- 1Department of Veterinary and Biomedical Sciences, College of Veterinary Medicine, University of Minnesota, Saint Paul, MN, United States
- 2University of Minnesota Informatics Institute, University of Minnesota, Minneapolis, MN, United States
- 3Department of Animal Sciences, The Ohio State University, Ohio Agricultural Research and Development Center, Wooster, OH, United States
- 4Department of Food Science and Human Nutrition, Michigan State University, East Lansing, MI, United States
Precise regulation of gene expression is critical for normal muscle growth and development. Changes in gene expression patterns caused by external stressors such as temperature can have dramatic effects including altered cellular structure and function. Understanding the cellular mechanisms that underlie muscle growth and development and how these are altered by external stressors are crucial in maintaining and improving meat quality. This study investigated circular RNAs (circRNAs) as an emerging aspect of gene regulation. We used data mining to identify circRNAs and characterize their expression profiles within RNAseq data collected from thermally challenged turkey poults of the RBC2 and F-lines. From sequences of 28 paired-end libraries, 8924 unique circRNAs were predicted of which 1629 were common to all treatment groups. Expression analysis identified significant differentially expressed circRNAs (DECs) in comparisons between thermal treatments (41 DECs) and between genetic lines (117 DECs). No intersection was observed between the DECs and differentially expressed gene transcripts indicating that the DECs are not simply the result of expression changes in the parental genes. Comparative analyses based on the chicken microRNA (miRNA) database suggest potential interactions between turkey circRNAs and miRNAs. Additional studies are needed to reveal the functional significance of the predicted circRNAs and their role in muscle development in response to thermal challenge. The DECs identified in this study provide an important framework for future investigation.
Introduction
Efficient production of animal protein for human consumption is an important component of agriculture as animal protein = muscle = meat. Although various approaches have been investigated to increase production efficiency (Abo Ghanima et al., 2020; Alagawany et al., 2021), genetic selection for growth performance and the production of heavier market weight birds have increased the incidence of muscle diseases (myopathies) that cause significant losses to the industry (Mudalal et al., 2015; Velleman, 2015). For example, conditions such as White Striping and Woody Breast are becoming more common in the broiler industry (Petracci et al., 2013) and conditions such as pale, soft, exudative (PSE meat) are a concern for the turkey industry (Owens et al., 2000; Petracci et al., 2009; Zampiga et al., 2020). The genetic predisposition and etiology of these diseases are still poorly understood, although there is evidence of dysregulated lipid and glucose metabolism (Lake and Abasht, 2020).
Previous and ongoing studies are aimed at better understanding the effects that external factors, such as growth selection and temperature extremes have on gene expression and subsequent muscle development in poultry. Prolonged exposure to temperature extremes has detrimental effects on meat quality, including increased fat deposition, localized muscle fiber disorganization, and compromised protein functionality. For example, in studies of cultured turkey breast muscle satellite cells (SCs), exposure to thermal stress altered expression of adipogenic genes and increased lipid deposition (Clark et al., 2016; Clark and Velleman, 2016), suggesting potential conversion of satellite cells to an adipogenic lineage (Clark et al., 2017). In vitro study of proliferating and differentiating SCs from growth-selected lines compared to controls demonstrated a significant alteration in gene expression as a result of thermal challenge (Reed et al., 2017a, b). Expression of adipogenic genes by cultured SCs from commercial turkeys is more responsive to thermal challenge during proliferation than during differentiation (Xu et al., 2021a, b). Likewise, SCs from growth-selected turkeys are more sensitive to thermal stress compared to non-selected birds.
An in vivo study of newly hatched poults found the breast muscle of thermally challenged growth-selected birds responded through changes in gene expression predicted to have downstream transcriptional effects resulting in reduced muscle growth (Barnes et al., 2019). Non-selected birds responded through modulation of lipid-related genes, suggesting a reduction in lipid storage, transport and synthesis, consistent with changes in energy metabolism. In order to mitigate the incidence of myopathies and thereby improve meat quality and quantity, we need to better understand the cellular mechanisms that underlie muscle growth and development and how these are altered.
An emerging area in the study of gene regulation is circular RNAs (circRNAs). CircRNAs are novel, naturally occurring, single-stranded RNAs that are generated from exonic/intronic sequences joined head to tail and are widely expressed in eukaryotes (Wang et al., 2014; Patop et al., 2019). They lack polyA tails, function independently of ribosomes and rRNA, are resistant to RNA exonuclease (RNase R), and persist in the cell longer than mRNAs. Several mechanisms have been proposed for their generation (Qu et al., 2015), but they are identifiable in RNAseq data as back-spliced reads. Once thought to represent errors in RNA splicing, the abundance of circRNAs has been unappreciated in conventional bioinformatic analysis of genome-wide sequence data, but examination of this data with new algorithms has found circRNAs to be widely expressed (Liang et al., 2017; Xu et al., 2017). Although their functions are still poorly understood and mostly unknown, these RNAs appear to act as modifiers of gene expression by regulating transcription, RNA splicing, and translation by acting as microRNA (miRNA) sinks (Wilusz, 2018; Zhang et al., 2018; Kristensen et al., 2019). The closed loop structure of circRNAs makes them resistant to degradation by RNA exonuclease and thus more stable than linear RNAs. Their stability and abundance, especially in some body fluids, make them potential biomarkers (Zhang et al., 2018).
Based on sequence and expression analysis, circRNAs appear to be moderately conserved and expressed in a tissue and development-specific manner. Most are expressed at low levels, but some are expressed at levels higher than their linear RNA counterparts (Wilusz, 2018). Recent studies in several species, including some of agricultural importance, suggest that circRNAs modulate gene expression during myogenesis (Das et al., 2020) and play important roles in cell proliferation, differentiation, autophagy, and apoptosis (Kulcheski et al., 2016; Liang et al., 2017; Panda et al., 2017; Cao et al., 2018). For example, circRNAs are abundant and differentially expressed during chicken embryonic muscle development (Ouyang et al., 2018b), interact with innate response genes (Li et al., 2015; Zhao et al., 2015; Samir et al., 2016), and promote resistance to highly pathogenic J-strain avian leucosis virus (Zhang et al., 2017). Regarding muscle development, Ouyang et al. (2018a) identified a circRNA in the chicken supervillin gene (circSVIL) that could function as a sponge for miR-203, upregulating expression of c-JUN and MEF2C to promote the proliferation and differentiation of primordial muscle cells (myoblasts), a crucial process in muscle development. The objective of this study was to use a data-mining approach to characterize circRNA expression profiles within turkey skeletal muscle. This methodology was successfully used to characterize circRNAs in RNAseq data from humans and other model organisms (Memczak et al., 2013; Xu et al., 2017). Here, we used RNAseq data previously collected from a thermal challenge study of newly hatched turkey poults (Barnes et al., 2019). This first study of turkey skeletal muscle, identified 8924 unique circRNAs and significant differential expression was found in comparisons among thermal treatments and genetic lines.
Materials and Methods
circRNA Prediction and Expression Analysis
We used the original RNAseq sequence data of Barnes et al. (2019) for data mining (SRA BioProject PRJNA419215). In the challenge experiment, breast muscle tissues were harvested from hatchlings of two closed population lines (F and RBC2) exposed 3 days to reduced (31°C), elevated (39°C) or control temperature (35°C). The F-line was selected from the Randombred Control Line 2 (RBC2) only for 16-wk body weight and is faster growing (Nestor, 1977, 1984). The Randombred Control Line 2 (RBC2) represents a commercial bird from the late 1960s and has been maintained at The Ohio State University, Poultry Research Center (Wooster, OH) without conscious selection for any trait. Sequence data (RNAseq reads from 28 libraries, ∼18 million reads per library) was trimmed (Trimmomatic, Bolger et al., 2014) and quality-filtered (FastQC, Andrews, 2010). The resulting reads were mapped to the turkey genome (UMD5.1) using BWA-MEM (Li and Durbin, 2009). Circular RNAs were predicted using the CIRI software package (CIRI2, Gao et al., 2015), a multi-scan pipeline. The closest annotated gene to each predicted circRNA was obtained with BEDtools – closest option (Quinlan and Hall, 2010). Read counts for each circRNA were used for differential expression analysis using DESeq2 (CLC Genomics Workbench, v11.0.1).
