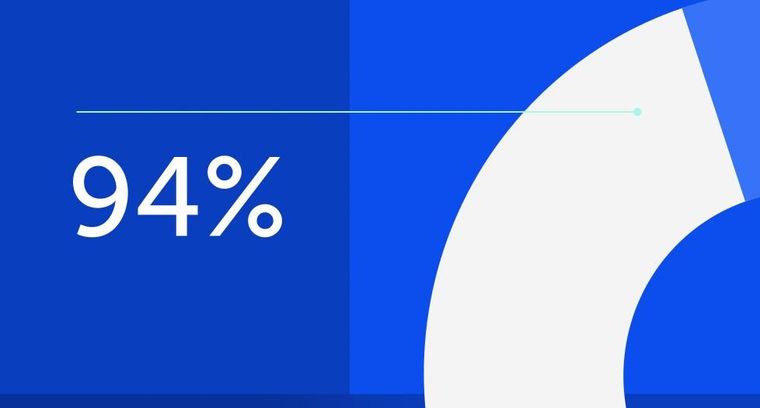
94% of researchers rate our articles as excellent or good
Learn more about the work of our research integrity team to safeguard the quality of each article we publish.
Find out more
ORIGINAL RESEARCH article
Front. Physiol., 20 October 2022
Sec. Exercise Physiology
Volume 13 - 2022 | https://doi.org/10.3389/fphys.2022.1004863
Background: Exercise can modulate gut microbiota and lower the risk of cardiovascular disease (CVD). However, the association between exercise-induced changes in gut microbiota and CVD risk have not been investigated.
Objective: This study determined the effects of exercise training on CVD risk and gut microbiota in physically active elderly women and whether exercise-induced gut microbiota changes were associated with CVD risk.
Methods: An 8-week randomized controlled trial was conducted with 14 elderly women assigned to exercise group (n = 8) or control group (n = 6). Physical function, sarcopenic obesity, and metabolic syndrome were evaluated as components of CVD risk. Gut microbiota composition was determined using 16S rRNA gene sequencing. Repeated-measures analysis of variance was used to examine intra-group and inter-group differences.
Results: A significant group × time interaction was observed for chair sit-and-reach (F = 8.262, p = 0.014), single-leg standing with eyes closed (F = 7.340, p = 0.019), waist circumference (F = 6.254, p = 0.028), and body fat mass (F = 12.263, p = 0.004), for which the exercise group showed improved trends. The exercise group exhibited significant improvements in skeletal muscle mass (p = 0.041) and fasting blood glucose (p = 0.017). Regarding gut microbiota, a significant interaction was observed for the class Betaproteobacteria (F = 6.822, p = 0.023) and genus Holdemania (F = 4.852, p = 0.048).
Conclusion: The 8-week exercise training improved physical function, lowered CVD risk, and modulated relative abundance of gut microbiota associated with CVD in physically active elderly women.
Cardiovascular disease (CVD) is characterized by pathologic changes of the heart, blood vessels and cerebrovascular system (Cannon, 2013), which is the major cause of death globally, accounting for approximately 17.8 million deaths in 2017 (Kyu et al., 2018). The prevalence of CVD is high in the elderly Chinese population and there is an increasing trend in the younger population (Wang et al., 2015). Previous studies have reported that multiple risk factors contribute to the development of CVD such as metabolic syndrome (Lakka et al., 2002), sarcopenia (Matsubara et al., 2017). Among these aspects, physical inactivity is an important factor in the induction of CVD, which is also the modifiable behavioral risk factor most strongly associated with CVD except for tobacco use (Yusuf et al., 2020). Conversely, regular exercise can have a favorable effect on many risk factors for CVD (Thompson et al., 2003). However, the effects of the type and volume of exercise on CVD risk requires additional attention.
A recent study showed that combined aerobic and resistance exercise may provide more comprehensive benefits and a greater reduction of CVD risk compared to that of aerobic or resistance exercise alone (Schroeder et al., 2019). Moreover, randomized controlled trial studies of exercise-induced cardiovascular risk reduction have focused on physically inactive older adults (Beavers et al., 2018). This has led to a lack of effective evidence regarding people with habits of physical activity. The significance of this is highlighted by the fact that a dose–response association was observed between physical activity level and risk of CVD in a recent large cohort study (Ramakrishnan et al., 2021). The association was found whether total physical activity level, moderate-intensity physical activity level, or vigorous-intensity physical activity level was considered. Thus, it is of interest and relevance to determine the effect of combined aerobic and resistance exercise on CVD risk in elderly people that maintain exercise habits.
The potential mechanism of aerobic exercise-induced CVD risk reduction is via a pathway that may lead to increased insulin sensitivity (Gao et al., 2002), while resistance exercise may regulate mitophagy and inhibiting oxidative stress (Li et al., 2021). However, deeper potential mechanisms need to be explored. Interestingly, the effects of changes in the gut microbiota composition on CVD risk has attracted attention and gut microbiota dysbiosis may contribute to a higher risk of CVD (Witkowski et al., 2020). Furthermore, accumulating evidence demonstrates that exercise can influence the gut microbiota composition in humans. We previously reported that an 8-week exercise training regimen can induce the reduction of bacteria associated with pro-inflammation, such as Proteobacteria, in physically inactive older women (Zhong et al., 2020). The underlying mechanisms that exercise-induced changes of gut microbiota involved the acceleration of the mixing of internal contents (Mailing et al., 2019) and colon transit (Song et al., 2012), and the improvement of the permeability of the intestinal mucosal barrier (Holland et al., 2015). Furthermore, exercise can regulate the hypothalamic–pituitary–adrenal (HPA) axis, thereby affecting the composition of the gut microbiota (Bermon et al., 2015). However, little is currently known regarding the association between exercise-induced change in gut microbiota composition and CVD risk.
Our current study investigated the effects of an 8-week combined aerobic and resistance training regimen on CVD risk and gut microbiota composition in physically active elderly women. We also aimed to determine whether exercise-induced changes in the composition of gut microbiota were associated with CVD risk.
An 8-week RCT of combined aerobic and resistance training was performed. The study was conducted from December 2020 to February 2021. The study protocol was approved by the Ethics Committee at the School of Public Health Zhejiang University (Code: EGL201810-2) and registered in the Chinese Clinical Trial Registry (Code: ChiCTR1800019711). All study participants provided written informed consent.
