- 1Department of Family Medicine, Faculty of Medicine, University of Ottawa, Ottawa, ON, Canada
- 2Institut du Savoir Montfort (Research), University of Ottawa, Ottawa, ON, Canada
Managing chronic diseases, such as heart disease, stroke, diabetes, chronic lung disease and Alzheimer’s disease, account for a large proportion of health care spending, yet they remain in the top causes of premature mortality and are preventable. It is currently accepted that an unhealthy lifestyle fosters a state of chronic low-grade inflammation that is linked to chronic disease progression. Although this is known to be related to inflammatory cytokines, how an unhealthy lifestyle causes cytokine release and how that in turn leads to chronic disease progression are not well known. This article presents a theory that an unhealthy lifestyle fosters chronic disease by changing interstitial cell behavior and is supported by a six-level hierarchical network analysis. The top three networks include the macroenvironment, social and cultural factors, and lifestyle itself. The fourth network includes the immune, autonomic and neuroendocrine systems and how they interact with lifestyle factors and with each other. The fifth network identifies the effects these systems have on the microenvironment and two types of interstitial cells: macrophages and fibroblasts. Depending on their behaviour, these cells can either help maintain and restore normal function or foster chronic disease progression. When macrophages and fibroblasts dysregulate, it leads to chronic low-grade inflammation, fibrosis, and eventually damage to parenchymal (organ-specific) cells. The sixth network considers how macrophages change phenotype. Thus, a pathway is identified through this hierarchical network to reveal how external factors and lifestyle affect interstitial cell behaviour. This theory can be tested and it needs to be tested because, if correct, it has profound implications. Not only does this theory explain how chronic low-grade inflammation causes chronic disease progression, it also provides insight into salutogenesis, or the process by which health is maintained and restored. Understanding low-grade inflammation as a stalled healing process offers a new strategy for chronic disease management. Rather than treating each chronic disease separately by a focus on parenchymal pathology, a salutogenic strategy of optimizing interstitial health could prevent and mitigate multiple chronic diseases simultaneously.
Introduction
Prior to the COVID-19 pandemic, Americans spent almost $4 trillion a year on healthcare services, 90% of which was spent on chronic disease (Buttorff et al., 2017; Martin et al., 2021). Sixty percent of all adults in the United States have at least one chronic condition, and 80% of older adults have multiple chronic diseases (Buttorff et al., 2017). Yet despite all the medications and all the health care given, many chronic conditions—such as heart disease, stroke, diabetes, chronic lung disease, and Alzheimer’s disease—are in the top ten causes of death and have remained there largely unchanged for decades (Kochanek et al., 2019). It has been estimated that this “silent epidemic” of chronic disease killed almost four times more Americans in 2020 than COVID-19 (Ahmad and Anderson, 2021; Rippe, 2021).
The World Health Organization (WHO) reported that, between 2000–2019, the world’s most common cause of death was ischemic heart disease, and it was responsible for the largest increase in deaths worldwide over that time period (World Health Organization, 2020). And it is preventable. Multiple studies have shown a healthy lifestyle can help prevent myocardial infarctions regardless of genetic susceptibility (Abraham et al., 2016; Khera et al., 2016; Sotos-Prieto et al., 2016; Said et al., 2018). A New England Journal of Medicine study analyzed over 50,000 people and found that for those at high genetic risk, not smoking, avoiding obesity, regular physical activity, and a healthy diet were associated with a nearly 50% lower relative risk of coronary artery disease than those at high genetic risk with an unfavorable lifestyle (Khera et al., 2016). Another study of over 300,000 people found the effect size even larger (Said et al., 2018).
Physical activity is the cornerstone of a healthy lifestyle. A recent Cochrane review of 187 randomized controlled trials (RCTs) confirmed that both healthy and medically compromised individuals can significantly improve function, physical and mental health, and decrease mortality by exercising more (Posadzki et al., 2020). A clear dose-response relationship between physical activity and longevity has been found (Ekelund et al., 2019; Geidl et al., 2020). In contrast, WHO has noted that sedentariness—or insufficient physical activity—is one of the leading risk factors for premature mortality (World Health Organization, 2022). The general public knows that getting regular physical activity is good for you and unhealthy eating and stress are not, yet most Americans do not meet the United States guidelines for healthy eating or physical activity (Physical Activity Guidelines Advisory Committee, 2018), and anxiety levels and social unease are more common than ever.
Over the last two decades, research from multiple sources has shown that chronic disease is associated with chronic low-grade inflammation linked with an unhealthy lifestyle and aging (Fülöp et al., 2019). Low grade inflammation is a prominent feature of atherosclerosis (Wolf and Ley, 2019; Vergallo and Crea, 2020), cardiovascular disease (Fioranelli et al., 2018) and stroke (Murray et al., 2013). It plays a critical role in chronic lung disease (Yamasaki and Eeden, 2018), obesity (Kohlgruber and Lynch, 2015) and type 2 diabetes (Shapouri-Moghaddam et al., 2018), as well as cognitive impairment (Bettcher and Kramer, 2013) and Alzheimer’s disease (Giunta et al., 2008).
Two basic questions remain: How does an unhealthy lifestyle cause chronic low-grade inflammation? And: How does inflammation cause chronic disease progression? These questions are starting to be addressed by recent advances in physiology, such as understanding the interactions of the immune system with food and the microbiome, intercellular cross-talk, and the epigenetics of phenotypic shifts. And to bring this all together, is another relatively new field: network analysis.
Network analysis is the study of the interactions involved in complex physical, social and biological processes. It is often illustrated with a figure where key factors are represented by nodes and key influences and interactions among these factors are represented by edges or connecting lines. Nodes that have multiple interactions tend to have the most influence and are called hubs. Networks can be single, multiple, or multiple and hierarchical. Network analysis has been applied to physiology and medicine. Network physiology addresses how physiological systems and subsystems coordinate and interact to optimize health and adapt to changing physiologic states (Bartsch et al., 2015; Ivanov et al., 2016; Lehnertz et al., 2020). It has been applied to lifestyle factors, such as exercise, to examine the dynamic interactions of skeletal muscle with organs and fat (Balagué et al., 2020). And it has been applied more broadly to identify health as an emergent state that arises from hierarchical network interactions between a person’s external environment and their internal physiology (Sturmberg et al., 2019). Network medicine has been used to understand the interacting genetic and non-genetic factors associated with diabetes (Zhang et al., 2021) and to-analyze how genomic, proteomic, and metabolic factors lead to different disease phenotypes (Ivanov et al., 2016).
The aim of this network analysis is to consider how lifestyle is embedded in a network of influences, how the physical and social environment influences peoples’ lifestyles and how, in turn, both a healthy and an unhealthy lifestyle have an influence on chronic disease progression. This network analysis represents a map of the connections between the macroenvironment, an intermediary mesoenvironment, and the microenvironment. Evidence is presented that leads to the conclusion chronic disease progression arises from a stalled healing process orchestrated by immune and connective tissue cells in the microenvironment. When immune cells get dysregulated, it leads to chronic low-grade inflammation and when connective tissue cells get dysregulated it leads to progressive fibrosis. The outcome of this cellular behaviour is illustrated by describing what happens in the microenvironment with heart disease. Due to its scope, this analysis is not all-inclusive. Rather, a pathway of inter-connectivity is identified within and between these six networks to influence the very cells that either help to maintain and restore health or foster chronic disease progression.
Two External Networks
A hierarchical network has distinct layers, that operate at different spatial and temporal scales. There are connections that occur among factors within a layer and between layers. Influences are generally top-down from one layer to the next, but it is possible to have emerging influences arise from the bottom up. As a result of this bi-directional potential, circular causality relationships among network levels can occur, creating self-regulating systems, such as negative feedback loops. These self-regulating systems can get disrupted, and either dysfunctional or spontaneous “new normal” causality relationships can develop as part of the body’s effort to adapt (Noble et al., 2019). In this network analysis there are six layers (Figure 1). Starting at the top there are two external networks that influence lifestyle.
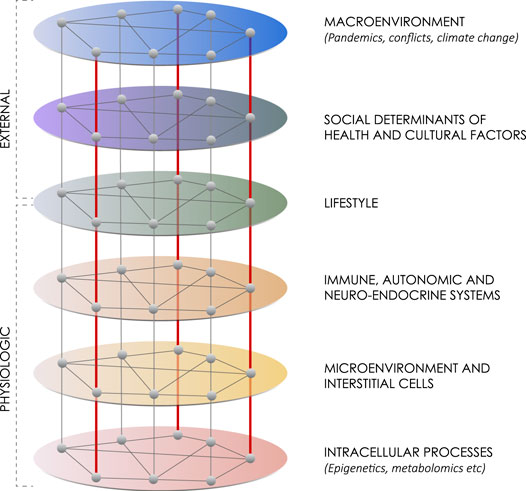
FIGURE 1. Lifestyle within a six-layer hierarchical network. This network diagram summarizes six layers of influences on maintaining health or advancing chronic disease. The first network includes macroenvironment influences like major weather, climate or political events, such as war. The second network includes social determinants of health and cultural factors. The third network includes lifestyle itself made up of physical activity, diet and stress or well-being. The fourth network includes the interacting immune, autonomic and neuroendocrine systems. The fifth network includes the interactions among interstitial cells within the microenvironment. The sixth network includes intracellular processes, such as epigenetics, genomics, transcriptomics and metabolomics. Although generally influences work from the top down, there can also be an emergence of influence from the bottom up.
The Larger Macroenvironment
The two external networks that influence lifestyle are the larger macroenvironment and the local social and cultural environment. The larger macroenvironment is a network of interacting environmental and geopolitical factors. The COVID-19 pandemic is a stark example of this. By June 2022, there were over half a billion cases and over 6 million deaths reported worldwide (World Health Organization, 2022). Due to lockdowns in many parts of the world, even those who did not get ill, had been socially isolated, stressed and had become less active (Mukhtar, 2020). Other macroenvironmental factors including extreme weather events, such as flooding and heat waves, and political events such as war, all profoundly affect lifestyle and health (Rocque et al., 2021; Shahin et al., 2021).
The Social and Cultural Environment
The second external network includes the social determinants of health and other cultural factors. When almost three in four American adults are overweight or obese (Fryar et al., 2020), this suggests more is at play than individual choice. Social determinants of health have identified that a healthy lifestyle is much easier to realize and maintain for those who have economic stability and access to healthy activities, health services and social support, than for those who do not (CDC, 2021). Although this is true, it is more complex than that (Frank et al., 2020). People’s lifestyle choices are profoundly shaped by their culture, family history and the social networks they are embedded in (Zhang et al., 2018), and this can trump the social determinants of health. For example, affluent executives may have all the social determinants of health but if they are constantly working and do not find time to eat a healthy diet or exercise, they are likely to develop a lifestyle-related chronic disease. The high prevalence of stress, screen-based activities and a diet high in fat and sugar in Western countries are part of Western culture. Fortunately, there are other culturally congruent influences for a healthy lifestyle, and this is the one level in the hierarchical network where personal choice can override countervailing influences.
Lifestyle as a Network
Definitions of healthy and unhealthy lifestyles vary. Although there is general agreement that a healthy lifestyle includes regular physical activity and a healthy diet some definitions also include smoking cessation, social and psychological well-being, moderate to no alcohol use (World Health Organization, 1999) and adequate sleep (Banks and Dinges, 2007). Most descriptions of an unhealthy lifestyle include some marker of psycho-social stress, an unhealthy diet that typically leads to obesity, sedentariness (Mainous et al., 2010), and sometimes addictions (Weinstock et al., 2017). Whereas obesity, excess alcohol and addictions can be seen as signs of dysregulation (Koob and Volkow, 2016; Becker, 2017; Narayanaswami and Dwoskin, 2017), some aspects of a healthy lifestyle, such as regular physical activity and normal weight can be seen as signs of self-regulation (Campos-Uscanga et al., 2017). And there is a growing recognition of the impact of social isolation. A recent meta-analysis of over 1,000 studies and almost 1.5 million participants found loneliness had an effect size similar to smoking and obesity in terms of the increased risk of chronic disease and mortality (Vila, 2021). For this analysis, the key features of a healthy lifestyle are regular physical activity, a healthy diet typically associated with self-regulation and some marker of psycho-social health. The key features of an unhealthy lifestyle include some marker of psycho-social stress, sedentariness and an unhealthy diet often linked with dysregulation and obesity. (Table 1)
Lifestyle is a type of network in that its key features interact with each other. In an unhealthy lifestyle, for example, sustained levels of ambient stress often lead to dysregulation. Binge eating, binge drinking, and addictions are ways to seek stress-relief. When people are dysregulated, it is difficult to start and maintain a regular exercise program. With progressive sedentariness, it is easier to gain weight. In contrast, the foundational feature of a healthy lifestyle is regular physical activity (Mok et al., 2019), which has been shown to not only improve mood and decrease anxiety (Rodriguez-Ayllon et al., 2019), but also improve memory and executive functioning (Hoffmann et al., 2021)—making it easier to self-regulate and socialize.
