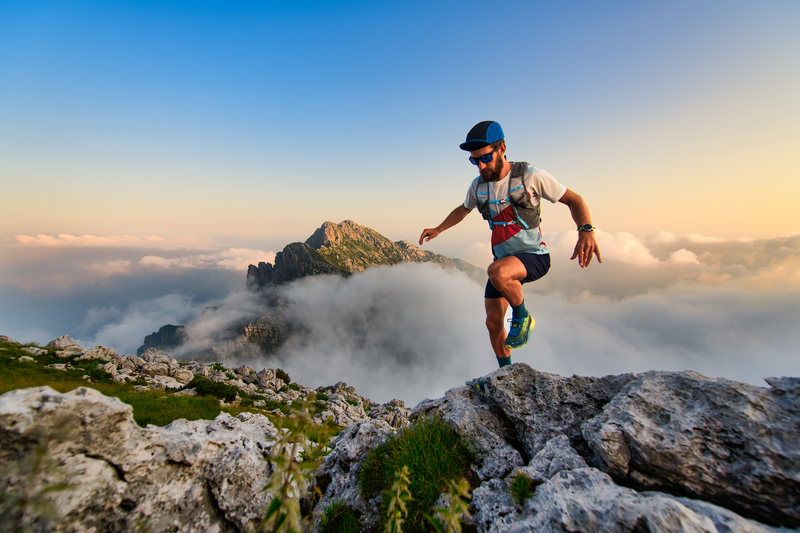
94% of researchers rate our articles as excellent or good
Learn more about the work of our research integrity team to safeguard the quality of each article we publish.
Find out more
ORIGINAL RESEARCH article
Front. Physiol. , 08 August 2022
Sec. Invertebrate Physiology
Volume 13 - 2022 | https://doi.org/10.3389/fphys.2022.954228
Manipulating the hypomagnetic field (HMF), which is the absence or significant weakening (<5 μT) of the geomagnetic field (GMF), offers a unique tool to investigate magnetic field effects on organismal physiology, development, behavior and life history. Reverse transcription quantitative polymerase chain reaction (RT-qPCR) has been utilized to study changes in gene expression associated with exposure to the HMF. However, selecting appropriate reference genes (RGs) with confirmed stable expression across environments for RT-qPCR is often underappreciated. Using three algorithms (BestKeeper, NormFinder, and GeNorm), we investigated the expression stability of eight candidate RGs when exposed to the HMF condition versus local GMF during developmental from juveniles to adults in the migratory insect pest, the brown planthopper Nilaparvata lugens. During the nymphal stage, RPL5 & α-TUB1, EF1-α & ARF1, RPL5 & AK, EF1-α & RPL5, and ARF1 & AK were suggested as the most stable RG sets in the 1st to 5th instars, respectively. For 1- to 3-day-old adults, AK & ARF1, AK & α-TUB1, AK & ARF1 and EF1-α & RPL5, AK & α-TUB1, AK & EF1-α were the optimal RG sets for macropterous and brachypterous females, respectively. ACT1 & RPL5, RPL5 & EF1-α, α-TUB1 & ACT1 and EF1-α & RPL5, ARF1 & ACT1, ACT1 & ARF1 were the optimal RG sets for macropterous and brachypterous males, respectively. These results will facilitate accurate gene expression analyses under the HMF in N. lugens. The verification approach illustrated in this study highlights the importance of identifying reliable RGs for future empirical studies of magnetobiology (including magnetoreception) that involve magnetic field intensity as a factor.
The geomagnetic field (GMF) provides organisms with protection from solar wind and cosmic radiation, making the Earth hospitable. Living organisms on Earth are immersed in and interact with the GMF. Many animals exploit the vector GMF for orientation and navigation, which is achieved by magnetoreception (Lohmann, 2010; Mouritsen, 2018; Kishkinev et al., 2021; Wynn et al., 2022). Three potential mechanisms, including radical-pair-based quantum compass(Hore and Mouritsen, 2016; Wan et al., 2021; Xu et al., 2021), magnetite-based mechanisms(Kirschvink, 2001; Monteil and Lefevre, 2020) and iron-sulfur cluster assembly 1 (IscA1 or MagR)-Cryptochrome (Cry) magnetosensing complex model (Qin et al., 2016), have received the most attention to date in attempting to explain this enigmatic process.
In addition to magnetoreception which normally functions under the typically-experienced physiological GMF that ranges in strength from ∼ 24 to 66 μT (Alken et al., 2021), bioeffects induced by magnetic fields outside this intensity range on organisms have also been extensively explored (Miyakoshi, 2005; Saunders, 2005; Ghodbane et al., 2013; Zhang et al., 2017; Tian and Pan, 2018; Binhi and Rubin, 2022). A magnetic field that is significantly reduced is usually termed a hypomagnetic field (HMF). An HMF can be found naturally on some planets or satellites (such as Venus, Mars, and the Earth’s moon) (Svedhem et al., 2007; Watters et al., 2007; Berguig et al., 2011) and in the interplanetary space of the solar system. Moreover, it can be artificially achieved on Earth by GMF compensation or shielding strategy using a coils system or high-permeability magnetic material (such as mu-metal or permalloy), respectively. The manipulated HMF is frequently used in mimicking magnetic field intensity during deep space flight or celestial exploration (Binhi and Prato, 2017; Zhang Z. et al., 2021), working as the sham or manipulated treatment group for research into bioeffects induced by changes in field intensity, magnetoreception mechanisms (Fedele et al., 2014a; Binhi and Prato, 2017) and paleomagnetic studies (Qin et al., 2020). Bioeffects of HMFs have been a topic of considerable investigation (Wan et al., 2014; Wan et al., 2015; Binhi and Prato, 2017; Tian and Pan, 2018; Wan et al., 2020b; Zhang and Tian, 2020; Zhang Z. et al., 2021; Xue et al., 2021; Binhi and Rubin, 2022), with demonstrated effects on embryogenesis (Fesenko et al., 2010), development (Mo et al., 2012), reproduction (Wan et al., 2014; Wan et al., 2015), cytoskeleton structure (Mo et al., 2016), nervous system dysfunction and related behavioral outputs (Choleris et al., 2002; Zhang et al., 2004; Binhi and Sarimov, 2009), circadian clockwork (Bliss and Heppner, 1976; Fedele et al., 2014a), migratory regulation (Wan et al., 2015; Wan et al., 2016), and reactive oxygen species levels (Sherrard et al., 2018; Zhang B. et al., 2021). However, the specific mechanisms and signaling pathways underlying phenotypic responses to the HMF remain poorly understood (Binhi and Prato, 2017; 2018).
Gene expression analyses have provided insight into the complex regulatory architecture underlying HMF-triggered bioeffects (Xu et al., 2012; Fedele et al., 2014a; Mo et al., 2014; Wan et al., 2014; Wan et al., 2015; Bae et al., 2016; Fu et al., 2016; Mo et al., 2016; Wan et al., 2016; Agliassa et al., 2018; Agliassa and Maffei, 2019; Zhang B. et al., 2021; Yan et al., 2021) and magnetoreception (Yoshii et al., 2009; Gegear et al., 2010; Xu et al., 2012; Fedele et al., 2014a; Fedele et al., 2014b; Mo et al., 2014; Wan et al., 2014; Wan et al., 2015; Bae et al., 2016; Bazalova et al., 2016; Fu et al., 2016; Mo et al., 2016; Wan et al., 2016; Fitak et al., 2017; Agliassa et al., 2018; Gunther et al., 2018; Wang et al., 2018; Agliassa and Maffei, 2019; Wan et al., 2020a; Hochstoeger et al., 2020; Zhang B. et al., 2021; Gao et al., 2021; Wan et al., 2021; Yan et al., 2021). Reverse transcription quantitative polymerase chain reaction (RT-qPCR) is a powerful tool commonly employed to detect mRNA transcription levels (Derveaux et al., 2010). Accurate normalization is a vital prerequisite for biologically-relevant gene expression analysis. Since no single gene can be used as an internal control under all environmental conditions, the expression stability of the intended reference genes (RGs) has to be verified across environments before use in a formal experiment (Andersen et al., 2004). Uncontrolled variation in detected mRNA amounts can arise due to many factors including tissue type, sampling protocol, total RNA extraction and reverse-transcription efficacy, with unreliable RGs leading to poor reproducibility in genetic and gene expression studies of magnetobiology (Ponton et al., 2011). Insects, with relatively short generation times and powerful molecular toolboxes [e.g., fruit fly (Gegear et al., 2008; Yoshii et al., 2009; Gegear et al., 2010; Foley et al., 2011; Fedele et al., 2014a; Fedele et al., 2014b), monarch butterfly (Gegear et al., 2010; Wan et al., 2021), termite (Gao et al., 2021), cockroach (Bazalova et al., 2016), firebug (Netusil et al., 2021), rice planthopper (Wan et al., 2014; Wan et al., 2015; Wan et al., 2016; Wan et al., 2020a; Zhang Y. et al., 2020; Wan et al., 2020b)], provide excellent models for studying the gene regulatory networks mediating biological responses to changes in magnetic field intensity or direction. However, studies assessing the stability of RGs prior to conducting gene expression analyses in insect magnetobiology studies are rare (Liu et al., 2019).