The participants were recruited from the community and of the age between 60 and 75 years (inclusive). These participants were capable of living independently. Participants were excluded for the following reasons: 1) had been treated for a malignant tumor; 2) had experienced acute myocardial infarction, heart failure; 3) had a history of stroke; 4) was taking antihypertensive or lipid lowering medications; 5) had any disease that restricted physical activity; 6) had any medical condition that limited the ability to perform safe exercise; 7) presented with fasting triglyceride ≥2.26 mmol/L, serum creatinine ≥200 μmol/L, systolic blood pressure ≥160 mmHg, or diastolic blood pressure ≥100 mmHg; 8) had some degree of cognitive impairment with a Mini-Mental State Examination (MMSE) score of less than 24; 9) had taken antibiotics within 1 month prior to the start of the study.
A total of 112 older adults were recruited in the community through telephone and posters, of which 63 were willing to participate in this study, and 46 of them attended the health advocacy conference on time. Individuals were selected via a questionnaire and health examination. 29 subjects were excluded: 15 individuals did not meet the age requirements, 2 individuals had CVD, 8 individuals had hypertension, 3 individuals had hyperlipidemia, 1 individual was a breast cancer patient. 2 people withdrew prior to the hospital-based medical examination. Ultimately, 15 participants were randomly assigned to the Exe group (n = 8) and Con group (n = 7). A computer-generated list of random numbers was used to assign participants to the groups. After the intervention, one participant assigned to the Con group dropped out of the study. Therefore, the final analysis included eight subjects in the Exe group and six subjects in the Con group. The study design of the trial is shown in Figure 1.
FIGURE 1. Fow diagram of the research design of the randomized controlled trial. Recruitment, screening, randomization, intervention, outcomes assessment, and data analysis.
Participants assigned to the Con group were to maintain daily life. The subjects in the Exe group took part in exercise program in which they met four times per week from 9:30 am to 10:30 am. Each session lasted approximately 60 min, including a warm-up period (10 min), aerobic exercise (20 min), resistance exercise (25 min), and a cool down period (5 min). The protocols of the exercise intervention have been described previously (Zhong et al., 2020).
Information regarding socio-demographics (age, sex, monthly income, educational level, marital status, residence status), health-related behaviors (smoking status, alcohol use), and physical activity were collected. Individuals with a Physical Activity Scale for the Elderly (PASE) score greater than 90 tend to undergo moderate to intense activity in their daily life (Curcio et al., 2019). A standardized protocol was used to assess body height (cm) and weight (kg). BMI was calculated [weight (kg)/height squared (m2)].
Chair sit-and-reach, single-leg standing with eyes closed, 2-minute step test, grip strength, 30-s chair stand test, and timed up and go test were used to assess flexibility, balance, aerobic capacity, muscle strength, and functional mobility of the participants (Podsiadlo and Richardson, 1991; Rikli and Jones, 1999). A V-BODY HBF-701 bioelectrical impedance analyzer (Omron Healthcare Co. Ltd., Kyoto, Japan) was used to estimate the percentage of skeletal muscle mass. Skeletal muscle mass (kg) was calculated as body weight (kg) × skeletal muscle percentage and body fat (kg) was calculated as body weight (kg) × body fat percentage (Ohara et al., 2014; Ohara et al., 2015).
Stool samples of the participants were collected before the baseline test and after the eight weeks of exercise training. A MagPure Stool DNA KF Kit B (Magen, China) was used to extract microbial community DNA. The 16S rRNA gene of the gut microbiota was amplified using degenerate PCR primers of regions V3–V4. Sequencing was performed using the Illumina MiSeq platform (BGI, Shenzhen, China) and 2 × 300 bp paired-end reads were generated. After filtering the raw reads, the Fast Length Adjustment of SHort reads (FLASH, v1.2.11) software was used for adding the paired-end reads to tags (Magoc and Salzberg, 2011). The UPARSE (USEARCH version 7.0.1090) software (Edgar, 2013) was used to cluster the tags into operational taxonomic units (OTUs) and UCHIME (v4.2.40) (Edgar et al., 2011) was used to detect chimera sequences. The OTU representative sequences were classified using Ribosomal Database Project (RDP) Classifier v.2.2 and trained using QIIME v1.8.0 software (Caporaso et al., 2010). USEARCH_global was used to compare tags back to the OTUs and then OTU abundance was obtain for each sample (Edgar, 2010).
SPSS Statistics version 23.0 software (SPSS Inc., Chicago, IL, United States) was used to analyze the data. Mean ± standard deviation is used to describe continuous variables and percentage is used to expressed categorical variables. Normal distribution of the variables was verified using the Shapiro-Wilk test. The independent samples t-test (normally distributed data) and Mann-Whitney U test (non-normally distributed data) were used for the comparison between two groups. Comparisons of categorical variables were estimated using Fisher’s exact test. Paired samples t-test (normally distributed data) and Wilcoxon signed-ranks test (non-normally distributed data) were used for comparisons within groups. Repeated-measures analysis of variance (RM-ANOVA) was used to examine intra-group and inter-group differences of the main observational variables. A 95% confidence interval (CI) was selected to calculate differences between groups, there was statistical differences when p < 0.05. The association between two variables was evaluated using Pearson correlation analysis.
Baseline characteristics of the study participants are shown in Table 1. At baseline, there were no significant differences in demographic or anthropometric parameters between the two groups. The age of the participants in the Exe group was 66.38 ± 4.07 years and 68.50 ± 3.78 years in the Con group. The average height of the Exe group was 157.94 ± 7.07 cm compared to 156.58 ± 5.30 cm for the Con group. The average weight was 52.85 ± 6.82 kg for the Exe group and 52.88 ± 2.90 kg for the Con group. The body mass index (BMI) for the Exe group was 21.16 ± 2.17 kg/m2 compared to 21.61 ± 1.50 kg/m2 for the Con group. Physical Activity Scale for the Elderly (PASE) scores for the Exe group was 117.11 ± 24.49 and 105.36 ± 49.90 for the Con group. Therefore, the participants of our study were physically active elderly women. Finally, no statistical differences were detected in education, marital status, residential status, smoking, or alcohol use between the two study groups.
Significant increases were found in alpha diversity of the Sobs index (p = 0.008), Chao index (p = 0.017), and Ace index (p = 0.002) in the Exe group after intervention (Supplementary Table S1; Figure 2). A significant time main effect also was observed for these three variables [Sobs (F = 15.273, p = 0.002); Chao (F = 13.402, p = 0.003); Ace (F = 16.637, p = 0.002)].
FIGURE 2. Changes in the alpha diversity indices of gut microbiota after exercise training. Exe: combined aerobic and resistance exercise, Con: maintaining daily life; sobs, observed species index; chao, Chao index; ace, Ace index; shannon, Shannon index; simpson, Simpson index; (A–E) Pre-exercise. (F–J) After 8-week exercise regimen.