In summary, the first three networks in this analysis include the macroenvironment of environmental and geopolitical factors, local social and cultural factors, and lifestyle itself. Lifestyle marks the pivot point between external networks and internal networks. So, what are the internal networks in the body that interact with lifestyle?
The Immune, Autonomic and Neuro Endocrine Network
The fourth network is the mesoenvironment that mediates between the macroenvironment and microenvironment. It includes three interacting regulatory systems of the body: the immune, autonomic and neuroendocrine systems. These systems constitute a network, as there are interactions among them. So how do these interacting regulatory systems respond to a healthy and an unhealthy lifestyle?
The Immune System
The innate immune system responds to apparent threats, be they infectious, traumatic or physiologic, and plays a central role in maintaining health. When the immune response gets dysregulated, it leads to chronic low-grade inflammation. The quintessential cell driving this process is the macrophage.
The macrophage is one of the most ubiquitous and protean cells in the body. Although best known as a white blood cell that circulates in the blood, there are also “resident macrophages” that are present in all organs and tissues. The macrophage displays multiple phenotypes or forms of cellular behaviour. In its steady state, M0 macrophages have a surveillance capacity. When exposed to hypoxia or damage-associated molecular patterns (DAMPs), macrophages change into an M1 inflammatory state and release pro-inflammatory cytokines. Cytokines are signalling molecules that are released into the microenvironment and then into the blood stream to attract cells both locally and systemically to assist in responding to the threat. Macrophages can literally engulf and consume microbes as well as cellular debris. Once the source of the threat is neutralized, macrophages go into a recuperative M2 phenotype. M2 macrophages release anti-inflammatory cytokines and assist with tissue repair and angiogenesis. Once that is complete, macrophages return to their quiescent, M0, surveillance state.
Lifestyle and the Immune System
Food and physical activity have a profound effect on the immune system. In general, not getting enough to eat is associated with immunosuppression (Alwarawrah et al., 2018) and getting too much to eat is associated with low-grade inflammation. Food interacts with our innate immune system primarily through the gastro-intestinal tract and the microbiome. Macrophages line the intestinal tract (Chiaranunt et al., 2021). A healthy diet and a normal body mass index (BMI) are known to foster a healthy microbiome and anti-inflammatory bacteria (Sohail et al., 2019; Chen et al., 2021). Conversely an unhealthy diet high in carbohydrates and saturated fats is known to decrease diversity in the microbiome and increase pro-inflammatory bacteria (Tilg et al., 2020; Malesza et al., 2021). An unhealthy diet is associated with weight gain and the accumulation of fat cells that are filled with macrophages that produce adipokines—a type of pro-inflammatory cytokine that causes low-grade inflammation (Ouchi et al., 2011; Wynn et al., 2013).
Physical activity has both direct and indirect beneficial effects on the immune system. Skeletal muscle has been described as an immune regulatory organ as it generates a range of proteins, such as myokines, that have an anti-inflammatory effect (Duggal et al., 2019). Endurance training has been shown to increase anti-inflammatory myokines and interleukin (IL)-13 (Knudsen et al., 2020; Seaborne and Sharples, 2020). A recent meta-analysis of over 25 studies of people who were overweight or obese, exercise training decreased circulating pro-inflammatory cytokines (Gonzalo-Encabo et al., 2021). Physical activity also has an indirect effect on the immune system by fostering a healthy microbiome and this has been shown to increase immune competence (Krüger et al., 2016; Chen et al., 2021).
In contrast, sedentariness has a number of adverse effects on the immune system. Sedentariness fosters an inflammatory microbiome (O’Toole and Shiels, 2020), increases circulating pro-inflammatory cytokines (Bergens et al., 2021), and impairs the anti-inflammatory myokine response (Leal et al., 2018). The association of sedentariness and low-grade inflammation remains even when confounding factors, such as intermittent physical activity, BMI, hyperglycaemia and obesity are accounted for (Henson et al., 2013). Sarcopenia, or a progressive loss of muscle mass, strength and power, was initially linked with aging, but increasingly it is linked with sedentariness (Suzuki, 2019; Shur et al., 2021). And because sedentariness and obesity are both common, they often occur together leading to sarcopenic obesity (Batsis and Villareal, 2018), making the immune-related benefits of exercise all the more challenging to restore.
Psycho-social factors appear to largely interact indirectly with the immune system through the autonomic and the neuro-hormonal systems.
The Autonomic Nervous System
The autonomic nervous system (ANS) and the immune system often provide an integrated response to perceived threats (Bhowmick et al., 2009; Shouman and Benarroch, 2021). Tissue disruption will evoke both an immune cell reaction and a sympathetic response—be it from injury, infection or pathology arising in the parenchymal cells of an organ (Bellinger and Lorton, 2014). Furthermore, a sustained sympathetic response can disrupt the immune response (Bellinger and Lorton 2014; Kenney and Ganta 2014). Prolonged stress, from recurrent physical threats, anxiety, uncertainty and negative social experiences causes low-grade sympathetic overdrive (Eisenberger et al., 2017). Sympathetic neurons release an unremitting stream of norepinephrine which bind to adrenergic receptors on the surface of macrophages and amplify the release of pro-inflammatory cytokines (Bellinger and Lorton 2014). (Figure 2) In contrast, good social experiences and psycho-social support stimulate the parasympathetic and neuroendocrine systems (Haykin and Rolls, 2021), and create a buffering effect on stress and inflammation (Vila, 2021).
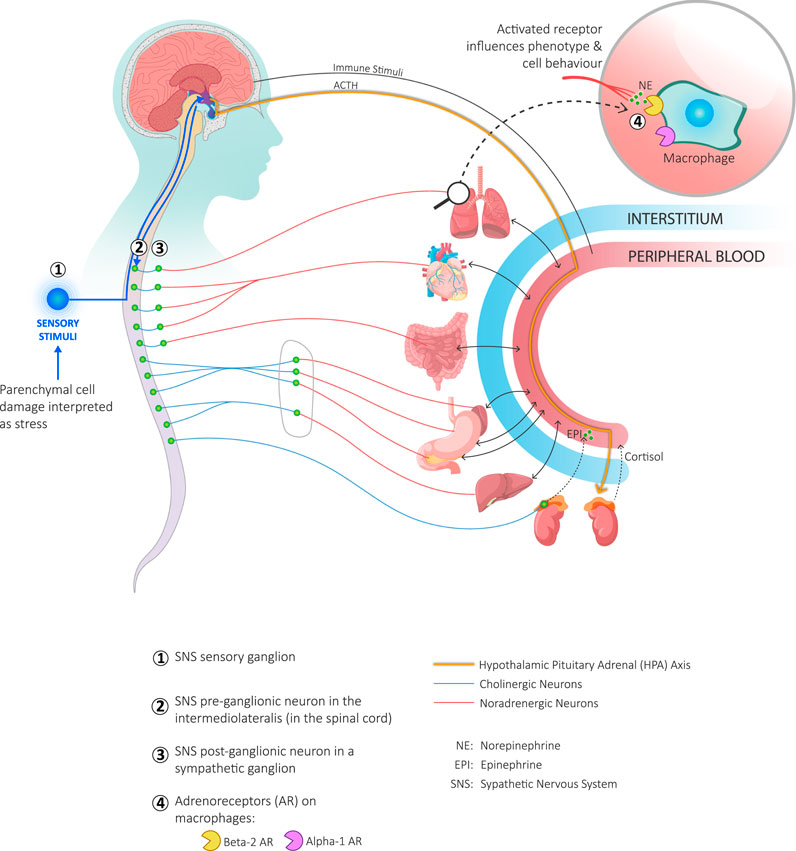
FIGURE 2. Coordination of the immune and sympathetic nervous systems with the hypothalamic-pituitary-adrenal (HPA) axis in response to parenchymal damage. Local parenchymal cell damage is interpreted as a threat by interstitial cells and as stress by sympathetic nerves. Interstitial cells respond to threats by releasing cytokines (not shown). Local sensory neurons respond to stress by activating the afferent branch of the sympathetic nervous system (SNS) and sending an impulse to the hypothalamus (lower left-hand corner of the figure). The hypothalamus responds to the information received from both the circulating cytokines and SNS stimulation (Bellinger and Lorton, 2014) in two ways: it stimulates both the efferent branch of the SNS as well as the HPA axis. Stimulation of the efferent SNS goes through the preganglionic neuron in the intermediolateral nucleus of the spinal cord (green circles). Stimulated preganglionic neurons transmit the signal through a cholinergic axon to post-ganglionic neurons (green circles) and, in a metameric fashion, through a noradrenergic axon to the affected organ where norepinephrine is released. A preganglionic neuron may also transmit directly to chromaffin cells in the adrenal medulla which in turn stimulates the release of epinephrine directly into the blood stream. When the HPA axis is stimulated, the hypothalamus releases corticotropin releasing hormone that stimulates the release of adrenocorticotrophic hormone (ACTH) from the anterior pituitary. ACTH binds to receptors on the adrenal cortex and stimulates the release of cortisol into the blood stream. Both epinephrine and norepinephrine interact with adrenergic receptors on immune cells, including macrophages (upper right-hand corner of the figure). This activates signalling pathways that constitutes one of the influences on the phenotype and behaviour of macrophages.
Regular physical activity protects against the upregulation of inflammatory cytokines and can dampen the sympathetic response (Alemasi et al., 2019). Daily exercise stimulates a parasympathetic response via the vagal nerve and cholinergic anti-inflammatory pathways. Acetylcholine released from the vagus nerve attenuates the release of pro-inflammatory cytokines (Lujan and DiCarlo, 2013; Kenney and Ganta, 2014). The vagal nerve is best known for its effect upon the heart, resulting in a lower basal heart rate and a lower heart rate during submaximal exertion (Lujan and Dicarlo, 2013). Some mind-body exercises, such as yoga and tai chi, are known for their parasympathetic effect as measured by increased heart rate variability (Zou et al., 2018). The parasympathetic nervous system only directly innervates a few organs. Most of its anti-inflammatory effects, such as in the gut, are mediated through the cholinergic anti-inflammatory pathway (Goverse et al., 2016).
The autonomic nervous system is involved in regulating feeding behaviour. Afferent vagal nerves in the gut transmit information to the hypothalamus that stimulate efferent nerves involved with the release of leptin in adipose tissue and ghrelin in the stomach (Imai and Katagiri, 2022). Diet affects the microbiota, and the microbiota and the brain communicate with each other via the enteric nervous system as well as the immune and autonomic systems (Yoo and Mazmanian, 2017; Millar et al., 2021) forming what is known as the Microbiota-Gut-Brain axis (Cryan et al., 2019). Adverse alterations of this axis lead to gut dysbiosis that has been linked to hypertension and kidney disease (Yang et al., 2018), stroke (Battaglini et al., 2020), metabolic syndrome, diabetes, obesity (Westfall et al., 2017) and Alzheimer’s disease (Megur et al., 2020). Physical activity has an influence on the Microbiota-Gut-Brain axis. For example, in people with pre-diabetes, exercise-induced alterations in the gut microbiota were found to improve glucose control and insulin sensitivity (Liu et al., 2020).
The Neuroendocrine System
The neuroendocrine system includes the hypothalamic, pituitary and adrenal (HPA) axis, the amygdala and mediating hormones and is linked to both the ANS and the immune system through the hypothalamus. The HPA axis coordinates the neural and endocrine responses to both stress and relaxation. A healthy lifestyle is linked with relaxing activities that stimulate a parasympathetic response. Positive social interactions stimulate both a parasympathetic response and the release of oxytocin, also known as the “bonding hormone” (Jurek and Neumann, 2018). Other neurohormones that interact in this network are melatonin and dehydroepiandrosterone sulfate (DHEAS) (Luo et al., 2020; Noushad et al., 2021). Melatonin has been shown to influence immune cell phenotype (Anderson and Reiter, 2020). A recent meta-analysis of 31 studies found melatonin had significant anti-inflammatory effects (Cho et al., 2021), but this effect may be lessened with insomnia (Nicolaides et al., 2020).
When an unhealthy lifestyle activates an inflammatory response, the sympathetic nervous system (SNS) and the HPA axis are also activated. To counteract the inflammation, cortisol is released from the adrenal gland to calm the inflammation. When inflammation becomes chronic, however, there is an uncoupling of the HPA axis from the SNS. Cortisol levels drop off and this uncoupling may contribute to chronic disease progression (Kenney and Ganta, 2014; Bennett and Sturmberg, 2016).
Although cortisol is the main hormone released to moderate the response to stress, melatonin and other neurohormones are also involved (Noushad et al., 2021). Stress and binge eating increases the release of the hormone ghrelin that increases appetite, thus creating a positive feedback loop (Bremner et al., 2020). In addition, leptin is an adipocyte-derived hormone that has an effect on both the immune and metabolic systems and is associated with the inflammatory state found in obesity and metabolic syndrome (Pérez-Pérez et al., 2017).
