distributed loads during self-selected stance. J. Mot. Behav. 43 (4), 345–355. doi:10.1080/00222895.2011.596169
Han J., Anson J., Waddington G., Adams R. (2013). Proprioceptive performance of bilateral upper and lower limb joints: side-general and site-specific effects. Exp. Brain Res. 226 (3), 313–323. doi:10.1007/s00221-013-3437-0
Hatta T. (2007). Handedness and the brain: A review of brain-imaging techniques. Magn. Reson. Med. Sci. 6 (2), 99–112. doi:10.2463/mrms.6.99
Hausselle J., Haddox A. G., Kasitz J., Azoug A. (2021). An interventional exploratory study to assess the effect of footwear on postural stability and strategy during quiet standing. Int. Biomech. 8 (1), 63–74. doi:10.1080/23335432.2021.1985610
Hendrickson J., Patterson K. K., Inness E. L., McIlroy W. E., Mansfield A. (2014). Relationship between asymmetry of quiet standing balance control and walking post-stroke. Gait Posture 39 (1), 177–181. doi:10.1016/j.gaitpost.2013.06.022
Héroux M. E., Dakin C. J., Luu B. L., Inglis J. T., Blouin J.-S. (2014). Absence of lateral gastrocnemius activity and differential motor unit behavior in soleus and medial gastrocnemius during standing balance. J. Appl. Physiol. 116 (2), 140–148. doi:10.1152/japplphysiol.00906.2013
Héroux M. E., Butler A. A., Robertson L. S., Fisher G., Gandevia S. C. (1985). Proprioception: A new look at an old concept. J. Appl. Physiol. 132 (3), 811–814. doi:10.1152/japplphysiol.00809.2021
Hodson-Tole E. F., Loram I. D., Vieira T. M. (2013). Myoelectric activity along human gastrocnemius medialis: Different spatial distributions of postural and electrically elicited surface potentials. J. Electromyogr. Kinesiol. 23 (1), 43–50. doi:10.1016/j.jelekin.2012.08.003
Hoffman M., Schrader J., Applegate T., Koceja D. (1998). Unilateral postural control of the functionally dominant and nondominant extremities of healthy subjects. J. Athl. Train. 33 (4), 319–322.
Jacono M., Casadio M., Morasso P. G., Sanguineti V. (2004). The sway-density curve and the underlying postural stabilization process. Mot. Control 8 (3), 292–311. doi:10.1123/mcj.8.3.292
Johnson M. A., Polgar J., Weightman D., Appleton D. (1973). Data on the distribution of fibre types in thirty-six human muscles: An autopsy study. J. Neurol. Sci. 18 (1), 111–129. doi:10.1016/0022-510x(73)90023-3
Kapreli E., Athanasopoulos S., Papathanasiou M., Van Hecke P., Strimpakos N., Gouliamos A., et al. (2006). Lateralization of brain activity during lower limb joints movement. An fMRI study. Neuroimage 32 (4), 1709–1721. doi:10.1016/j.neuroimage.2006.05.043
Karthick P. A., Ramakrishnan S. (2016). Surface electromyography based muscle fatigue progression analysis using modified B distribution time–frequency features. Biomed. Signal Process. Control 26, 42–51. doi:10.1016/j.bspc.2015.12.007
Khushaba R. N., Al-Timemy A. H., Al-Ani A., Al-Jumaily A. (2017). A framework of temporal-spatial descriptors-based feature extraction for improved myoelectric pattern recognition. IEEE Trans. Neural Syst. Rehabil. Eng. 25 (10), 1821–1831. doi:10.1109/TNSRE.2017.2687520
Konradsen L. (2002). Factors contributing to chronic ankle instability: Kinesthesia and joint position sense. J. Athl. Train. 37 (4), 381–385.
Krishnamoorthy V., Goodman S., Zatsiorsky V., Latash M. L. (2003a). Muscle synergies during shifts of the center of pressure by standing persons: Identification of muscle modes. Biol. Cybern. 89 (2), 152–161. doi:10.1007/s00422-003-0419-5
Krishnamoorthy V., Latash M. L., Scholz J. P., Zatsiorsky V. M. (2003b). Muscle synergies during shifts of the center of pressure by standing persons. Exp. Brain Res. 152 (3), 281–292. doi:10.1007/s00221-003-1574-6
Krishnamoorthy V., Latash M. L., Scholz J. P., Zatsiorsky V. M. (2004). Muscle modes during shifts of the center of pressure by standing persons: Effect of instability and additional support. Exp. Brain Res. 157 (1), 18–31. doi:10.1007/s00221-003-1812-y
Kurian G., Sharma N. K., Santhakumari K. (1989). Left-arm dominance in active positioning. Percept. Mot. Ski. 68, 1312–1314. doi:10.2466/pms.1989.68.3c.1312
Lepers R., Bigard A. X., Diard J. P., Gouteyron J. F., Guezennec C. Y. (1997). Posture control after prolonged exercise. Eur. J. Appl. Physiol. Occup. Physiol. 76 (1), 55–61. doi:10.1007/s004210050212
Lima B. N., Lucareli P. R. G., Gomes W. A., Silva J. J., Bley A. S., Hartigan E. H., et al. (2014). The acute effects of unilateral ankle plantar flexors static- stretching on postural sway and gastrocnemius muscle activity during single-leg balance tasks. J. Sports Sci. Med. 13 (3), 564–570.
