- 1Institute of Basic Theory for Chinese Medicine, China Academy of Chinese Medical Sciences, Beijing, China
- 2School of Chinese Medicine, Hong Kong Baptist University, Hong Kong, China
The presence of hypertension (HTN) in type 2 diabetes mellitus (DM) is a common phenomenon in more than half of the diabetic patients. Since HTN constitutes a predictor of vascular complications and cardiovascular disease in type 2 DM patients, it is of significance to understand the molecular and cellular mechanisms of type 2 DM binding to HTN. This review attempts to understand the mechanism via the perspective of the metabolites. It reviewed the metabolic perturbations, the biological function of perturbated metabolites in two diseases, and the mechanism underlying metabolic perturbation that contributed to the connection of type 2 DM and HTN. DM-associated metabolic perturbations may be involved in the pathogenesis of HTN potentially in insulin, angiotensin II, sympathetic nervous system, and the energy reprogramming to address how perturbated metabolites in type 2 DM affect the pathogenesis of HTN. The recent integration of the metabolism field with microbiology and immunology may provide a wider perspective. Metabolism affects immune function and supports immune cell differentiation by the switch of energy. The diverse metabolites produced by bacteria modified the biological process in the inflammatory response of chronic metabolic diseases either. The rapidly evolving metabolomics has enabled to have a better understanding of the process of diseases, which is an important tool for providing some insight into the investigation of diseases mechanism. Metabolites served as direct modulators of biological processes were believed to assess the pathological mechanisms involved in diseases.
Introduction
Diabetes mellitus (DM) is an endocrine metabolic disease with an increasing prevalence all over the world. According to data released by the International Diabetes Federation, the number of DM patients is predicted to rise to 552 million by the year 2030. Type 1 and type 2 are the two main types, with type 2 DM accounting for the majority (>85%) of total DM prevalence (Forouhi and Wareham, 2014). Hypertension (HTN) is a crucial risk factor for type 2 DM-associated vascular complications. Vascular process whereby type 2 DM and HTN predispose to cardiovascular diseases. When combined with DM, HTN has been shown to predict and promote increased risk for cardiovascular diseases, which is the major cause of mortality in patients with type 2 DM (Petrie et al., 2018). Furthermore, up to 75% of diabetic cardiovascular diseases may be attributable to HTN (Sowers et al., 2001). There is a clinical impression that HTN occurs more frequently among diabetic patients than in the healthy population. It was proved to present in more than 50% of patients with type 2 DM. It is closely related to the occurrence and development of diabetic microvascular and macrovascular diseases. Indeed, DM and HTN were four times more likely to develop cardiovascular disease than non-diabetic controls with normal blood pressure. They also increased the incidence of other diabetes complications, such as kidney disease and retinopathy (Lastra et al., 2014). Hence, it is urgent to understand the molecular and cellular mechanisms of the combination of DM and HTN.
The rapidly evolving field of metabolomics is aiming at a comprehensive measurement of all endogenous metabolisms in biological systems (Acevedo-Calado et al., 2017). It represents both the upstream input from the environment and the downstream output of the genome (Wishart, 2016). Panels of multiple biomarkers reflecting their specificity and potentials for prediction have been developed (Savolainen et al., 2017a). In recent years, the value of metabolomics has been redefined from biomarker recognition tools as a technology for active drivers of biological processes (Johnson et al., 2016). The search for genes that are associated with metabolite levels, or whose products catalyze reactions, including disease-related metabolites and pathways, may explain the way these metabolic phenotypes are regulated (Zhang et al., 2018).
Moreover, metabolic research could also make it possible to understand the effects of specific microbes on specific metabolites better. Growing evidence accumulated that gut microbiota may not be overlooked in the pathogenesis of diseases. The gut microbiota affects the host by producing metabolites systematically. Small-molecule metabolites are one of the contributions of gut bacteria to host biology (Dodd et al., 2017). Metabolomics is a promising technology to contribute to the disease mechanism investigation since metabolites were served as direct modulators of biological processes and active entities in a biological process.
This review attempts to understand the mechanism of the combination of type 2 DM and HTN via the perspective of the metabolites. Firstly, it focuses on the metabolic perturbations of type 2 DM and HTN. After summarizing the biological function of perturbated metabolites, the paper associated the perturbated metabolites with the mechanisms in type 2 DM and HTN. Finally, the review reveals the effect of type 2 DM-associated metabolic perturbations on the pathogenesis of HTN.