At the class, order, and family level, a significant group × time interaction was observed for the relative abundance of Betaproteobacteria (F = 6.822, p = 0.023), and Sutterellaceae (F = 7.075, p = 0.021), respectively (Table 2) and (Figure 3). These bacteria in the Con group showed a significant simple effect of group. Additionally, there was a significant group × time interaction for the genus Holdemania (F = 4.852, p = 0.048) and a significant simple effect of group was demonstrated in the Exe group (F = 6.055, p = 0.030).
TABLE 2. Changes in gut microbiota and cardiovascular risk factors in each group following the intervention.
FIGURE 3. Changes in the relative abundance of gut microbiota components after exercise training. Exe: combined aerobic and resistance exercise, Con: maintaining daily life. (A–E) Pre-exercise. (F–J) After 8-week exercise regimen. (A,F) Phylum; (B,G) Class; (C,H) Order; (D,I) Family; (E,J) Genus.
The relative abundance of members of the order Coriobacteriales (p = 0.012) and family Coriobacteriaceae (p = 0.012) were significantly increased in the Exe group. We also observed a significant increase in the relative abundance of Asaccharobacter (p = 0.028), Collinsella (p = 0.028), and Fusicatenibacter (p = 0.049) in the Exe group and a significant time main effect was demonstrated for Asaccharobacter (F = 4.875, p = 0.047) (Supplementary Table S1; Figure 3). Furthermore, a significant main effect of group was detected for the relative abundance of members of the class Bacilli (F = 6.336, p = 0.027), order Lactobacillales (F = 6.378, p = 0.027), family Bacteroidaceae (F = 9.711, p = 0.009), genera Anaerostipes (F = 10.160, p = 0.008) and Bacteroides (F = 9.711, p = 0.009) (Supplementary Table S1) and (Figure 3).
The physical function parameters of the subjects were evaluated, which included chair sit-and-reach, single-leg standing with eyes closed, 2-min step test, grip strength, 30-s chair stand test, and timed up and go test (Table 3). A significant group × time interaction was observed between the two groups for chair sit-and-reach (F = 8.262, p = 0.014) and single-leg standing with eyes closed (F = 7.340, p = 0.019). Both these physical functions in the Exe group were significantly improved compared to that in the Con group after exercise intervention [chair sit-and-reach (p = 0.002); single-leg standing with eyes closed (p = 0.046)]. The 2-min step test and grip strength scores did not change significantly, but did show a trend of improvement in the Exe group compared with that in the Con group.
Waist circumference, waist-hip ratio, BMI, skeletal muscle mass, and body fat mass are predictors of sarcopenic obesity. We found a significant group × time interaction for waist circumference (F = 6.254, p = 0.028) and waist-hip ratio (F = 5.935, p = 0.031). In the Con group, body fat mass increased significantly (F = 12.263, p = 0.004), which mainly reflected the waist circumference (F = 7.441, p = 0.018) and waist-hip ratio (F = 10.226, p = 0.008). The fat mass decreased significantly in the Exe group compared to that in the Con group (F = 8.392, p = 0.013). Moreover, skeletal muscle mass of subjects in the Exe group indicated a significant improvement after exercise intervention (p = 0.041) (Table 2, Table 3, and Figure 4).
FIGURE 4. Changes in body composition after exercise training. Exe: combined aerobic and resistance exercise, Con: maintaining daily life.
The metabolic syndrome components were evaluated based on recommendations on metabolic syndrome from the Chinese Diabetes Society (Metabolic Syndrome Research Group of Chinese Medical Association, 2004), which included BMI, fasting blood glucose, blood pressure, triglycerides (TG), and high density lipoprotein cholesterol (HDL-C). Although a significant main effect of time was found for fasting blood glucose (F = 12.551, p = 0.004), only a significant reduction was observed in the Exe group. Furthermore, HDL-C showed a significant main effect of time (F = 46.862, p < 0.001). TG increased to a greater extent in the Con group compared to that in the Exe group (Table 3).
We also evaluated other risk factors for CVD, such as serum creatinine (Cr), serum total cholesterol (TC), cortisol (COR), C-reactive protein (CRP), hemoglobin A1c (HbA1c), and tumor necrosis factor-alpha (TNF-α). A significant group × time interaction was observed for COR (F = 7.084, p = 0.021), which showed a decrease in the Exe group and increase in the Con group after exercise intervention. In addition, significant main effect of time was detected for TC (F = 5.981, p = 0.031) and TNF-α (F = 36.730, p < 0.001). TC increased to a greater extent in the Con group compared to that in the Exe group (Tables 2, 3).
Chair sit-and-reach was negatively associated with Betaproteobacteria (r = −0.0704, p = 0.005). Single-leg standing with eyes closed was inversely correlated with Holdemania (r = −0.553, p = 0.040). Grip strength was negatively associated with Betaproteobacteria (r = −0.551, p = 0.041). Meanwhile, chair sit-and-reach was negatively associated with Betaproteobacteria (r = −0.704, p = 0.005). Skeletal muscle mass was negatively associated with Holdemania (r = −0.580, p = 0.030) (Figure 5).
FIGURE 5. Relationship between changes in physical function and changes in gut microbiota after exercise intervention.
We believe this study is the first RCT to evaluate the effects of combined aerobic and resistance training on the gut microbiota and cardiovascular risk factors in physically active elderly women. Our main findings showed that the 8-week exercise program could improve physical function, lower CVD risk, alter alpha and beta diversity of the gut microbiota, and modulate the relative abundance of gut microbiota associated with CVD for the study participants. These findings indicate that exercise may still be effective in older adults that include exercise in their routines for preventing and controlling the risk of developing CVD.
Sarcopenic obesity is a strong predictor of CVD (Evans et al., 2021), characterized by the combination of low muscle mass (sarcopenia) and excess adiposity (obesity), which are evaluated according to skeletal muscle mass, fat mass, physical function, waist circumference, and BMI (Lee et al., 2016). Studies have shown that the skeletal muscle mass of elderly individuals who participated in combined aerobic and resistance exercise is increased and body fat is decreased (Chen et al., 2017), similar findings were also observed in our results. In addition to the improvement of body composition, we also observed enhancements in several physical function indicators such as balance function and flexibility, which were closely associated with the fall risk (Emilio et al., 2014). In fact, muscle weakness and poor balance ability are the most important risk factors of fall according to the ranking, and improvements in these functions can induce a reduction in fall risk (Rubenstein, 2006). In this study, exercise training ameliorates the muscle function and balance ability, which is consistent with previous study (Villareal et al., 2017). Thus, these findings suggest that the combination of structured aerobic and resistance training may reduce the risk of sarcopenic obesity and falls associated with impaired physical function in older adults.