The different regulatory systems interact through cytokines, other biosignalling molecules, and neural stimulation. So how does this signalling occur? How is perceived threat information received and responded to locally and systemically and how does this relate to chronic disease progression?
Intercellular Crosstalk
Intercellular crosstalk is the primary method of communication between the regulatory systems of the mesoenvironment and the immune and connective tissue cells in the microenvironment. There are a plethora of signalling molecules that mediate both pro-inflammatory and anti-inflammatory activities. These signalling molecules are picked up locally and remotely and are influenced by lifestyle-stimulated changes in the gut, brain, muscle and fat.
There are a number of aspects to this process. Chemokines and DAMPs attract macrophages and other white blood cells to a site of damage or infection. This typically leads to the release of pro-inflammatory cytokines, such as TNF-alpha, IL-1, IL-6, IL-8 and IL-12. Under other stimuli, anti-inflammatory cytokines are released such as IL-4, IL-10, and IL-13. Transforming growth factor beta (TGF-beta) is essential for the development of myofibroblasts and are initially anti-inflammatory but when sustained become inflammatory (Xu et al., 2018; Koliaraki et al., 2020). The nuclear factor kappa beta (NF-κB) signalling pathway links pathogenic and cellular danger signals. The MAPK signalling pathway transports signals through the cell membrane to the nucleus of the cell (Table 2).
Crosstalk occurs not only locally among cells but also between tissues. For example, there are a number of myokines released by muscle during exercise, that circulate in the blood stream and affect the brain by enhancing brain-derived neurotrophic factor (BDNF) production. This is thought to be linked to how exercise increases mental health and executive function (Pedersen, 2019). Myokines also interact with fat tissue. With regular aerobic activity, myokines have been linked with the browning of adipose tissue and may be one of the ways that physical activity improves body composition (Leal et al., 2018). White adipose tissue generates cytokines, hormones and growth factors that target peripheral tissues, and this has been linked with the development of metabolic syndrome, although the exact mechanism is not fully understood (Masoodi et al., 2015). So where does this crosstalk actually occur? Although biosignalling molecules are transported through the blood, a lot of intercellular crosstalk occurs in the microenvironment—and this constitutes the fifth network.
The Microenvironment Network
The fifth network is the microenvironment, which refers to the microscopic conditions of a specific part of the body. A myocardial infarction creates a microenvironment in the heart. Pancreatic cancer creates a tumor microenvironment in the pancreas. The microenvironment is part of the interstitium that includes a microscopic reticular network of extracellular matrix (ECM) which permeates all tissues and organs and provides structure and support to organ parenchymal cells. There is recent evidence that the interstitium is continuous with surrounding fascia (Cenaj et al., 2021). Once thought to be a simple and inert web-like substance, the interstitium is now known to be a complex and dynamic fluid-filled space that responds to local, systemic and external influences. It is precisely because of the local responsiveness of interstitium, that there are many different microenvironments in the body.
In addition to the ECM, the interstitium consists of interstitial fluid, interstitial cells, and nerve fibers (including sympathetic nerves), that coil around local capillaries (Cole et al., 2015) (Figure 3). Interstitial fluid arises from the blood by diffusing out of capillaries. (This fact is used by continuous glucose monitors that measure glucose in the interstitial fluid as a proxy for a blood glucose measurement.) This bioactive fluid circulates through the interstitium, interacts with interstitial cells and then is eventually drawn into lymphatic channels and back into the venous circulation (Scallan et al., 2010; Wiig and Swartz, 2012). Interstitial cells include macrophages and other immune cells, connective tissue cells called fibroblasts, stem cells and other cells that vary by location.
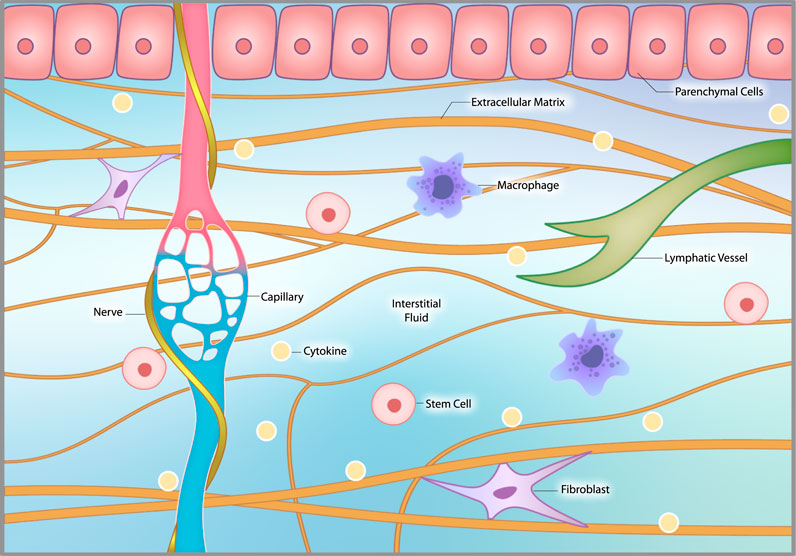
FIGURE 3. The interstitial microenvironment. The interstitium consists of extracellular matrix (ECM), interstitial fluid, interstitial cells and nerve fibers, including sympathetic nerves, that coil around local capillaries. The ECM provides structural support to organs and tissues. Interstitial fluid arises from the blood by diffusing out of capillaries. It circulates through the interstitium, interacts with interstitial cells and then is drawn into lymphatic channels and back into the venous circulation. Interstitial cells include macrophages, fibroblasts, occasional stem cells and other related cells that vary by location.
So how does lifestyle affect the interstitium and local microenvironments, and how does this affect chronic disease progression? It appears that lifestyle affects interstitial cells.
Interstitial Cells
There are two main types of cells in the body: parenchymal cells and interstitial cells. Parenchymal cells are organ and tissue specific, such as pneumocytes in the lung, hepatocytes in the liver and myocytes in muscle. Interstitial cells are found in every organ and tissue in the body alongside the parenchymal cells and play a major role in both the normal healing process and chronic disease progression.
The two main interstitial cells are macrophages (the immune cells that have already been described) and fibroblasts. Fibroblasts are connective tissue cells that produce the ECM, which provides the scaffolding of organs and tissues. Fibroblasts also play a central role in the maintenance and repair of virtually every tissue and organ in the body, and have been described as “tutoring the immune system” (Correa-Gallegos et al., 2021). Most interstitial macrophages and fibroblasts are of fetal origin and are called resident cells. Other macrophages and fibroblasts are derived from myelopoietic stem cells in the bone marrow. These stem cells differentiate into monocytes that circulate in the blood (Clarke, 2019), and then turn into either macrophages or fibroblasts in the interstitium. Stem cells are also present in the interstitium but not all have a regenerative capacity. Stem cells in the liver can transform into hepatocytes but stem cells in the heart cannot transform into cardiomyoctyes; it is not known why (Psarras et al., 2019).
Much like cell plasticity in the brain, both macrophages and fibroblasts display tremendous plasticity. Macrophages form microglial cells in the brain, osteoclasts in bone, Kupffer cells in the liver, foam cells in atherosclerotic plaques and can also turn into fibroblasts (Haider et al., 2019). Fibroblasts can turn into stellate cells in the liver, telocytes and dendritic cells throughout the body, and differentiate into other connective tissue cells to form cartilage, tendons, ligaments and fascia (Montero et al., 2012). Fibrocytes can also revert back to stem cells (Steens et al., 2020) and become macrophages or even parenchymal cells (Inoue et al., 2019; Ieda, 2020). Fibroblasts and mesenchymal stem cells have been referred to as “two sides of the same coin” (Soundararajan and Kannan, 2018), and both can turn into myofibroblasts (Bochaton-Piallat et al., 2016) that are central to scar formation and fibrosis.
So, how does cell plasticity occur–or get impeded? What directs cells to change phenotype and their behaviour? What determines whether macrophages and fibroblasts work towards health or disease?
The Normal Healing Process
Although the sources of injuries and chronic diseases vary widely, the body’s response to any injury or disruption is remarkably similar. Healing takes place in three consecutive phases: an inflammatory phase; a repair phase; and a final remodelling phase.
The initial inflammatory phase is an essential part of the healing process. It is typically triggered by tissue hypoxia and/or cell death. Resident and circulating macrophages changing from a quiescent M0 surveillance state into an M1 phenotype, release pro-inflammatory cytokines and this stimulates the recruitment of circulating monocytes, leukocytes and other cells. After M1 macrophages phagocytose dead cells, they present the debris to lymphatic channels for processing. Fibroblasts are also activated and release cytokines. They proliferate, migrate to the damaged tissue, and produce large amounts of ECM to isolate and repair the damaged tissue. In the case of deep wounds, myofibroblasts mobilize a prefabricated plug of interstitium-like fascia containing blood vessels, nerves, ECM and macrophages to fill and seal a wound (Correa-Gallegos et al., 2019).
In the repair phase, macrophages have a predominately M2 phenotype and release anti-inflammatory cytokines. They also support stem cell differentiation, angiogenesis, and matrix remodeling. (Chazaud, 2020; Weavers and Martin, 2020). Fibroblasts work in concert with macrophages by producing growth factors and anti-inflammatory cytokines (Psarras et al., 2019).
During the remodelling phase, fibroblasts realign the extracellular matrix with the biomechanics of the local tissue. When completed, fibroblasts return to their quiescent state, to maintain the ECM and support stem cell maintenance in specific niches (Koliaraki et al., 2020). This process has been captured on film in a mouse model of traumatic brain injury (NIH 2018; Russo et al., 2018).
Chronic Disease Progression
Chronic low-grade inflammation occurs when this three-phase healing process gets stalled (Koliaraki et al., 2020). Specifically, when the healing process is arrested in the inflammatory phase it leads to chronic low-grade inflammation. This appears to occur when there are repetitive conditions or chronic insults that recurrently stimulate an inflammatory response (Wynn et al., 2013). This is precisely what occurs with an unhealthy lifestyle. Sedentariness, poor diet and chronic stress are recurrent stimuli for inflammatory cytokines. The effects of an unhealthy lifestyle foster the dysregulation of macrophages that then remain in an M1 phenotype. Transforming growth factor-β (TGF-β) released by lymphocytes and macrophages appears to be one of the primary factors that leads to dysregulation in fibroblasts (Wynn, 2008; Meng et al., 2016). Dysregulated fibroblast and myofibroblast activity lead to tissue fibrosis. A vicious cycle is then established as the dysregulated fibroblasts create increased tissue stiffness, which in turn stimulates dysregulated macrophages to produce more pro-inflammatory cytokines (Solis et al., 2019; Sridharan et al., 2019).
Chronic inflammation and fibrosis ultimately destroy the parenchymal tissue and leads to loss of function (Shapouri-Moghaddam et al., 2018). This specter of chronic low-grade inflammation and progressive fibrosis followed by increasing parenchymal dysfunction is remarkably similar whether it occurs in the lung (Knudsen et al., 2017), liver (Kisseleva and Brenner, 2021), heart (Frangogiannis 2019), kidney (Sulikowska et al., 2015; Meng, 2019; Lin et al., 2020), or musculo-skeletal tissue (Mahdy, 2019). Fibrosis is one of the hallmarks of end-stage chronic disease, for which there is currently no treatment.
Heart Disease and the Microenvironment
The microenvironment in chronic disease is best understood in the heart. Unhealthy lifestyle factors activate endothelial cells lining coronary arteries to release a broad-spectrum of pro-inflammatory cytokines (Akhtar and Sharma, 2021). This attracts immune cells such as macrophages which turn into foam cells and contribute to atherosclerosis and unstable plaques (Chinetti-Gbaguidi et al., 2015). When a plaque is disrupted and blocks a coronary artery, it leads to angina and myocardial infarction.
The space between cardiac myocytes is the cardiac interstitium, which contains abundant macrophages and fibroblasts (Van Linthout et al., 2014; Pogontke et al., 2019). Hypoxic myocardial cells release DAMPs, which change macrophages from surveillance M0 phenotype to inflammatory M1 phenotype. M1 macrophages release pro-inflammatory cytokines that attract monocytes, leukocytes, and other cells to the area. (Pogontke et al., 2019; Psarras et al., 2019; Kuna et al., 2022). Monocytes mature in the damaged myocardium to create more M1 macrophages that pump out more pro-inflammatory cytokines activating fibroblasts and myofibroblasts. Fibroblasts start to pump out ECM to stabilize the injured myocardium (Haider et al., 2019). When dysregulated, myeloid cells, fibroblasts and stem cells convert to myofibroblasts, and progressive fibrosis ensues. The increasing stiffness in the myocardium and the leads to the development of heart failure (Eckhouse and Spinale 2012; Schelbert et al., 2019). Fibrosis in the heart is exacerbated when fibroblasts and stem cells adopt an osteoclastic phenotype, leading to calcified areas in cardiac tissue and atherosclerotic cardiac arteries (Psarras et al., 2019; Vergallo and Crea, 2020).
