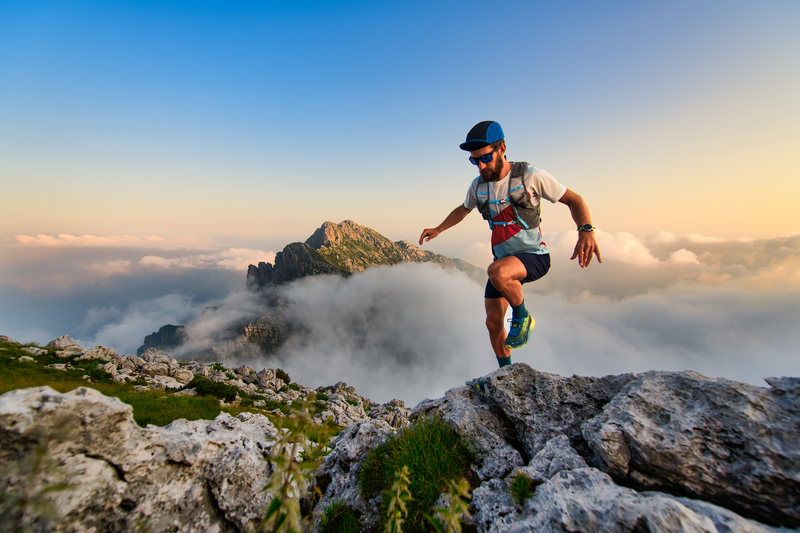
94% of researchers rate our articles as excellent or good
Learn more about the work of our research integrity team to safeguard the quality of each article we publish.
Find out more
EDITORIAL article
Front. Physiol. , 08 December 2022
Sec. Computational Physiology and Medicine
Volume 13 - 2022 | https://doi.org/10.3389/fphys.2022.1100037
This article is part of the Research Topic Computational Biomechanics for Ventricle-arterial Dysfunction and Remodeling in Heart Failure, Volume II View all 13 articles
Editorial on the Research Topic
Computational biomechanics for ventricle-arterial dysfunction and remodeling in heart failure, Volume II
Interactions between the left ventricle (LV) and systemic circulation, and between the right ventricle (RV) and the pulmonary circulation are key determinants of cardiac and cardiovascular function. The global performance of LV-arterial coupling (e.g., the ratio of effective arterial elastance, time-varying pressure-flow relations, and effects of wave reflections) has been applied to many clinical scenarios such as aging, hypertension, heart failure (HF), and dilated cardiomyopathy. The global approach, however, has significant limitations in heart failure with preserved ejection fraction (HFpEF), although it can provide useful information regarding the mechanical efficiency and performance in heart failure with reduced ejection fraction (HFrEF). This approach is also less informative in the study of RV dysfunction and its coupling with the pulmonary circulation in HF. With the development of simulation-based biomechanics in recent years, it is required to demonstrate accurate analysis of local ventricle-arterial functions and remodeling in health and disease, particularly in the progression of HF.
This Research Topic in computational biomechanics was conceived to improve our understanding and treatment of cardiac and cardiovascular dysfunction and their coupling abnormalities associated with the occurrence and development of HFpEF or HFrEF. There is a total of 12 published articles (11 original research and 1 brief research report) relevant to basic and clinical studies covering: 1) cardiac stress and strain analysis using computational models; 2) hemodynamics in systemic/pulmonary circulations or coronary circulation; 3) advanced biomechanics models of LV/RV-arterial coupling and remodeling; 4) computational models based on bio-imaging measurements in patients of HF; 5) machine learning methods to enhance the accuracy of computational biomechanics; and 6) computational models to aid in the development of medical devices for treatment of HF. Here, we briefly summarize the contributions from the 12 publications.
Computational analysis of the native cardiovascular system, and devices that are used to treat the diseased native cardiovascular system, is regularly conducted to enhance our understanding of patient anatomy and blood flow dynamics (Neidlin et al., 2021; Vatani et al., 2022). This enhanced understanding can lead to improvements in patient management, better cardiovascular devices, and additional applications of existing devices. Modern cardiovascular computational simulations have progressed beyond simplified geometries to patient-specific models based on medical imaging, with some progressing further to statistical shape models based on larger cohorts of patient data (Goubergrits et al., 2022).