Metabolic Perturbations in Type 2 DM and HTN
Metabolites are substrates or products of metabolic pathways. The changes in the metabolome directly reflect the gene expression, physiological status, and environmental effects on the biological system (Monteiro et al., 2013). Metabolic perturbations have been studied to diagnose and prediction of diseases by biomarkers. Over the past 15years, advances in metabolomics techniques, together with improvements in bioinformatics and mathematical modeling approaches, have provided the scientific community with tools to describe DM and HTN metabolomics. Three general techniques have become the main tools for metabolomics data acquisition: liquid chromatography-mass spectrometry (LC-MS), gas chromatography-mass spectrometry (GC-MS), and nuclear magnetic resonance (NMR). Metabolites are typically separated using gas chromatography (GC) and liquid chromatography (LC) before MS, and compounds are identified by mass and fragmentation patterns. However, there is no need to separate metabolites in NMR spectroscopy instead of measuring all metabolites at once. The use of multiple technologies was proved to broaden the level of metabolite coverage greatly. Metabolomics raw data processing involves noise reduction, spectrum deconvolution, peak detection and integration, chromatogram alignment, compound identification, and quantification (Shulaev, 2006). It is of great significance to perform statistical analysis on the processed data, reduce the number of variables, and obtain irrelevant features in the data. It can be best achieved either through unsupervised significance algorithms, such as principal component analysis (PCA), or through supervised methods, including t-test, χ2-tests, ANOVA, orthogonal partial least square discriminant analysis (OPLS-DA), and partial least squares (PLS; Lazar et al., 2015). The numbers of metabolites and metabolic classes have garnered much attention as biomarkers in the field of DM and HTN investigations. Validated biomarkers become alter markers that can substitute for clinical endpoints to monitor disease progression. An overview of the observed metabolic changes of type 2 DM and HTN is shown in Table 1. The comparison and correlation of the perturbated metabolites in type 2 DM and HTN were performed. The perturbated metabolites in common, perturbated metabolites in type 2 DM, and the perturbated metabolites in HTN were listed individually.
The metabolites, including amino acids, energy-related metabolites, lipids and fatty acids, carnitines, and bile acids were associated with type 2 DM and HTN. According to Table 1, we calculated proportions of different kinds of perturbated metabolites in the whole panel of metabolic markers of DM, HTN, and both of the two diseases individually and showed the ratios of each metabolic marker classes in DM, HTN, and the common of two diseases in the pie chart of Figure 1.
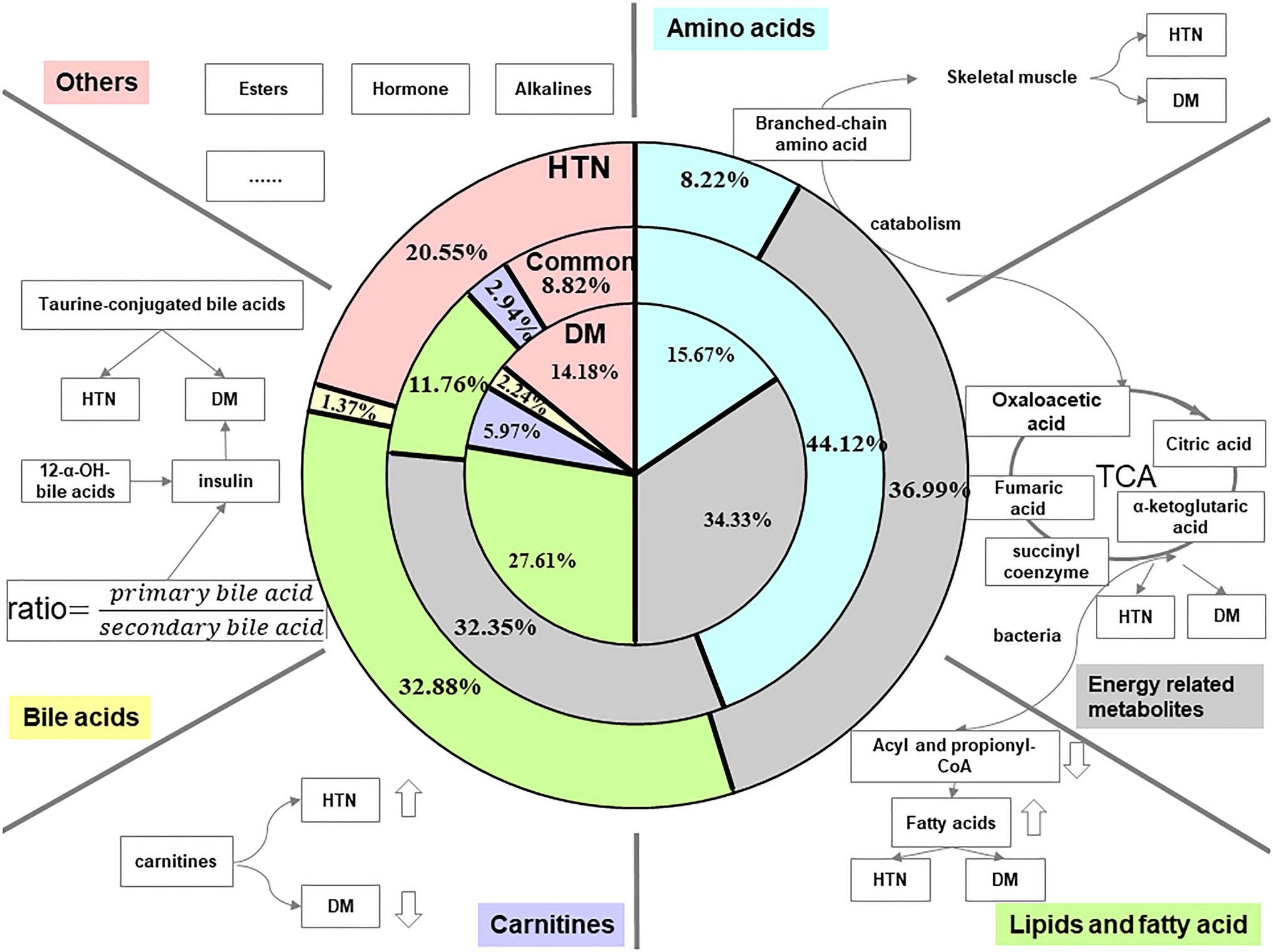