Eukaryotic elongation factor 1-α (EF1-α), 18S ribosomal RNA (18S), actin (ACT), ADP-ribosylation factor (ARF), ribosomal protein S (RPS), tubulin (TUB), arginine kinase (AK) and ribosomal protein L (RPL) genes are frequently chosen as internal references (Yuan et al., 2014; Wan et al., 2015; Lu et al., 2018; Wan et al., 2020a) in insect gene expression analyses. The brown planthopper, Nilaparvata lugens, a notorious rice pest, exhibits a partial seasonal migration strategy (Menz et al., 2019). Adult N. lugens exhibit environmentally-determined wing dimorphism consisting of macropterous migrants and brachypterous residents with enhanced fecundity (Cheng et al., 2003; Guerra, 2011). The candidate magnetite crystals (Pan et al., 2016), as well as putative essential genes in animal magnetoreception [i.e., Cry1, Cry2 (Xu et al., 2016), and IscA1 (Xu et al., 2019)] involved in the development of these alternative migratory phenotypes, have all been explored. In addition, previous studies have shown migration-related magnetoresponses of nymphal and 1- to 3-day-old N. lugens to variation in GMF intensity (Wan et al., 2014; Wan et al., 2020a; Zhang Y. et al., 2020; Zhang and Pan, 2021) [from HMF to moderate magnetic field intensity (Zhang et al., 2017)], establishing N. lugens, for which a high-quality genome is available, as a promising unconventional model for magnetobiology (including magnetoreception) study. Therefore, in this study we aimed to investigate the expression stability of eight candidate RGs including EF1-α, 18S, ACT1, ARF1, RPS15, α-TUB1, AK and RPL5, with three commonly used normalization algorithms [BestKeeper (Pfaffl et al., 2004), NormFinder (Andersen et al., 2004), GeNorm (Vandesompele et al., 2002)]. We assessed the expression of these potential RGs from the nymphal to the adult stage (including specific developmental stage, sex, and wing morph) of N. lugens exposed to HMF (versus the local GMF). We provide the first report to our knowledge of a systematic evaluation with follow-up validation of the reliability of RGs for use in gene expression pathway explorations of magnetobiology (including magnetoreception).
N. lugens were originally collected from paddy fields (32°01′50″N, 118°52′25″E) at Nanjing, Jiangsu province of China, during their migration season (mid-to-late July), and were housed indoors to establish a lab colony on susceptible Taichung Native 1 rice seedlings under a 14-h light: 10-h dark (LD) cycle at 26°C and 70%–80% relative humidity (all following assays were under the same environmental conditions except for magnetic fields). The colony was maintained under the local geomagnetic field condition before they were allocated to the experimental magnetic field groups.
The geomagnetic field (GMF) intensity at Earth’s surface generally ranges from ∼24 to 66 μT according to the thirteenth generation of the International Geomagnetic Reference Field (Alken et al., 2021). In this experiment, two three-axis DC-type Helmholtz coil systems (external diameter: 1200 mm) were used to mimic the local GMF (mean ± SD; 50000 ± 266 nT) at Nanjing city (32°3′42″N, 118°46′40″E) and the hypomagnetic field (HMF) (mean ± SD; 523 ± 29 nT) at approximately the same inclination and declination within the effective homogeneous areas of 300 mm × 300 mm × 300 mm (<2% heterogeneity). A Faraday cage inside each coil was used to shield the experimental insects from potential anthropogenic electromagnetic noise. The magnetic field parameters were measured and adjusted daily with a fluxgate magnetometer (Model 191A, HONOR TOP Magnetoelectric Technology Co., Ltd., Qingdao, China). The two groups were located in the same room as we did before to secure uniform environmental factors except for magnetic fields (Wan et al., 2020a; Wan et al., 2020b).
Following an established rearing protocol, brown planthoppers, N. lugens, were exposed to the HMF versus local GMF treatments from mated F0 females to 3-day-old F1 adults (Wan et al., 2016) that were used in the study. The individuals were maintained under corresponding magnetic conditions throughout the experiments and sampling before being quickly killed in liquid nitrogen for total RNA isolation.
Total RNA was isolated from eight biologically independent pools, each containing five heads of nymphs or adults for each group divided by developmental stage, sex, wing morph and magnetic field intensity. With TRIzol® (Invitrogen; Thermo Fisher Scientific, Inc., Waltham, MA, United States), RNA was extracted from these pooled samples. The quality and quantity of isolated RNA samples were individually analyzed using a NanoDrop 2000 (Thermo Fisher Scientific, Inc., Waltham, MA, United States). Before reverse transcription, each total RNA sample was checked again through electrophoresis in 1% agarose gels. cDNA was synthesized from 100 ng of total RNA in a 20 μl reaction using the PrimeScript RT reagent kit supplemented with a gDNA Eraser (Takara Bio Inc., Dalian, China).
A total of eight candidate N. lugens genes, including EF1-α, 18S, ACT1, ARF1, RPS15, α-TUB1, AK and RPL5, were selected as candidate reference genes and their nucleotide sequences were obtained from the GenBank. Primers specific to each gene were designed individually using the Oligo 7 software (Molecular Biology Insights, Inc., Cascade, CO, United States). The synthesis of primers was completed by GeneScript Biotechnology Co., Ltd. (China). A standard curve was generated from a 5-fold dilution of cDNA in a RT-qPCR assay. The PCR efficiency (E) and the correlation coefficient (R2) were calculated using the slope of the standard curve according to the equation E = [10−1∕slope−1] × 100%. Primer specificity was confirmed using melting-curve analysis after RT-qPCR and gel electrophoresis analysis (1.5%) of the amplicon. Primers and amplicon characteristics of the eight candidate reference genes are shown in Table 1.
RT-qPCR was performed with an Applied Biosystems® QuantStudio™ 5 Flex Real-Time PCR System (Thermo Fisher Scientific, Inc., Waltham, MA, United States) using SYBR Premix Ex Taq (Tli RNaseH Plus; Takara Bio Inc., Dalian, China), and reactions were conducted in a final volume of 20 μl (including 2 μl of a 1/20 dilution of the cDNA template and primers in a final concentration of 200 nM). Amplifications were performed with an initial 30 s step of 95°C followed by 40 denaturation cycles at 95°C for 5 s and primer annealing at 60°C for 34 s. The melting curve was generated ranging from 60 to 95°C (95°C 15 s; 60°C 1 min, 95°C 15 s).