In this Research Topic, three-dimensional statistical shape models of healthy patients and those with varying degrees of tricuspid valve regurgitation were generated from cardiac MRI data (Orkild et al.). Those with tricuspid regurgitation demonstrated increased right ventricular free wall bulging, narrowing of the base, and blunting of the right ventricular apex. Compared with tricuspid regurgitation, aortic stenosis is even more common, with low-flow and low-gradient aortic stenosis (LFLG AS) being a commonly recognized sub-type with controversial management strategies due in part to a lack of biomechanics knowledge. To improve the knowledge of LFLG AS biomechanics, a patient-specific model using ECG-gated cardiac computed tomography was developed using a previously validated multi-scale, multi-physics computational heart model coupled with a virtual circulatory system and calibrated to clinically-measured parameters in this issue (Wisneski et al.). Progression of valvular disease may necessitate placement of a mechanical replacement valve, with minimally invasive transcatheter aortic valve replacement (TAVR) increasing in popularity. In this issue, a fluid-structure interaction model of TAVR was used to investigate blood flow dynamics, revealing variations in maximum leaflet stress, opening area, and low-velocity areas when leaflet geometries were altered (Liu et al.).
Cardiovascular modelling techniques can combine blood flow simulations with structural models (e.g. fluid-structure interaction models), lumped parameter modelling, and even in-vivo experiments. In this issue, a combination of cardiac electrical signals, blood pressure and echocardiography imaging from a pacing animal model was combined with a cardiac fluid-structure interaction model to demonstrate the influence of pacemaker location on cardiac outcome (Fan et al.). In another research topic, a combination of patient images via computed tomography and lumped parameter modelling was used in this issue to investigate the influence of atrial fibrillation and left atrial appendage occlusion on thrombosis in a simulated patient supported by a left ventricular assist device (Ghodrati-Misek et al.). Results obtained from the advanced contractile left-heart model revealed unfavorable left atrial flow dynamics during atrial fibrillation which was improved after left atrial appendage occlusion, which may reduce the potential for thrombus formation within the left atrium.
Fractional flow reserve (FFR) is the Class Ia recommendation for guiding the decision to revascularize epicardial coronary stenoses by societal guidelines in Europe and United States (Knuuti et al., 2020; Writing Committee et al., 2022). Some practical restrictions limit the traditional wire-based FFR utilization, such as requiring drug-induced hyperemia, pressure drift of the pressure wire, and so on (Gong et al., 2020; Li et al., 2020; Gong et al., 2022). Multiple computational techniques have been developed to determine FFR based on computed tomography angiograms (CTA-FFR) (Taylor et al., 2013). The diagnostic accuracy of CTA-FFR, however, varied significantly across the spectrum of cardiovascular diseases (Cook et al., 2017). Hence, it is still required to improve the computational techniques. In this Research Topic, a method for calculating FFR based on steady-state geometric multiscale (FFRSS) was proposed based on a coronary artery model segmented from a patient’s coronary CTA images (Liu et al.). The diagnostic performance of FFRSS and traditional FFRCT was compared with the wire-based FFR. FFRss showed similar accuracy to FFRCT, but improved the calculation efficiency. Moreover, a fluid–structure interaction (FSI) algorithm with a physics-driven 3D–0D coupled mode was developed to improve the accuracy of CTA-FFR (Xi et al.). This method improved the diagnostic accuracy of CTA-FFR computation.