Confirmation of circRNAs
Confirmation of a set of predicted circRNAs was performed by PCR amplification and Sanger sequencing. For the selected circRNAs, flanking genome sequence was captured surrounding the splice site as indicated in CIRI. Oligonucleotide primer sets were designed for each circRNA using NIH-NCBI Primer-BLAST to target amplification of the circRNA junctions. The RNA samples were the same as originally used for RNAseq project (SRA BioProject PRJNA419215). Samples were first treated with RNase R that digests all linear RNA molecules except lariat or circular RNA structures. This depleted RNA was generated from 2 μg of total RNA using 5 units of RNase R exoribonuclease (Lucigen, Corp.) following manufacturer’s protocol (incubation reaction at 37°C for 20 min followed by 65°C for 20 min). Reverse transcription was performed with the Superscript IV kit (Invitrogen), 1 μg of RNase R depleted RNA and random hexamer priming as per manufacturer’s protocol (23°C for 10 min, 50°C for 10 min, followed by 80°C for 10 min). Aliquots of the resulting cDNA products were pooled as templates for PCR.
Amplification by PCR from the pool of RNase R depleted cDNA samples was conducted on 28 junction-flanking targets. PCR was performed using the Platinum Taq II system (Invitrogen) with 1 μl cDNA pool, and 0.4 μM each primer following manufacturer’s protocol. Thermal cycling conditions were as follows: 94°C for 2 min, then 30 cycles of 94°C for 15 s, 58°C for 15 s, 68°C for 30 s, followed by 10 min 72°C incubation. Products were resolved using 2% agarose electrophoresis and single products were selected for DNA sequencing. For sequencing, PCR products were purified using ExoSap-IT (Applied Biosciences) according to manufacturer’s protocol. Sanger sequencing was performed with both forward and reverse primers at the University of Minnesota Genomics Center core facility.
Functional Prediction
Gene ontology (GO) overrepresentation tests were conducted with Panther v16.0 (Mi et al., 2021). Functional annotation clustering of the parental genes was performed with DAVID (Huang et al., 2009). Predicted circRNAs were scanned for miRNA binding/interaction sites through adapting application of miRDB (Liu and Wang, 2019).
Results and Discussion
circRNA Prediction
Sequence reads from the 28 paired-end libraries averaged 18.7 Million reads/library (Barnes et al., 2019). The software CIRI detects junction reads denoting circRNA candidates by differentiating and calculating the percentage of back-splice junction reads from non-junction reads. Detection of circRNAs is dependent on sequence depth with some commercial sequencing services recommending > 40M reads per sample. Analysis of the mapped reads and relative counts of back-splice junction and non-junction reads with CIRI2 predicted a total of 8924 unique circRNAs among the 28 libraries (Supplementary Table 1). On average, 3735.5 circRNAs were predicted per library and 2368.5 were shared between libraries within treatment groups (Table 1). Of the 8924 unique circRNAs, 1629 were shared across all 28 libraries.
Genomic features including chromosome distribution, length and circRNA type were investigated. As expected the predicted circRNAs were distributed throughout the turkey genome and their frequency significantly corresponded to chromosome size (p-value < 0.00001, Figure 1A). Based on the position of the back-spliced reads in the genome, the predicted length of the circRNAs ranged from 134 bp to just under 200 kB (average 36.2 kB, Figure 1B). Using the current genome annotation (v102), the 8,924 predicted circRNAs were classified by CIRI2 as exonic (6.5%, average length 4,948 nt) or intronic (5.1%, average 23,543 nt). In addition, 88.4% fell outside of annotated genes and were designated as intergenic (average 39,289 nt). A common convention is to name circRNAs either in reference to their parental gene or identified function. Here we used the numbers assigned in the CIRI2 output to sequentially number the circRNAs along each chromosome progressing through the genome sequence (Supplementary Table 1).
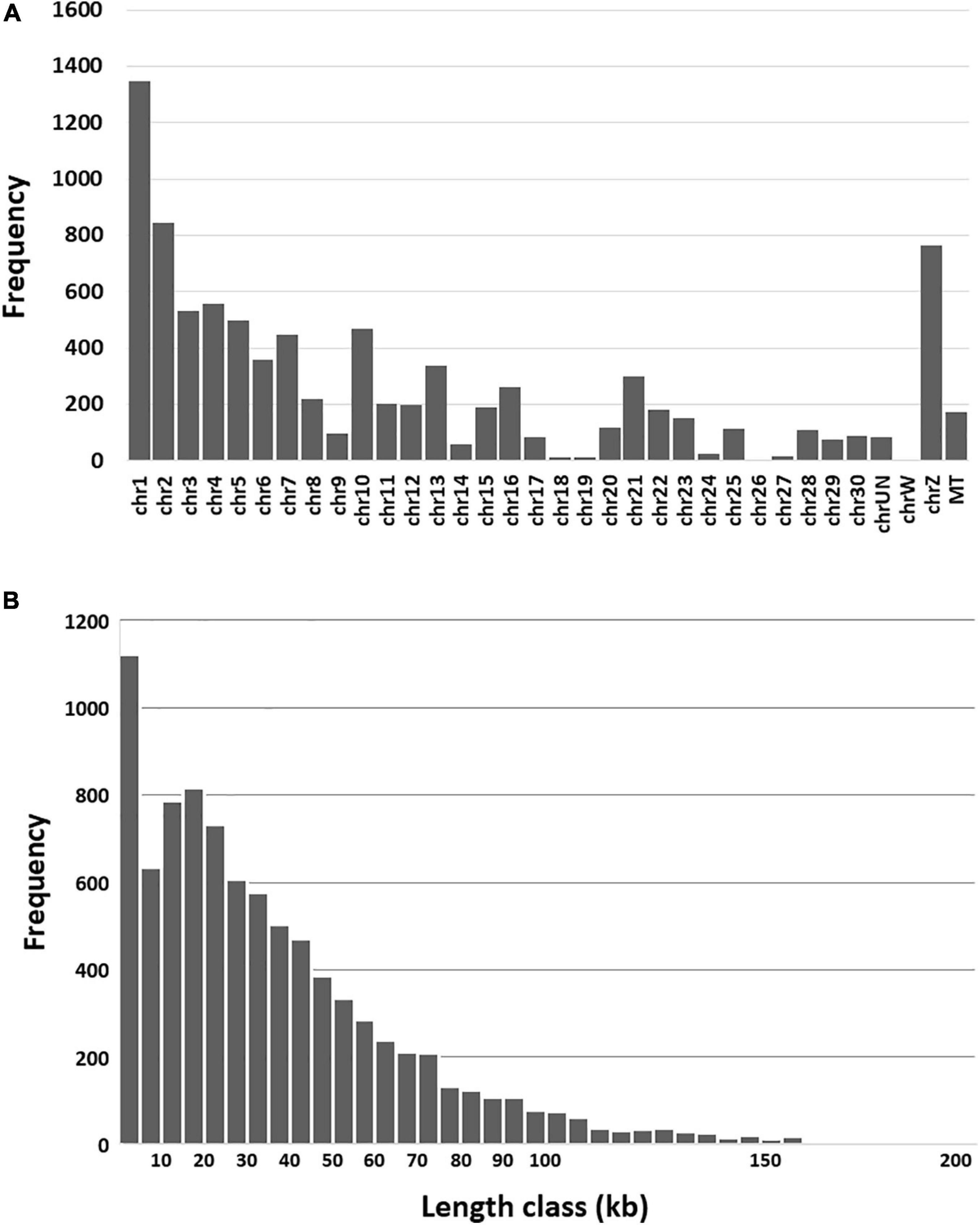
Figure 1. (A) Frequency of predicted circRNAs by chromosome. (B) Frequency of circRNAs by length class. Each vertical bar represents a window of 5 kb in length.