To evaluate the stability of selected candidate reference genes, BestKeeper, NormFinder, and GeNorm were first employed. For BestKeeper, we mainly adopted SD (the cut-off value of 1) and the coefficient of variance (CV) of the mean Ct values for RG stability evaluation (Pfaffl et al., 2004; Yuan et al., 2014; Wu et al., 2021). NormFinder evaluates the stability of RGs based on intra- and inter-group expression variation and ranks the candidates by the stability values (SV) (Andersen et al., 2004). GeNorm uses the average expression stability (M; the cut-off value of 1.5) to determine the stability of candidate RGs (Vandesompele et al., 2002). According to the original publications of the three algorithms and following practices, stable RGs generally have lower M, SV and SD values (Pfaffl et al., 2004; Yuan et al., 2014; Wu et al., 2021). Thus, a comprehensive ranking was further generated based on the results derived from the geometric mean of these three algorithms (Niu et al., 2015). Besides, GeNorm was also used to determine the optimal number of reference genes. The pairwise variations (Vn/n + 1) were calculated between normalization factors NFn and NFn + 1. The Vn/n + 1 value below 0.15 indicates that the addition of the n + 1 RG makes no significant contribution to the normalization (Vandesompele et al., 2002).
To verify the reliability of the selected reference genes, the relative expression levels of the Facilitated trehalose transporter Tret1 (TRET1), a conserved transporter for trehalose in insects (Kikawada et al., 2007; Kanamori et al., 2010), were analyzed in 2-day-old brachypterous adults normalized to the reference genes evaluated in this work, including the suggested stable RG (s) (individually or in combination) as well as the least stable RG under HMF versus local GMF. The fold change in gene expression was calculated by the 2−ΔΔCt method (Livak and Schmittgen, 2001). The one-way ANOVA was applied to compare the means of the HMF and GMF at α = 0.05. Effect sizes were estimated using partial η2 (small effect: partial η2 = 0.01; medium effect: partial η2 = 0.06; large effect: partial η2 = 0.14) based on the benchmarks of Cohen (Cohen, 2013).
All primers of the eight candidate reference genes (RGs) (EF1-α, 18S, ACT1, ARF1, RPS15, α-TUB1, AK, and RPL5) showed good amplification specificity with a single amplicon of the expected size by agarose gel electrophoresis analysis of the RT-qPCR products (Supplementary Figure 1A), and with a specific single peak by melting curve analysis (Supplementary Figure 1B). The RT-qPCR efficiency for all eight candidates ranged from 106.64% (AK) to 114.98% (RPS15), and the correlation coefficients (R2) varied from 0.986 (RPS15) to 0.999 (EF1-α and ACT1) (Table 1).
To assess the stability of the eight candidate RGs across all experimental samples (1st to 5th instar nymphs and 1- to 3-day-old female and male adults with different wing morphs under the HMF versus local GMF), their transcript abundances were determined by the mean threshold cycle (Ct) values through RT-qPCR. As shown in Figure 1, the Ct values of the eight reference genes range from 15.90 to 33.65 (mean Ct, 18.69–29.61). Among them, RPS15 showed the poorest expression, with the highest mean Ct (29.61) and standard deviation (SD, 2.16) values. ACT was the most abundantly expressed gene, with the lowest average Ct and the second-lowest SD (16.85 ± 0.78). EF1-α had the lowest SD value (0.66) with a modest mean Ct of 20.98. Moreover, the variability ranking of all genes based on the SD was as follows: EF1-α < ACT1 < RPL5 < α-TUB1 < ARF < 18S < AK < RPS15 (Supplementary Table 1), indicating that the transcript expressions of the candidate RGs varied considerably across experimental samples. Thus, it would be essential to select the most reliable RGs for organisms with specific traits (including specific developmental stage, sex and wing morph) under the HMF versus local GMF to ensure accurate gene expression analysis.
FIGURE 1. Expression profile of the eight candidate reference genes in all 272 samples under the hypomagnetic field versus local geomagnetic field. The cycle threshold (Ct) distribution is presented as box plots (median, centerline; mean, plus symbol; interquartile range (IQR), box; maximum and minimum values, whiskers).
Before the ranking evaluation, GeNorm was used to determine the optimal number of candidate RGs according to the pairwise variation (Vn/n+1, the cut-off value of 0.15) of normalization factors (Vandesompele et al., 2002). The pairwise variation value below 0.15 indicates that the addition of the n+1 RG makes no significant contribution to the normalization. As depicted in Figure 2, two reference genes (V2/3 < 0.15) were sufficient for accurate normalization of gene expression in the specific developmental stage (1st to 5th instar, 1- to 3-day-old adult), sex (female and male adult), and wing morph (macropterous and brachypterous adults) of N. lugens exposed to the HMF condition (versus local GMF).
FIGURE 2. The optimal number of reference genes required for accurate normalization of gene expression of N. lugens under the hypomagnetic field versus local geomagnetic field by GeNorm. The pairwise variations (Vn/n + 1) were calculated between normalization factors NFn and NFn + 1. The Vn/n + 1 value below 0.15 indicates that the addition of the n + 1 reference gene makes no significant contribution to the normalization.
The expression levels of the eight candidate RGs of N. lugens with specific traits (including developmental stage, sex and wing morph) exposed to the HMF condition (versus local GMF) were first determined by RT-qPCR and then the BestKeeper, NormFinder, GeNorm as well as comprehensive ranking algorithms were applied to seek the optimal RG(s) in each experimental group. According to the results in Section 2.2, the first two most stable RGs were regarded as the reliable RG combination for accurate gene expression analyses in the corresponding experimental group.
For the 1st to 5th instar nymphs, the top two stable RGs under the HMF treatment (versus local GMF) were respectively RPL5 & α-TUB1 (Figure 3D), EF1-α & ARF1 (Figure 3H), RPL5 & AK (Figure 3L), EF1-α & RPL5 (Figure 3P) and ARF1 & AK (Figure 3T), while RPS15 & ARF1 (Figure 3D), 18S & RPS15 (Figure 3H), RPS15 & α-TUB1 (Figure 3L), RPS15 & 18S (Figure 3P) and α-TUB1 & ACT1 (Figure 3T) were, respectively, the two least stable RGs based on BestKeeper, NormFinder, and GeNorm algorithms.
FIGURE 3. Expression stability evaluation of candidate reference genes respectively for 1st to 5th instar nymphs of N. lugens by BestKeeper, NormFinder, GeNorm and comprehensive analyses. Each row of the panel indicates that the experimental samples are respectively from 1st (A–D), 2nd (E–H), 3rd (I–L), 4th (M–P) and 5th (Q–T) instar nymphs under the hypomagnetic field versus local geomagnetic field. The standard deviation (SD) and coefficient of variation (CV) were given by BestKeeper (A,E,I,M,Q). The stability value (SV) was given by NormFinder (B,F,J,N,R). The average expression stability (M) was given by GeNorm (C,G,K,O,S). The comprehensive ranking was further generated based on the results derived from the geometric mean (GM) of these three algorithms (D,H,L,P,T). Stable reference genes generally have lower SD, SV, M and GM values.
In the 1st instar nymphs, expression stability of RPL5 was ranked top three by all the three algorithms, while α-TUB1 ranked 5th by SD value derived from BestKeeper (Figures 3A–C). EF1-α & ARF1 all ranked top three by the three algorithms in the 2nd instar nymphs (Figures 3E–G). RPL5 ranked top two by all the three algorithms, however, AK only ranked the second least stable based on the SD value of BestKeeper in the 3rd instar nymphs (Figures 3I–K). The three algorithms all rated EF1-α & RPL5 as the two most reliable RGs in the 4th instar nymphs (Figures 3M–O). However, in the 5th instar nymphs, inconsistent with the other two algorithms, AK only ranked as the 6th most stable RG according to the SD value by BestKeeper (Figures 3Q–S).
For the 1- to 3-day-old macropterous female adults, the top two stable RG combinations under the HMF treatment (versus local GMF) were respectively AK & ARF1 (Figure 4D), AK & α-TUB1 (Figure 4H) and AK & ARF1 (Figure 4L), while RPS15 & ACT1 (Figure 4D), RPS15 & 18S (Figure 4H) and RPS15 & 18S (Figure 4L) were respectively the two least stable RGs across the same time period evaluated by comprehensive analyses based on BestKeeper, NormFinder, and GeNorm algorithms. Moreover, for the 1- to 3-day-old brachypterous female adults, the top two stable RGs under the HMF treatment (versus local GMF) were respectively EF1-α & RPL5 (Figure 4P), AK & α-TUB1 (Figure 4T) and AK & EF1-α (Figure 4X), while ARF1 & ACT1 (Figure 4P), RPS15 & 18S (Figure 4T) and RPS15 & 18S (Figure 4X) were respectively the two least stable RGs evaluated across the same time period by comprehensive analyses.