Figure 1. Summary of related metabolites of DM and HTN. The three pie charts, respectively, illustrate data regarding the distribution of different kinds of metabolites in HTN, common, and DM. The blue color represents the proportion of amino acids in the total reported metabolic perturbations. Amino acids occupy 8.22, 15.67, and 44.12% of perturbated metabolites in HTN, DM, and the common biomarkers of both DM and HTN, respectively. The gray color indicated the percentage of energy-related metabolites. The proportion of energy-related metabolites is almost evenly in HTN, common, and DM. The green color shows the partition of lipids and fatty acids. The lipids and fatty acids section consumed a little bit lower of 11.76% in the common perturbation metabolites, while the rest two have an almost equal share of 30% approximately. The purple and yellow indicated the makeup of carnitine and bile acids in HTN, DM, and the conventional biomarkers of both DM and HTN. Compared to other metabolites, these two kinds of perturbation had the smallest number. The pink color represents other sorts of perturbations in HTN, DM, and the common. They share less than a quarter of metabolic perturbation in HTN and make up 14.18% in DM and 8.82% in common.
Amino acids – Based on the review of the relevant literature, we listed the disturbances of amino acids in DM and HTN in Table 1 and pictured the blue pie charts to represent the ratio of amino acids in the whole panel of metabolic markers in Figure 1. The level of branched-chain amino acids (BCAAs; valine, leucine, and isoleucine) and aromatic amino acids (AAAs; tyrosine, phenylalanine, and tryptophan) was increasing in DM adults (Walford et al., 2013). Although most research is conducted in European populations, an analysis in Chinese and Asian-Indian men showed that the associated decrease in glycine was consistent with the increase in valine and leucine levels with multiple presentations of the development of type 2 DM and insulin resistance (Huffman et al., 2011). However, their role in the few available pediatric studies was controversial, with lower levels of BCAA (Michaliszyn et al., 2012; Mihalik et al., 2012; Frohnert and Rewers, 2016). A case-cohort study showed that the increased plasma levels of BCAAs/AAAs were associated with higher type 2 diabetes risk (Ruiz-Canela et al., 2018). Glycine and glutamine are reduced in insulin resistance (Sekhar et al., 2011; Stančáková et al., 2012). The potential role of BCAAs and AAAs with incident HTN is increasingly recognized. High plasma concentrations of BCAAs and AAAs are proved to be associated with an increased risk of newly developed HTN (Teymoori et al., 2018; Flores-Guerrero et al., 2019). According to the clinical implications, BCAAs were associated with the incident of type 2 DM. They also seem on the pathway from DM to cardiovascular diseases for the attenuation of the association of BCAA and cardiovascular patients without type 2 DM. However, it is still too early to tell whether BCAAs are the marker of risk for DM or cardiovascular diseases in DM.
Energy-related metabolites – The gray pie charts represent the ratio of energy-related metabolites in the whole panel of metabolic markers in Figure 1. The energy metabolism abnormalities are commonly associated with DM and HTN. The two diseases are related to gluconeogenesis, glycolysis, pentose phosphate, and tricarboxylic acid (TCA) cycle (Iemitsu et al., 2003; Ho et al., 2013). Hexose sugars are primarily accounted for by disease-defining increasing glucose and positively associated with DM. And the elevations of fructose, mannose, sorbitol, lactate, and malate have been proved to be associated with DM (Fiehn et al., 2010; Hwang et al., 2010; Menni et al., 2013). α-ketoglutarate, fumarate, and succinate levels in urine are decreased in DM patients (Salek et al., 2007).