The coronary arterial trees include millions of blood vessels, most of them are small arterioles and pre-capillary vessels (Huo et al., 2009). An index of microcirculatory resistance (IMR) was proposed to quantify coronary microcirculatory dysfunction (Fearon et al., 2003; Aarnoudse et al., 2004; Fearon et al., 2004). However, its application within clinical practice remains extremely limited due to a complex guide wire measurement under hyperemia (Ai et al., 2020). Coronary angiography-derived IMR (caIMR) has been proven to have high correlation and diagnostic accuracy with invasive wire-based IMR (Ai et al., 2020; Choi et al., 2021). In this Research Topic, caIMR >40 was shown to be an independent predictor of the combined events including cardiovascular death or heart failure readmission and hence a promising method for prognosis in STEMI patients (Duan et al.).
Various abnormal hemodynamic parameters such as low wall shear stress (WSS), high oscillatory shear index (OSI), old blood volume fraction (OBVF), and old blood volume (OBV), have been proposed to investigate the occurrence and development of atherosclerosis (Huang et al., 2016; Fan et al., 2017; Feng et al., 2020). In this Research Topic, thrombosis risk was evaluated within occluded coronary arterial fistulas (CAF) with terminal aneurysms using untreated, aneurysm-reserved and aneurysm-removed numerical models (Jiang et al.). The OBV was found to be superior to the area of high OSI and low WSS in determining treatment type. On the other hand, competitive flow and anastomotic stenosis are two risk factors for poor instant patency of coronary artery bypass grafting (CABG) surgery (Fan et al., 2016; Fan et al., 2017). A 0D-3D coupled multiscale CABG model was developed to investigate anastomotic stenosis and competitive flow (Mao et al.). The graft flow waveform shape and flow fast Fourier transformation (FFT) ratio were found to predict the poor instant patency after CABG.
In vessel wall mechanics, wall tissue fatigue is a chronic failure process induced by repetitive loading and could impact plaque development under pulsatile blood pressure (Guo et al.). In this Research Topic, the relationship between fatigue and stenosis progression was investigated based on in-vivo intravascular ultrasound (IVUS) images and finite element models (Guo et al.). Stenosis progression was associated with the maximum stress amplitude, average stress amplitude and average strain amplitude.
In comparison with fine particle pollution (PM2.5), ultrafine particles (UFPs) (PM0.1) produce stronger chemical reactions given their small volume and large surface area (Li et al., 2021; Huo and Li). In this Research Topic, the change of cardiac function and peripheral hemodynamics was investigated in rats of myocardial infarction (MI) after long-term inhalation of ultrafine Zn particles (Huo and Li). The long-term inhalation of ultrafine zinc particles induced excessive accumulation of zinc in serum and tissue, which deteriorated cardiac and hemodynamic dysfunctions in MI rats.
In summary, this special edition incorporates novel cardiovascular modelling research into a single issue to advance the knowledge in cardiovascular disease, simulation techniques, medical devices, and more.
YH and SG wrote the manuscript together.
The authors declare that the research was conducted in the absence of any commercial or financial relationships that could be construed as a potential conflict of interest.
All claims expressed in this article are solely those of the authors and do not necessarily represent those of their affiliated organizations, or those of the publisher, the editors and the reviewers. Any product that may be evaluated in this article, or claim that may be made by its manufacturer, is not guaranteed or endorsed by the publisher.