FIGURE 4. Expression stability evaluation of candidate reference genes respectively for 1- to 3-day-old macropterous and brachypterous female adults of N. lugens by BestKeeper, NormFinder, GeNorm and comprehensive analyses. Each row of the panel indicates that the experimental samples are from 1-day-old (1d), 2-day-old (2d) and 3-day-old (3d) macropterous [1d, (A–D); 2d, (E–H); 3d, (I–L)] and brachypterous [1d, (M–P); 2d, (Q–T); 3d, (U–X)] female adults under the hypomagnetic field versus local geomagnetic field. The standard deviation (SD) and coefficient of variation (CV) were given by BestKeeper (A,E,I,M,Q,U). The stability value (SV) was given by NormFinder (B,F,J,N,R,V). The average expression stability (M) was given by GeNorm (C,G,K,O,S,W). The comprehensive ranking was further generated based on the results derived from the geometric mean (GM) of these three algorithms (D,H,L,P,T,X). Stable reference genes generally have lower SD, SV, M and GM values.
For the macropterous females, AK & ARF1 ranked top 50% out of the eight candidates according to NormFinder and GeNorm algorithms, but were ranked poorly based on SD value of BestKeeper in 1-day-old adults (Figures 4A–C). AK & α-TUB1 all ranked top 50% out of the eight candidates by the three algorithms in 2-day-old adults (Figures 4E–G). In the 3-day-old adults, AK & ARF1 ranked top 50% in the stability evaluation by both BestKeeper and GeNorm, while ARF1 ranked sixth out of eight based on NormFinder (Figures 4I–K). For the brachypterous females, EF1-α & RPL5 and AK & EF1-α all ranked top 50% in the expression stability evaluation by all the three algorithms in 1- (Figures 4M–O).and 3-day-old adults (Figures 4U–W). However, in 2-day-old adults, inconsistent with the other two software algorithms, α-TUB1 only ranked sixth out of eight candidate RGs by NormFinder (Figures 4Q–S).
For the 1- to 3-day-old macropterous male adults, the top two stable RGs under the HMF treatment (versus local GMF) were respectively ACT1 & RPL5 (Figure 5D), RPL5 & EF1-α (Figure 5H) and α-TUB1 & ACT1 (Figure 5L), while across the same time period α-TUB1 & RPS15 (Figure 5D), α-TUB1 & RPS15 (Figure 5H) and 18S & AK (Figure 5L) were the two least stable RGs evaluated by comprehensive analyses based on BestKeeper, NormFinder, and GeNorm algorithms. Moreover, for the 1- to 3-day-old brachypterous male adults, the top two stable RGs under the HMF treatment (versus local GMF) were respectively EF1-α & RPL5 (Figure 5P), ARF1 & ACT1 (Figure 5T) and ACT1 & ARF1 (Figure 5X), while across the same time period RPS15 & ACT1 (Figure 5P), RPS15 & 18S (Figure 5T) and RPS15 & AK (Figure 5X) were the two least stable RGs evaluated by comprehensive analyses.
FIGURE 5. Expression stability evaluation of candidate reference genes respectively for 1- to 3-day-old macropterous and brachypterous male adults of N. lugens by BestKeeper, NormFinder, GeNorm and comprehensive analyses. Each row of the panel indicates that the experimental samples are from 1-day-old (1d), 2-day-old (2d) and 3-day-old (3d) macropterous [1d, (A–D); 2d, (E–H); 3d, (I–L)] and brachypterous [1d, (M–P); 2d, (Q–T); 3d, (U–X)] male adults under the hypomagnetic field versus local geomagnetic field. The standard deviation (SD) and coefficient of variation (CV) were given by BestKeeper (A,E,I,M,Q,U). The stability value (SV) was given by NormFinder (B,F,J,N,R,V). The average expression stability (M) was given by GeNorm (C,G,K,O,S,W). The comprehensive ranking was further generated based on the results derived from the geometric mean (GM) of these three algorithms (D,H,L,P,T,X). Stable reference genes generally have lower SD, SV, M and GM values.
For the macropterous males, ACT1 & RPL5 and α-TUB1 & ACT1 respectively ranked top 50% out of the eight candidates in 1- (Figures 5A–C) and 3-day-old (Figures 5I–L) adults according to all three algorithms. RPL5 & EF1-α ranked top two out of the eight candidates by NormFinder and GeNorm, while EF1-α ranked only fifth out of the eight based on the SD value of BestKeeper in 2-day-old adults (Figures 5E–G). In addition, for the brachypterous males, EF1-α & RPL5 and ACT1 & ARF1 ranked top three out of the eight candidates in 1- (Figures 5M–O) and 3-day-old (Figures 5U–W) adults respectively according to all the three algorithms. ARF1 & ACT1 were rated as the top two out of eight stable RGs by both BestKeeper and GeNorm, however, ACT1 ranked only fifth out of the eight by NormFinder in 2-day-old adults (Figures 5Q–S).
Facilitated trehalose transporter Tret1 (TRET1), a conserved transporter for trehalose in insects (Kikawada et al., 2007; Kanamori et al., 2010), was significantly differentially expressed based on our pilot transcriptome analysis for 2-day-old brachypterous female N. lugens subjected to the HMF versus local GMF conditions. We first verified the specificity and performance of the RT-qPCR primer of TRET1, as shown in Supplementary Figure 2. Then, to validate the selected reference genes, the relative expression levels of the target gene TRET1 in 2-day-old brachypterous females normalized to the reference genes evaluated in this work, including the top two stable RGs AK and α-TUB1 (individually or in combination use) and the least stable RPS15, were assessed under the HMF versus local GMF using RT-qPCR. Consistently, 2-day-old brachypterous females showed significant differences in TRET1 transcript expression levels between the HMF and local GMF groups using the suggested AK and α-TUB1 as RGs (F1, 6 = 9.376; p = 0.022; partial η2 = 0.61). When using AK (F1, 6 = 4.629; p = 0.075; partial η2 = 0.44) or α-TUB1 (F1, 6 = 5.247; p = 0.062; partial η2 = 0.47) as RG individually, consistent TRET1 transcript expression patterns can also be found, although the difference was not significant at the p < 0.05 level between the two magnetic field conditions. However, comparable expression levels of TRET1, but in a different pattern, were found between groups when the least stable RPS15 (F1, 6 = 0.079; p = 0.788; partial η2 = 0.01) was used as the only RG (Figure 6).
FIGURE 6. The transcript expression analyses for TRET1 in 2-day-old brachypterous females normalized to the reference genes evaluated in this work. The top two stable candidate reference genes AK and α-TUB1 (individually or in combination use) and the least stable candidate reference gene RPS15 were picked for the validation assay under the hypomagnetic field (HMF) versus local geomagnetic field (GMF). Samples of four biologically independent pools were used. The columns represent averages with vertical bars indicating standard errors. Asterisk (*) denotes significant differences between the HMF versus local GMF by one-way ANOVA at p < 0.05.