A reduced risk of metabolic syndrome has also observed in exercise groups, which is important in that metabolic syndrome is a precursor of CVD (Wilson et al., 2005). A 12-week regimen of resistance band exercise training has been shown to improved glucose and blood lipid profile in obese postmenopausal women, thereby reducing the risk factors for metabolic syndrome (Son and Park, 2021). Both combined high-intensity aerobic and resistance exercise and low-intensity physical activity programs can improve some parameters of metabolic syndrome in elderly women, such as blood pressure, waist circumference, and TG (Kemmler et al., 2009). In our study, exercise significantly improved blood glucose of the participants, while TG in the Con group showed an increasing trend after 8 weeks. However, the HDL-C of individuals showed an overall decreasing trend in both study groups after intervention, which accordingly may have been related to temperature. The HDL-C is negatively associated with environmental temperature, being higher in the winter and lower in the spring (Wang et al., 2020). In our current study, the pre-test season was winter with the average temperature in the first 5 days of physical examination being 8 °C. The post-test season had just entered early spring with the average temperature in the first 5 days of physical examination being 9.9°C. The HDL-C of individuals showed an overall decreasing trend in both study groups after intervention, which accordingly may have been related to temperature.
In regard to other CVD risk factors, a previous study indicated a trend for greater increased total cholesterol of the control group compared to that of the exercise group after an 8-week combination of aerobic and resistance exercise (Ho et al., 2012). This finding is consistent with our current results. Moreover, our exercise training induced a significant decrease in the pro-inflammatory marker cortisol, which was similar to a previous study (Roberts et al., 2013). In addition, consideration of seasonal factors is important in evaluating inflammatory markers, including TNF-a, IL-6, IL-10, and CRP (Stewart et al., 2007; McFarlane et al., 2012). Serum creatinine of children with urinary 1-hydroxypyrene exhibits significant seasonal differences with it being higher in cold seasons compared to that in warm seasons (Chen et al., 2015). There were some seasonal differences before and after the intervention program of our study. Therefore, the significant consistent changes of serum creatinine and TNF-α in the Exe group and Con group may have been season associated.
Our findings also indicate that exercise could influence gut microbiota composition. Greater gut microbiota diversity is more protective for maintaining intestinal homeostasis, improving the integrity of the intestinal mucosal barrier (Human Microbiome Project, 2012; Faith et al., 2013), and resisting the harmful factors in the environment (Sommer et al., 2017). Conversely, a lack of gut microbiota diversity in humans is associated with multiple diseases, including type 2 diabetes (Miao et al., 2020) and CVD (Kelly et al., 2016). Thus, a more diverse gut microbiota can be considered beneficial to health. Previous studies indicate that exercise training can contribute to higher alpha diversity (Hampton-Marcell et al., 2020), which was athletes who participated in high-intensity and great-frequency training. Patients with quiescent inflammatory bowel disease undergoing a moderate-intensity progressive combination of aerobic and resistance exercise (3 times per week for 8 weeks) also show a slight increase in alpha diversity after the exercise intervention (Cronin et al., 2019). However, there are also several previous reports suggesting that moderate and low-to-moderate intensity aerobic exercise (or combined aerobic and resistance exercise) performed three times a week for durations ranging from 5 to 8 weeks did not significantly affect the gut microbiota alpha diversity of elderly individuals (Taniguchi et al., 2018), overweight or obese people (Cronin et al., 2018), or sedentary people (Munukka et al., 2018). Of note, our results show that an 8-week exercise training four times per week could significantly increase some indicators of alpha diversity of gut microbiota in active elderly people. Therefore, increasing frequency of exercise during short-term exercise interventions may partially increase the alpha diversity of gut microbiota.
The association between parameters of sarcopenic obesity and gut microbiota have been only rarely reported. Our results revealed a possible relationship between these parameters and consistent with previous studies. For example, flexibility (chair sit-and-reach) and muscle strength (grip strength-weight ratio) were inversely correlated with Betaproteobacteria, a class of bacteria associated with obesity (Quiroga et al., 2020). Skeletal muscle mass was negatively associated with Holdemania, which is associated with impaired glucose metabolism (Lippert et al., 2017). Previous studies have shown that Betaproteobacteria is more abundant in obese individuals compared to that in healthy individuals (Quiroga et al., 2020). Our current study indicated that prevalence of Betaproteobacteria decreased slightly after exercise intervention and the risk of obesity decreased for the Exe group. These changes showed opposite trends in the Con group. A higher abundance of Fusicatenibacter is observed in non-obese adults compared to that in obese adults (Jonduo et al., 2020) and exercise training significantly increased the relative abundance of this bacteria in our study. Still, other bacteria associated with obesity, such as the genus Coprococcus, are higher in non-obese subjects compare to that in obese subjects (Jonduo et al., 2020). It is reported that exercise training can increase the relative abundance of Coprococcus (Hampton-Marcell et al., 2020), which is consistent with our current results. Moreover, there is increasing evidence that suggests Sutterella is more abundant in obese individuals compared to that in individuals of normal weight (Chen et al., 2020). Our results indicated an increase of Sutterella in the Con group. Thus, according to our results and previous findings, these bacteria may be associated with sarcopenic obesity.
With respect to the association between gut microbiota and glycolipid metabolism, a previous study revealed the relative abundance of the genus Holdemania is associated with impaired lipid and glucose metabolism in older adults (Lippert et al., 2017). Although our study did not observe a statistically significant association between Holdemania and glycolipid metabolism, the abundance of this bacteria was decreased in the Exe group after exercise intervention. Notably, the family Coriobacteriaceae is considered to be a contributor to some metabolic functions, such as glucose homeostasis and lipid metabolism (Clavel et al., 2014; Liu et al., 2018) and higher abundance of Coriobacteriaceae members may be beneficial to the host (Kim et al., 2020). Moreover, a higher proportion of Collinsella has also been observed in overweight or obese women with better lipid profiles (Gomes et al., 2019). Our current results indicated a significant increase for the two bacteria in the Exe group after intervention. Thus, exercise may modulate the abundance of these bacteria and thereby reduce the risk of CVD.