Lipids and fatty acids – The green pie charts represent the ratio of lipids and fatty acids in the whole panel of metabolic markers in Figure 1. Given the significance of obesity as one of the risk factors for type 2 DM and HTN, it is not surprising that both DM and HTN patients have increased fat-derived metabolites. Phosphatidylcholine (PC), lysophosphatidylcholine (LPC), phosphatidylethanolamine (PE), and diacylglycerol (DAG) increased the risk of DM (Floegel et al., 2013). Conversely, a major class of sphingolipids, sphingomyelins (SM), and ceramide (Cer) was linked with a decreased risk of DM (Fall et al., 2016). Thromboxane, linoleic, phosphatidic acid (PA), lysophosphatidic acid (LPA), and phytosphingosine were increased, while sphinganine decreased in the HTN group (Tian et al., 2018). More and more evidence pointed to a mental disorder sphingolipid pathway trigger HTN. Hydroxyoctadecadienoic acids (HODEs) are related to HTN, the generation of which is increased where oxidative stress is increased. 9-HODE and 13-HODE are generated nonenzymatically in atherosclerosis. Increased HODE levels were proved to contribute to HTN and atherosclerosis progression (Vangaveti et al., 2010). Richard et al. highlight the discovery of 20-hydroxyeicosatetraenoic acid (20-HETE) in the regulation of renal function, vascular tone, and the development of HTN (Roman and Fan, 2018). The increasing levels of 12-HETE and 12-hydroperoxyeicosatetraenoic acid (12-HPETE) in HTN patients suggested roles for these metabolites in HTN (González-Núñez et al., 2000). The perturbed lipids in DM and HTN are listed in Table 1.
Carnitines – The purple pie charts represent the ratio of carnitines in the whole panel of metabolic markers in Figure 1. Propionyl carnitine, α-methyl butyl carnitine, and isovaleryl carnitine were elevated in type 2 DM (Pena et al., 2014; Qiu et al., 2016). The decrease of short-chain acylcarnitines and long-chain acylcarnitines, including C14:1 and C14:2, was observed in HTN patients (Bai et al., 2018).
Bile acids (BAs) – The yellow pie charts represent the ratio of carnitines in the whole panel of metabolic markers in Figure 1. In healthy subjects, insulin resistance increased by 12α-hydroxylated BAs. The ratios of non-12α-hydroxylated and 12α-hydroxylate d BAs are related to the main characteristics of insulin resistance (Hausler et al., 2013). Marlene et al. revealed that total taurine-conjugated BA concentration was higher in DM patients (Wewalka et al., 2014). A systematic evaluation of bile acid kinetics revealed that the contribution of specific types of bile acids varies in type 2 DM even though there was no difference in the size of the total BA pool. The level of deoxycholic acid was increased, and chenodeoxycholic acid was decreased (Brufau et al., 2010). Another study showed that lower cholic acid and higher plasma levels of deoxycholic acid occurred in diabetic patients compared to controls (Suhre et al., 2010).
Amino acids, energy-related metabolites, lipids, and fatty acids made up the biggest percentage of the total disturbed metabolites compared with BAs and other metabolites. They may play a significant role in the pathogenesis of HTN in DM. Hence, the biological function of these metabolites should be illustrated. It also can be found that the commonality of DM and HTN among metabolites is limited. To reveal how they are different or more interestingly amalgamated in both disease phenotypes, which leads to an intermediate or tertiary state that the patient’s metabolic physiology, the biological mechanism should also be discussed.
The Biological Function of Perturbated Metabolites in Type 2 DM and HTN
The metabolic biomarkers served as mechanistic discoveries. Metabolites modulate biological processes and phenotypes directly (Johnson et al., 2016). Direct modulation was associated with alterations in host metabolism, mainly through immune systems and hormone secretion (Li et al., 2018a). The metabolic potential of the gut microbiota is another contributing element in the development of DM and HTN (Kitai and Tang, 2017), with metabolites acting on distant target organs like the human host’s endocrine organ. The representative biological functions of each class of metabolites are shown in Figure 1.
Amino acids – Studies of BCAAs, such as valine, leucine, and isoleucine supplementation in animals and humans, suggest that circulating amino acids may promote insulin resistance directly, possibly via disrupting insulin signaling in skeletal muscle. BCAA in combination with hyperinsulinemia exerts a large amount of secretory pressure on pancreatic accessory cells, resulting in dysfunction of accessory cells. BCAAs catabolism offered intermediates to the TCA cycle, which may boost energy production (O’Connell, 2013). Metabolism studies indicated that changes in TCA flux in diabetic patients (Guan et al., 2013; Li et al., 2013). The reduction of the tricarboxylic acid cycle was shown in DM and HTN (Brindle et al., 2003). Betaine is a tertiary amine dimethylglycine (DMG) produced by homocysteine remethylation to methionine. It is catalyzed by betaine-homocysteine methyltransferase (BHMT). DMG is catalyzed by DMG dehydrogenase (DMGDH) and sarcosine dehydrogenase in turn. Epidemiological data indicated that plasma levels of betaine correlate with key components of the metabolic syndrome in the opposite direction (Konstantinova et al., 2008). High plasma glycine levels are also thought to be associated with increased insulin sensitivity (Menni et al., 2013).