The genome position is the defining circRNA feature since circRNA predictions are experiment-based and parental gene of origin may not be unique. For example, within the exonic class of circRNAs, 20 had 2 separate parental genes (Supplementary Table 1). Of these, 15 occurred where annotation of the two identified loci overlapped in the turkey genome. For the remaining 5 (circ0481, circ3782, circ4150, circ6709, and circ7308), the 2 genes were adjacent in the genome suggesting possible origin as chimeric RNA. Similarly, 20 “multigene” circRNAs were found in the intron class with 14 involving overlapping loci and 4 adjacent loci.
The 8924 circRNAs only mapped to a total of 4,513 parental or “closest” genes and a number of circRNAs had common parental genes. This is not uncommon in that some protein-coding genes generate multiple circRNAs through alternative circularization (Wilusz, 2018). Striking examples in our dataset include Myosin-7-like (LOC104913726) that had 35 associated circRNAs (circ6724-6758), and Thrombospondin 4 (THBS4) that had 33 (circ8115-8147). In the case of these multi-circRNA genes, individual circRNAs typically had different 3′ acceptor sites but common 5′ donors. Notable muscle genes with multiple circRNAs included the troponin genes (TNNC2, TNNI2, TNNT2, and TNNT3) which had 17, 14, 5, and 10 assigned circRNAs, respectively (Supplementary Table 1). The formation of circRNAs may be coupled to exon skipping events raising the potential that alternatively spliced genes may generate more circRNAs.
Functional annotation clustering of the parental genes found the cluster with the highest enrichment score (5.60) included genes in the GO categories Bioprocess (GO:0006412, Translation, Fold Enrichment = 2.52, FDR p-value = 1.40E-03), Molecular Function (GO:0003735, Structural constituent of ribosome, Fold Enrichment = 2.37, FDR p-value = 1.56E-03) and Cellular Component (GO:0022625, Cytosolic large ribosomal subunit, Fold Enrichment = 3.30, FDR p-value = 6.32E-03).
Differential circRNA Expression
Expression of the predicted circRNAs was summarized for each of the six treatment groups of Barnes et al. (2019). These included the slower-growing RBC2 line (cold and heat treatments), the faster growing F-line (cold and heat treatments) and the F and RBC2 controls. Differentially expressed circRNAs (DECs) were classified by significance (FDR p-value) and observed fold change (| Log2FC| > 1.0 or > 2.0, Table 2).
Temperature Effects
Temperature effects on circRNAs were examined in 4 pairwise, within-line comparisons: CS = cold-brooded vs. control-brooded (31 vs. 35°C) slower growing poults (RBC2), HS = heat- vs. control-brooded (39 vs. 35°C) slower-growing poults (RBC2); CF = cold- vs. control-brooded (31 vs. 35°C) faster-growing poults (F-line); and HF = heat- vs. control-brooded (39 vs. 35°C) faster-growing poults (F-line). A total of 41 DECs that met the criteria of having FDR p-value < 0.05 and | Log2FC| > 2.0 was identified. The response of circRNAs in the breast muscle of turkey poults to thermal challenge was similar to the response in gene expression in that a greater number of circRNAs were differentially affected by heat than cold (Barnes et al., 2019). Four differentially expressed DECs were identified in the CS comparison (Tables 2, 3 and Figure 2A). Included were two significantly up regulated (circ3577 and circ7597) and two (circ6722 and circ3428) down regulated by the cold treatment. Three of the four were intergenic circRNAs with an average predicted length of 12,442 nt, and the fourth was exonic and 283 nt (Supplementary Table 1). Each of the associated genes are involved in muscle function and/or structure. Only a single DEC (circ5707, 1,445 nt) was identified in the CF comparison. This DEC was located in the intergenic region of tropomyosin 1 (alpha) and was up regulated by cold treatment. None of the DECs were in common between the two cold treatment comparisons (CS and CF). The greatest number of DECs was observed for the HS comparison (Table 3). These 35 DECs included 4 that were up regulated and 31 down regulated by the heat treatment. The majority (27) were classified as intergenic (average 27,961 nt) with 7 being intronic (average 18,852 nt) and 1 exonic (13,384 nt). One of the down regulated DECs (circ6722) was also similarly down regulated in the CS comparison. Lastly, 3 DECs were identified in the HF comparison, all of which were intergenic. The single up regulated DEC (circ5707) was also up regulated in the CF comparison.
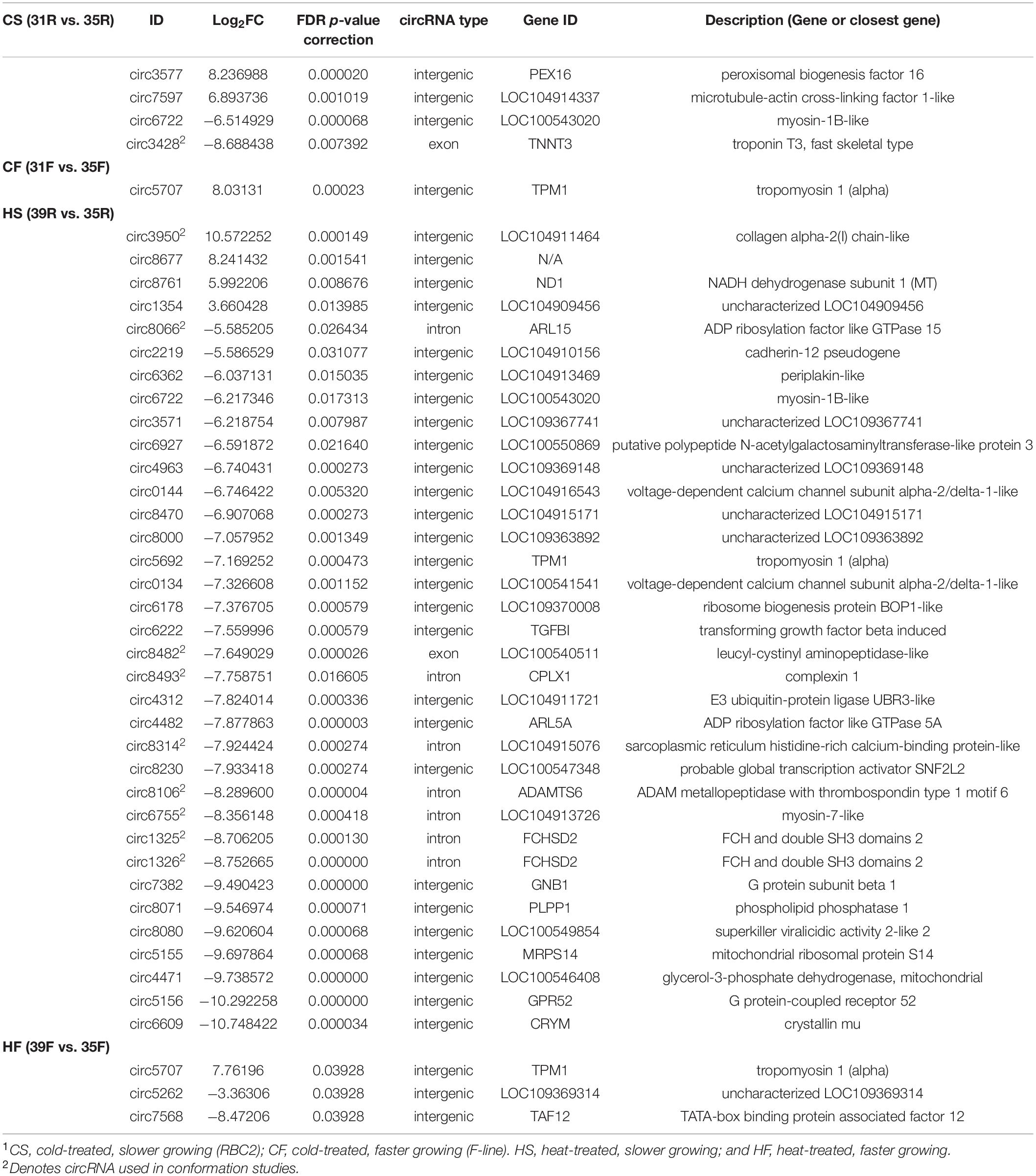
Table 3. Significant differentially expressed circRNAs (FDR P < 0.05 and | Log2FC| > 2.0) in within-line treatment comparisons (see Figure 2A)1.