Aarnoudse W., Fearon W. F., Manoharan G., Geven M., Van de Vosse F., Rutten M., et al. (2004). Epicardial stenosis severity does not affect minimal microcirculatory resistance. Circulation 110 (15), 2137–2142. doi:10.1161/01.CIR.0000143893.18451.0E
Ai H., Feng Y., Gong Y., Zheng B., Jin Q., Zhang H. P., et al. (2020). Coronary angiography-derived index of microvascular resistance. Front. Physiol. 11, 605356. doi:10.3389/fphys.2020.605356
Choi K. H., Dai N., Li Y., Kim J., Shin D., Lee S. H., et al. (2021). Functional coronary angiography-derived index of microcirculatory resistance in patients with ST-segment elevation myocardial infarction. JACC. Cardiovasc. Interv. 14 (15), 1670–1684. doi:10.1016/j.jcin.2021.05.027
Cook C. M., Petraco R., Shun-Shin M. J., Ahmad Y., Nijjer S., Al-Lamee R., et al. (2017). Diagnostic accuracy of computed tomography-derived fractional flow reserve : A systematic review. JAMA Cardiol. 2 (7), 803–810. doi:10.1001/jamacardio.2017.1314
Duan Y., Wang Y., Zhang M., Li Z., Chen L., Miao H., et al. (2022). Computational pressure-fluid dynamics applied to index of microcirculatory resistance, predicting the prognosis of drug-coated balloons compared with drug-eluting stents in STEMI patients. Front. Physiol. 13, 898659. doi:10.3389/fphys.2022.898659
Fan T., Feng Y., Feng F., Yin Z., Luo D., Lu Y., et al. (2017). A comparison of postoperative morphometric and hemodynamic changes between saphenous vein and left internal mammary artery grafts. Physiol. Rep. 5 (21), e13487. doi:10.14814/phy2.13487
Fan T., Lu Y., Gao Y., Meng J., Tan W., Huo Y., et al. (2016). Hemodynamics of left internal mammary artery bypass graft: Effect of anastomotic geometry, coronary artery stenosis, and postoperative time. J. Biomech. 49 (5), 645–652. doi:10.1016/j.jbiomech.2016.01.031
Fearon W. F., Aarnoudse W., Pijls N. H., De Bruyne B., Balsam L. B., Cooke D. T., et al. (2004). Microvascular resistance is not influenced by epicardial coronary artery stenosis severity: Experimental validation. Circulation 109 (19), 2269–2272. doi:10.1161/01.CIR.0000128669.99355.CB
Fearon W. F., Balsam L. B., Farouque H. M., Caffarelli A. D., Robbins R. C., Fitzgerald P. J., et al. (2003). Novel index for invasively assessing the coronary microcirculation. Circulation 107 (25), 3129–3132. doi:10.1161/01.CIR.0000080700.98607.D1
Feng Y., Liu J., Fan T., Zhang W., Yin X., Tan Y. E., et al. (2020). Vertebral artery stenoses contribute to the development of diffuse plaques in the basilar artery. Front. Bioeng. Biotechnol. 8, 168. doi:10.3389/fbioe.2020.00168
Ghodrati-Misek M., Schlöglhofer T., Gross C., Maurer A., Zimpfer D., Beitzke D., et al. (2022). Left atrial appendage occlusion in ventricular assist device patients to decrease thromboembolic events: A computer simulation study. Front. Physiol. 13, 1010862. doi:10.3389/fphys.2022.1010862
Gong Y., Feng Y., Yi T., Yang F., Li Y., Zhang L., et al. (2020). Coronary angiography-derived diastolic pressure ratio. Front. Bioeng. Biotechnol. 8, 596401. doi:10.3389/fbioe.2020.596401
Gong Y. J., Zheng B., Yi T. C., Yang F., Hong T., Liu Z. P., et al. (2022). Coronary angiography-derived contrast fractional flow reserve. Catheter. Cardiovasc. Interv. 99 (3), 763–771. doi:10.1002/ccd.29558
Goubergrits L., Vellguth K., Obermeier L., Schlief A., Tautz L., Bruening J., et al. (2022). CT-based analysis of left ventricular hemodynamics using statistical shape modeling and computational fluid dynamics. Front. Cardiovasc. Med. 9.doi:10.3389/fcvm.2022.90190
Huang X., Yin X., Xu Y., Jia X., Li J., Niu P., et al. (2016). Morphometric and hemodynamic analysis of atherosclerotic progression in human carotid artery bifurcations. Am. J. Physiol. Heart Circ. Physiol. 310 (5), H639–H647. doi:10.1152/ajpheart.00464.2015