Metabolites involved in energy metabolism – Energy metabolism includes energy release and energy restoration, which provides a source of energy for the daily activity of cells. Mitochondrial is of significance in maintaining cellular energy metabolic homeostasis (Alston et al., 2017). The disequilibrium of mitochondrial functions in type 2 DM leads to the deficits of downstream in several parts, including cardiac output, skeletal muscle contraction, β-cell insulin production, and hepatocyte metabolism (Pinti et al., 2019). HTN, one of the cardiovascular diseases, is closely related to myocardial energy metabolism (Polak-Iwaniuk et al., 2019). Recent research proved that the improved skeletal muscle energy metabolism correlates to the recovery of β cell function and benefits the glucose control of type 2 patients (Tang et al., 2019). Fatty acids are the main fuel for the heart, while glucose and lactate serve the remaining need (Calvani et al., 2000). It is reported that the changes in glucose and fatty acids utilization appear in the diabetic heart fuel selection (Stanley et al., 1997). A downshifting of ketone body production and the breakdown and the tricarboxylic acid cycle was observed in insulin resistance (Heinonen et al., 2015). HTN shifts substrate preference toward increased glucose utilization in cardiac muscle. Citrate, pyruvate, malate, and adenosine monophosphate were the common metabolic markers for DM and HTN. When perfusion is reduced, there is an increase in the rate of glycolysis and a switch from lactate uptake to lactate production. During reperfusion, fatty acid oxidation quickly recovers. Otherwise, carbohydrates, such as hexoses, fructose, and gluconic acid, and nucleotides, such as adenosine, were the marker for DM and HTN, which linked to endothelial dysfunction, insulin resistance (Khitan and Kim, 2013).
Lipids and fatty acids – DM significantly decreased levels of acetyl- and propionyl-CoA means that it prevents the degradation of fatty acids, amino acids and ketone bodies. In contrast, an increase in free fatty acids (FFAs) occurred in HTN (Aa et al., 2010). FFAs regulate glucose counter-regulation by mediating hepatic glucose production (Fanelli et al., 1993). Lipolysis also mediates the delayed glucose-antiregulation pathway of growth hormone and cortisol stimulation. Hyperinsulinemic hypoglycemia increases the production of non-esterified fatty acids, indicating the role of lipids as alternative energy sources (Voss et al., 2017). The prevailing theory of lipid-induced hepatic insulin resistance is that lipid species accumulate due to oxidative damage to fatty acids, leading to the redirection of long-chain acyl-coenzyme A (LC-CoAs) to endoplasmic reticulum (ER) localization and cytosolic lipid species, such as triglycerides, diacylglycerols, and ceramides (Muoio and Newgard, 2008). Type 2 diabetes can be the result of the loss of or deficiency of beneficial functions, such as short-chain fatty acid produced by carbohydrate fermentation in the intestinal ecosystem (Zhao et al., 2018). Meanwhile, FFAs influence HTN (Aa et al., 2010). 12-HETE, transformed from arachidonic acid, acted as vasoconstrictors in the renal arteries, which cause the elevation of blood pressure (González-Núñez et al., 2000). The critical role of 20-HETE in the regulation of renal function and vascular tone makes it participate in the development of HTN and cardiovascular diseases.
Carnitines – According to the researches, 13 kinds of carnitines were the biomarkers of DM (Kappel et al., 2017). It transported long-chain fatty acids to the mitochondria and then to be oxidized to produce energy. In the cardiac muscle and skeletal, carnitine utilizes fatty acids as fuel. Insulin resistance is associated with oxidative defects in muscle fatty acids and played a crucial part in the development of type 2 DM (Mingrone, 2004). Studies also have shown that intravenous L-carnitine supplementation reduces insulin sensitivity in the distal muscles of diabetic patients (Gaetano et al., 1999). Finding from the study shows that carnitine lowers diastolic blood pressure in adults (Askarpour et al., 2019). L-carnitine and propionyl-L-carnitine can reduce the species of reactive oxygen species in HTN and increase the involvement of nitric oxide (NO) in endothelium-dependent relaxation (Bueno et al., 2005). The action of carnitine-dependent enzymes produces acetyl-CoA through the β-oxidation pathway, which modulates the intramitochondrial acetyl-CoA/CoA ratio affecting glucose oxidation (Calvani et al., 2000). An affected carbohydrate and lipid metabolism are verified in type 2 DM and HTN. The dysregulated fatty acid metabolism, along with tissue lipid accumulation, is associated with the development of HTN in type 2 DM. As the dominant role of carnitines in the balance of carbohydrate and fatty acid metabolism, it is likely to be a potential adjuvant in the treatment of type 2 DM.
BAs – BAs are steroid acids found in the bile of mammals predominantly. Primary BAs (cholic acid and chenodeoxycholic acid) are synthesized in the liver. Intestinal bacterial converts primary BAs into secondary BAs (Russell, 2003). In humans, taurocholic acid and glycocholic acid and taurochenodeoxycholic acid and glycochenodeoxycholic acid are the main bile salts in bile, with approximately equal concentrations (Hofmann, 1999). The level of taurine-conjugated BAs is elevated in type 2 diabetes (Liu et al., 2013), and the ratio of 12-α OH BAs has been reported to relative to insulin resistance (Hausler et al., 2013). Acarbose increases the ratio of primary BAs and secondary BAs. Uncombined BAs have also been proved to be elevated in type 2 DM patients at the beginning of treatment (Gu et al., 2017). Taurine is higher in DM and HTN groups (Fujiwara et al., 2005; Yokoi et al., 2015). An enhancement of creatinine was occurred in HTN, while it decreases in type 2 DM (Grapov et al., 2015). The supplementation with cholic acid and activation of the G protein-coupled bile acid receptor can significantly reduce the blood pressure (Shi et al., 2021).