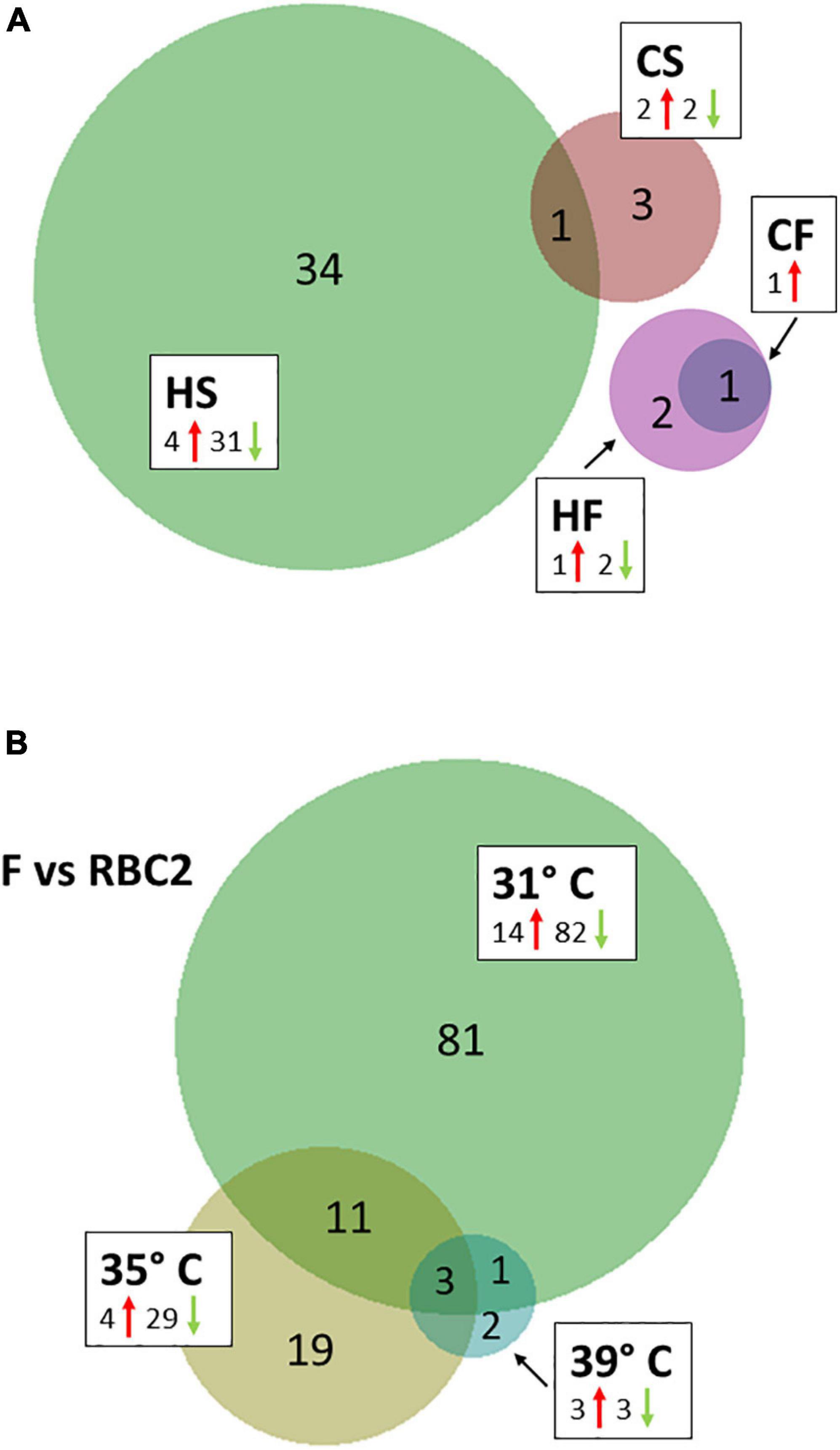
Figure 2. (A) Venn diagram of significant differentially expressed circRNAs (DEC) shared between temperature comparisons. HS, heat-treated slower growing (RBC2); CS, cold-treated slower growing; HF, heat-treated, faster growing (F-line); and CF, cold-treated, faster growing. (B) Distribution of significant differentially expressed circRNAs (DECs) in between-line comparisons.
Line Effects
We also tested for interaction between brooding temperature and line effects by contrasting circRNA expression between the lines at each brooding temperature. For each comparison, the RBC2 line served as a control in contrast to the comparatively faster growing F-line. A total of 117 DECs was identified (FDR p-value < 0.05 and | Log2FC| > 2.0). At the control temperature (35°C) 33 DECs were observed between the two lines (Table 4 and Figure 2B). The majority (29) showed higher expression in the RBC2 group (down regulated in the F-line). Consistent with other comparisons, most of DECs were classified as intergenic with 5 intronic and 2 exonic. In the heat-treatment comparison, only 6 DECs were observed with 3 up regulated and 3 down regulated. The three down regulated DECs (circ3159, circ5016, and circ6976) were also significant and similarly differentially expressed in the control temperature comparison. These circRNAs and the up regulated circ3421 were also significant in the cold-treatment comparison. The greatest number of DECs was observed for the cold-treatment comparison where 96 circRNAs were differentially expressed. Again, the majority (82) were down regulated in the 16-wk bodyweight-selected F-line. Fourteen were shared with the control temp (35°C) and 4 with the heat-treated group (39°C). Differences in the circRNAs identified between the F and RBC2 lines can be attributed to past selection for 16 wk body weight and not genetic background differences as the F-line was derived from the RBC2 line (Nestor, 1977).
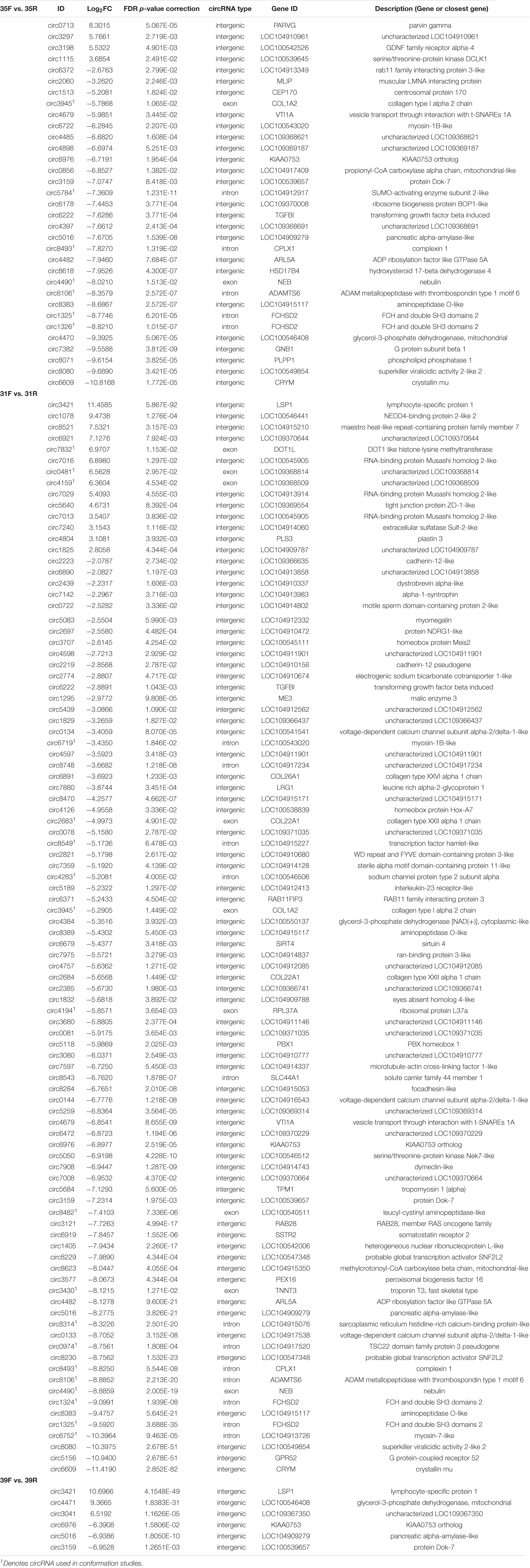
Table 4. Significant differentially expressed circRNAs (FDR P < 0.05 and | Log2FC| > 2.0) in within-temperature, between-line comparisons (see Figure 2B).