The potential mechanisms of exercise affecting cardiovascular and metabolic risk factors may be mediated through microbial metabolic pathways. Exercise is known to induce increased concentrations of short-chain fatty acids (SCFAs) such as butyrate, which are metabolized by gut microbiota (Allen et al., 2018). Many bacteria have the ability to produce SCFAs, including Clostridia (Louis and Flint, 2009) and Ruminococcaceae (Choi et al., 2021). These gut microbiota can regulate blood pressure, body fat, and glucose by producing SCFAs (Morrison and Preston, 2016), which showed an increasing trend in our study after exercise intervention compared to that of the Con group. Furthermore, it has been previously reported that SCFAs can induce a decrease in blood pressure (Onyszkiewicz et al., 2019) and fat mass (Sahuri-Arisoylu et al., 2016). Host SCFA G-protein-coupled receptors may play a role in SCFA-mediated host-microbe communication in regulating blood pressure (Poll et al., 2020) and fat mass development (Lu et al., 2016). In addition, intestinal permeability, low-grade endotoxemia, and branched-chain amino acids may all be involved in possible mechanisms linking the gut microbiota and glucose homeostasis (Utzschneider et al., 2016). Meanwhile, regular exercise can protect the function and permeability of the gut barrier and promote the gut microbiota in supporting a healthy phenotype (Fiuza-Luces et al., 2018). Also, crosstalk between the gut microbiota and skeletal muscle is a two-way process and changes in the gut microbiota may modulate skeletal muscle bioenergetics by altering substrate availability (Hawley, 2020). However, the precise mechanism of the interaction between exercise, the gut microbiota, and CVD risk remains to be elucidated.
Several limitations of our current study should be considered. First, confounding factors were not considered when observing the effects of exercise training on gut microbiota composition and cardiovascular disease risk, which may partially influence our findings. In order to lower the influence of confounding factors such as dietary intakes (Scott et al., 2013), the RCT was designed, which can optimize the likelihood that confounding variables from measurement and non-measurement are distributed equally, and enable any difference in results to be attributed to the exercise training (Handley et al., 2018). Second, the absence analysis of gut microbiota metabolites may influence our further discussion regarding the mechanisms of exercise affecting CVD risk. Third, some results and findings from this study may be underestimated due to the relatively small sample size and further studies using large sample sizes are needed.
Our study indicated that an 8-week regimen of combined aerobic and resistance training could improve the physical function of physically active elderly women and lower their risk of sarcopenic obesity and metabolic syndrome. Exercise altered the alpha diversity of the gut microbiota and modulated the relative abundance of gut microbiota associated with CVD, such as Betaproteobacteria and Holdemania. In the future, metabolic analyses will be necessary to further interpret these findings and identify the potential pathways involved.
Raw reads were uploaded to the NCBI SRA (National Center for Biotechnology information Sequence Read Archive) database under BioProject ID PRJNA763801 (http://www.ncbi.nlm.nih.gov/bioproject/763801). The other datasets supporting these studies conclusion are available from the corresponding author.
The studies involving human participants were reviewed and approved by Ethics Committee at the School of Public Health Zhejiang University. The participants provided their written informed consent to participate in this study.
FZ, YX, XL, and CH: designing the experiment; FZ and CH: Implementing exercise intervention; FZ, YX, H-YL, MY, LC, XL, and CH: acquisition of subjects and data; FZ, YX, H-YL, MY, LC, XS, YY, and CH: statistical analyses and interpretation of data; FZ, YX, and CH: drafting the manuscript; FZ, YX, H-YL, MY, LC, XS, JW, WL, and CH: revision of the manuscript; YX, XS, YY, JW, WL, and CH: study supervision. All authors revised and approved the final version.
This study was supported by the Hangzhou philosophy and social science project (Z20JC074), China; The Fundamental Research Funds for the Central Universities (204201*172220192), China; The National Social Science Fund of China (20FTYB014), China.
The authors sincerely thank the participants of taking part in this study. We would also like to express our earnest appreciation to the staffs from Kunshan Old Companion Home Care Service Agency and Community Neighborhood Committee of Xiu Yi in Kunshan City.
The authors declare that the research was conducted in the absence of any commercial or financial relationships that could be construed as a potential conflict of interest.
All claims expressed in this article are solely those of the authors and do not necessarily represent those of their affiliated organizations, or those of the publisher, the editors and the reviewers. Any product that may be evaluated in this article, or claim that may be made by its manufacturer, is not guaranteed or endorsed by the publisher.
The Supplementary Material for this article can be found online at: https://www.frontiersin.org/articles/10.3389/fphys.2022.1004863/full#supplementary-material
Allen J. M., Mailing L. J., Niemiro G. M., Moore R., Cook M. D., While B. A., et al. (2018). Exercise alters gut microbiota composition and function in lean and obese humans. Med. Sci. Sports Exerc. 50 (4), 747–757. doi:10.1249/MSS.0000000000001495
Beavers K. M., Walkup M. P., Weaver A. A., Lenchik L., Kritchevsky S. B., Nicklas B. J., et al. (2018). Effect of exercise modality during weight loss on bone health in older adults with obesity and cardiovascular disease or metabolic syndrome: A randomized controlled trial. J. Bone Min. Res. 33 (12), 2140–2149. doi:10.1002/jbmr.3555
Bermon S., Petriz B., Kajeniene A., Prestes J., Castell L., Franco O. L. (2015). The microbiota: An exercise immunology perspective. Exerc. Immunol. Rev. 21, 70–79.
Cannon B. (2013). Cardiovascular disease: Biochemistry to behaviour. Nature 493 (7434), S2–S3. doi:10.1038/493S2a
Caporaso J. G., Kuczynski J., Stombaugh J., Bittinger K., Bushman F. D., Costello E. K., et al. (2010). QIIME allows analysis of high-throughput community sequencing data. Nat. Methods 7 (5), 335–336. doi:10.1038/nmeth.f.303
Chen H. T., Chung Y. C., Chen Y. J., Ho S. Y., Wu H. J. (2017). Effects of different types of exercise on body composition, muscle strength, and IGF-1 in the elderly with sarcopenic obesity. J. Am. Geriatr. Soc. 65 (4), 827–832. doi:10.1111/jgs.14722
Chen X., Sun H., Jiang F., Shen Y., Li X., Hu X., et al. (2020). Alteration of the gut microbiota associated with childhood obesity by 16S rRNA gene sequencing. PeerJ 8, e8317. doi:10.7717/peerj.8317
Chen Y. T., Huang Y. K., Luvsan M. E., Gombojav E., Ochir C., Bulgan J., et al. (2015). The influence of season and living environment on children's urinary 1-hydroxypyrene levels in Ulaanbaatar, Mongolia. Environ. Res. 137, 170–175. doi:10.1016/j.envres.2014.11.022
Choi S. I., Son J. H., Kim N., Kim Y. S., Nam R. H., Park J. H., et al. (2021). Changes in cecal microbiota and short-chain fatty acid during lifespan of the rat. J. Neurogastroenterol. Motil. 27 (1), 134–146. doi:10.5056/jnm20148
Clavel T., Desmarchelier C., Haller D., Gerard P., Rohn S., Lepage P., et al. (2014). Intestinal microbiota in metabolic diseases: From bacterial community structure and functions to species of pathophysiological relevance. Gut Microbes 5 (4), 544–551. doi:10.4161/gmic.29331
Cronin O., Barton W., Moran C., Sheehan D., Whiston R., Nugent H., et al. (2019). Moderate-intensity aerobic and resistance exercise is safe and favorably influences body composition in patients with quiescent inflammatory bowel disease: A randomized controlled cross-over trial. BMC Gastroenterol. 19 (1), 29. doi:10.1186/s12876-019-0952-x
Cronin O., Barton W., Skuse P., Penney N. C., Garcia-Perez I., Murphy E. F., et al. (2018). A prospective metagenomic and metabolomic analysis of the impact of exercise and/or whey protein supplementation on the gut microbiome of sedentary adults. mSystems 3 (3), e00044-18. doi:10.1128/mSystems.00044-18
Curcio F., Liguori I., Cellulare M., Sasso G., Della-Morte D., Gargiulo G., et al. (2019). Physical activity Scale for the elderly (PASE) score is related to sarcopenia in noninstitutionalized older adults. J. Geriatr. Phys. Ther. 42 (3), 130–135. doi:10.1519/JPT.0000000000000139
Edgar R. C. (2010). Search and clustering orders of magnitude faster than BLAST. Bioinformatics 26 (19), 2460–2461. doi:10.1093/bioinformatics/btq461
Edgar R. C. (2013). UPARSE: highly accurate OTU sequences from microbial amplicon reads. Nat. Methods 10 (10), 996–998. doi:10.1038/nmeth.2604
Edgar R. C., Haas B. J., Clemente J. C., Quince C., Knight R. (2011). UCHIME improves sensitivity and speed of chimera detection. Bioinformatics 27 (16), 2194–2200. doi:10.1093/bioinformatics/btr381
Emilio E. J., Hita-Contreras F., Jimenez-Lara P. M., Latorre-Roman P., Martinez-Amat A. (2014). The association of flexibility, balance, and lumbar strength with balance ability: Risk of falls in older adults. J. Sports Sci. Med. 13 (2), 349–357.