Gut microbiome-related metabolites – In recent years, the relationship between the complexity and diversity of the gut microbiota and host diseases has been demonstrated (Weiss and Hennet, 2017; Li et al., 2018b). The gut microbiota constantly communicates hypotheses were brain-gut-microbiome axis (Mahony et al., 2015), brain-gut-kidney axis (Yang et al., 2018), and gut-liver axis (Tripathi et al., 2018). It produces several metabolites and accumulated in the bloodstream (Nicholson et al., 2012). High levels of AAAs, such as AA-phenylalanine, tyrosine, and glycine, occurred (Neis et al., 2015). Antidiabetic medication Acarbose was proved to alter plasma and fecal BAs composition via the mediate gut microbiota. The relative abundances of Lactobacillus and Bifidobacterium in the gut microbiota increased, while Bacteroides reduced. Moderating the relative abundance of microbial genes is related to bile acid metabolism (Gu et al., 2017). Studies have shown that the negative correlation between the hippurate reflects the physiological relationship with gut microbial (Holmes et al., 2008). Minocycline can cure resistant HTN patients because of its potent antihypertensive effect on the gut microbiota (Qi et al., 2015). Gut microbial composition research found that hypertensive rats having reduced taxa richness and altered microbial composition. The Firmicutes to Bacteroidetes ratio was higher in the hypertensive rats (Yang et al., 2015). Salt-sensitive rats showed higher blood pressure rather than salt-resistant rats, which was related to the elevated plasma levels of the short-chain fatty acids (SCFAs). It suggested gut microbiota-derived SCFAs produce the endocrine hormones. SCFAs, as a source of energy for colonocytes and bacterial communities, help maintain gut barrier function by inhibiting pathogenic microorganisms and reducing luminal pH. Host-signaling mechanisms, such as the G-protein-coupled receptors (GPR) 41 and olfactory receptor 78 (Olfr78), can modulate blood pressure (Tang and Hazen, 2017). Trimethylamine-N-oxide (TMAO), amino acids, and creatine related to energy metabolism were proved to contribute to HTN (Mell et al., 2015). TMAO was proved to be a strong predictor of coronary artery disease risk. Mechanistically, gut microbes transformed trimethylamine-containing nutrients, such as carnitine, phosphatidylcholine, and choline to TMAO, which leads to atherogenesis and thrombosis. In mice, increasing TMAO level decreased reverse cholesterol transport and altered bile acid transport, composition and pool size (Koeth et al., 2013). Meanwhile, TMAO modulated stimulus-dependent calcium mobilization in platelets, enhancing platelet responsiveness (Zhu et al., 2016). Accompanying metabolism functional changes, gut dysbiosis has been implicated in the pathogenesis ranging from insulin resistance to atherosclerosis and thrombosis.
What is noteworthy is that the biggest percentage of the total disturbed metabolites share a common biological function: provide energy. Besides amino acids, energy-related metabolites, lipids, and fatty acids, carnitines play the regulation role of the fatty acids and glucose metabolism in physiological and pathological conditions. A decreased ratio can relieve the transfer of acetyl groups from acetyl-CoA to carnitine, forming acetylcarnitine, a reaction catalyzed by carnitine acetyl-transferase. This activity of L-carnitine in the modulation of the intramitochondrial acetyl-CoA/CoA ratio affects glucose oxidation (Calvani et al., 2000). Other circulating substrates, such as ketones or BCAAs, may become an alternative source of energy.
The Connection of Mechanism Underlying Metabolic Perturbation Between Type 2 DM and HTN
The development of metabolomics has facilitated the pathophysiology discovery of DM and HTN. The biomarker identified to reveal the mechanism of diseases via early changes highlights pathways and targets. Integration of the metabolism field with other disciplines, such as endocrinology and immunology, hints the metabolic alterations and substrate utilization. Here, as shown in Figure 2, we associated the biological function of metabolites with the mechanism of DM and HTN. DM and HTN are frequent comorbid conditions that may share underlying metabolic pathways, including the sympathetic nervous and renin-angiotensin-aldosterone system, insulin resistance, and inflammation processes (Schutta, 2007; Cheung and Li, 2012).