Lopez-Fernandez J., Garcia-Unanue J., Sanchez-Sanchez J., Colino E., Hernando E., Gallardo L. (2020). Bilateral asymmetries assessment in elite and sub-elite male futsal players. Int. J. Environ. Res. Public Health 17 (9), E3169. ARTN 3169. doi:10.3390/ijerph17093169
Maki S. G. (1991). An experimental approach to the postural origins theory of neurobiological asymmetries in primates. Austin, Texas, United States: Unpublished Ph. D. dissertation, University of Texas at Austin
Masani K., Popovic M. R., Nakazawa K., Kouzaki M., Nozaki D. (2003). Importance of body sway velocity information in controlling ankle extensor activities during quiet stance. J. Neurophysiol. 90 (6), 3774–3782. doi:10.1152/jn.00730.2002
Matsuda S., Demura S., Uchiyama M. (2008). Centre of pressure sway characteristics during static one-legged stance of athletes from different sports. J. Sports Sci. 26 (7), 775–779. doi:10.1080/02640410701824099
Misiti M., Misiti Y., Oppenheim G., Poggi J.-M. (1996). Wavelet toolbox user’s guide. Natick, Massachusetts, United States: The Math Works Ins.
Naito E., Roland P. E., Grefkes C., Choi H. J., Eickhoff S., Geyer S., et al. (2005). Dominance of the right hemisphere and role of area 2 in human kinesthesia. J. Neurophysiol. 93 (2), 1020–1034. doi:10.1152/jn.00637.2004
Negyesi J., Galamb K., Szilagyi B., Nagatomi R., Hortobagyi T., Tihanyi J. (2019). Age-specific modifications in healthy adults' knee joint position sense. Somatosens. Mot. Res. 36, 262–269. doi:10.1080/08990220.2019.1684888
Nishizawa S. (1991). Different pattern of hemisphere specialization between identical kinesthetic spatial and weight discrimination tasks. Neuropsychologia 29 (4), 305–312. doi:10.1016/0028-3932(91)90044-9
Noda M., Demura S. (2006). Comparison of quantitative analysis and fractal analysis of center of pressure based on muscle fatigue. Percept. Mot. Ski. 102 (2), 529–542. doi:10.2466/pms.102.2.529-542
Oldfield R. C. (1971). The assessment and analysis of handedness: The Edinburgh inventory. Neuropsychologia 9 (1), 97–113. doi:10.1016/0028-3932(71)90067-4
Paillard T., Noé F. (2015). Techniques and methods for testing the postural function in healthy and pathological subjects. Biomed. Res. Int. 2015, 891390. doi:10.1155/2015/891390
Paillard T., Noe F., Riviere T., Marion V., Montoya R., Dupui P. (2006). Postural performance and strategy in the unipedal stance of soccer players at different levels of competition. J. Athl. Train. 41 (2), 172–176.
Papegaaij S., Hortobágyi T. (2017). “Age-related changes in the neural control of standing balance,” in Locomotion and posture in older adults: The role of aging and movement disorders. Editors F. A. Barbieri, and R. Vitório (Cham: Springer International Publishing), 427–444.
Peat J. K., Barton B., Elliott E. J. (2008). Statistics workbook for evidence-based healthcare. Malden, Mass: Blackwell.
Perelle I. B., Ehrman L. (2005). On the other hand. Behav. Genet. 35 (3), 343–350. doi:10.1007/s10519-005-3226-z
Perennou D. A., Mazibrada G., Chauvineau V., Greenwood R., Rothwell J., Gresty M. A., et al. (2008). Lateropulsion, pushing and verticality perception in hemisphere stroke: A causal relationship? Brain 131, 2401–2413. doi:10.1093/brain/awn170
Phinyomark A., Phukpattaranont P., Limsakul C. (2012a). Feature reduction and selection for EMG signal classification. Expert Syst. Appl. 39 (8), 7420–7431. doi:10.1016/j.eswa.2012.01.102
Phinyomark A., Phukpattaranont P., Limsakul C. (2012b). Fractal analysis features for weak and single-channel upper-limb EMG signals. Expert Syst. Appl. 39 (12), 11156–11163. doi:10.1016/j.eswa.2012.03.039
Phinyomark A., Quaine F., Charbonnier S., Serviere C., Tarpin-Bernard F., Laurillau Y. (2014). Feature extraction of the first difference of EMG time series for EMG pattern recognition. Comput. Methods Programs Biomed. 117 (2), 247–256. doi:10.1016/j.cmpb.2014.06.013
Pompeiano O. (1985). “Experimental central nervous system lesions and posture,” in Vestibular and visual control on posture and locomotor equilibrium (Basel, Switzerland: Karger Publishers), 218–231.