Huo Y., Kaimovitz B., Lanir Y., Wischgoll T., Hoffman J. I., Kassab G. S. (2009). Biophysical model of the spatial heterogeneity of myocardial flow. Biophys. J. 96 (10), 4035–4043. doi:10.1016/j.bpj.2009.02.047
Knuuti J., Wijns W., Saraste A., Capodanno D., Barbato E., Funck-Brentano C., et al. (2020). 2019 ESC Guidelines for the diagnosis and management of chronic coronary syndromes. Eur. Heart J. 41 (3), 407–477. doi:10.1093/eurheartj/ehz425
Li J., Gong Y., Wang W., Yang Q., Liu B., Lu Y., et al. (2020). Accuracy of computational pressure-fluid dynamics applied to coronary angiography to derive fractional flow reserve: Flash FFR. Cardiovasc. Res. 116 (7), 1349–1356. doi:10.1093/cvr/cvz289
Li L., Niu P., Wang X., Bing F., Tan W., Huo Y. (2021). Short-term inhalation of ultrafine zinc particles could alleviate cardiac dysfunctions in rats of myocardial infarction. Front. Bioeng. Biotechnol. 9, 646533. doi:10.3389/fbioe.2021.646533
Liu J., Wang X., Li B., Huang S., Sun H., Zhang L., et al. (2022a). Non-invasive quantification of fraction flow reserve based on steady-state geometric multiscale models. Front. Physiol. 13, 881826. doi:10.3389/fphys.2022.881826
Neidlin M., Liao S., Li Z., Simpson B., Kaye D. M., Steinseifer U., et al. (2021). Understanding the influence of left ventricular assist device inflow cannula alignment and the risk of intraventricular thrombosis. Biomed. Eng. Online 20 (1), 47. doi:10.1186/s12938-021-00884-6
Taylor C. A., Fonte T. A., Min J. K. (2013). Computational fluid dynamics applied to cardiac computed tomography for noninvasive quantification of fractional flow reserve scientific basis. J. Am. Coll. Cardiol. 61 (22), 2233–2241. doi:10.1016/j.jacc.2012.11.083
Vatani A., Liao S., Burrell A. J. C., Carberry J., Azimi M., Steinseifer U., et al. (2022). Improved drainage cannula design to reduce thrombosis in veno-arterial extracorporeal membrane oxygenation. Asaio J. 68 (2), 205–213. doi:10.1097/MAT.0000000000001440
Writing Committee M., Lawton J. S., Tamis-Holland J. E., Bangalore S., Bates E. R., Beckie T. M., et al. (2022). 2021 ACC/AHA/SCAI guideline for coronary artery revascularization: A report of the American college of cardiology/American heart association joint committee on clinical practice guidelines. J. Am. Coll. Cardiol. 79 (2), e21–e129.doi:10.1161/CIR.0000000000001039
Keywords: computational biomechanics, heart failiure, hemodynamics, FFR = fractional flow reserve, soft tissue mechanical properties
Citation: Huo Y and Gregory SD (2022) Editorial: Computational biomechanics for ventricle-arterial dysfunction and remodeling in heart failure, Volume II. Front. Physiol. 13:1100037. doi: 10.3389/fphys.2022.1100037
Received: 16 November 2022; Accepted: 28 November 2022;
Published: 08 December 2022.
Edited and reviewed by:
Raimond L. Winslow, Northeastern University, United StatesCopyright © 2022 Huo and Gregory. This is an open-access article distributed under the terms of the Creative Commons Attribution License (CC BY). The use, distribution or reproduction in other forums is permitted, provided the original author(s) and the copyright owner(s) are credited and that the original publication in this journal is cited, in accordance with accepted academic practice. No use, distribution or reproduction is permitted which does not comply with these terms.
*Correspondence: Yunlong Huo, aHVveXVubG9uZ0BzanR1LmVkdS5jbg==; Shaun D. Gregory, c2hhdW4uZ3JlZ29yeTFAZ21haWwuY29t
Disclaimer: All claims expressed in this article are solely those of the authors and do not necessarily represent those of their affiliated organizations, or those of the publisher, the editors and the reviewers. Any product that may be evaluated in this article or claim that may be made by its manufacturer is not guaranteed or endorsed by the publisher.
Research integrity at Frontiers
Learn more about the work of our research integrity team to safeguard the quality of each article we publish.