Given the larger number of DECs identified in the between-line comparisons of turkey poults we performed overrepresentation tests (Panther v16.0, Mi et al., 2021) to test for functional clustering of the parental genes. In the control temperature (35°C) group comparison, analysis of the 33 DECs found significant overrepresentation for GO categories of extracellular matrix (GO:0031012) and extracellular matrix organization (GO:0030198) (Table 5). Similarly, analysis of the 96 DECs in the cold temperature (31°C) comparison, also found significant overrepresentation for extracellular matrix categories (GO:0030198 and GO:0031012) but also actin cytoskeleton and cellular component organization and the cellular component (supramolecular fiber, GO:0099512). This finding contrasts results from the transcriptome where the slower growing RBC2 birds responded to thermal stress primarily with changes in lipid-related genes (Barnes et al., 2019). Muscle of F-line hatchlings responded to thermal stress through changes in genes involved in ubiquitination and modulators of gene expression, with a predicted reduction in muscle growth. Interaction networks among the transcribed RNAs (mRNA, miRNA, circRNA and other non-coding RNAs) and the response of these networks to physiologic stressors merit further investigation.
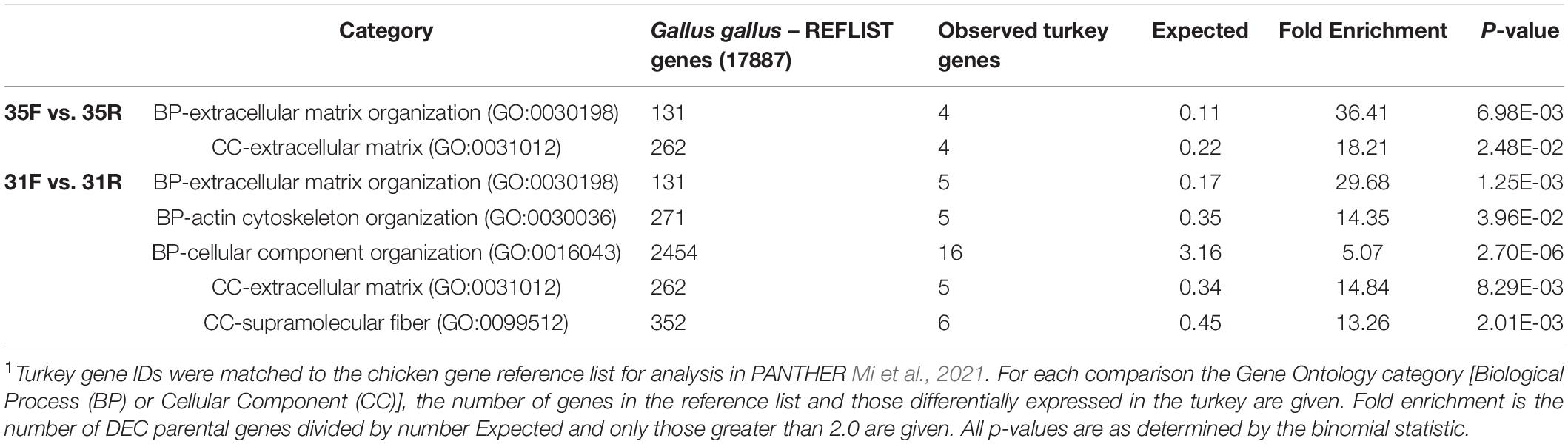
Table 5. Summary of PANTHER Overrepresentation Test of the parental genes of differentially expressed circRNAs (DECs) in between-line comparisons of turkey poults after heat exposure1.
Finally, we compared the DECs from both temperature and line comparisons to the significant differentially expressed genes (DEGs) identified for the same treatment comparisons in the original RNAseq study (Barnes et al., 2019). No intersection was observed between the DEC gene IDs and the DEGs (| Log2FC| > 2.0). We also compared the directionality of expression differences for DECs in the exonic and intronic classes (clearly defined parental genes) with the parental gene transcript expression. In most cases, expression of the parental gene transcripts were essentially invariant (| Log2FC| < 0.5) and in many of the treatment comparisons, the directionality of change was opposite that observed for the DECs. Only a single parental gene (LOC100540511, leucyl-cystinyl aminopeptidase-like) in the 31F vs. 31R comparison showed significant expression changes in both the circRNA (circ8482, Log2FC = −7.41, FDR p-value = 7.34E-06) and parental gene transcript (Log2FC = −1.25, FDR p-value = 0.037). This indicates that the differences in circRNA expression are not just a function of expression changes in the parental genes.
Confirmation of circRNAs
Although we identified thousands of putative circRNAs, confirmation of these predictions with orthogonal techniques is necessary prior to further functional interpretation. As circRNAs are computationally predicted on an experiment-level basis, performing this for all predicted circRNAs would be daunting. In this respect, differential expression analysis can help focus and guide these efforts toward the set of circRNAs that are impacted by the treatments of interest. We selected 28 circRNAs for confirmation by a PCR-based approach designed to specifically target the splice junction. The circRNAs for analysis were selected to included representatives from the exonic and intronic classes (Supplementary Table 2), varied ranges in predicted length (143 to 49,826 nt), with the majority being differentially expressed in the experimental comparisons. Primers were designed to produce fragments of approximately 250 bp with an average of 125 bp of 5′ and 3′ flanking sequence. Using pooled cDNA synthesized from RNase R depleted RNA, 23 of 28 predicted circRNA junctions were amplified by PCR. Of the 23 amplified products, 14 cleanly sequenced through and confirmed the predicted back-spliced junction. The remaining 9 either produced very faint PCR products or multiple bands that could not be directly sequenced (Supplementary Table 2). This reiterates the necessity for orthogonal confirmation. The 5 circRNA junctions that did not amplify could represent linear RNAs present in the original RNA samples but not resistant to RNase R (false positives). Ideally, characterization of circRNAs should include independent sequencing of libraries created from depleted RNA (RNase R digested) to help eliminate false positives and identify minor classes of circRNAs with low expression. This work is ongoing in our laboratory.
Functional Analysis of circRNAs
The function of individual circRNAs appears to be diverse (Zhang et al., 2018). Some have been shown to function as miRNA sponges or through binding endogenous competing RNAs, whereas others interact with RNA binding proteins and mRNAs to regulate alternative splicing and/or transcription (Huang et al., 2020). Some circRNAs remain in the nucleus interacting with Pol II machinery to regulate expression of their parental genes while others may have a role in protein translation including the potential for coding proteins (Abe et al., 2015; Bose and Ain, 2018; Lei et al., 2020). Unlike differentially expressed gene transcripts (DEGs) identified in traditional RNAseq studies, the functional significance of DECs (circRNAs) are more difficult to discern in that it is dependent on potential interactions with other RNA molecules and not necessarily tied to function of the parental gene of origin.