Obesity and metabolic syndrome increase sympathetic tone (Grassi et al., 2019; Bakkar et al., 2020) and can lead to the deposit of adipocytes in the heart, increasing low-grade inflammation (Berezin et al., 2020). Chronic sympathetic overdrive stimulates inflammation, and a progressive autonomic neuropathy develops in the heart associated with fibrosis. To counteract this, there have been calls for trials to treat heart failure with oxytocin to foster a parasympathetic response (Gutkowska and Jankowski 2012; Dyavanapalli, 2020; Jankowski et al., 2020).
Initial attempts to use stem cell therapies post-myocardial infarction failed. Rather than turn into cardiomyocytes, the stem cells appeared to turn into myofibroblasts and only increased the fibrosis (Packer, 2018). More recent work suggests that stem cells with a chemical inducer may help convert macrophages into a more anti-inflammatory phenotype and modify the activity of cardiac fibroblasts to make less ECM (Vagnozzi et al., 2020). Some early research suggests fibroblasts could then be induced to convert to cardiomyocytes (Cao et al., 2016).
In summary, macroenvironmental factors influence lifestyle which affect the immune, autonomic and neuro-hormonal systems in the body to influence both recovery from organ damage and chronic disease progression. Macrophages and fibroblasts in the microenvironment orchestrate the recovery process. When macrophages and fibroblasts become dysregulated, recovery stops and chronic low-grade inflammation and fibrosis begin. If perpetuated, inflammation and fibrosis lead to parenchymal damage and end-stage chronic disease. So, the final question is: How do macrophages and fibroblasts dysregulate? How does lifestyle actually change cell behaviour? This brings us to the sixth and final network.
The Intracellular Network
It has been said: The “M1/M2 macrophage balance… governs the fate of an organ” (Shapouri-Moghaddam et al., 2018). Ultimately, chronic disease progression occurs when macrophages get stalled in an M1 phenotype and the anti-inflammatory M2 phenotype associated with healing cannot take hold. So, what determines phenotype? A number of interacting factors are involved. Addressing what is known involves delving into the intracellular network and the different types of macrophage metabolism.
Yamasaki and Eeden have asserted that macrophages phenotype and function depend primarily on the microenvironment in which they reside (Yamasaki and Eeden, 2018). Support for this includes that fact that the microenvironment reflects the neural and endocrine influences that arise from lifestyle as described above (Shapouri-Moghaddam et al., 2018; Locati et al., 2020).
Zhang and colleagues have identified that it is epigenetics, or different forms of gene expression, that determine cellular phenotype (Zhang et al., 2020). Epigenetic changes often occur in response to changes in the microenvironment. But this is not simple. For example, almost 40 different genes have been linked to M1 and M2 polarization (Murray, 2017). Locati and colleagues have discovered that it is largely one type of epigenetic change - microRNA networks - that underlie the adaptability of macrophages to different environmental cues (Locati et al., 2020). MicroRNAs can be produced locally or find their way into the microenvironment via the blood stream. Olivieri and colleagues have found that it is specifically the non-coding microRNAs (miRNAs), miR-21-5p and miR-146a-5p, interacting with NF-κB-driven inflammatory pathways, that mediate chronic low-grade inflammation (Olivieri et al., 2021).
But what drives the epigenetics? Díaz-Bulnes and colleagues have discovered that macrophage activation and polarization are closely linked with metabolic rewiring (Díaz-Bulnes et al., 2020). Specifically, the metabolism in macrophages changes, depending on whether they are under physiologic or stressed conditions (Peruzzotti-Jametti et al., 2021). Under physiologic conditions, cells use oxidative metabolism and the tricarboxylic acid (TCA) cycle. The proinflammatory functions of the M1 phenotype create high energy demands that overwhelm the TCA cycle so there is a switch to glycolytic metabolism (Zhu et al., 2021). This “Warburg effect” occurs in both chronic disease and cancer microenvironments—but there is a big difference between the two (Certo et al., 2021). Whereas the microenvironment in chronic disease is associated with dysfunctional M1 macrophages and inflammation, the microenvironment in cancer tissues is associated with dysfunctional M2 macrophages and immunosuppression (Hinshaw and Shevde 2019). In addition, metabolites released into the microenvironment reflect more than cell metabolism. Recent advances in metabolomics have identified that metabolites also act as master regulators of all the other “omics.” In other words, metabolites have been shown to influence gene expression (genomics), mRNA metabolism (transcriptomics), protein activity (proteomics), and cell physiology (Rinschen et al., 2019).
Ultimately, all these factors influence macrophage behaviour. Epigenetic mechanisms are turned on or off in response to changes in the microenvironment and stimulate phenotypic shifts which change the cellular metabolism within macrophages. The subsequent metabolites can then trigger new epigenetic events (Jha et al., 2015; Saha et al., 2017; Zarzour et al., 2019) that induce other changes (Zaghlool et al., 2020). The relative weight of these, and other influences, are only starting to be mapped out.
This completes the six-layered hierarchical network analysis. Level one identifies that environmental and geopolitical events can adversely affect lifestyle. Level two identifies that people are embedded in a social and cultural context that profoundly affects lifestyle. Level three considers the interactions among the different aspects of lifestyle itself. Level four explores how the regulatory systems of the body—the immune, autonomic and neurohormonal systems—respond to lifestyle factors and how they interact with each other. Level five outlines how the immune, autonomic and neuro-hormonal responses to lifestyle affect macrophages and fibroblasts in the microenvironment in ways that either help maintain or regain normal tissue function or contribute to chronic disease progression. And level six considers how the phenotype or behaviour of macrophages and fibroblasts are determined by multiple interacting factors in the microenvironment, such as cytokines, hormones, neurotransmitters, epigenetics, and metabolites. The effects of lifestyle at all these levels are summarized in Table 3.
Discussion
This is the first time the theory has been put forward that the reason why lifestyle is linked with the primary causes of mortality is not because lifestyle affects the parenchymal cells of the heart, lungs or brain, but because it affects interstitial cells. This is based on the growing body of evidence that chronic disease progression arises from a stalled healing process orchestrated by interstitial macrophages and fibroblasts in response to a perceived threat. Network analysis was used to identify how the microenvironment, as well as social and cultural factors, influences lifestyle and how lifestyle, in turn, affects regulatory systems in the mesoenvironment and interstitial cells in the microenvironment. The analysis showed that an unhealthy lifestyle causes chronic low-grade inflammation through the dysregulation of immune cells causing chronic disease progression, and by the dysregulation of connective tissue cells causing fibrosis, that eventually interferes with parenchymal function.
Strengths and Limitations
The strength of this theory is that it connects evidence about lifestyle with recent advances in understanding inter and intra-cellular dynamics associated with chronic disease progression. Insights into the cellular behaviour of macrophages and fibroblasts in the microenvironment have started to change how we see chronic disease and its treatment. The importance of fibrosis in chronic disease progression is only beginning to be more widely appreciated. It has recently been estimated that 40% of all deaths in the industrialized world is due to fibrosis (Friedman, 2022). And insights into interstitial cell dysregulation are starting to be incorporated into new treatment strategies. The first macrophage modifying medication has been approved for the treatment of diabetic foot ulcers (Huang et al., 2021), and a new chimeric antigen receptor (CAR) T cell is under development that uses technology from mRNA vaccines to destroy myofibroblasts in the heart, resulting in decreased fibrosis and improved myocardial function (Rurik et al., 2022). Although the microenvironment in chronic disease progression is only starting to be explored, the importance of the microenvironment in cancer is well-established. It is well-accepted that inflammatory cells are an indispensable part of cancer cell survival, proliferation, and spread (Coussens and Werb, 2002; Hinshaw and Shevde, 2019) and that this is mediated by metabolomics and epigenetics (Elia and Haigis, 2021). Several epigenetic drugs have now been authorized for cancer treatment (Bates, 2020).
The key weakness of this theory—and of network theory in general—is that it is not always clear how strong the different interactions and influences are within and between different networks. So, although important interactions between sympathetic neurotransmitters and macrophages have been identified (Bennett et al., 2018), how important these interactions are, remain unclear. The macroenvironmental influences on lifestyle have typically been underemphasized. And the relative influence of local versus circulating inflammatory cytokines remain unknown. Is there a competing effect between inflammatory adipokines from obesity and anti-inflammatory myokines from physical activity? We simply do not know. The relative strength of different influences is starting to be estimated by interactomics. A recent interactomic study of obesity found autonomic dysfunction was a hub, suggesting it has a significant influence (Geronikolou et al., 2017). Another weakness of network analysis is that it is difficult to know if all the influences and interactions have been included. For this analysis, it was clearly noted that the network described was not all-inclusive. It was silent on the effects of smoking and air pollution for example, in part because these are already well-known. There are most certainly others. Network theory lends itself to increasing complexity. The goal with this analysis was simply to identify a path from the macroenvironment through the mesoenvironment to the microenvironment; there remains much to explore.
There are other limitations to consider. First, this theory provides no insight into the actual etiology of chronic diseases. The theory explains chronic disease progression, not etiology. Why a chronic disease process takes hold in the heart, instead of the brain or the kidney is only generally addressed by the concept of repetitive insults. Second, lifestyle is an umbrella term that has been defined in multiple ways and although epidemiologic and outcome studies are compelling, what factors should be included, and the relative weights of those factors, is still being sorted out (Lohse et al., 2016). Third, our understanding of interstitial cells is still under development. It well-known, for example, that characterizing macrophage phenotypes as M0, M1 and M2 is vastly oversimplified (Murray 2017). Other transitional phenotypes have been described and completely distinct phenotypes are being discovered—such as “cloaking” macrophages that surround an injured area to protect it from an overreaction by T cells (Uderhardt et al., 2019). And there is much that remains unknown, such as when does healthy acute inflammation become unhealthy chronic inflammation (Rohleder, 2019) or why does the risk of a stalled M1 phenotype tend to increase with age?
Future Research and Clinical Implications
This theory can be tested. For example, artificial intelligence and machine learning (AI/ML) studies are designed to identify patterns arising from multiple interacting variables. Thousands of people could be followed over time who have different diets, activities and levels of psycho-social stress or well-being. Data gathered would include the usual measures of weight, food intake, activity tracking, psychosocial assessments, BMI and health outcomes, as well as heart rate variability, VO2 max, and a plethora of cytokine, genetic, epigenetic and metabolic markers. Then all this data could be analyzed by AI/ML to see what emerges. A general proof of concept for this type of study has been completed (Price et al., 2017) and more focused studies have been done, such as gathering data from thousands of people to correlate different gut microbiome compositions with different lifestyles and clinical conditions (Manor et al., 2020). AI/ML studies are limited by the quality and extent of the data used and replication of results may prove to be difficult. The results would not be definitive, but could generate new hypotheses—about the relative weight of influences for example—that can then be tested clinically.
The broader field of network analysis has confirmed that chronic disease is a consequence of perturbed interactions among multiple biological networks rather than a single altered molecular pathway. This has important implications for clinical care and future research. Network analysis has identified sub-groups within diseases, such as diabetes and Parkinson’s disease, to help explain heterogenous outcomes and tailor treatment strategies (Sturmberg, 2021; Zhang et al., 2021) and can risk stratify patients with exercise intolerance (Oldham et al., 2018). Network physiology has identified there is a direct relationship between inter-organ connectivity and survival in the critically ill (Asada et al., 2016; Tan et al., 2020). Through network physiology, we now are beginning to understand how muscle affects the immune system, fat cells, the brain and other vital organs (McGee and Hargreaves, 2019; Gomes et al., 2020). And there have been new insights into the muscle denervation process that occurs with aging and why once sarcopenia is established, it becomes increasingly challenging to stop (Pascual-Fernández, 2020; Gustaffson and Ulfhake, 2021).
The big question is: Can this knowledge be used to help people adopt a healthier lifestyle? Understanding the network of interacting factors that influence health may contribute to the new concept of whole person health that encompasses interconnecting factors across physiological, psycho-social and environmental domains (Langevin, 2022). Understanding that interstitial cells throughout your body are either working to maintain your health or are starting to dysregulate and undermine your health may enable a more proactive approach to wellness, consistent with current trends in participatory and personalized medicine (Hood and Auffray, 2013; Topol, 2019; Lamb, et al., 2022).
Perhaps most importantly, understanding that lifestyle has an effect on cell behaviour provides a physiologic basis to the re-emerging interest in salutogenesis, or the study of how health is maintained and restored (Becker and Rhynders, 2013; Langevin, 2022). Salutogenesis can be applied to prevent and mitigate chronic disease. In contrast to the predominant health care strategy that focuses on interfering with parenchymal pathology and treating each chronic disease separately, a salutogenic strategy focuses on optimizing interstitial health with the potential to prevent or mitigate multiple chronic diseases simultaneously. Optimizing lifestyle is part of a salutogenic strategy but not all of it. For example, interactomics has been used to identify what drugs might be repurposed to help regulate interstitial cells and mitigate all chronic diseases (Lee et al., 2021). And salutogenic strategies are not limited to chronic disease. For example, there is now a tantalizing possibility that spinal cord injury may not have to incur permanent damage after it was shown that a transcriptome was all that was needed to activate a latent regulatory program to remyelinate nerve cells (Monje, 2021).