Static magnetic fields are generally classified as hypomagnetic (HMF) (<5 μT) (Zhang B. et al., 2021), weak (<1mT), moderate (1 mT–1 T), strong (1–20 T) and ultra-strong (>20 T) magnetic fields (Zhang et al., 2017). The HMF offers a unique option to help shed light on how magnetic fields (especially the GMF) influence life and the potential mechanisms relevant to the phenotypic effects of magnetic fields as well as magnetoreception mechanisms. Combined with multi-omic and reverse genetic tools, gene expression analysis plays a crucial role in uncovering the complex gene regulatory architecture underlying the HMF-triggered bioeffects and magnetoreception. However, few magnetobiology studies have included a preliminary stability assessment of the reference genes used in gene expression analyses of the target genes under investigation (Di et al., 2011; Fu et al., 2016; Agliassa et al., 2018; Agliassa and Maffei, 2019; Liu et al., 2019; El May et al., 2021). Using the migratory N. lugens, which has the potential to be an unconventional insect model for magnetobiology and magnetoreception, we systematically assessed the stability of eight selected candidate reference genes (RGs) across developmental stages, sexes, and wing morphs with three widely used algorithms [BestKeeper (Pfaffl et al., 2004), NormFinder (Andersen et al., 2004), and GeNorm (Vandesompele et al., 2002)]. A follow-up validation assay with 2-day-old brachypterous females targeting TRET1, a conserved trehalose transporter, was then conducted to test the reliability of the suggested RG (s) (Kikawada et al., 2007; Kanamori et al., 2010).
According to the GeNorm, two reference genes were suggested here for normalization of gene expression in N. lugens under the two magnetic field groups, consistent with that of Laodelphax striatellus, another notorious species of rice planthopper (Liu et al., 2019). In the validation assay, the difference in transcript expression of target gene TRET1, which functions in mediating the trehalose exchanges among various tissues in insects (Kikawada et al., 2007; Kanamori et al., 2010), reached a significant level between the HMF versus GMF only when using the combination of the suggested top two stable RGs. This result is consistent with our previous work showing that trehalose levels varied significantly between two different magnetic field intensities. Moreover, the validation assay also indicated that combining two stable RGs rather than a single one increased the effect size, further supporting the importance of introducing another stable RG to secure more accurate experimental results. Although there have different screening principles and emphases (Vandesompele et al., 2002; Andersen et al., 2004; Pfaffl et al., 2004) for expression stability analysis amongst BestKeeper (Pfaffl et al., 2004), NormFinder (Andersen et al., 2004), and GeNorm (Vandesompele et al., 2002), the recommended top two most stable RGs were consistent in most conditions. Nevertheless, a comprehensive analysis was still further applied based on the results derived from the geometric mean of these three algorithms, which offered good comprehensive ranking performance based on common practices (Xie et al., 2012; Niu et al., 2015; Zhang Z. et al., 2020) and our validation assay.
Previous studies in vitro have shown that changes in GMF intensity [including the strong magnetic field (Qian et al., 2010) and HMF (Wang et al., 2008; Mo et al., 2016)] can affect cytoskeleton and cytoskeleton-associated genes, which may be due to quantum effects (Zadeh-Haghighi and Simon, 2022). In particular, the in vitro assembly of TUB (Wang et al., 2008) and F-ACT (Mo et al., 2016) at the protein level can be affected by the HMF. However, our previous work suggested α2-TUB as the most stable RG in newly emerged brachypterous male adults of L. striatellus. The transcript expression stability of ACT1 and α-TUB1 of N. lugens was also assessed in the current study. According to the expression variability (SD) of the eight candidate RGs in all 272 samples under the HMF versus GMF, ACT1 and α-TUB1 were respectively ranked as the 2nd and 4th most stable RGs (Supplementary Table 1). Although the expression stability of ACT1 was ranked poorly during the nymphal stage and in 1-3-day-old macropterous female adults for most situations, its expression stability performed well in the majority of the rest groups based on the three algorithms and corresponding comprehensive rankings. For α-TUB1, its evaluated expression stability also varied across developmental stages, sexes, and wing morphs (Figures 3–5). Moreover, our validation assay using α-TUB1 as one of the top two stable RGs in 2-day-old brachypterous females further affirmed the constant expression of α-TUB1 between the HMF versus GMF as well as the reliability of the expression stability ranking scored by the algorithms (Figure 6). All these results indicate that the reported effects triggered by the HMF on F-ACT and TUB are likely to exert only at the protein level or in a trait-specific way.
As with the current study, the only two other systematic reference gene selection studies, to our knowledge, also found that commonly used housekeeping genes are not always consistently expressed between magnetic field intensities in vivo (Liu et al., 2019) and in vitro (Di et al., 2011). To some extent, as an extension of our previous RG selection work with brachypterous female and male L. striatellus (Liu et al., 2019), the current study with N. lugens further showed that the assessed RG expression stability varied across not only sex but also developmental stage and wing morph under the HMF versus local GMF. Having a closer look, the top two stable RGs frequently varied while RPS15 remained to be ranked as the last three out of eight across different groups. Thus, it should be noted that even though PRS15 was reported as the most suitable RT-qPCR reference gene for N. lugens at different developmental stages (Yuan et al., 2014), it is not a reliable reference gene under the HMF condition. When adopting PRS15 as the only RG for magnetic field intensity treatment, comparable expression levels of TRET1 in a contrary pattern were found compared to using suggested stable RG(s). Interestingly, unlike RPS15, another ribosomal protein gene widely used as the housekeeping gene, RPL5, was scored much better by the algorithms, which may be due to the difference in sensitivity to magnetic field intensity change regarding their ribosome-independent functions (Zhou et al., 2015).
Overall, increasing evidence indicates that the magnetic field intensity is a tricky environmental factor to control for and requires more attention in the design and analysis of gene expression studies (Makinistian and Belyaev, 2018). The gene expression stability assay presented here highlights the potential importance of using reliable RG(s) in gene expression investigations of magnetobioloy including magnetoreception. This study provides a basis for more reliable future studies as we unveil the potential signal pathways underlying responses to changes in magnetic field intensity in the important migratory pest, N. lugens.
The original contributions presented in the study are included in the article/Supplementary Materials, further inquiries can be directed to the corresponding author.
Conceptualization, GW, FC, and WP; methodology, GW, LZ, YZ, and MZ; validation, YZ, LZ, GW, and YW; formal analysis, YZ, LZ, GW, and FY; writing—original draft preparation, YZ, GW, and LZ; writing—review and editing, GW, GS, WP, FY, and FC; supervision, GW. All authors have read and agreed to the published version of the manuscript.
This research was funded by the National Natural Science Foundation of China (32072413, 32172414, and 31870367), the Natural Science Foundation of Jiangsu Province (BK20221510), the National Key Research and Development Program of China (2021YFD1401100), the Young Scientific and Technological Talents of Jiangsu Association for Science and Technology (TJ-2021-003), the Start-up Fund of Jiangsu Province Advantageous Discipline Construction Project (020809005), the Overseas Researcher Science and Technology Innovation Project of Nanjing.
We would like to thank Xu Huang, Zhenzhen Gao, Changning Lv, and Yilihamu Remaiti for maintaining the colony.
The authors declare that the research was conducted in the absence of any commercial or financial relationships that could be construed as a potential conflict of interest.
All claims expressed in this article are solely those of the authors and do not necessarily represent those of their affiliated organizations, or those of the publisher, the editors and the reviewers. Any product that may be evaluated in this article, or claim that may be made by its manufacturer, is not guaranteed or endorsed by the publisher.