We used computational methods to assess the potential for the turkey circRNAs to interact with one class of small RNA molecules, miRNAs. As “sponges” circRNAs sequester miRNAs reducing the number of freely available interacting molecules and thereby suppressing their activity on target genes. Likewise, binding of RNA-binding proteins could also regulate gene expression. Scanning circRNAs for miRNA target sites was instrumental in identifying the novel RNA interactions involving the mouse Sry (Memczak et al., 2013) and human CDR1 genes (Hansen et al., 2011). The now classic example of RNA sponge activity is the circular antisense CDR1 transcript in humans that contains > 70 binding site seed matches for miR-17 (Hansen et al., 2011). Unfortunately, a miRNA database is currently not available for the turkey and therefore we relied on comparative analyses. As such, detailed analysis of the potential significance of circRNA differential expression and downstream effects on RNA interactions (such as miRNA binding) are limited.
To explore potential interactions, we used miRDB (Liu and Wang, 2019) and the miRNA dataset of the closely related chicken to scan for miRNA binding sites within the turkey circRNAs. Because extremely long RNAs are penalized within the miRDB algorithm (Xiaowei Wang, pers com), we selected the 24 DECs with predicted lengths of less than 5000 nt for analysis. Target sites for miRNAs were identified in 20 of the DECs with an average of 8.4 sites per circRNA (Figure 3). As might be expected the number of target sites increased positively with circRNA length. The greatest number of target sites (21) was identified in circ1354 (3811 nt) with miRNA gga-miR-6677-3p having the highest target score (80). Within the miRDB database, this chicken miRNA has 713 predicted gene targets. The highest miRNA target score (91) was observed for interaction of circ6609 with gga-miR-7437-3p. A total of 9 predicted miRNA target sites was identified for this 2487 nt circRNA, which was significantly underrepresented in the HS poult comparison. Understanding the potential interactions is complicated as within miRDB database a total of 740 predicted gene targets are included for gga-miR-7437-3p.
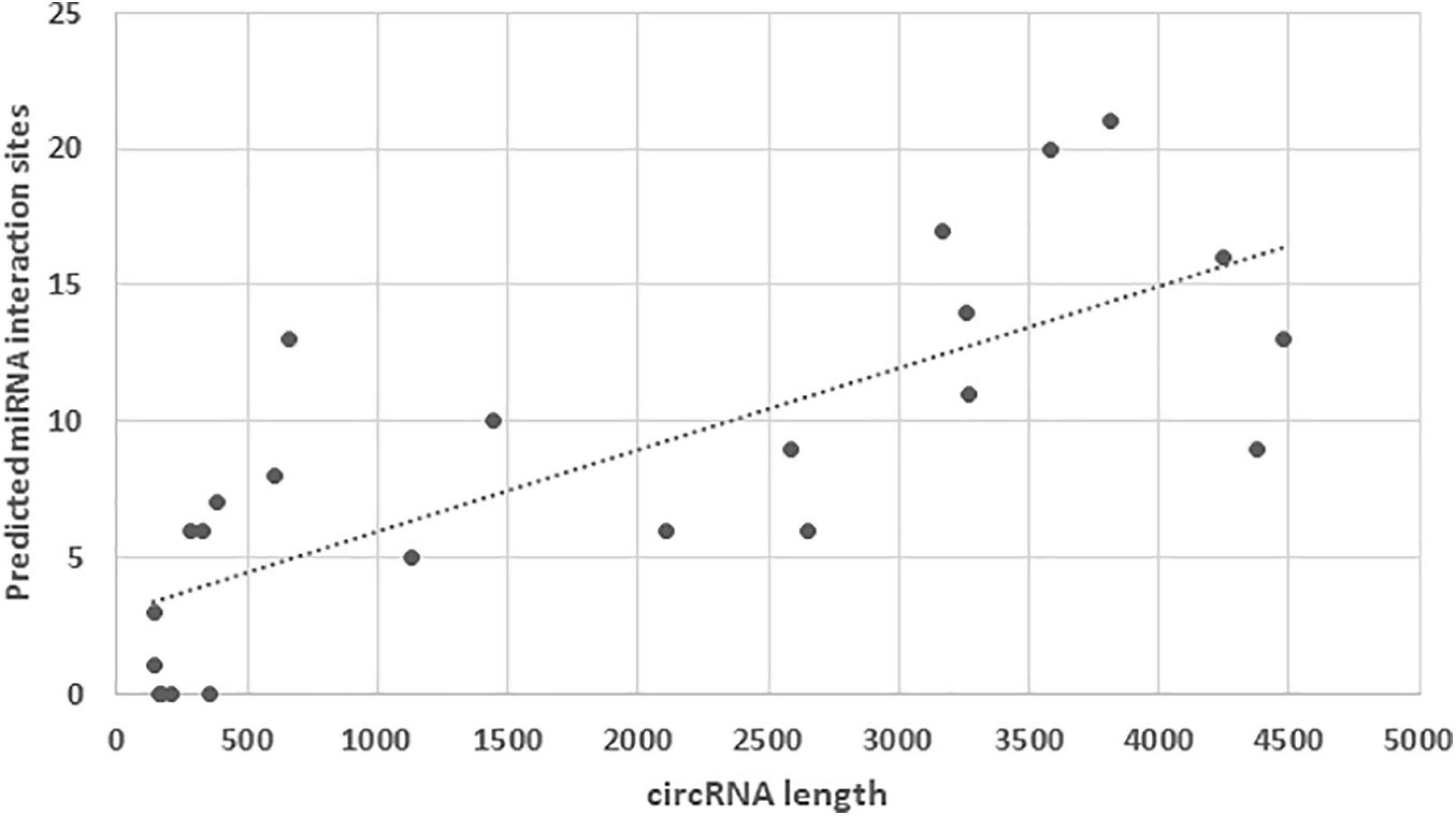
Figure 3. Distribution of miRNA target sites identified within the 24 DECs with lengths predicted by miRDB of less than 5000 nt.
We also examined two of the circRNAs of the multi-circRNA troponin T3 (TNNT3) gene. The exonic circRNA (circ3428) derived from TNNT3 is one of the smaller predicted circRNAs at 283 nt and it was significantly down regulated (Log2FC = −8.69) in the CS treatment comparison (Table 3). A second exonic DEC (circ3430, 327 nt) with sequence overlap with circ3428 within TNNT3, was also significantly downregulated in the F-Line under cold treatment (Log2FC = −8.12). These short circRNAs had 13 and 12 predicted miRNA target sites, respectively, with highest target scores corresponding to miRNAs gga-miR-7483-3p (83) and gga-miR-7437-5p (74). These miRNAs have 204 and 714 predicted target genes in the chicken, respectively. Interestingly, the junction regions of both of these circRNAs could be amplified by PCR, but failed in sequencing. We attribute the difficulty in sequencing to the likelihood that multiple amplicons were generated from these overlapping circRNAs. There is increasing evidence for the importance of miRNA interactions in muscle biology. For example, miR-24 (3p) and miR-128 (3p) play a role in myogenic satellite cell migration in turkey with a potential impact on muscle growth and development (Harding and Velleman, 2016; Velleman and Harding, 2017). Interactions between miRNAs and circRNAs have the potential to alter these processes (Liang et al., 2017; Ouyang et al., 2018a) and the circRNAs identified herein provide a framework for generating new hypotheses.
Conclusion
As circRNAs have not been previously characterized for any tissue in the turkey, we have only just begun to explore potential implications. Here we demonstrated the ability to identify circRNAs that are abundant and differentially expressed in turkey skeletal muscle in response to thermal stress. Analysis of a subset of these confirmed their presence and resistance to RNase R digestion and indicates presence of functional sequence elements within the predicted circRNAs. Characterization of the predicted circRNAs is complicated by their abundance and also potentially by completeness of the turkey genome. The majority of the circRNAs identified in this study, as defined by the junction reads, encompassed genomic regions > 10 Kb that included sequence gaps. Therefore, we suspect that this average length is potentially biased and necessitates confirmation of gaps by PCR and sequencing. Identifying differentially expressed circRNAs provides a framework for future investigation and detailed molecular and physiologic studies are needed to reveal their functional significance.