Conclusion
Chronic diseases represent a huge burden of illness for people and the health care system. As more becomes known about the hierarchy of interacting factors that influence interstitial cell behaviour, it is bound to change how chronic disease progression is understood and managed. Network physiology and network medicine can contribute to the emerging field of salutogenesis to help identify new ways to support healthy lifestyle change as well as new strategies to arrest cell dysregulation and restore health.
Data Availability Statement
The original contributions presented in the study are included in the article, further inquiries can be directed to the corresponding author.
Author Contributions
The author confirms being the sole contributor of this work and has approved it for publication.
Conflict of Interest
The author declares that the research was conducted in the absence of any commercial or financial relationships that could be construed as a potential conflict of interest.
Publisher’s Note
All claims expressed in this article are solely those of the authors and do not necessarily represent those of their affiliated organizations, or those of the publisher, the editors and the reviewers. Any product that may be evaluated in this article, or claim that may be made by its manufacturer, is not guaranteed or endorsed by the publisher.
Acknowledgments
Many thanks to Dr. Neil Theise for his insights into the interstitium and to Dr. Helene Langevin for her insights into fibroblast behaviour and promoting a renewed interest in salutogenesis, and my thanks to them both for feedback on early drafts of this manuscript. In addition, my thanks to Adam Krajewski, the graphic designer, who created these figures from my rough sketches.
References
Abraham G., Havulinna A. S., Bhalala O. G., Byars S. G., De Livera A. M., Yetukuri L., et al. (2016). Genomic Prediction of Coronary Heart Disease. Eur. Heart J. 37 (43), 3267–3278. doi:10.1093/eurheartj/ehw450
Ahmad F. B., Anderson R. N. (2021). The Leading Causes of Death in the US for 2020. JAMA 325 (18), 1829–1830. doi:10.1001/jama.2021.5469
Akhtar S., Sharma A. (2021). Endothelial Dysfunction Sustains Immune Response in Atherosclerosis: Potential Cause for Ineffectiveness of Prevailing Drugs. Int. Rev. Immunol. 41, 123–134. doi:10.1080/08830185.2020.1866568
Alemasi A., Cao N., An X., Wu J., Gu H., Yu H., et al. (2019). Exercise Attenuates Acute β-Adrenergic Overactivation-Induced Cardiac Fibrosis by Modulating Cytokines. J. Cardiovasc. Trans. Res. 12 (6), 528–538. doi:10.1007/s12265-019-09894-1
Alwarawrah Y., Kiernan K., MacIver N. J. (2018). Changes in Nutritional Status Impact Immune Cell Metabolism and Function. Front. Immunol. 9, 1055. doi:10.3389/fimmu.2018.01055
Anderson G., Reiter R. J. (2020). Melatonin: Roles in Influenza, Covid-19, and Other Viral Infections. Rev. Med. Virol. 30 (3), e2109. doi:10.1002/rmv.2109
Asada T., Aoki Y., Sugiyama T., Yamamoto M., Ishii T., Kitsuta Y., et al. (2016). Organ System Network Disruption in Nonsurvivors of Critically Ill Patients. Crit. care Med. 44 (1), 83–90. doi:10.1097/CCM.0000000000001354
Bakkar N.-M. Z., Dwaib H. S., Fares S., Eid A. H., Al-Dhaheri Y., El-Yazbi A. F. (2020). Cardiac Autonomic Neuropathy: A Progressive Consequence of Chronic Low-Grade Inflammation in Type 2 Diabetes and Related Metabolic Disorders. Ijms 21 (23), 9005. doi:10.3390/ijms21239005
Balagué N., Hristovski R., Almarcha M., Garcia-Retortillo S., Ivanov P. C. (2020). Network Physiology of Exercise: Vision and Perspectives. Front. Physiol. 11, 611550. doi:10.3389/fphys.2020.611550
Banks S., Dinges D. F. (2007). Behavioral and Physiological Consequences of Sleep Restriction. J. Clin. sleep Med. JCSM official Publ. Am. Acad. Sleep Med. 3 (5), 519–528. doi:10.5664/jcsm.26918
Bartsch R. P., Liu K. K. L., Bashan A., Ivanov P. C. (2015). Network Physiology: How Organ Systems Dynamically Interact. PloS one 10 (11), e0142143. doi:10.1371/journal.pone.0142143
Bates S. E. (2020). Epigenetic Therapies for Cancer. N. Engl. J. Med. 383 (7), 650–663. doi:10.1056/NEJMra1805035
Batsis J. A., Villareal D. T. (2018). Sarcopenic Obesity in Older Adults: Aetiology, Epidemiology and Treatment Strategies. Nat. Rev. Endocrinol. 14 (9), 513–537. doi:10.1038/s41574-018-0062-9
Battaglini D., Pimentel-Coelho P. M., Robba C., Dos Santos C. C., Cruz F. F., Pelosi P., et al. (2020). Gut Microbiota in Acute Ischemic Stroke: From Pathophysiology to Therapeutic Implications. Front. Neurol. 11, 598. doi:10.3389/fneur.2020.00598
Becker C. M., Rhynders P. (2013). It's Time to Make the Profession of Health about Health. Scand. J. Public Health 41 (1), 1–3. doi:10.1177/1403494812467506
Becker H. C. (2017). Influence of Stress Associated with Chronic Alcohol Exposure on Drinking. Neuropharmacology 122, 115–126. doi:10.1016/j.neuropharm.2017.04.028
Bellinger D. L., Lorton D. (2014). Autonomic Regulation of Cellular Immune Function. Aut. Neurosci. 182, 15–41. doi:10.1016/j.autneu.2014.01.006
Bennett J. M., Reeves G., Billman G. E., Sturmberg J. P. (2018). Inflammation-Nature's Way to Efficiently Respond to All Types of Challenges: Implications for Understanding and Managing "the Epidemic" of Chronic Diseases. Front. Med. 5, 316. doi:10.3389/fmed.2018.00316
Bennett J. M., Sturmberg J. P. (2016). “Depression: Not Just a Top–Down Phenomenon,” in The Value of Systems and Complexity Sciences for Healthcare. Editor J. Sturmberg (Cham: Springer).
Berezin A. E., Berezin A. A., Lichtenauer M. (2020). Emerging Role of Adipocyte Dysfunction in Inducing Heart Failure Among Obese Patients with Prediabetes and Known Diabetes Mellitus. Front. Cardiovasc. Med. 7, 583175. doi:10.3389/fcvm.2020.583175
Bergens O., Nilsson A., Papaioannou K.-G., Kadi F. (2021). Sedentary Patterns and Systemic Inflammation: Sex-specific Links in Older Adults. Front. Physiol. 12, 625950. doi:10.3389/fphys.2021.625950
Bettcher B. M., Kramer J. H. (2013). Inflammation and Clinical Presentation in Neurodegenerative Disease: a Volatile Relationship. Neurocase 19 (2), 182–200. doi:10.1080/13554794.2011.654227
Bhowmick S., Singh A., Flavell R. A., Clark R. B., O'Rourke J., Cone R. E. (2009). The Sympathetic Nervous System Modulates CD4+ FoxP3+ Regulatory T Cells via a TGF-β-dependent Mechanism. J. Leukoc. Biol. 86 (6), 1275–1283. doi:10.1189/jlb.0209107
Bochaton-Piallat M.-L., Gabbiani G., Hinz B. (2016). The Myofibroblast in Wound Healing and Fibrosis: Answered and Unanswered Questions. F1000Res 5, 752. doi:10.12688/f1000research.8190.1
Bremner J., Moazzami K., Wittbrodt M., Nye J., Lima B., Gillespie C., et al. (2020). Diet, Stress and Mental Health. Nutrients 12 (8), 2428. doi:10.3390/nu12082428
Buttorff C., Ruder T., Bauman M. (2017). Multiple Chronic Conditions in the United States. Santa Monica. Available at: https://www.rand.org/content/dam/rand/pubs/tools/TL200/TL221/RAND_TL221.pdf.
Campos-Uscanga Y., Gutiérrez-Ospina G., Morales-Romero J., Romo-González T. (2017). Self-regulation of Eating and Physical Activity Is Lower in Obese Female College Students as Compared to Their Normal Weight Counterparts. Eat. Weight Disord. 22 (2), 311–319. doi:10.1007/s40519-016-0338-9
Cao N., Huang Y., Zheng J., Spencer C. I., Zhang Y., Fu J.-D., et al. (2016). Conversion of Human Fibroblasts into Functional Cardiomyocytes by Small Molecules. Science 352 (6290), 1216–1220. doi:10.1126/science.aaf1502\
Cenaj O., Allison D. H. R., Imam R., Zeck B., Drohan L. M., Chiriboga L., et al. (2021). Evidence for Continuity of Interstitial Spaces across Tissue and Organ Boundaries in Humans. Commun. Biol. 4 (1), 436. doi:10.1038/s42003-021-01962-0
Centers for Disease Control and Prevention (2021). Social Determinants of Health: Know what Affects Health. Available at: https://www.cdc.gov/publichealthgateway/sdoh/index.html.
Certo M., Tsai C.-H., Pucino V., Ho P.-C., Mauro C. (2021). Lactate Modulation of Immune Responses in Inflammatory versus Tumour Microenvironments. Nat. Rev. Immunol. 21 (3), 151–161. doi:10.1038/s41577-020-0406-2
Chazaud B. (2020). Inflammation and Skeletal Muscle Regeneration: Leave it to the Macrophages! Trends Immunol. 41 (6), 481–492. doi:10.1016/j.it.2020.04.006
Chen H., Shen L., Liu Y., Ma X., Long L., Ma X., et al. (2021). Strength Exercise Confers Protection in Central Nervous System Autoimmunity by Altering the Gut Microbiota. Front. Immunol. 12, 628629. doi:10.3389/fimmu.2021.628629
Chiaranunt P., Tai S. L., Ngai L., Mortha A. (2021). Beyond Immunity: Underappreciated Functions of Intestinal Macrophages. Front. Immunol. 12, 749708. doi:10.3389/fimmu.2021.749708
Chinetti-Gbaguidi G., Colin S., Staels B. (2015). Macrophage Subsets in Atherosclerosis. Nat. Rev. Cardiol. 12 (1), 10–17. doi:10.1038/nrcardio.2014.173
Cho J. H., Bhutani S., Kim C. H., Irwin M. R. (2021). Anti-inflammatory Effects of Melatonin: A Systematic Review and Meta-Analysis of Clinical Trials. Brain, Behav. Immun. 93, 245–253. doi:10.1016/j.bbi.2021.01.034
Clarke M. F. (2019). Clinical and Therapeutic Implications of Cancer Stem Cells. N. Engl. J. Med. 380 (23), 2237–2245. doi:10.1056/NEJMra1804280
Cole S. W., Nagaraja A. S., Lutgendorf S. K., Green P. A., Sood A. K. (2015). Sympathetic Nervous System Regulation of the Tumour Microenvironment. Nat. Rev. Cancer 15 (9), 563–572. doi:10.1038/nrc3978
Correa‐Gallegos D., Jiang D., Rinkevich Y. (2021). Fibroblasts as Confederates of the Immune System. Immunol. Rev. 302 (1), 147–162. doi:10.1111/imr.12972
Correa-Gallegos D., Jiang D., Christ S., Ramesh P., Ye H., Wannemacher J., et al. (2019). Patch Repair of Deep Wounds by Mobilized Fascia. Nature 576 (7786), 287–292. doi:10.1038/s41586-019-1794-y
Coussens L. M., Werb Z. (2002). Inflammation and Cancer. Nature 420 (6917), 860–867. doi:10.1038/nature01322
Cryan J. F., O'Riordan K. J., Cowan C. S. M., Sandhu K. V., Bastiaanssen T. F. S., Boehme M., et al. (2019). The Microbiota-Gut-Brain Axis. Physiol. Rev. 99 (4), 1877–2013. doi:10.1152/physrev.00018.2018
Díaz-Bulnes P., Saiz M. L., López-Larrea C., Rodríguez R. M. (2020). Crosstalk between Hypoxia and ER Stress Response: A Key Regulator of Macrophage Polarization. Front. Immunol. 10, 2951. doi:10.3389/fimmu.2019.02951
Duggal N. A., Niemiro G., Harridge S. D. R., Simpson R. J., Lord J. M. (2019). Can Physical Activity Ameliorate Immunosenescence and Thereby Reduce Age-Related Multi-Morbidity? Nat. Rev. Immunol. 19 (9), 563–572. doi:10.1038/s41577-019-0177-9
Dyavanapalli J. (2020). Novel Approaches to Restore Parasympathetic Activity to the Heart in Cardiorespiratory Diseases. Am. J. Physiology-Heart Circulatory Physiology 319 (6), H1153–H1161. doi:10.1152/ajpheart.00398.2020
Eckhouse S. R., Spinale F. G. (2012). Changes in the Myocardial Interstitium and Contribution to the Progression of Heart Failure. Heart Fail. Clin. 8 (1), 7–20. doi:10.1016/j.hfc.2011.08.012
Eisenberger N. I., Moieni M., Inagaki T. K., Muscatell K. A., Irwin M. R. (2017). In Sickness and in Health: The Co-regulation of Inflammation and Social Behavior. Neuropsychopharmacol 42, 242–253. doi:10.1038/npp.2016.141
Ekelund U., Tarp J., Steene-Johannessen J., Hansen B. H., Jefferis B., Fagerland M. W., et al. (2019). Dose-response Associations between Accelerometry Measured Physical Activity and Sedentary Time and All Cause Mortality: Systematic Review and Harmonised Meta-Analysis. Bmj 366, l4570. doi:10.1136/bmj.l4570
Elia I., Haigis M. C. (2021). Metabolites and the Tumour Microenvironment: from Cellular Mechanisms to Systemic Metabolism. Nat. Metab. 3 (1), 21–32. doi:10.1038/s42255-020-00317-z
Fioranelli M., Bottaccioli A. G., Bottaccioli F., Bianchi M., Rovesti M., Roccia M. G. (2018). Stress and Inflammation in Coronary Artery Disease: A Review Psychoneuroendocrineimmunology-Based. Front. Immunol. 9, 2031. doi:10.3389/fimmu.2018.02031
Frangogiannis N. G. (2019). Cardiac Fibrosis: Cell Biological Mechanisms, Molecular Pathways and Therapeutic Opportunities. Mol. aspects Med. 65, 70–99. doi:10.1016/j.mam.2018.07.001
Frank J., Abel T., Campostrini S., Cook S., Lin V. K., McQueen D. V. (2020). The Social Determinants of Health: Time to Re-think?. Ijerph 17 (16), 5856. doi:10.3390/ijerph17165856
Friedman S. L. (2022). Fighting Cardiac Fibrosis with CAR T Cells. N. Eng. J. Med. 386 (16), 1576–1578. doi:10.1056/NEJMcibr2201182
Fryar C. D., Carroll M. D., Afful J. (2020). Prevalence of Overweight, Obesity, and Severe Obesity Among Adults Aged 20 and over: United States, 1960–1962 through 2017–2018. NCHS Health E-Stats. Available at: https://www.cdc.gov/nchs/data/hestat/obesity-adult-17-18/overweight-obesity-adults-H.pdf.