The Supplementary Material for this article can be found online at: https://www.frontiersin.org/articles/10.3389/fphys.2022.954228/full#supplementary-material
Agliassa C., Maffei M. E. (2019). Reduction of geomagnetic field (GMF) to near null magnetic field (NNMF) affects some Arabidopsis thaliana clock genes amplitude in a light independent manner. J. Plant Physiol. 232, 23–26. doi:10.1016/j.jplph.2018.11.008
Agliassa C., Narayana R., Christie J. M., Maffei M. E. (2018). Geomagnetic field impacts on cryptochrome and phytochrome signaling. J. Photochem Photobiol. B 185, 32–40. doi:10.1016/j.jphotobiol.2018.05.027
Alken P., Thébault E., Beggan C. D., Amit H., Aubert J., Baerenzung J., et al. (2021). International geomagnetic reference field: The thirteenth generation. Earth Planets Space 73, 49. doi:10.1186/s40623-020-01288-x
Andersen C. L., Jensen J. L., Orntoft T. F. (2004). Normalization of real-time quantitative reverse transcription-PCR data: A model-based variance estimation approach to identify genes suited for normalization, applied to bladder and colon cancer data sets. Cancer Res. 64 (15), 5245–5250. doi:10.1158/0008-5472.CAN-04-0496
Bae J. E., Bang S., Min S., Lee S. H., Kwon S. H., Lee Y., et al. (2016). Positive geotactic behaviors induced by geomagnetic field in Drosophila. Mol. Brain 9 (1), 55. doi:10.1186/s13041-016-0235-1
Bazalova O., Kvicalova M., Valkova T., Slaby P., Bartos P., Netusil R., et al. (2016). Cryptochrome 2 mediates directional magnetoreception in cockroaches. Proc. Natl. Acad. Sci. U. S. A. 113 (6), 1660–1665. doi:10.1073/pnas.1518622113
Berguig M. S., Hamoudi M., Lemouël J. L., Cohen Y. (2011). Validate global mapping of internal lunar magnetic field. Arab. J. Geosci. 6 (4), 1063–1072. doi:10.1007/s12517-011-0406-4
Binhi V. N., Prato F. S. (2017). Biological effects of the hypomagnetic field: An analytical review of experiments and theories. PLoS One 12 (6), e0179340. doi:10.1371/journal.pone.0179340
Binhi V. N., Prato F. S. (2018). Rotations of macromolecules affect nonspecific biological responses to magnetic fields. Sci. Rep. 8 (1), 13495. doi:10.1038/s41598-018-31847-y
Binhi V. N., Rubin A. B. (2022). Theoretical concepts in magnetobiology after 40 years of research. Cells 11 (2), 274. doi:10.3390/cells11020274
Binhi V. N., Sarimov R. M. (2009). Zero magnetic field effect observed in human cognitive processes. Electromagn. Biol. Med. 28 (3), 310–315. doi:10.3109/15368370903167246
Bliss V. L., Heppner F. H. (1976). Circadian activity rhythm influenced by near zero magnetic field. Nature 261 (5559), 411–412. doi:10.1038/261411a0
Cheng X. N., Wu J. C., Ma F. (2003). Brown planthopper: Occurrence and control. Beijing, China: China Agricultural Press.
Choleris E., Del Seppia C., Thomas A. W., Luschi P., Ghione G., Moran G. R., et al. (2002). Shielding, but not zeroing of the ambient magnetic field reduces stress-induced analgesia in mice. Proc. Biol. Sci. 269 (1487), 193–201. doi:10.1098/rspb.2001.1866
Derveaux S., Vandesompele J., Hellemans J. (2010). How to do successful gene expression analysis using real-time PCR. Methods 50 (4), 227–230. doi:10.1016/j.ymeth.2009.11.001
Di S., Tian Z., Qian A., Gao X., Yu D., Brandi M. L., et al. (2011). Selection of suitable reference genes from bone cells in large gradient high magnetic field based on GeNorm algorithm. Electromagn. Biol. Med. 30 (4), 261–269. doi:10.3109/15368378.2011.608869
El May A., Zouaoui J., Snoussi S., Ben Mouhoub R., Landoulsi A. (2021). RelA and spoT gene expression is modulated in Salmonella grown under static magnetic field. Curr. Microbiol. 78 (3), 887–893. doi:10.1007/s00284-021-02346-7
Fedele G., Edwards M. D., Bhutani S., Hares J. M., Murbach M., Green E. W., et al. (2014a). Genetic analysis of circadian responses to low frequency electromagnetic fields in Drosophila melanogaster. PLoS Genet. 10 (12), e1004804. doi:10.1371/journal.pgen.1004804
Fedele G., Green E. W., Rosato E., Kyriacou C. P. (2014b). An electromagnetic field disrupts negative geotaxis in Drosophila via a CRY-dependent pathway. Nat. Commun. 5, 4391. doi:10.1038/ncomms5391
Fesenko E. E., Mezhevikina L. M., Osipenko M. A., Gordon R. Y., Khutzian S. S. (2010). Effect of the "zero" magnetic field on early embryogenesis in mice. Electromagn. Biol. Med. 29 (1-12), 1–8. doi:10.3109/15368371003627290
Fitak R. R., Wheeler B. R., Ernst D. A., Lohmann K. J., Johnsen S. (2017). Candidate genes mediating magnetoreception in rainbow trout (Oncorhynchus mykiss). Biol. Lett. 13 (4), 20170142. doi:10.1098/rsbl.2017.0142
Foley L. E., Gegear R. J., Reppert S. M. (2011). Human cryptochrome exhibits light-dependent magnetosensitivity. Nat. Commun. 2, 356. doi:10.1038/ncomms1364
Fu J. P., Mo W. C., Liu Y., Bartlett P. F., He R. Q. (2016). Elimination of the geomagnetic field stimulates the proliferation of mouse neural progenitor and stem cells. Protein Cell 7 (9), 624–637. doi:10.1007/s13238-016-0300-7
Gao Y., Wen P., Carde R. T., Xu H., Huang Q. (2021). In addition to cryptochrome 2, magnetic particles with olfactory co-receptor are important for magnetic orientation in termites. Commun. Biol. 4 (1), 1121. doi:10.1038/s42003-021-02661-6
Gegear R. J., Casselman A., Waddell S., Reppert S. M. (2008). Cryptochrome mediates light-dependent magnetosensitivity in Drosophila. Nature 454 (7207), 1014–1018. doi:10.1038/nature07183
Gegear R. J., Foley L. E., Casselman A., Reppert S. M. (2010). Animal cryptochromes mediate magnetoreception by an unconventional photochemical mechanism. Nature 463 (7282), 804–807. doi:10.1038/nature08719
Ghodbane S., Lahbib A., Sakly M., Abdelmelek H. (2013). Bioeffects of static magnetic fields: Oxidative stress, genotoxic effects, and cancer studies. Biomed. Res. Int. 2013, 602987. doi:10.1155/2013/602987
Guerra P. A. (2011). Evaluating the life-history trade-off between dispersal capability and reproduction in wing dimorphic insects: A meta-analysis. Biol. Rev. Camb Philos. Soc. 86 (4), 813–835. doi:10.1111/j.1469-185X.2010.00172.x
Günther A., Einwich A., Sjulstok E., Feederle R., Bolte P., Koch K. W., et al. (2018). Double-cone localization and seasonal expression pattern suggest a role in magnetoreception for European robin cryptochrome 4. Curr. Biol. 28 (2), 211–223.e4. doi:10.1016/j.cub.2017.12.003
Hochstoeger T., Al Said T., Maestre D., Walter F., Vilceanu A., Pedron M., et al. (2020). The biophysical, molecular, and anatomical landscape of pigeon CRY4: A candidate light-based quantal magnetosensor. Sci. Adv. 6 (33), eabb9110. doi:10.1126/sciadv.abb9110
Hore P. J., Mouritsen H. (2016). The radical-pair mechanism of magnetoreception. Annu. Rev. Biophys. 45, 299–344. doi:10.1146/annurev-biophys-032116-094545
Kanamori Y., Saito A., Hagiwara-Komoda Y., Tanaka D., Mitsumasu K., Kikuta S., et al. (2010). The trehalose transporter 1 gene sequence is conserved in insects and encodes proteins with different kinetic properties involved in trehalose import into peripheral tissues. Insect Biochem. Mol. Biol. 40 (1), 30–37. doi:10.1016/j.ibmb.2009.12.006