Evans K., Abdelhafiz D., Abdelhafiz A. H. (2021). Sarcopenic obesity as a determinant of cardiovascular disease risk in older people: A systematic review. Postgrad. Med. 133 (8), 831–842. doi:10.1080/00325481.2021.1942934
Faith J. J., Guruge J. L., Charbonneau M., Subramanian S., Seedorf H., Goodman A. L., et al. (2013). The long-term stability of the human gut microbiota. Science 341 (6141), 1237439. doi:10.1126/science.1237439
Fiuza-Luces C., Santos-Lozano A., Joyner M., Carrera-Bastos P., Picazo O., Zugaza J. L., et al. (2018). Exercise benefits in cardiovascular disease: Beyond attenuation of traditional risk factors. Nat. Rev. Cardiol. 15 (12), 731–743. doi:10.1038/s41569-018-0065-1
Gao F., Gao E., Yue T. L., Ohlstein E. H., Lopez B. L., Christopher T. A., et al. (2002). Nitric oxide mediates the antiapoptotic effect of insulin in myocardial ischemia-reperfusion: The roles of PI3-kinase, akt, and endothelial nitric oxide synthase phosphorylation. Circulation 105 (12), 1497–1502. doi:10.1161/01.cir.0000012529.00367.0f
Gomes A. C., Hoffmann C., Mota J. F. (2019). Gut microbiota is associated with adiposity markers and probiotics may impact specific genera. Eur. J. Nutr. 59 (4), 1751–1762. doi:10.1007/s00394-019-02034-0
Hampton-Marcell J. T., Eshoo T. W., Cook M. D., Gilbert J. A., Horswill C. A., Poretsky R. (2020). Comparative analysis of gut microbiota following changes in training volume Among swimmers. Int. J. Sports Med. 41 (5), 292–299. doi:10.1055/a-1079-5450
Handley M. A., Lyles C. R., McCulloch C., Cattamanchi A. (2018). Selecting and improving quasi-experimental designs in effectiveness and implementation research. Annu. Rev. Public Health 39, 5–25. doi:10.1146/annurev-publhealth-040617-014128
Hawley J. A. (2020). Microbiota and muscle highway - two way traffic. Nat. Rev. Endocrinol. 16 (2), 71–72. doi:10.1038/s41574-019-0291-6
Ho S. S., Dhaliwal S. S., Hills A. P., Pal S. (2012). The effect of 12 weeks of aerobic, resistance or combination exercise training on cardiovascular risk factors in the overweight and obese in a randomized trial. BMC Public Health 12, 704. doi:10.1186/1471-2458-12-704
Holland A. M., Hyatt H. W., Smuder A. J., Sollanek K. J., Morton A. B., Roberts M. D., et al. (2015). Influence of endurance exercise training on antioxidant enzymes, tight junction proteins, and inflammatory markers in the rat ileum. BMC Res. Notes 8, 514. doi:10.1186/s13104-015-1500-6
Human Microbiome Project C. (2012). Structure, function and diversity of the healthy human microbiome. Nature 486 (7402), 207–214. doi:10.1038/nature11234
Jonduo M. E., Wawae L., Masiria G., Suda W., Hattori M., Takayasu L., et al. (2020). Gut microbiota composition in obese and non-obese adult relatives from the highlands of Papua New Guinea. FEMS Microbiol. Lett. 367 (19), fnaa161. doi:10.1093/femsle/fnaa161
Kelly T. N., Bazzano L. A., Ajami N. J., He H., Zhao J., Petrosino J. F., et al. (2016). Gut microbiome associates with lifetime cardiovascular disease risk profile Among bogalusa heart study participants. Circ. Res. 119 (8), 956–964. doi:10.1161/CIRCRESAHA.116.309219
Kemmler W., Von Stengel S., Engelke K., Kalender W. A. (2009). Exercise decreases the risk of metabolic syndrome in elderly females. Med. Sci. Sports Exerc. 41 (2), 297–305. doi:10.1249/MSS.0b013e31818844b7
Kim M. H., Yun K. E., Kim J., Park E., Chang Y., Ryu S., et al. (2020). Gut microbiota and metabolic health among overweight and obese individuals. Sci. Rep. 10 (1), 19417. doi:10.1038/s41598-020-76474-8
Kyu H. H., Abate D., Abate K. H., Abay S. M., Abbafati C., Abbasi N., et al. (2018). Global, regional, and national disability-adjusted life-years (DALYs) for 359 diseases and injuries and healthy life expectancy (HALE) for 195 countries and territories, 1990-2017: A systematic analysis for the global burden of disease study 2017. Lancet 392 (10159), 1859–1922. doi:10.1016/S0140-6736(18)32335-3
Lakka H. M., Laaksonen D. E., Lakka T. A., Niskanen L. K., Kumpusalo E., Tuomilehto J., et al. (2002). The metabolic syndrome and total and cardiovascular disease mortality in middle-aged men. JAMA 288 (21), 2709–2716. doi:10.1001/jama.288.21.2709
Lee D. C., Shook R. P., Drenowatz C., Blair S. N. (2016). Physical activity and sarcopenic obesity: Definition, assessment, prevalence and mechanism. Future Sci. OA 2 (3), FSO127. doi:10.4155/fsoa-2016-0028
Li H., Qin S., Liang Q., Xi Y., Bo W., Cai M., et al. (2021). Exercise training enhances myocardial mitophagy and improves cardiac function via irisin/FNDC5-PINK1/parkin pathway in MI mice. Biomedicines 9 (6), 701. doi:10.3390/biomedicines9060701
Lippert K., Kedenko L., Antonielli L., Kedenko I., Gemeier C., Leitner M., et al. (2017). Gut microbiota dysbiosis associated with glucose metabolism disorders and the metabolic syndrome in older adults. Benef. Microbes 8 (4), 545–556. doi:10.3920/BM2016.0184
Liu H., Zhang H., Wang X., Yu X., Hu C., Zhang X. (2018). The family Coriobacteriaceae is a potential contributor to the beneficial effects of Roux-en-Y gastric bypass on type 2 diabetes. Surg. Obes. Relat. Dis. 14 (5), 584–593. doi:10.1016/j.soard.2018.01.012