Fülöp T., Larbi A., Witkowski J. M. (2019). Human Inflammaging. Gerontology 65 (5), 495–504. doi:10.1159/000497375
Geidl W., Schlesinger S., Mino E., Miranda L., Pfeifer K. (2020). Dose-response Relationship between Physical Activity and Mortality in Adults with Noncommunicable Diseases: a Systematic Review and Meta-Analysis of Prospective Observational Studies. Int. J. Behav. Nutr. Phys. Act. 17 (1), 109. doi:10.1186/s12966-020-01007-5
Geronikolou S. A., Pavlopoulou A., Cokkinos D., Chrousos G. (2017). Interactome of Obesity: Obesidome. Adv. Exp. Med. Biol. 987, 233–241. doi:10.1007/978-3-319-57379-3_21
Giunta B., Fernandez F., Nikolic W. V., Obregon D., Rrapo E., Town T., et al. (2008). Inflammaging as a Prodrome to Alzheimer's Disease. J. neuroinflammation 5, 51. doi:10.1186/1742-2094-5-51
Gomes C., Almeida J. A., Franco O. L., Petriz B. (2020). Omics and the Molecular Exercise Physiology. Adv. Clin. Chem. 96, 55–84. doi:10.1016/bs.acc.2019.11.003
Gonzalo-Encabo P., Maldonado G., Valadés D., Ferragut C., Pérez-López A. (2021). The Role of Exercise Training on Low-Grade Systemic Inflammation in Adults with Overweight and Obesity: A Systematic Review. Ijerph 18 (24), 13258. doi:10.3390/ijerph182413258
Goverse G., Stakenborg M., Matteoli G. (2016). The Intestinal Cholinergic Anti-inflammatory Pathway. J. Physiol. 594 (20), 5771–5780. doi:10.1113/JP271537
Grassi G., D’Arrigo G., Pisano A., Bolignano D., Mallamaci F., Dell’Oro R., et al. (2019). Sympathetic Neural Overdrive in Congestive Heart Failure and its Correlates. J. Hypertens. 37 (9), 1746–1756. doi:10.1097/HJH.0000000000002093
Gustafsson T., Ulfhake B. (2021). Sarcopenia: What Is the Origin of This Aging-Induced Disorder?. Front. Genet. 12, 688526. doi:10.3389/fgene.2021.688526
Gutkowska J., Jankowski M. (2012). Oxytocin Revisited: its Role in Cardiovascular Regulation. J. Neuroendocrinol. 24 (4), 599–608. doi:10.1111/j.1365-2826.2011.02235.x
Haider N., Boscá L., Zandbergen H. R., Kovacic J. C., Narula N., González-Ramos S., et al. (2019). Transition of Macrophages to Fibroblast-like Cells in Healing Myocardial Infarction. J. Am. Coll. Cardiol. 74 (25), 3124–3135. doi:10.1016/j.jacc.2019.10.036
Haykin H., Rolls A. (2021). The Neuroimmune Response during Stress: A Physiological Perspective. Immunity 54 (9), 1933–1947. doi:10.1016/j.immuni.2021.08.023
Henson J., Yates T., Edwardson C. L., Khunti K., Talbot D., Gray L. J., et al. (2013). Sedentary Time and Markers of Chronic Low-Grade Inflammation in a High Risk Population. PloS one 8 (10), e78350. doi:10.1371/journal.pone.0078350
Hinshaw D. C., Shevde L. A. (2019). The Tumor Microenvironment Innately Modulates Cancer Progression. Cancer Res. 79 (18), 4557–4566. doi:10.1158/0008-5472.CAN-18-3962
Hoffmann C. M., Petrov M. E., Lee R. E. (2021). Aerobic Physical Activity to Improve Memory and Executive Function in Sedentary Adults without Cognitive Impairment: A Systematic Review and Meta-Analysis. Prev. Med. Rep. 23, 101496. doi:10.1016/j.pmedr.2021.101496
Hood L., Auffray C. (2013). Participatory Medicine: a Driving Force for Revolutionizing Healthcare. Genome Med. 5 (12), 110. doi:10.1186/gm514
Huang Y.-Y., Lin C.-W., Cheng N.-C., Cazzell S. M., Chen H.-H., Huang K.-F., et al. (2021). Effect of a Novel Macrophage-Regulating Drug on Wound Healing in Patients with Diabetic Foot Ulcers. JAMA Netw. Open 4 (9), e2122607. doi:10.1001/jamanetworkopen.2021.22607
Ieda M. (2020). Key Regulators of Cardiovascular Differentiation and Regeneration: Harnessing the Potential of Direct Reprogramming to Treat Heart Failure. J. cardiac Fail. 26 (1), 80–84. doi:10.1016/j.cardfail.2019.09.005
Imai J., Katagiri H. (2022). Regulation of Systemic Metabolism by the Autonomic Nervous System Consisting of Afferent and Efferent Innervation. Int. Immunol. 34 (2), 67–79. doi:10.1093/intimm/dxab023
Inoue Y., Kishida T., Kotani S.-i., Akiyoshi M., Taga H., Seki M., et al. (2019). Direct Conversion of Fibroblasts into Urothelial Cells that May Be Recruited to Regenerating Mucosa of Injured Urinary Bladder. Sci. Rep. 9 (1), 13850. doi:10.1038/s41598-019-50388-6
Ivanov P. C., Bartsch R. P., Bartsch R. P. (2016). Focus on the Emerging New Fields of Network Physiology and Network Medicine. New J. Phys. 18, 100201. doi:10.1088/1367-2630/18/10/100201
Jankowski M., Broderick T. L., Gutkowska J. (2020). The Role of Oxytocin in Cardiovascular Protection. Front. Psychol. 11, 2139. doi:10.3389/fpsyg.2020.02139
Jha A. K., Huang S. C., Sergushichev A., Lampropoulou V., Ivanova Y., Loginicheva E., et al. (2015). Network Integration of Parallel Metabolic and Transcriptional Data Reveals Metabolic Modules that Regulate Macrophage Polarization. Immunity 42 (3), 419–430. doi:10.1016/j.immuni.2015.02.005
Jurek B., Neumann I. D. (2018). The Oxytocin Receptor: From Intracellular Signaling to Behavior. Physiol. Rev. 98 (3), 1805–1908. doi:10.1152/physrev.00031.2017
Kenney M. J., Ganta C. K. (2014). Autonomic Nervous System and Immune System Interactions. Compr. Physiol. 4 (3), 1177–1200. doi:10.1002/cphy.c130051
Khera A. V., Emdin C. A., Drake I., Natarajan P., Bick A. G., Cook N. R., et al. (2016). Genetic Risk, Adherence to a Healthy Lifestyle, and Coronary Disease. N. Engl. J. Med. 375 (24), 2349–2358. doi:10.1056/NEJMoa1605086
Kisseleva T., Brenner D. (2021). Molecular and Cellular Mechanisms of Liver Fibrosis and its Regression. Nat. Rev. Gastroenterol. Hepatol. 18 (3), 151–166. doi:10.1038/s41575-020-00372-7
Knudsen L., Ruppert C., Ochs M. (2017). Tissue Remodelling in Pulmonary Fibrosis. Cell Tissue Res. 367 (3), 607–626. doi:10.1007/s00441-016-2543-2
Knudsen N. H., Stanya K. J., Hyde A. L., Chalom M. M., Alexander R. K., Liou Y.-H., et al. (2020). Interleukin-13 Drives Metabolic Conditioning of Muscle to Endurance Exercise. Science 368 (6490), eaat3987. doi:10.1126/science.aat3987
Kochanek K. D., Xu J., Arias E. (2019). Key Findings Data from the National Vital Statistics System How Long Can We Expect to Live? Available at: https://www.cdc.gov/nchs/products/index.htm.