Kikawada T., Saito A., Kanamori Y., Nakahara Y., Iwata K., Tanaka D., et al. (2007). Trehalose transporter 1, a facilitated and high-capacity trehalose transporter, allows exogenous trehalose uptake into cells. Proc. Natl. Acad. Sci. U. S. A. 104 (28), 11585–11590. doi:10.1073/pnas.0702538104
Kirschvink J. (2001). Magnetite-based magnetoreception. Curr. Opin. Neurobiol. 11 (4), 462–467. doi:10.1016/s0959-4388(00)00235-x
Kishkinev D., Packmor F., Zechmeister T., Winkler H. C., Chernetsov N., Mouritsen H., et al. (2021). Navigation by extrapolation of geomagnetic cues in a migratory songbird. Curr. Biol. 31 (7), 1563–1569. doi:10.1016/j.cub.2021.01.051
Liu F., Wan G., Zeng L., Li C., Pan W., Chen F. (2019). Selection of stable internal reference genes for transcript expression analyses in Laodelphax striatellus under near-zero magnetic field. Zhongguo Nong Ye Ke Xue 52 (19), 10. doi:10.3864/j.issn.0578-1752.2019.19.006
Livak K. J., Schmittgen T. D. (2001). Analysis of relative gene expression data using real-time quantitative PCR and the 2−ΔΔct Method. Methods 25 (4), 402–408. doi:10.1006/meth.2001.1262
Lohmann K. J. (2010). Q&A: Animal behaviour: Magnetic-field perception. Nature 464 (7292), 1140–1142. doi:10.1038/4641140a
Lu J., Yang C., Zhang Y., Pan H. (2018). Selection of reference genes for the normalization of RT-qPCR data in gene expression studies in insects: A systematic review. Front. Physiol. 9, 1560. doi:10.3389/fphys.2018.01560
Makinistian L., Belyaev I. (2018). Magnetic field inhomogeneities due to CO2 incubator shelves: A source of experimental confounding and variability? R. Soc. Open Sci. 5 (2), 172095. doi:10.1098/rsos.172095
Menz M. H. M., Reynolds D. R., Gao B., Hu G., Chapman J. W., Wotton K. R. (2019). Mechanisms and consequences of partial migration in insects. Front. Ecol. Evol. 7, 403. doi:10.3389/fevo.2019.00403
Miyakoshi J. (2005). Effects of static magnetic fields at the cellular level. Prog. Biophys. Mol. Biol. 87 (2-3), 213–223. doi:10.1016/j.pbiomolbio.2004.08.008
Mo W. C., Liu Y., Bartlett P. F., He R. Q. (2014). Transcriptome profile of human neuroblastoma cells in the hypomagnetic field. Sci. China Life Sci. 57 (4), 448–461. doi:10.1007/s11427-014-4644-z
Mo W. C., Liu Y., Cooper H. M., He R. Q. (2012). Altered development of Xenopus embryos in a hypogeomagnetic field. Bioelectromagnetics 33 (3), 238–246. doi:10.1002/bem.20699
Mo W. C., Zhang Z. J., Wang D. L., Liu Y., Bartlett P. F., He R. Q. (2016). Shielding of the geomagnetic field alters actin assembly and inhibits cell motility in human neuroblastoma cells. Sci. Rep. 6, 22624. doi:10.1038/srep22624
Monteil C. L., Lefevre C. T. (2020). Magnetoreception in microorganisms. Trends Microbiol. 28 (4), 266–275. doi:10.1016/j.tim.2019.10.012
Mouritsen H. (2018). Long-distance navigation and magnetoreception in migratory animals. Nature 558 (7708), 50–59. doi:10.1038/s41586-018-0176-1
Netusil R., Tomanova K., Chodakova L., Chvalova D., Dolezel D., Ritz T., et al. (2021). Cryptochrome-dependent magnetoreception in a heteropteran insect continues even after 24 h in darkness. J. Exp. Biol. 224 (19), jeb243000. doi:10.1242/jeb.243000
Niu X., Qi J., Zhang G., Xu J., Tao A., Fang P., et al. (2015). Selection of reliable reference genes for quantitative real-time PCR gene expression analysis in Jute (Corchorus capsularis) under stress treatments. Front. Plant Sci. 6, 848. doi:10.3389/fpls.2015.00848
Pan W., Wan G., Xu J., Li X., Liu Y., Qi L., et al. (2016). Evidence for the presence of biogenic magnetic particles in the nocturnal migratory Brown planthopper, Nilaparvata lugens. Sci. Rep. 6, 18771. doi:10.1038/srep18771
Pfaffl M. W., Tichopad A., Prgomet C., Neuvians T. P. (2004). Determination of stable housekeeping genes, differentially regulated target genes and sample integrity: BestKeeper – excel-based tool using pair-wise correlations. Biotechnol. Lett. 26 (6), 509–515. doi:10.1023/b:bile.0000019559.84305.47
Ponton F., Chapuis M. P., Pernice M., Sword G. A., Simpson S. J. (2011). Evaluation of potential reference genes for reverse transcription-qPCR studies of physiological responses in Drosophila melanogaster. J. Insect Physiol. 57 (6), 840–850. doi:10.1016/j.jinsphys.2011.03.014
Qian A. R., Yang P. F., Hu L. F., Zhang W., Di S. M., Wang Z., et al. (2010). High magnetic gradient environment causes alterations of cytoskeleton and cytoskeleton-associated genes in human osteoblasts cultured in vitro. Adv. Space Res. 46 (6), 687–700. doi:10.1016/j.asr.2010.04.012
Qin H., Zhao X., Liu S., Paterson G. A., Jiang Z., Cai S., et al. (2020). An ultra-low magnetic field thermal demagnetizer for high-precision paleomagnetism. Earth Planets Space 72, 170. doi:10.1186/s40623-020-01304-0
Qin S., Yin H., Yang C., Dou Y., Liu Z., Zhang P., et al. (2016). A magnetic protein biocompass. Nat. Mater 15 (2), 217–226. doi:10.1038/nmat4484
Saunders R. (2005). Static magnetic fields: Animal studies. Prog. Biophys. Mol. Biol. 87 (2-3), 225–239. doi:10.1016/j.pbiomolbio.2004.09.001
Sherrard R. M., Morellini N., Jourdan N., El-Esawi M., Arthaut L. D., Niessner C., et al. (2018). Low-intensity electromagnetic fields induce human cryptochrome to modulate intracellular reactive oxygen species. PLoS Biol. 16 (10), e2006229. doi:10.1371/journal.pbio.2006229
Svedhem H., Titov D. V., Taylor F. W., Witasse O. (2007). Venus as a more Earth-like planet. Nature 450 (7170), 629–632. doi:10.1038/nature06432
Tian L., Pan Y. (2018). The geomagnetic field effects on animals: A review. Chin. Sci. Bull. 64 (8), 761–772. doi:10.1360/n972018-00650
Vandesompele J., De Preter K., Pattyn F., Poppe B., Van Roy N., De Paepe A., et al. (2002). Accurate normalization of real-time quantitative RT-PCR data by geometric averaging of multiple internal control genes. Genome Biol. 3 (7). doi:10.1186/gb-2002-3-7-research0034.1
Wan G., Hayden A. N., Iiams S. E., Merlin C. (2021). Cryptochrome 1 mediates light-dependent inclination magnetosensing in monarch butterflies. Nat. Commun. 12 (1), 771. doi:10.1038/s41467-021-21002-z
Wan G., Jiang S., Zhang M., Zhao J., Zhang Y., Pan W., et al. (2020b). Geomagnetic field absence reduces adult body weight of a migratory insect by disrupting feeding behavior and appetite regulation. Insect Sci. 28 (1), 251–260. doi:10.1111/1744-7917.12765
Wan G., Jiang S., Zhao Z., Xu J., Tao X., Sword G. A., et al. (2014). Bio-effects of near-zero magnetic fields on the growth, development and reproduction of small Brown planthopper, Laodelphax striatellus and Brown planthopper, Nilaparvata lugens. J. Insect Physiol. 68, 7–15. doi:10.1016/j.jinsphys.2014.06.016
Wan G., Liu R., Li C., He J., Pan W., Sword G. A., et al. (2020a). Change in geomagnetic field intensity alters migration-associated traits in a migratory insect. Biol. Lett. 16 (4), 20190940. doi:10.1098/rsbl.2019.0940