Data Availability Statement
Publicly available datasets were analyzed in this study. This data can be found here: SRA BioProject PRJNA419215.
Ethics Statement
Data used in this study was previously collected from experiments performed under institutionally approved protocol.
Author Contributions
All authors have made a substantial and intellectual contribution to the work, and approved the submitted article. KR conceived and designed the experiments with GS and SV. KM and JA performed the experiments and statistical analysis. KR wrote the manuscript. SV, GS, KM, and JA revised the manuscript.
Funding
This project was supported by Grants (2014-67003-21812 and 2020-67015-30827) from the Agriculture and Food Research Initiative of the United States Department of Agriculture to GS, KR, and SV.
Conflict of Interest
The authors declare that the research was conducted in the absence of any commercial or financial relationships that could be construed as a potential conflict of interest.
Publisher’s Note
All claims expressed in this article are solely those of the authors and do not necessarily represent those of their affiliated organizations, or those of the publisher, the editors and the reviewers. Any product that may be evaluated in this article, or claim that may be made by its manufacturer, is not guaranteed or endorsed by the publisher.
Supplementary Material
The Supplementary Material for this article can be found online at: https://www.frontiersin.org/articles/10.3389/fphys.2021.732208/full#supplementary-material
References
Abe, N., Matsumoto, K., Nishihara, M., Nakano, Y., Shibata, A., Maruyama, H., et al. (2015). Rolling circle translation of circular RNA in living human cells. Sci. Rep. 5:16435.
Abo Ghanima, M.M., Alagawany, M., Abd El-Hack, M.E., Taha, A., Elnesr, S.S., Ajarem, J., et al. (2020). Consequences of various housing systems and dietary supplementation of thymol, carvacrol, and euganol on performance, egg quality, blood chemistry, and antioxidant parameters. Poultry Sci. 99, 4384–4397. doi: 10.1016/j.psj.2020.05.028
Alagawany, M., Elnesr, S. S., Farag, M. R., Abd El-Hack, M. E., Barkat, R. A., and Gabr, A. A. (2021). Potential role of important nutraceuticals in poultry performance and health - A comprehensive review. Res. Vet. Sci. 137, 9–29. doi: 10.1016/j.rvsc.2021.04.009
Andrews, S. (2010). FastQC: a quality control tool for high throughput sequence data. Available Online at: http://www.bioinformatics.babraham.ac.uk/projects/fastqc
Barnes, N. E., Mendoza, K. M., Strasburg, G. M., Velleman, S. G., and Reed, K. M. (2019). Thermal challenge alters the transcriptional profile of the breast muscle in turkey poults. Poult. Sci. 98, 74–91. doi: 10.3382/ps/pey401
Bolger, A. M., Lohse, M., and Usadel, B. (2014). Trimmomatic: a flexible trimmer for Illumina sequence data. Bioinformatics 30, 2114–2120. doi: 10.1093/bioinformatics/btu170
Bose, R., and Ain, R. (2018). Regulation of transcription by circular RNAs. Adv. Exp. Med. Biol. 1087, 81–94. doi: 10.1007/978-981-13-1426-1_7
Cao, Y., You, S., Yao, Y., Wei, J., Hazi, W., Li, C., et al. (2018). Expression profiles of circular RNAs in sheep skeletal muscle. Asian-Australas J. Anim. Sci. 31, 1550–1557. doi: 10.5713/ajas.17.0563
Clark, D. L., Coy, C. S., Strasburg, G. M., Reed, K. M., and Velleman, S. G. (2016). Temperature effect on proliferation and differentiation of satellite cells from turkeys with different growth rates. Poult. Sci. 95, 934–947. doi: 10.3382/ps/pev437
Clark, D. L., Strasburg, G. M., Reed, K. M., and Velleman, S. G. (2017). Influence of temperature and growth selection on turkey pectoralis major muscle satellite cell adipogenic gene expression and lipid accumulation. Poult. Sci. 96, 1015–1027. doi: 10.3382/ps/pew374
Clark, D. L., and Velleman, S. G. (2016). Spatial influence on breast muscle morphological structure, myofiber size, and gene expression associated with the wooden breast myopathy in broilers. Poult. Sci. 95, 2930–2945. doi: 10.3382/ps/pew243
Das, A., Das, A., Das, D., Abdelmohsen, K., and Panda, A. C. (2020). Circular RNAs in myogenesis. Biochim. Biophys. Acta 1863:194372. doi: 10.1016/j.bbagrm.2019.02.011
Gao, Y., Wang, J., and Zhao, F. (2015). CIRI: An efficient and unbiased algorithm for de novo circular RNA. Genome Biol. 16:4.
Hansen, T. B., Wiklund, E. D., Bramsen, J. B., Villadsen, S. B., Statham, A. L., Clark, S. J., et al. (2011). MiRNA-dependent gene silencing involving Ago2-mediated cleavage of a circular antisense RNA. EMBO J. 30, 4414–4422. doi: 10.1038/emboj.2011.359
Harding, R. L., and Velleman, S. G. (2016). MicroRNA regulation of myogenic satellite cell proliferation and differentiation. Mol. Cell. Biochem. 412, 181–195. doi: 10.1007/s11010-015-2625-6
Huang, A., Zheng, H., Wu, Z., Chen, M., and Huang, Y. (2020). Circular RNA-protein interactions: functions, mechanisms, and identification. Theranostics 10, 3503–3517. doi: 10.7150/thno.42174
Huang, D. W., Sherman, B. T., and Lempicki, R. A. (2009). Bioinformatics enrichment tools: paths toward the comprehensive functional analysis of large gene lists. Nucl. Acids Res. 37, 1–13. doi: 10.1093/nar/gkn923
Kristensen, L. S., Andersen, M. S., Stagsted, L. V. W., Ebbesen, K. K., Hansen, T. B., and Kjemset, J. (2019). The biogenesis, biology and characterization of circular RNAs. Nat. Rev. Genet. 20, 675–691. doi: 10.1038/s41576-019-0158-7
Kulcheski, F. R., Christoff, A. P., and Margis, R. (2016). Circular RNAs are miRNA sponges and can be used as a new class of biomarker. J. Biotechnol. 238, 42–51. doi: 10.1016/j.jbiotec.2016.09.011
Lake, J. A., and Abasht, B. (2020). Glucolipotoxicity: a proposed etiology for wooden breast and related myopathies in commercial broiler chickens. Front. Physiol. 11:169. doi: 10.3389/fphys.2020.00169
Lei, M., Zheng, G., Ning, Q., Zheng, J., and Dong, D. (2020). Translation and functional roles of circular RNAs in human cancer. Mol. Cancer 19:30.
Li, H., and Durbin, R. (2009). Fast and accurate short read alignment with Burrows-Wheeler transform. Bioinformatics 25, 1754–1760. doi: 10.1093/bioinformatics/btp324
Li, Z., Zhang, J., Su, J., Liu, Y., Guo, J., Zhang, Y., et al. (2015). MicroRNAs in the immune organs of chickens and ducks indicate divergence of immunity against H5N1 avian influenza. FEBS Lett. 589, 419–425. doi: 10.1016/j.febslet.2014.12.019
Liang, G., Yang, Y., Niu, G., Tang, Z., and Li, K. (2017). Genome-wide profiling of Sus scrofa circular RNAs across nine organs and three developmental stages. DNA Res. 24, 523–535. doi: 10.1093/dnares/dsx022
Liu, W., and Wang, X. (2019). Prediction of functional microRNA targets by integrative modeling of microRNA binding and target expression data. Genome Biol. 20:18.