Kohlgruber A., Lynch L. (2015). Adipose Tissue Inflammation in the Pathogenesis of Type 2 Diabetes. Curr. Diab Rep. 15 (11), 92. doi:10.1007/s11892-015-0670-x
Koliaraki V., Prados A., Armaka M., Kollias G. (2020). The Mesenchymal Context in Inflammation, Immunity and Cancer. Nat. Immunol. 21 (9), 974–982. doi:10.1038/s41590-020-0741-2
Koob G. F., Volkow N. D. (2016). Neurobiology of Addiction: a Neurocircuitry Analysis. Lancet Psychiatry 3 (8), 760–773. doi:10.1016/S2215-0366(16)00104-8
Krüger K., Mooren F.-C., Pilat C. (2016). The Immunomodulatory Effects of Physical Activity. Cpd 22 (24), 3730–3748. doi:10.2174/1381612822666160322145107
Kuna J., Żuber Z., Chmielewski G., Gromadziński L., Krajewska-Włodarczyk M. (2022). Role of Distinct Macrophage Populations in the Development of Heart Failure in Macrophage Activation Syndrome. Ijms 23 (5), 2433. doi:10.3390/ijms23052433
Lamb J. J., Stone M., D’Adamo C. R., Volkov A., Metti D., Aronica L., et al. (2022). Personalized Lifestyle Intervention and Functional Evaluation Health Outcomes SurvEy: Presentation of the LIFEHOUSE Study Using N-Of-One Tent-Umbrella-Bucket Design. Jpm 12 (1), 115. doi:10.3390/jpm12010115
Langevin H. M. (2022). Making Connections to Improve Health Outcomes. Glob. Adv. Health Med. 11, 2164957X2210797. doi:10.1177/2164957X221079792
Leal L. G., Lopes M. A., Batista M. L. (2018). Physical Exercise-Induced Myokines and Muscle-Adipose Tissue Crosstalk: A Review of Current Knowledge and the Implications for Health and Metabolic Diseases. Front. Physiol. 9, 1307. doi:10.3389/fphys.2018.01307
Lee L. Y., Pandey A. K., Maron B. A., Loscalzo J. (2021). Network Medicine in Cardiovascular Research. Cardiovasc. Res. 117 (10), 2186–2202. doi:10.1093/cvr/cvaa321
Lehnertz K., Bröhl T., Rings T. (2020). The Human Organism as an Integrated Interaction Network: Recent Conceptual and Methodological Challenges. Front. Physiol. 11, 598694. doi:10.3389/fphys.2020.598694
Lin J., Jiang Z., Liu C., Zhou D., Song J., Liao Y., et al. (2020). Emerging Roles of Long Non-coding RNAs in Renal Fibrosis. Life 10 (8), 131. doi:10.3390/life10080131
Liu Y., Wang Y., Ni Y., Cheung C. K. Y., Lam K. S. L., Wang Y., et al. (2020). Gut Microbiome Fermentation Determines the Efficacy of Exercise for Diabetes Prevention. Cell metab. 31 (1), 77–91. e5. doi:10.1016/j.cmet.2019.11.001
Locati M., Curtale G., Mantovani A. (2020). Diversity, Mechanisms, and Significance of Macrophage Plasticity. Annu. Rev. Pathol. Mech. Dis. 15, 123–147. doi:10.1146/annurev-pathmechdis-012418-012718
Lohse T., Rohrmann S., Bopp M., Faeh D. (2016). Heavy Smoking Is More Strongly Associated with General Unhealthy Lifestyle Than Obesity and Underweight. PloS one 11 (2), e0148563. doi:10.1371/journal.pone.0148563
Lujan H. L., DiCarlo S. E. (2013). Physical Activity, by Enhancing Parasympathetic Tone and Activating the Cholinergic Anti-inflammatory Pathway, Is a Therapeutic Strategy to Restrain Chronic Inflammation and Prevent Many Chronic Diseases. Med. hypotheses 80 (5), 548–552. doi:10.1016/j.mehy.2013.01.014
Luo F., Sandhu A. F., Rungratanawanich W., Williams G. E., Akbar M., Zhou S., et al. (2020). Melatonin and Autophagy in Aging-Related Neurodegenerative Diseases. Int. J. Mol. Sci. 21 (19), 7174. doi:10.3390/ijms21197174
Mahdy M. A. A. (2019). Skeletal Muscle Fibrosis: an Overview. Cell Tissue Res. 375 (3), 575–588. doi:10.1007/s00441-018-2955-2
Mainous A. G., Everett C. J., Diaz V. A., Player M. S., Gebregziabher M., Smith D. W. (2010). Life Stress and Atherosclerosis: a Pathway through Unhealthy Lifestyle. Int. J. Psychiatry Med. 40 (2), 147–161. doi:10.2190/PM.40.2.b
Malesza I. J., Malesza M., Walkowiak J., Mussin N., Walkowiak D., Aringazina R., et al. (2021). High-Fat, Western-Style Diet, Systemic Inflammation, and Gut Microbiota: A Narrative Review. Cells 10 (11), 3164. doi:10.3390/cells10113164
Manor O., Dai C. L., Kornilov S. A., Smith B., Price N. D., Lovejoy J. C., et al. (2020). Health and Disease Markers Correlate with Gut Microbiome Composition across Thousands of People. Nat. Commun. 11 (1), 5206. doi:10.1038/s41467-020-18871-1
Martin A. B., Hartman M., Lassman D., Catlin A.National Health Expenditure Accounts Team (2021). National Health Care Spending in 2019: Steady Growth for the Fourth Consecutive Year. Health Aff. 40 (1), 14–24. doi:10.1377/hlthaff.2020.02022
Masoodi M., Kuda O., Rossmeisl M., Flachs P., Kopecky J. (2015). Lipid Signaling in Adipose Tissue: Connecting Inflammation & Metabolism. Biochimica Biophysica Acta (BBA) - Mol. Cell Biol. Lipids 1851 (4), 503–518. doi:10.1016/j.bbalip.2014.09.023
McGee S. L., Hargreaves M. (2019). Epigenetics and Exercise. Trends Endocrinol. Metabolism 30 (9), 636–645. doi:10.1016/j.tem.2019.06.002
Megur A., Baltriukienė D., Bukelskienė V., Burokas A. (2020). The Microbiota-Gut-Brain Axis and Alzheimer's Disease: Neuroinflammation Is to Blame? Nutrients 13 (1), 37. doi:10.3390/nu13010037
Meng X.-M., Nikolic-Paterson D. J., Lan H. Y. (2016). TGF-β: the Master Regulator of Fibrosis. Nat. Rev. Nephrol. 12 (6), 325–338. doi:10.1038/nrneph.2016.48
Meng X.-M. (2019). Inflammatory Mediators and Renal Fibrosis. Adv. Exp. Med. Biol. 1165, 381–406. doi:10.1007/978-981-13-8871-2_18
Millar S. R., Navarro P., Harrington J. M., Perry I. J., Phillips C. M. (2021). Dietary Quality Determined by the Healthy Eating Index-2015 and Biomarkers of Chronic Low-Grade Inflammation: A Cross-Sectional Analysis in Middle-To-Older Aged Adults. Nutrients 13 (1), 222. doi:10.3390/nu13010222
Mok A., Khaw K.-T., Luben R., Wareham N., Brage S. (2019). Physical Activity Trajectories and Mortality: Population Based Cohort Study. Bmj 365, l2323. doi:10.1136/bmj.l2323
Monje M. (2021). Spinal Cord Injury - Healing from within. N. Engl. J. Med. 384 (2), 182–184. doi:10.1056/NEJMcibr2030836
Montero J. A., Lorda-Diez C. I., Hurlé J. M. (2012). Regenerative Medicine and Connective Tissues: Cartilage versus Tendon. J. Tissue Eng. Regen. Med. 6 (5), 337–347. doi:10.1002/term.436
Mukhtar S. (2020). Psychological Health during the Coronavirus Disease 2019 Pandemic Outbreak. Int. J. Soc. Psychiatry 66 (5), 512–516. doi:10.1177/0020764020925835
Murray K. N., Buggey H. F., Denes A., Allan S. M. (2013). Systemic Immune Activation Shapes Stroke Outcome. Mol. Cell. Neurosci. 53, 14–25. doi:10.1016/j.mcn.2012.09.004
Murray P. J. (2017). Macrophage Polarization. Annu. Rev. Physiol. 79, 541–566. doi:10.1146/annurev-physiol-022516-034339
Narayanaswami V., Dwoskin L. P. (2017). Obesity: Current and Potential Pharmacotherapeutics and Targets. Pharmacol. Ther. 170, 116–147. doi:10.1016/j.pharmthera.2016.10.015
National Institutes of Health Media Release (2018). NIH Scientists Watch Brain’s Lining Heal after a Head Injury. Available at: https://www.nih.gov/news-events/news-releases/nih-scientists-watch-brains-lining-heal-after-head-injury (Acessed on April 16, 2018).
Nicolaides N. C., Vgontzas A. N., Kritikou I., Chrousos G. (2020). “HPA Axis and Sleep,” in Endotext. MDText.Com, Inc. Editor K. R. Feingold. Editors.
Noble R., Tasaki K., Noble P. J., Noble D. (2019). Biological Relativity Requires Circular Causality but Not Symmetry of Causation: So, where, what and when Are the Boundaries? Front. Physiol. 10, 827. doi:10.3389/fphys.2019.00827
Noushad S., Ahmed S., Ansari B., Mustafa U. H., Saleem Y., Hazrat H. (2021). Physiological Biomarkers of Chronic Stress: A Systematic Review. Int. J. Health Sci. (Qassim) 15 (5), 46–59.
O'Toole P. W., Shiels P. G. (2020). The Role of the Microbiota in Sedentary Lifestyle Disorders and Ageing: Lessons from the Animal Kingdom. J. Intern Med. 287 (3), 271–282. doi:10.1111/joim.13021
Oldham W. M., Oliveira R. K. F., Wang R.-S., Opotowsky A. R., Rubins D. M., Hainer J., et al. (2018). Network Analysis to Risk Stratify Patients with Exercise Intolerance. Circ. Res. 122 (6), 864–876. doi:10.1161/CIRCRESAHA.117.312482
Olivieri F., Prattichizzo F., Giuliani A., Matacchione G., Rippo M. R., Sabbatinelli J., et al. (2021). miR-21 and miR-146a: The microRNAs of Inflammaging and Age-Related Diseases. Ageing Res. Rev. 70, 101374. doi:10.1016/j.arr.2021.101374
Ouchi N., Parker J. L., Lugus J. J., Walsh K. (2011). Adipokines in Inflammation and Metabolic Disease. Nat. Rev. Immunol. 11 (2), 85–97. doi:10.1038/nri2921
Packer M. (2018). The Alchemist's Nightmare. Circulation 137 (19), 2068–2073. doi:10.1161/CIRCULATIONAHA.117.032190
Pascual-Fernández J., Fernández-Montero A., Córdova-Martínez A., Pastor D., Martínez-Rodríguez A., Roche E. (2020). Sarcopenia: Molecular Pathways and Potential Targets for Intervention. Ijms 21 (22), 8844. doi:10.3390/ijms21228844
Pedersen B. K. (2019). Physical Activity and Muscle-Brain Crosstalk. Nat. Rev. Endocrinol. 15 (7), 383–392. doi:10.1038/s41574-019-0174-x
Pérez-Pérez A., Vilariño-García T., Fernández-Riejos P., Martín-González J., Segura-Egea J. J., Sánchez-Margalet V. (2017). Role of Leptin as a Link between Metabolism and the Immune System. Cytokine & growth factor Rev. 35, 71–84. doi:10.1016/j.cytogfr.2017.03.001
Peruzzotti-Jametti L., Willis C. M., Hamel R., Krzak G., Pluchino S. (2021). Metabolic Control of Smoldering Neuroinflammation. Front. Immunol. 12, 705920. doi:10.3389/fimmu.2021.705920
Physical Activity Guidelines Advisory Committee (2018). Physical Activity Guidelines Advisory Committee Scientific Report. Washington, DC: U.S. Department of Health and Human Services. Available at: https://health.gov/sites/default/files/2019-09/PAG_Advisory_Committee_Report.pdf.
Pogontke C., Guadix J. A., Ruiz‐Villalba A., Pérez‐Pomares J. M. (2019). Development of the Myocardial Interstitium. Anat. Rec. 302 (1), 58–68. doi:10.1002/ar.23915
Posadzki P., Pieper D., Bajpai R., Makaruk H., Könsgen N., Neuhaus A. L., et al. (2020). Exercise/physical Activity and Health Outcomes: an Overview of Cochrane Systematic Reviews. BMC Public Health 20 (1), 1724. doi:10.1186/s12889-020-09855-3
Price N. D., Magis A. T., Earls J. C., Glusman G., Levy R., Lausted C., et al. (2017). A Wellness Study of 108 Individuals Using Personal, Dense, Dynamic Data Clouds. Nat. Biotechnol. 35 (8), 747–756. doi:10.1038/nbt.3870
Psarras S., Beis D., Nikouli S., Tsikitis M., Capetanaki Y. (2019). Three in a Box: Understanding Cardiomyocyte, Fibroblast, and Innate Immune Cell Interactions to Orchestrate Cardiac Repair Processes. Front. Cardiovasc. Med. 6, 32. doi:10.3389/fcvm.2019.00032
Rinschen M. M., Ivanisevic J., Giera M., Siuzdak G. (2019). Identification of Bioactive Metabolites Using Activity Metabolomics. Nat. Rev. Mol. Cell Biol. 20 (6), 353–367. doi:10.1038/s41580-019-0108-4
Rippe J. M. (2021). The Silent Epidemic. Am. J. Med. 134 (2), 164–165. doi:10.1016/j.amjmed.2020.09.028
Rocque R. J., Beaudoin C., Ndjaboue R., Cameron L., Poirier-Bergeron L., Poulin-Rheault R.-A., et al. (2021). Health Effects of Climate Change: an Overview of Systematic Reviews. BMJ open 11 (6), e046333. doi:10.1136/bmjopen-2020-046333
Rodriguez-Ayllon M., Cadenas-Sánchez C., Estévez-López F., Muñoz N. E., Mora-Gonzalez J., Migueles J. H., et al. (2019). Role of Physical Activity and Sedentary Behavior in the Mental Health of Preschoolers, Children and Adolescents: A Systematic Review and Meta-Analysis. Sports Med. 49 (9), 1383–1410. doi:10.1007/s40279-019-01099-5
Rohleder N. (2019). Stress and Inflammation - the Need to Address the Gap in the Transition between Acute and Chronic Stress Effects. Psychoneuroendocrinology 105, 164–171. doi:10.1016/j.psyneuen.2019.02.021
Rurik J. G., Tombácz I., Yadegari A., Méndez Fernández P. O., Shewale S. V., Li L., et al. (2022). CAR T Cells Produced In Vivo to Treat Cardiac Injury. Science 375 (6576), 91–96. doi:10.1126/science.abm0594
Russo M. V., Latour L. L., McGavern D. B. (2018). Distinct Myeloid Cell Subsets Promote Meningeal Remodeling and Vascular Repair after Mild Traumatic Brain Injury. Nat. Immunol. 19 (5), 442–452. doi:10.1038/s41590-018-0086-2
Saha S., Shalova I. N., Biswas S. K. (2017). Metabolic Regulation of Macrophage Phenotype and Function. Immunol. Rev. 280 (1), 102–111. doi:10.1111/imr.12603
Said M. A., Verweij N., van der Harst P. (2018). Associations of Combined Genetic and Lifestyle Risks with Incident Cardiovascular Disease and Diabetes in the UK Biobank Study. JAMA Cardiol. 3 (8), 693–702. doi:10.1001/jamacardio.2018.1717
Scallan J., Huxley V. H., Korthuis R. J. (2010). Capillary Fluid Exchange: Regulation, Functions, and Pathology. Chapter 2, The Interstitium. San Rafael (CA): Morgan & Claypool Life Sciences. Available at: https://www.ncbi.nlm.nih.gov/books/NBK53446/.