Wan G., Wang W., Xu J., Yang Q., Dai M., Zhang F., et al. (2015). Cryptochromes and hormone signal transduction under near-zero magnetic fields: New clues to magnetic field effects in a rice planthopper. PLoS One 10 (7), e0132966. doi:10.1371/journal.pone.0132966
Wan G., Yuan R., Wang W., Fu K., Zhao J., Jiang S., et al. (2016). Reduced geomagnetic field may affect positive phototaxis and flight capacity of a migratory rice planthopper. Anim. Behav. 121, 107–116. doi:10.1016/j.anbehav.2016.08.024
Wang D. L., Wang X. S., Xiao R., Liu Y., He R. Q. (2008). Tubulin assembly is disordered in a hypogeomagnetic field. Biochem. Biophys. Res. Commun. 376 (2), 363–368. doi:10.1016/j.bbrc.2008.08.156
Wang X., Jing C., Selby C. P., Chiou Y. Y., Yang Y., Wu W., et al. (2018). Comparative properties and functions of type 2 and type 4 pigeon cryptochromes. Cell Mol. Life Sci. 75 (24), 4629–4641. doi:10.1007/s00018-018-2920-y
Watters T. R., McGovern P. J., Irwin R. P. (2007). Hemispheres apart: The crustal dichotomy on Mars. Annu. Rev. Earth Planet Sci. 35 (1), 621–652. doi:10.1146/annurev.earth.35.031306.140220
Wu Y., Zhang C., Yang H., Lyu L., Li W., Wu W. (2021). Selection and validation of candidate reference genes for gene expression analysis by RT-qPCR in Rubus. Int. J. Mol. Sci. 22 (19), 10533. doi:10.3390/ijms221910533
Wynn J., Padget O., Mouritsen H., Morford J., Jaggers P., Guilford T. (2022). Magnetic stop signs signal a European songbird's arrival at the breeding site after migration. Science 375 (6579), 446–449. doi:10.1126/science.abj4210
Xie F., Xiao P., Chen D., Xu L., Zhang B. (2012). miRDeepFinder: a miRNA analysis tool for deep sequencing of plant small RNAs. Plant Mol. Biol. 80 (1), 75–84. doi:10.1007/s11103-012-9885-2
Xu C., Yin X., Lv Y., Wu C., Zhang Y., Song T. (2012). A near-null magnetic field affects cryptochrome-related hypocotyl growth and flowering in Arabidopsis. Adv. Space Res. 49 (5), 834–840. doi:10.1016/j.asr.2011.12.004
Xu J., Jarocha L. E., Zollitsch T., Konowalczyk M., Henbest K. B., Richert S., et al. (2021). Magnetic sensitivity of cryptochrome 4 from a migratory songbird. Nature 594 (7864), 535–540. doi:10.1038/s41586-021-03618-9
Xu J., Wan G., Hu D., He J., Chen F., Wang X., et al. (2016). Molecular characterization, tissue and developmental expression profiles of cryptochrome genes in wing dimorphic Brown planthoppers, Nilaparvata lugens. Insect Sci. 23 (6), 805–818. doi:10.1111/1744-7917.12256
Xu J., Zhang Y., Wu J., Wang W., Li Y., Wan G., et al. (2019). Molecular characterization, spatial‐temporal expression and magnetic response patterns of iron‐sulfur cluster assembly1 (IscA1) in the rice planthopper, Nilaparvata lugens. Insect Sci. 26 (3), 413–423. doi:10.1111/1744-7917.12546
Xue X., Ali Y. F., Luo W., Liu C., Zhou G., Liu N. A. (2021). Biological effects of space hypomagnetic environment on circadian rhythm. Front. Physiol. 12, 643943. doi:10.3389/fphys.2021.643943
Yan M., Zhang L., Cheng Y., Sappington T. W., Pan W., Jiang X. (2021). Effect of a near-zero magnetic field on development and flight of oriental armyworm (Mythimna separata). J. Integr. Agric. 20 (5), 1336–1345. doi:10.1016/s2095-3119(20)63287-7
Yoshii T., Ahmad M., Helfrich-Forster C. (2009). Cryptochrome mediates light-dependent magnetosensitivity of Drosophila's circadian clock. PLoS Biol. 7 (4), e1000086. doi:10.1371/journal.pbio.1000086
Yuan M., Lu Y., Zhu X., Wan H., Shakeel M., Zhan S., et al. (2014). Selection and evaluation of potential reference genes for gene expression analysis in the Brown planthopper, Nilaparvata lugens (Hemiptera: Delphacidae) using reverse-transcription quantitative PCR. PLoS One 9 (1), e86503. doi:10.1371/journal.pone.0086503
Zadeh-Haghighi H., Simon C. (2022). Radical pairs may play a role in microtubule reorganization. Sci. Rep. 12 (1), 6109. doi:10.1038/s41598-022-10068-4
Zhang B., Lu H., Xi W., Zhou X., Xu S., Zhang K., et al. (2004). Exposure to hypomagnetic field space for multiple generations causes amnesia in Drosophila melanogaster. Neurosci. Lett. 371 (2-3), 190–195. doi:10.1016/j.neulet.2004.08.072
Zhang B., Tian L. (2020). Reactive oxygen species: Potential regulatory molecules in response to hypomagnetic field exposure. Bioelectromagnetics 41 (8), 573–580. doi:10.1002/bem.22299
Zhang B., Wang L., Zhan A., Wang M., Tian L., Guo W., et al. (2021a). Long-term exposure to a hypomagnetic field attenuates adult hippocampal neurogenesis and cognition. Nat. Commun. 12 (1), 1174. doi:10.1038/s41467-021-21468-x
Zhang Y., Pan W. (2021). Removal or component reversal of local geomagnetic field affects foraging orientation preference in migratory insect Brown planthopper Nilaparvata lugens. PeerJ 9, e12351. doi:10.7717/peerj.12351
Zhang Y., Wan G., Wang W., Li Y., Yu Y., Zhang Y., et al. (2020a). Enhancement of the geomagnetic field reduces the phototaxis of rice Brown planthopper Nilaparvata lugens associated with frataxin down-regulation. Insect Sci. 27 (5), 1043–1052. doi:10.1111/1744-7917.12714
Zhang Z., Li C., Zhang J., Chen F., Gong Y., Li Y., et al. (2020b). Selection of the reference gene for expression normalization in Papaver somniferum L. under abiotic stress and hormone treatment. Genes (Basel) 11 (2), 124. doi:10.3390/genes11020124
Zhang Z., Xue Y., Yang J., Shang P., Yuan X. (2021b). Biological effects of hypomagnetic field: Ground-based data for space exploration. Bioelectromagnetics 42 (6), 516–531. doi:10.1002/bem.22360
Keywords: migratory insect, magnetic effects, reference gene, gene expression analysis, hypomagnetic field, magnetobiology, magnetoreception, rice planthopper
Citation: Zhang Y, Zeng L, Wei Y, Zhang M, Pan W, Sword GA, Yang F, Chen F and Wan G (2022) Reliable reference genes for gene expression analyses under the hypomagnetic field in a migratory insect. Front. Physiol. 13:954228. doi: 10.3389/fphys.2022.954228
Received: 27 May 2022; Accepted: 07 July 2022;
Published: 08 August 2022.
Edited by:
Senthil-Nathan, Sengottayan, Manonmaniam Sundaranar University, IndiaCopyright © 2022 Zhang, Zeng, Wei, Zhang, Pan, Sword, Yang, Chen and Wan. This is an open-access article distributed under the terms of the Creative Commons Attribution License (CC BY). The use, distribution or reproduction in other forums is permitted, provided the original author(s) and the copyright owner(s) are credited and that the original publication in this journal is cited, in accordance with accepted academic practice. No use, distribution or reproduction is permitted which does not comply with these terms.
*Correspondence: Guijun Wan, Z3VpanVud2FuQG5qYXUuZWR1LmNu
Disclaimer: All claims expressed in this article are solely those of the authors and do not necessarily represent those of their affiliated organizations, or those of the publisher, the editors and the reviewers. Any product that may be evaluated in this article or claim that may be made by its manufacturer is not guaranteed or endorsed by the publisher.
Research integrity at Frontiers
Learn more about the work of our research integrity team to safeguard the quality of each article we publish.