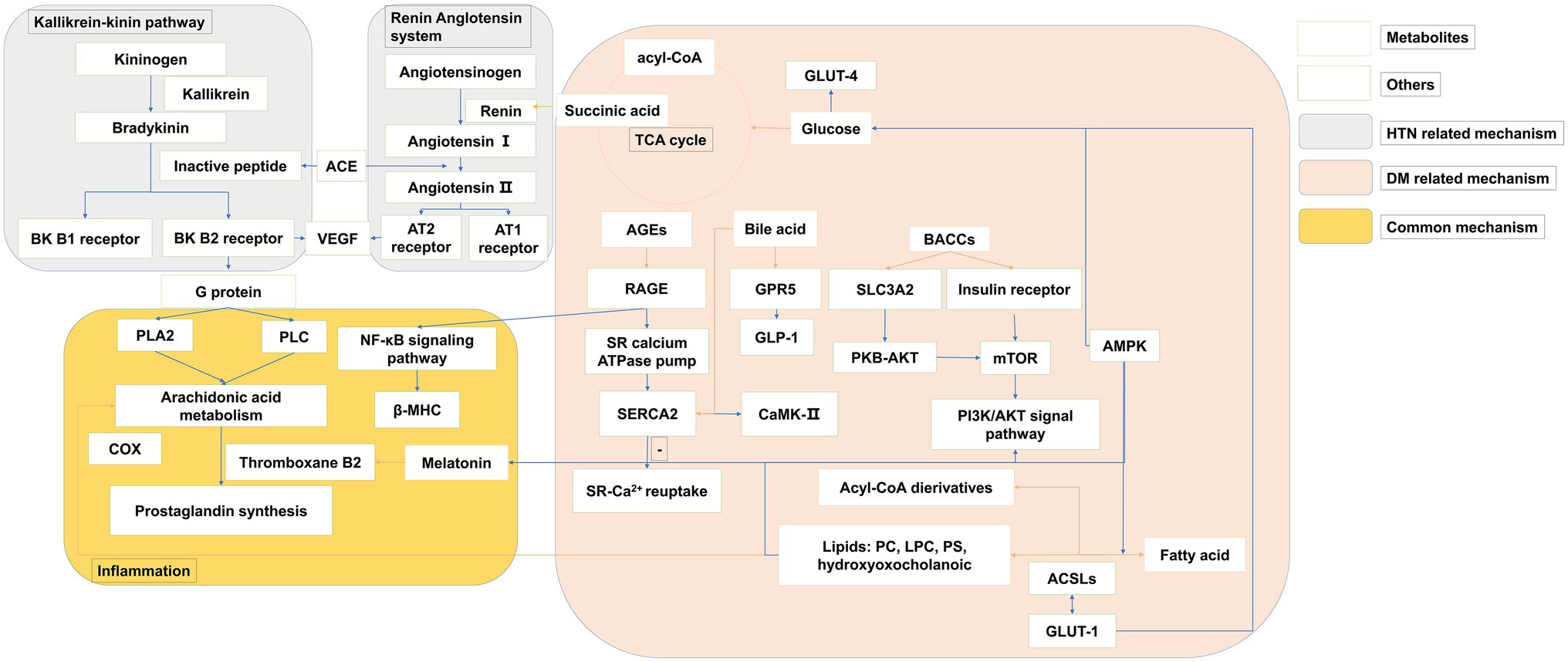
Figure 2. The overview of metabolites related common mechanisms of type 2 DM and HTN. The pink panel summarized the metabolites mechanism related to type 2 DM. The gray panel consists of the kallikrein-kinin pathway and the renin-angiotensin system, which are the main progress of HTN. The yellow panel presented the common metabolic mechanism of both type 2 DM and HTN. Orange row arrows the association of metabolites and biomolecules.
Role of Metabolic Perturbations in HTN
A variety of disorders have been identified in HTN, including the renin-angiotensin system, the autonomic nervous system, and the immune system (as shown in the gray panel in Figure 2). Changes in the autonomic nervous system associated with increases in peripheral and neuroinflammation are associated with the pathogenesis of drug resistance to HTN. Recent evidence suggests that the increased peripheral and neuronal inflammation of HTN is due to the effect of autonomic nerves on bone marrow activity (Zubcevic et al., 2014). The SCFA olfactory Olf78 is also expressed in the kidneys, where it regulates blood pressure. Elevated FFAs may alter membrane fluidity by altering Na+-K+ ATPase pump, Na+, K+, and Ca2+ currents, membrane phospholipids structure, and intracellular Na+ and Ca2+ levels. This is the mechanism that increased the vascular muscle tension and consequently HTN (Ordway et al., 1991).
Emerging evidence suggested that gut microbiota plays a crucial part in the development of HTN. This effect was thought to be mainly due to the SCFAs (Holmes et al., 2008). The phylum Bacteroidetes and family Veillonellaceae were proved to be more abundant in salt-sensitive than in salt-resistant rats (Mell et al., 2015).