Schelbert E. B., Butler J., Diez J. (2019). Why Clinicians Should Care about the Cardiac Interstitium. JACC Cardiovasc. Imaging 12 (11 Pt 2), 2305–2318. doi:10.1016/j.jcmg.2019.04.025
Seaborne R. A., Sharples A. P. (2020). The Interplay between Exercise Metabolism, Epigenetics, and Skeletal Muscle Remodeling. Exerc. sport Sci. Rev. 48 (4), 188–200. doi:10.1249/JES.0000000000000227
Shahin W., Stupans I., Kennedy G. (2021). Health Beliefs and Chronic Illnesses of Refugees: a Systematic Review. Ethn. health 26 (5), 756–768. doi:10.1080/13557858.2018.1557118
Shapouri‐Moghaddam A., Mohammadian S., Vazini H., Taghadosi M., Esmaeili S. A., Mardani F., et al. (2018). Macrophage Plasticity, Polarization, and Function in Health and Disease. J. Cell Physiol. 233 (9), 6425–6440. doi:10.1002/jcp.26429
Shouman K., Benarroch E. E. (2021). Peripheral Neuroimmune Interactions: Selected Review and Some Clinical Implications. Clin. Auton. Res. 31 (4), 477–489. doi:10.1007/s10286-021-00787-5
Shur N. F., Creedon L., Skirrow S., Atherton P. J., MacDonald I. A., Lund J., et al. (2021). Age-related Changes in Muscle Architecture and Metabolism in Humans: The Likely Contribution of Physical Inactivity to Age-Related Functional Decline. Ageing Res. Rev. 68, 101344. doi:10.1016/j.arr.2021.101344
Sohail M. U., Yassine H. M., Sohail A., Thani A. A. A. (2019). Impact of Physical Exercise on Gut Microbiome, Inflammation, and the Pathobiology of Metabolic Disorders. Rev. Diabet. Stud. 15, 35–48. doi:10.1900/RDS.2019.15.35
Solis A. G., Bielecki P., Steach H. R., Sharma L., Harman C. C. D., Yun S., et al. (2019). Mechanosensation of Cyclical Force by PIEZO1 Is Essential for Innate Immunity. Nature 573 (7772), 69–74. doi:10.1038/s41586-019-1485-8
Sotos‐Prieto M., Baylin A., Campos H., Qi L., Mattei J. (2016). Lifestyle Cardiovascular Risk Score, Genetic Risk Score, and Myocardial Infarction in Hispanic/Latino Adults Living in Costa Rica. Jaha 5 (12), e004067. doi:10.1161/JAHA.116.004067
Soundararajan M., Kannan S. (2018). Fibroblasts and Mesenchymal Stem Cells: Two Sides of the Same Coin? J. Cell. Physiology 233 (12), 9099–9109. doi:10.1002/jcp.26860
Sridharan R., Cavanagh B., Cameron A. R., Kelly D. J., O'Brien F. J. (2019). Material Stiffness Influences the Polarization State, Function and Migration Mode of Macrophages. Acta biomater. 89, 47–59. doi:10.1016/j.actbio.2019.02.048
Steens J., Unger K., Klar L., Neureiter A., Wieber K., Hess J., et al. (2020). Direct Conversion of Human Fibroblasts into Therapeutically Active Vascular Wall-Typical Mesenchymal Stem Cells. Cell. Mol. Life Sci. 77 (17), 3401–3422. doi:10.1007/s00018-019-03358-0
Sturmberg J. P. (2021). Health and Disease Are Dynamic Complex-Adaptive States Implications for Practice and Research. Front. Psychiatry 12, 595124. doi:10.3389/fpsyt.2021.595124
Sturmberg J. P., Picard M., Aron D. C., Bennett J. M., Bircher J., deHaven M. J., et al. (2019). Health and Disease-Emergent States Resulting from Adaptive Social and Biological Network Interactions. Front. Med. 6, 59. doi:10.3389/fmed.2019.00059
Sulikowska B., Rutkowski B., Marszałek A., Manitius J. (2015). The Role of Interstitial Changes in the Progression of Chronic Kidney Disease. Postepy Hig. Med. Dosw 69, 830–837. doi:10.5604/17322693.1162570
Suzuki K. (2019). Chronic Inflammation as an Immunological Abnormality and Effectiveness of Exercise. Biomolecules 9 (6), 223. doi:10.3390/biom9060223
Tan Y. Y., Montagnese S., Mani A. R. (2020). Organ System Network Disruption Is Associated with Poor Prognosis in Patients with Chronic Liver Failure. Front. Physiol. 11, 983. doi:10.3389/fphys.2020.00983
Tilg H., Zmora N., Adolph T. E., Elinav E. (2020). The Intestinal Microbiota Fuelling Metabolic Inflammation. Nat. Rev. Immunol. 20 (1), 40–54. doi:10.1038/s41577-019-0198-4
Topol E. (2019). How Artificial Intelligence Can Make Healthcare Human Again. New York, NY: Basic Books, Inc. Division of HarperCollins. 978-1-5416-4463-2.
Uderhardt S., Martins A. J., Tsang J. S., Lämmermann T., Germain R. N. (2019). Resident Macrophages Cloak Tissue Microlesions to Prevent Neutrophil-Driven Inflammatory Damage. Cell 177 (3), 541–555. doi:10.1016/j.cell.2019.02.028
Vagnozzi R. J., Maillet M., Sargent M. A., Khalil H., Johansen A. K. Z., Schwanekamp J. A., et al. (2020). An Acute Immune Response Underlies the Benefit of Cardiac Stem Cell Therapy. Nature 577 (7790), 405–409. doi:10.1038/s41586-019-1802-2
Van Linthout S., Miteva K., Tschope C. (2014). Crosstalk between Fibroblasts and Inflammatory Cells. Cardiovasc. Res. 102 (2), 258–269. doi:10.1093/cvr/cvu062
Vergallo R., Crea F. (2020). Atherosclerotic Plaque Healing. N. Engl. J. Med. 383 (9), 846–857. doi:10.1056/NEJMra2000317
Vila J. (2021). Social Support and Longevity: Meta-Analysis-Based Evidence and Psychobiological Mechanisms. Front. Psychol. 12, 717164. doi:10.3389/fpsyg.2021.717164
Weavers H., Martin P. (2020). The Cell Biology of Inflammation: From Common Traits to Remarkable Immunological Adaptations. J. cell Biol. 219 (7), e202004003. doi:10.1083/jcb.202004003
Weinstock J., Farney M. R., Elrod N. M., Henderson C. E., Weiss E. P. (2017). Exercise as an Adjunctive Treatment for Substance Use Disorders: Rationale and Intervention Description. J. Subst. abuse Treat. 72, 40–47. doi:10.1016/j.jsat.2016.09.002
Westfall S., Lomis N., Kahouli I., Dia S. Y., Singh S. P., Prakash S. (2017). Microbiome, Probiotics and Neurodegenerative Diseases: Deciphering the Gut Brain axis. Cell Mol. Life Sci. 74 (20), 3769–3787. doi:10.1007/s00018-017-2550-9
Wiig H., Swartz M. A. (2012). Interstitial Fluid and Lymph Formation and Transport: Physiological Regulation and Roles in Inflammation and Cancer. Physiol. Rev. 92 (3), 1005–1060. doi:10.1152/physrev.00037.2011
Wolf D., Ley K. (2019). Immunity and Inflammation in Atherosclerosis. Circ. Res. 124 (2), 315–327. doi:10.1161/CIRCRESAHA.118.313591
World Health Organization (2022). Global Health Observatory; Metadata Registry List: Physical Inactivity. Available at: https://www.who.int/data/gho/indicator-metadata-registry/imr-details/3416.
World Health Organization (1999). Healthy Living: What Is a Healthy Lifestyle? WHO Regional Office for Europe. Available at: http://apps.who.int/iris/bitstream/handle/10665/108180/EUR_ICP_LVNG_01_07_02.pdf.
World Health Organization (2020). The Top Ten Causes of Death. Available at: https://www.who.int/news-room/fact-sheets/detail/the-top-10-causes-of-death.
World Health Organization (2022). WHO Coronavirus (COVID-19) (2022). Dashboard as 5:22pm CEST, 7 June 2022. Available at: https://covid19.who.int/.
Wynn T. A., Chawla A., Pollard J. W. (2013). Macrophage Biology in Development, Homeostasis and Disease. Nature 496 (7446), 445–455. doi:10.1038/nature12034
Wynn T. (2008). Cellular and Molecular Mechanisms of Fibrosis. J. Pathol. 214 (2), 199–210. doi:10.1002/path.2277
Xu X., Zheng L., Yuan Q., Zhen G., Crane J. L., Zhou X., et al. (2018). Transforming Growth Factor-β in Stem Cells and Tissue Homeostasis. Bone Res. 6, 2. doi:10.1038/s41413-017-0005-4
Yamasaki K., Eeden S. F. v. (2018). Lung Macrophage Phenotypes and Functional Responses: Role in the Pathogenesis of COPD. Ijms 19 (2), 582. doi:10.3390/ijms19020582
Yang T., Richards E. M., Pepine C. J., Raizada M. K. (2018). The Gut Microbiota and the Brain-Gut-Kidney axis in Hypertension and Chronic Kidney Disease. Nat. Rev. Nephrol. 14 (7), 442–456. doi:10.1038/s41581-018-0018-2
Yoo B. B., Mazmanian S. K. (2017). The Enteric Network: Interactions between the Immune and Nervous Systems of the Gut. Immunity 46 (6), 910–926. doi:10.1016/j.immuni.2017.05.011
Zaghlool S. B., Kühnel B., Elhadad M. A., Kader S., Halama A., Thareja G., et al. (2020). Epigenetics Meets Proteomics in an Epigenome-wide Association Study with Circulating Blood Plasma Protein Traits. Nat. Commun. 11 (1), 15. doi:10.1038/s41467-019-13831-w
Zarzour A., Kim H. W., Weintraub N. L. (2019). Epigenetic Regulation of Vascular Diseases. Atvb 39 (6), 984–990. doi:10.1161/ATVBAHA.119.312193
Zhang L., Lu Q., Chang C. (2020). Epigenetics in Health and Disease. Adv. Exp. Med. Biol. 1253, 3–55. doi:10.1007/978-981-15-3449-2_1
Zhang S., de la Haye K., Ji M., An R. (2018). Applications of Social Network Analysis to Obesity: a Systematic Review. Obes. Rev. 19 (7), 976–988. doi:10.1111/obr.12684
Zhang Y., Li S., Cao Z., Cheng Y., Xu C., Yang H., et al. (2021). A Network Analysis Framework of Genetic and Nongenetic Risks for Type 2 Diabetes. Rev. Endocr. Metab. Disord. 22 (2), 461–469. doi:10.1007/s11154-020-09585-2
Zhu X., Chen Z., Shen W., Huang G., Sedivy J. M., Wang H., et al. (2021). Inflammation, Epigenetics, and Metabolism Converge to Cell Senescence and Ageing: the Regulation and Intervention. Sig Transduct. Target Ther. 6 (1), 245. doi:10.1038/s41392-021-00646-9
Zou L., Sasaki J., Wei G.-X., Huang T., Yeung A., Neto O., et al. (2018). Effects of Mind-Body Exercises (Tai Chi/Yoga) on Heart Rate Variability Parameters and Perceived Stress: A Systematic Review with Meta-Analysis of Randomized Controlled Trials. Jcm 7 (11), 404. doi:10.3390/jcm71104010.3390/jcm7110404
Keywords: chronic disease, sedentariness, autonomic nervous system, physical activity, microenvironment, macrophages (M1/M2), chronic low-grade inflammation, fibroblasts
Citation: Huston P (2022) A Sedentary and Unhealthy Lifestyle Fuels Chronic Disease Progression by Changing Interstitial Cell Behaviour: A Network Analysis. Front. Physiol. 13:904107. doi: 10.3389/fphys.2022.904107
Received: 25 March 2022; Accepted: 27 May 2022;
Published: 08 July 2022.
Edited by:
Simon Higgins, Elon University, United StatesReviewed by:
Natàlia Balagué, University of Barcelona, SpainJoachim P. Sturmberg, The University of Newcastle, Australia
Copyright © 2022 Huston. This is an open-access article distributed under the terms of the Creative Commons Attribution License (CC BY). The use, distribution or reproduction in other forums is permitted, provided the original author(s) and the copyright owner(s) are credited and that the original publication in this journal is cited, in accordance with accepted academic practice. No use, distribution or reproduction is permitted which does not comply with these terms.
*Correspondence: Patricia Huston, cGh1c3RvbkB1b3R0YXdhLmNh, b3JjaWQub3JnLzAwMDAtMDAwMi0yOTI3LTExNzY=