Louis P., Flint H. J. (2009). Diversity, metabolism and microbial ecology of butyrate-producing bacteria from the human large intestine. FEMS Microbiol. Lett. 294 (1), 1–8. doi:10.1111/j.1574-6968.2009.01514.x
Lu Y., Fan C., Li P., Lu Y., Chang X., Qi K. (2016). Short chain fatty acids prevent high-fat-diet-induced obesity in mice by regulating G protein-coupled receptors and gut microbiota. Sci. Rep. 6, 37589. doi:10.1038/srep37589
Magoc T., Salzberg S. L. (2011). FLASH: fast length adjustment of short reads to improve genome assemblies. Bioinformatics 27 (21), 2957–2963. doi:10.1093/bioinformatics/btr507
Mailing L. J., Allen J. M., Buford T. W., Fields C. J., Woods J. A. (2019). Exercise and the gut microbiome: A review of the evidence, potential mechanisms, and implications for human health. Exerc. Sport Sci. Rev. 47 (2), 75–85. doi:10.1249/JES.0000000000000183
Matsubara Y., Matsumoto T., Inoue K., Matsuda D., Yoshiga R., Yoshiya K., et al. (2017). Sarcopenia is a risk factor for cardiovascular events experienced by patients with critical limb ischemia. J. Vasc. Surg. 65 (5), 1390–1397. doi:10.1016/j.jvs.2016.09.030
McFarlane D., Wolf R. F., McDaniel K. A., White G. L. (2012). The effect of season on inflammatory response in captive baboons. J. Med. Primatol. 41, 341–348. doi:10.1111/j.1600-0684.2012.00560.x
Metabolic Syndrome Research Group of Chinese Medical Association (2004). Suggestions on metabolic syndrome from Chinese diabetes society. Chin. J. Diabetes 12 (3), 156–161. doi:10.3321/j.issn:1006-6187.2004.03.002
Miao Z., Lin J. S., Mao Y., Chen G. D., Zeng F. F., Dong H. L., et al. (2020). Erythrocyte n-6 polyunsaturated fatty acids, gut microbiota, and incident type 2 diabetes: A prospective cohort study. Diabetes Care 43 (10), 2435–2443. doi:10.2337/dc20-0631
Morrison D. J., Preston T. (2016). Formation of short chain fatty acids by the gut microbiota and their impact on human metabolism. Gut Microbes 7 (3), 189–200. doi:10.1080/19490976.2015.1134082
Munukka E., Ahtiainen J. P., Puigbó P., Jalkanen S., Pahkala K., Keskitalo A., et al. (2018). Six-week endurance exercise alters gut metagenome that is not reflected in systemic metabolism in over-weight women. Front. Microbiol. 9, 2323. doi:10.3389/fmicb.2018.02323
Ohara M., Kohara K., Tabara Y., Igase M., Miki T. (2015). Portable indices for sarcopenia are associated with pressure wave reflection and central pulse pressure: the J-SHIPP study. J. Hypertens 33 (2), 314–322. doi:10.1097/HJH.0000000000000394
Ohara M., Kohara K., Tabara Y., Ochi M., Nagai T., Igase M., et al. (2014). Sarcopenic obesity and arterial stiffness, pressure wave reflection and central pulse pressure: the J-SHIPP study. Int. J. Cardiol. 174 (1), 214–217. doi:10.1016/j.ijcard.2014.03.194
Onyszkiewicz M., Gawrys-Kopczynska M., Konopelski P., Aleksandrowicz M., Sawicka A., Kozniewska E., et al. (2019). Butyric acid, a gut bacteria metabolite, lowers arterial blood pressure via colon-vagus nerve signaling and GPR41/43 receptors. Pflugers Arch. 471 (11-12), 1441–1453. doi:10.1007/s00424-019-02322-y
Podsiadlo D., Richardson S. (1991). The timed “Up & Go”: a test of basic functional mobility for frail elderly persons. J. Am. Geriatr. Soc. 39 (2), 142–148. doi:10.1111/j.1532-5415.1991.tb01616.x
Poll B. G., Cheema M. U., Pluznick J. L. (2020). Gut microbial metabolites and blood pressure regulation: Focus on SCFAs and TMAO. Physiol. (Bethesda) 35 (4), 275–284. doi:10.1152/physiol.00004.2020
Quiroga R., Nistal E., Estébanez B., Porras D., Juárez-Fernández M., Martínez-Flórez S., et al. (2020). Exercise training modulates the gut microbiota profile and impairs inflammatory signaling pathways in obese children. Exp. Mol. Med. 52 (7), 1048–1061. doi:10.1038/s12276-020-0459-0
Ramakrishnan R., Doherty A., Smith-Byrne K., Rahimi K., Bennett D., Woodward M., et al. (2021). Accelerometer measured physical activity and the incidence of cardiovascular disease: Evidence from the UK Biobank cohort study. PLoS Med. 18 (1), e1003487. doi:10.1371/journal.pmed.1003487
Rikli R. E., Jones C. J. (1999). Development and validation of a functional fitness test for community-residing older adults. J. Aging Phys. Act. 7 (2), 129–161. doi:10.1123/japa.7.2.129
Roberts C. K., Croymans D. M., Aziz N., Butch A. W., Lee C. C. (2013). Resistance training increases SHBG in overweight/obese, young men. Metabolism. 62 (5), 725–733. doi:10.1016/j.metabol.2012.12.004
Rubenstein L. Z. (2006). Falls in older people: Epidemiology, risk factors and strategies for prevention. Age Ageing 35, ii37–ii41. doi:10.1093/ageing/afl084
Sahuri-Arisoylu M., Brody L. P., Parkinson J. R., Parkes H., Navaratnam N., Miller A. D., et al. (2016). Reprogramming of hepatic fat accumulation and 'browning' of adipose tissue by the short-chain fatty acid acetate. Int. J. Obes. 40 (6), 955–963. doi:10.1038/ijo.2016.23
Schroeder E. C., Franke W. D., Sharp R. L., Lee D. C. (2019). Comparative effectiveness of aerobic, resistance, and combined training on cardiovascular disease risk factors: A randomized controlled trial. PLoS One 14 (1), e0210292. doi:10.1371/journal.pone.0210292