Previc F. H. (1991). A general theory concerning the prenatal origins of cerebral lateralization in humans. Psychol. Rev. 98 (3), 299–334. doi:10.1037/0033-295x.98.3.299
Prieto T. E., Myklebust J. B., Hoffmann R. G., Lovett E. G., Myklebust B. M. (1996). Measures of postural steadiness: Differences between healthy young and elderly adults. IEEE Trans. Biomed. Eng. 43 (9), 956–966. doi:10.1109/10.532130
Richman J. S., Moorman J. R. (2000). Physiological time-series analysis using approximate entropy and sample entropy. Am. J. Physiol. Heart Circ. Physiol. 278 (6), H2039–H2049. doi:10.1152/ajpheart.2000.278.6.H2039
Rocchi L., Chiari L., Cappello A. (2004). Feature selection of stabilometric parameters based on principal component analysis. Med. Biol. Eng. Comput. 42 (1), 71–79. doi:10.1007/bf02351013
Roggio F., Ravalli S., Maugeri G., Bianco A., Palma A., Di Rosa M., et al. (2021). Technological advancements in the analysis of human motion and posture management through digital devices. World J. Orthop. 12 (7), 467–484. doi:10.5312/wjo.v12.i7.467
Rougier P., Genthon N. (2009). Dynamical assessment of weight-bearing asymmetry during upright quiet stance in humans. Gait Posture 29 (3), 437–443. doi:10.1016/j.gaitpost.2008.11.001
Roy E. A., MacKenzie C. (1978). Handedness effects in kinesthetic spatial location judgements. Cortex. 14 (2), 250–258. doi:10.1016/s0010-9452(78)80051-3
Sainburg R. L. (2014). Convergent models of handedness and brain lateralization. Front. Psychol. 5, 1092. doi:10.3389/fpsyg.2014.01092
Samuel O. W., Zhou H., Li X., Wang H., Zhang H., Sangaiah A. K., et al. (2018). Pattern recognition of electromyography signals based on novel time domain features for amputees' limb motion classification. Comput. Electr. Eng. 67, 646–655. doi:10.1016/j.compeleceng.2017.04.003
Sartarelli M. (2016). Handedness, earnings, ability and personality. Evidence from the lab. PLoS One 11 (10), e0164412. doi:10.1371/journal.pone.0164412
Schurr S. A., Marshall A. N., Resch J. E., Saliba S. A. (2017). Two-dimensional video analysis is comparable to 3d motion capture in lower extremity movement assessment. Int. J. Sports Phys. Ther. 12 (2), 163–172.
Seth A., Hicks J. L., Uchida T. K., Habib A., Dembia C. L., Dunne J. J., et al. (2018). OpenSim: Simulating musculoskeletal dynamics and neuromuscular control to study human and animal movement. PLoS Comput. Biol. 14 (7), e1006223. doi:10.1371/journal.pcbi.1006223
Soga N., Sato S., Nakahara H. (2021). Energy-efficient ECG signals outlier detection hardware using a sparse robust deep autoencoder. IEICE Trans. Inf. Syst. E104.D (8), 2020LOP0011–1129. doi:10.1587/transinf.2020LOP0011
Spry S., Zebas C., Visser M. (1993). “What is leg in Biomechanics in sport XI. Proceedings of the XI symposium of the international society of biomechanics in sports. Editor J. Hamill (MA: Amherst: University of Massachusett).