Memczak, S., Jens, M., Elefsinioti, A., Torti, F., Krueger, J., Rybak, A., et al. (2013). Circular RNAs are a large class of animal RNAs with regulatory potency. Nature 495, 333–338. doi: 10.1038/nature11928
Mi, H., Ebert, D., Muruganujan, A., Mills, C., Albou, L., Mushayamaha, T., et al. (2021). PANTHER version 16: a revised family classification, tree-based classification tool, enhancer regions and extensive API. Nucl. Acids Res. 49, D394–D403.
Mudalal, S., Lorenzi, M., Soglia, F., Cavani, C., and Petracci, M. (2015). Implications of white striping and wooden breast abnormalities on quality traits of raw and marinated chicken meat. Animal 9, 728–734. doi: 10.1017/s175173111400295x
Nestor, K. E. (1977), The stability of two randombred control populations of turkeys. Poult. Sci. 56, 54–57. doi: 10.3382/ps.0560054
Nestor, K. E. (1984). Genetics of growth and reproduction in the turkey. 2. Selection for increased body weight and egg production. Poult. Sci. 63, 2114–2122.
Ouyang, H., Chen, X., Li, W., Li, Z., Nie, Q., and Zhang, X. (2018a). Circular RNA circSVIL promotes myoblast proliferation and differentiation by sponging miR-203 in chicken. Front. Genet. 9:172. doi: 10.3389/fgene.2018.00172
Ouyang, H., Chen, X., Wang, Z., Yu, J., Jia, X., Li, Z., et al. (2018b). Circular RNAs are abundant and dynamically expressed during embryonic muscle development in chickens. DNA Res. 25, 71–86. doi: 10.1093/dnares/dsx039
Owens, C. M., Hirschler, E. M., McKee, S. R., Martinez-Dawson, R., and Sams, A. R. (2000). The characterization and incidence of pale, soft, exudative turkey meat in a commercial plant. Poult. Sci. 79, 553–558. doi: 10.1093/ps/79.4.553
Panda, A. C., Grammatikakis, I., Munk, R., Gorospe, M., and Abdelmohsen, K. (2017). Emerging roles and context of circular RNAs. Wiley Interdiscip. Rev. RNA 8:wrna.1386.
Patop, I. L., Wüst, S., and Kadener, S. (2019). Past, present, and future of circRNAs. EMBO J. 38:e100836. doi: 10.15252/embj.2018100836
Petracci, M., Bianchi, M., and Cavani, C. (2009). The European perspective on pale, soft, exudative conditions in poultry. Poult. Sci. 88, 1518–1523. doi: 10.3382/ps.2008-00508
Petracci, M., Mudalal, S., Bonfiglio, A., and Cavani, C. (2013). Occurrence of white striping under commercial conditions and its impact on breast meat quality in broiler chickens. Poult. Sci. 92, 1670–1675. doi: 10.3382/ps.2012-03001
Qu, S., Yang, X., Li, X., Wang, J., Gao, Y., Shang, R., et al. (2015). Circular RNA: A new star of noncoding RNAs. Cancer Lett. 365, 141–148. doi: 10.1016/j.canlet.2015.06.003
Quinlan, A. R., and Hall, I. M. (2010). BEDTools: a flexible suite of utilities for comparing genomic features. Bioinformatics 26, 841–842. doi: 10.1093/bioinformatics/btq033
Reed, K. M., Mendoza, K. M., Abrahante, J. E., Barnes, N. E., Velleman, S. G., and Strasburg, G. M. (2017a). Response of turkey muscle satellite cells to thermal challenge. I. Transcriptome effects in proliferating cells. BMC Genom. 18:352. doi: 10.1186/s12864-017-3740-4
Reed, K. M., Mendoza, K. M., Strasburg, G. M., and Velleman, S. G. (2017b). Response of turkey muscle matellite cells to thermal challenge. II. Transcriptome effects in differentiating cells. Front. Physiol. 8:948. doi: 10.3389/fphys.2017.00948
Samir, M., Vaas, L. A. I., and Pessler, F. (2016). MicroRNAs in the host response to viral infections of veterinary importance. Front. Vet. Sci. 3:86. doi: 10.3389/fvets.2016.00086
Velleman, S. G. (2015). Relationship of skeletal muscle development and growth to breast muscle myopathies: A review. Avian Dis. 59, 525–531. doi: 10.1637/11223-063015-review.1
Velleman, S. G., and Harding, R. L. (2017). Regulation of turkey myogenic satellite cell migration by microRNAs miR-128 and miR-24. Poult. Sci. 96, 1910–1917. doi: 10.3382/ps/pew434
Wang, P. L., Bao, Y., Yee, M.-C., Barrett, S. P., Hogan, G. J., Olsen, M. N., et al. (2014). Circular RNA is expressed across the eukaryotic tree of life. PLoS One 9:e90859. doi: 10.1371/journal.pone.0090859
Wilusz, J. E. (2018). A 360° view of circular RNAs: From biogenesis to functions. Wiley Interdiscip. Rev. RNA 9:e1478. doi: 10.1002/wrna.1478
Xu, J., Strasburg, G. M., Reed, K. M., and Velleman, S. G. (2021a). Effect of temperature and selection for growth on intracellular lipid accumulation and adipogenic gene expression in turkey pectoralis major muscle satellite cells. Front. Physiol. 12:667814. doi: 10.3389/fphys.2021.667814
Xu, J., Strasburg, G. M., Reed, K. M., and Velleman, S. G. (2021b). Response of turkey pectoralis major muscle satellite cells to hot and cold thermal stress: Effect of growth selection on satellite cell proliferation and differentiation. Comp. Biochem. Physiol. A Mol. Integr. Physiol. 252:110823. doi: 10.1016/j.cbpa.2020.110823
Xu, T., Wu, J., Han, P., Zhao, Z., and Song, X. (2017). Circular RNA expression profiles and features in human tissues: A study using RNA-seq data. BMC Genom. 18:680. doi: 10.1186/s12864-017-4029-3
Zampiga, M., Soglia, F., Baldi, G., Petracci, M., Strasburg, G. M., and Sirri, F. (2020). Muscle abnormalities and meat quality consequences in modern turkey hybrids. Front. Physiol. 11:554. doi: 10.3389/fphys.2020.00554
Zhang, X., Yan, Y., Lei, X., Li, A., Zhang, H., Dai, Z., et al. (2017). Circular RNA alterations are involved in resistance to avian leucosis virus subgroup-J-induced tumor formation in chickens. Oncotarget 8, 34961–34970. doi: 10.18632/oncotarget.16442
Zhang, Z., Yang, T., and Xiao, J. (2018). Circular RNAs: Promising biomarkers for human diseases. EBioMedicine 34, 267–274. doi: 10.1016/j.ebiom.2018.07.036
Keywords: RNAseq, differential expression, circular RNA, turkey skeletal muscle, poult
Citation: Reed KM, Mendoza KM, Abrahante JE, Velleman SG and Strasburg GM (2021) Data Mining Identifies Differentially Expressed Circular RNAs in Skeletal Muscle of Thermally Challenged Turkey Poults. Front. Physiol. 12:732208. doi: 10.3389/fphys.2021.732208
Received: 28 June 2021; Accepted: 06 August 2021;
Published: 25 August 2021.
Edited by:
Mahmoud M. Alagawany, Zagazig University, EgyptReviewed by:
Mahmoud Madkour, National Research Centre, EgyptHamada A. M. Elwan, Minia University, Egypt
Shaaban Saad Elnesr, Fayoum University, Egypt
Copyright © 2021 Reed, Mendoza, Abrahante, Velleman and Strasburg. This is an open-access article distributed under the terms of the Creative Commons Attribution License (CC BY). The use, distribution or reproduction in other forums is permitted, provided the original author(s) and the copyright owner(s) are credited and that the original publication in this journal is cited, in accordance with accepted academic practice. No use, distribution or reproduction is permitted which does not comply with these terms.
*Correspondence: Kent M. Reed, cmVlZHgwNTRAdW1uLmVkdQ==