Scott K. P., Gratz S. W., Sheridan P. O., Flint H. J., Duncan S. H. (2013). The influence of diet on the gut microbiota. Pharmacol. Res. 69 (1), 52–60. doi:10.1016/j.phrs.2012.10.020
Sommer F., Anderson J. M., Bharti R., Raes J., Rosenstiel P. (2017). The resilience of the intestinal microbiota influences health and disease. Nat. Rev. Microbiol. 15 (10), 630–638. doi:10.1038/nrmicro.2017.58
Son W.-M., Park J.-J. (2021). Resistance band exercise training prevents the progression of metabolic syndrome in obese postmenopausal women. J. Sports Sci. Med. 20, 291–299. doi:10.52082/jssm.2021.291
Song B. K., Cho K. O., Jo Y., Oh J. W., Kim Y. S. (2012). Colon transit time according to physical activity level in adults. J. Neurogastroenterol. Motil. 18 (1), 64–69. doi:10.5056/jnm.2012.18.1.64
Stewart N., Taylor B., Ponsonby A. L., Pittas F., van der Mei I., Woods G., et al. (2007). The effect of season on cytokine expression in multiple sclerosis and healthy subjects. J. Neuroimmunol. 188 (1-2), 181–186. doi:10.1016/j.jneuroim.2007.06.012
Taniguchi H., Tanisawa K., Sun X., Kubo T., Hoshino Y., Hosokawa M., et al. (2018). Effects of short-term endurance exercise on gut microbiota in elderly men. Physiol. Rep. 6 (23), e13935. doi:10.14814/phy2.13935
Thompson P. D., Buchner D., Pina I. L., Balady G. J., Williams M. A., Marcus B. H., et al. (2003). Exercise and physical activity in the prevention and treatment of atherosclerotic cardiovascular disease: A statement from the council on clinical cardiology (subcommittee on exercise, rehabilitation, and prevention) and the council on nutrition, physical activity, and metabolism (subcommittee on physical activity). Circulation 107 (24), 3109–3116. doi:10.1161/01.CIR.0000075572.40158.77
Utzschneider K. M., Kratz M., Damman C. J., Hullar M. (2016). Mechanisms linking the gut microbiome and glucose metabolism. J. Clin. Endocrinol. Metab. 101 (4), 1445–1454. doi:10.1210/jc.2015-4251
Villareal D. T., Aguirre L., Gurney A. B., Waters D. L., Sinacore D. R., Colombo E., et al. (2017). Aerobic or resistance exercise, or both, in dieting obese older adults. N. Engl. J. Med. 376 (20), 1943–1955. doi:10.1056/NEJMoa1616338
Wang D., Yu S., Zou Y., Li H., Cheng X., Qiu L., et al. (2020). Data mining: Seasonal fluctuations and associations between thyroid stimulating hormone and lipid profiles. Clin. Chim. Acta. 506, 122–128. doi:10.1016/j.cca.2020.03.012
Wang Y., Liu J., Wang W., Wang M., Qi Y., Xie W., et al. (2015). Lifetime risk for cardiovascular disease in a Chinese population: The Chinese multi-provincial cohort study. Eur. J. Prev. Cardiol. 22 (3), 380–388. doi:10.1177/2047487313516563
Wilson P. W., D'Agostino R. B., Parise H., Sullivan L., Meigs J. B. (2005). Metabolic syndrome as a precursor of cardiovascular disease and type 2 diabetes mellitus. Circulation 112 (20), 3066–3072. doi:10.1161/CIRCULATIONAHA.105.539528
Witkowski M., Weeks T. L., Hazen S. L. (2020). Gut microbiota and cardiovascular disease. Circ. Res. 127 (4), 553–570. doi:10.1161/CIRCRESAHA.120.316242
Yusuf S., Joseph P., Rangarajan S., Islam S., Mente A., Hystad P., et al. (2020). Modifiable risk factors, cardiovascular disease, and mortality in 155 722 individuals from 21 high-income, middle-income, and low-income countries (PURE): A prospective cohort study. Lancet 395 (10226), 795–808. doi:10.1016/S0140-6736(19)32008-2
Keywords: physical function, sarcopenic obesity, metabolic syndrome, gut microbiota, elderly adults, randomized control trial
Citation: Zhong F, Xu Y, Lai H-Y, Yang M, Cheng L, Liu X, Sun X, Yang Y, Wang J, Lv W and Huang C (2022) Effects of combined aerobic and resistance training on gut microbiota and cardiovascular risk factors in physically active elderly women: A randomized controlled trial. Front. Physiol. 13:1004863. doi: 10.3389/fphys.2022.1004863
Received: 27 July 2022; Accepted: 28 September 2022;
Published: 20 October 2022.
Edited by:
Vassilis Mougios, Aristotle University of Thessaloniki, GreeceReviewed by:
Ismael Martínez Guardado, Nebrija University, SpainCopyright © 2022 Zhong, Xu, Lai, Yang, Cheng, Liu, Sun, Yang, Wang, Lv and Huang. This is an open-access article distributed under the terms of the Creative Commons Attribution License (CC BY). The use, distribution or reproduction in other forums is permitted, provided the original author(s) and the copyright owner(s) are credited and that the original publication in this journal is cited, in accordance with accepted academic practice. No use, distribution or reproduction is permitted which does not comply with these terms.
*Correspondence: Cong Huang, Y29odWFuZ0B6anUuZWR1LmNu
Disclaimer: All claims expressed in this article are solely those of the authors and do not necessarily represent those of their affiliated organizations, or those of the publisher, the editors and the reviewers. Any product that may be evaluated in this article or claim that may be made by its manufacturer is not guaranteed or endorsed by the publisher.
Research integrity at Frontiers
Learn more about the work of our research integrity team to safeguard the quality of each article we publish.