Stone K. D., Bryant D. C., Gonzalez C. L. (2013). Hand use for grasping in a bimanual task: Evidence for different roles? Exp. Brain Res. 224 (3), 455–467. doi:10.1007/s00221-012-3325-z
Sun R., Moon Y., McGinnis R., Seagers K., Motl Robert W., Sheth N., et al. (2018). Assessment of postural sway in individuals with multiple sclerosis using a novel wearable inertial sensor. Digit. Biomark. 2, 1–10. doi:10.1159/000485958
Takahashi H., Emami A., Shinozaki T., Kunii N., Matsuo T., Kawai K. (2020). Convolutional neural network with autoencoder-assisted multiclass labelling for seizure detection based on scalp electroencephalography. Comput. Biol. Med. 125, 104016. doi:10.1016/j.compbiomed.2020.104016
Tokuno C. D., Carpenter M. G., Thorstensson A., Garland S. J., Cresswell A. G. (2007). Control of the triceps surae during the postural sway of quiet standing. Acta Physiol. 191 (3), 229–236. doi:10.1111/j.1748-1716.2007.01727.x
Toledo-Pérez D. C., Rodríguez-Reséndiz J., Gómez-Loenzo R. A. (2020). A study of computing zero crossing methods and an improved proposal for EMG signals. IEEE Access 8, 8783–8790. doi:10.1109/ACCESS.2020.2964678
Too J., Rahim A., Mohd N. S. (2019). Classification of hand movements based on discrete wavelet transform and enhanced feature extraction. Int. J. Adv. Comput. Sci. Appl. 10 (6), 83–89. doi:10.14569/IJACSA.2019.0100612
Torres-Oviedo G., Ting L. H. (2007). Muscle synergies characterizing human postural responses. J. Neurophysiol. 98 (4), 2144–2156. doi:10.1152/jn.01360.2006
Verma A. R., Gupta B. (2019). Detecting neuromuscular disorders using EMG signals based on TQWT features. Augment. Hum. Res. 5 (1), 8. doi:10.1007/s41133-019-0020-7
Vidakovic B., Mueller P. (1994). Wavelets for kids. Madrid, Spain: Instituto de Estadística, Universidad de Duke, 161.
Vuillerme N., Danion F., Marin L., Boyadjian A., Prieur J. M., Weise I., et al. (2001). The effect of expertise in gymnastics on postural control. Neurosci. Lett. 303(2), 83–86. doi:10.1016/S0304-3940(01)01722-0
Vuoksimaa E., Koskenvuo M., Rose R. J., Kaprio J. (2009). Origins of handedness: A nationwide study of 30, 161 adults. Neuropsychologia 47 (5), 1294–1301. doi:10.1016/j.neuropsychologia.2009.01.007
Waris A., Kamavuako E. N. (2018). Effect of threshold values on the combination of EMG time domain features: Surface versus intramuscular EMG. Biomed. Signal Process. Control 45, 267–273. doi:10.1016/j.bspc.2018.05.036
Winter D. A., Patla A. E., Ishac M., Gage W. H. (2003). Motor mechanisms of balance during quiet standing. J. Electromyogr. Kinesiol. 13 (1), 49–56. doi:10.1016/S1050-6411(02)00085-8
Winter D. A. (1995). Human balance and posture control during standing and walking. Gait Posture 3 (4), 193–214. doi:10.1016/0966-6362(96)82849-9
Keywords: balance, hand dominance, laterality, leg-dominance, motion capture, posture
Citation: Négyesi J, Petró B, Salman DN, Khandoker A, Katona P, Wang Z, Almaazmi AISQ, Hortobágyi T, Váczi M, Rácz K, Pálya Z, Grand L, Kiss RM and Nagatomi R (2022) Biosignal processing methods to explore the effects of side-dominance on patterns of bi- and unilateral standing stability in healthy young adults. Front. Physiol. 13:965702. doi: 10.3389/fphys.2022.965702
Received: 10 June 2022; Accepted: 09 August 2022;
Published: 16 September 2022.
Edited by:
Giuseppe De Vito, University of Padua, ItalyReviewed by:
Lorenzo Rum, Foro Italico University of Rome, ItalyLior Shamir, Kansas State University, United States
Copyright © 2022 Négyesi, Petró, Salman, Khandoker, Katona, Wang, Almaazmi, Hortobágyi, Váczi, Rácz, Pálya, Grand, Kiss and Nagatomi. This is an open-access article distributed under the terms of the Creative Commons Attribution License (CC BY). The use, distribution or reproduction in other forums is permitted, provided the original author(s) and the copyright owner(s) are credited and that the original publication in this journal is cited, in accordance with accepted academic practice. No use, distribution or reproduction is permitted which does not comply with these terms.
*Correspondence: János Négyesi, negyesi@tohoku.ac.jp
†ORCID: János Négyesi, orcid.org/0000-0001-5055-3242; Báliant Petró, orcid.org/0000-0003-3920-5161; Ahsan Khandoker, orcid.org/0000-0002-0636-1646; Ziheng Wang, orcid.org/0000-0002-3953-0493; Tibor Hortobágyi, orcid.org/0000-0001-5732-7942; Kristóf Rácz, orcid.org/0000-0001-5427-1787; Zsófia Pálya, orcid.org/0000-0002-6295-3160; László Grand, orcid.org/0000-0003-3607-8435; Rita M. Kiss, orcid.org/0000-0001-5391-3266; Ryoichi Nagatomi, orcid.org/0000-0003-3038-7202