Role of Metabolic Perturbations in DM
As shown in the pink panel in Figure 2, the increased level of BCAAs was improved to make an influence in insulin sensitivity via the mammalian target of rapamycin complex (mTORC) and target ribosomal protein S6 kinase 1 (S6K1) in the downstream (O’Connell, 2013). The effector of mTOR, S6K1, is sensitive to both AA and insulin. Phosphatidylinositol 3-kinase (PI3K)/Akt signaling can downregulate autophagy by inhibiting the mTOR, and the changes in autophagy were associated with reduced PI3K/Akt signaling in insulin resistance (Bugger and Abel, 2014). The numbers of metabolites separating the DM groups were associated with mitochondria. The centrality of the mitochondrial function in catabolic and metabolic diseases of BCAA has been noted previously (Newgard, 2012). BCAA may damage mitochondrial oxidation of glucose and lipids, which can lead to mitochondrial stress and impaired insulin secretion and action. Impaired mitochondrial function in DM could decrease the capacity of the mitochondria to decompose BCAAs, resulting in elevated levels of BCAAs and BCKAs. The dysfunction of educed mitochondrial biogenesis was rescued by augmentation of adenosine 5′monophosphate-activated protein kinase (AMPK) activity (Dugan et al., 2013). The studies showed that the peptide hormone is produced by adipose cells, such as adiponectin/Acre 30 and retinol-binding protein-4 (RBP4). They also produce resistin and proinflammatory cytokines, such as interleukin-6 (IL-6) and tumor necrosis factor-α (TNF-α). Several BA species have been recognized as modulators of energy metabolism by activation of nuclear receptors, such as G-protein-coupled BA receptor (TGR5) and farnesoid X receptor (FXR) (Thomas et al., 2008). The activation of FXR induces fibroblasts to grow in the intestine. Although the mechanisms are not yet fully understood, the release of glucagon-like peptide 1 (GLP-1) and peptide YY (PYY), as well as changes in BA metabolism and enhancing signaling via FXR, is thought to play a role. TGR5 is necessary to improve glucose metabolism, which may be related to DM (Tremaroli et al., 2015). SCFAs can stimulate GPR 41 and 43, and these are expressed in the renal vasculature. These effects include several processes such as inflammation and intestinal endocrine regulation (Samuel et al., 2008). However, the balance across these G-protein-coupled receptor activities is complex and likely to be dynamic (Pluznick, 2013). SCFAs can also trigger GPR41 and GPR43 to secrete GLP-1, which plays a substantial role in pancreatic function and insulin release. GPR41 induces intestinal endocrine hormone PYY expression in the epithelial L cells through increasing dietary energy gain (Samuel et al., 2008). Adipose cells also produce other peptide hormones, including retinol-binding protein RBP4 and adiponectin/Acrp 30). Fat-specific glucose transporter 4 (GLUT4) knockout mice showed elevated RBP4 levels (Yang et al., 2005). The expression of monocyte chemotactic protein-1 (MCP1) and IκB kinase catalyzes injury and infection of subunit-β (IKKβ) recruitment sites in the adipocyte. It may be a mechanism for increased inflammatory signals during the development of DM (Arkan et al., 2005; Chen et al., 2005).
Recent studies show that individual bacteria concerned with insulin resistance have the same phenotype for recipient mice when transferred to normal, specific, and non-pathogenic mice (Pedersen et al., 2016; Liu et al., 2017b). Amino acids act on mTOR receptor regulating pathways and physiological processes, like insulin. Gut microbiota can regulate TGR5 signal-generating receptor agonists and FXR signal metabolism antagonists, such as tauro-β-muricholic acid (Sayin et al., 2013). TGR5 is required for improved metabolism of glucose (Tremaroli et al., 2015). Propionate SCFs stimulate GLP1 and PYY hormone release in the primary gut culture of mice through the FFA receptor 2 (FFAR2) dependent mechanism (Tolhurst et al., 2012). Gut microbiota induces PYY in an FFAR3-dependent manner (Psichas et al., 2015).
Role of Metabolic Perturbations in DM and HTN
As shown in Figure 2 (yellow panel), both of the metabolic perturbation mechanisms of DM and HTN link to the inflammation. Subjects with DM had elevated plasma FFA levels, which played a detrimental role in the pathogenesis of HTN. FFAs lead to multiple mechanisms, including the activation of the renin-angiotensin system, impaired insulin signaling and NO production, oxidative stress, inflammation, and apoptosis in the endothelial cells, which make an influence in the DM and HTN co-exist (Ghosh et al., 2017). Melatonin has a special place in the prevention and treatment of metabolic syndromes, such as DM and HTN (Cardinali et al., 2011). It has an anti-inflammatory property, partly for its role as a metabolic regulator. Melatonin’s resistance to inflammation occurs in the correction of metabolic disorders, which prevent insulin resistance. Impaired serine phosphorylation of insulin receptor substrate 1 (IRS-1) and the subsequent upregulation of IRS-1 expression may be crucial (She et al., 2014). Via the activation of CREB-PGC-1α pathway, melatonin could prevent insulin resistance and mitochondrial dysfunction (Teodoro et al., 2014). Both melatonin and melatonergic agonists counteract the blockade of this critical step in insulin signal (She et al., 2009; Quan et al., 2015). Regeneration and pancreatic β-cells lead to decreased blood glucose after melatonin treatment in the diabetic rats model (KanterEmail et al., 2006). Melatonin treatment improves insulin sensitivity and lipid metabolism in type 2 diabetic rats (R.C. Mazepa et al., 1999; Shieh et al., 2009). The inhibition effect of melatonin on platelet aggregation and thromboxane B2 was also proved.
Type 2 DM-Associated Metabolic Perturbations Potentially Involved in the Pathogenesis of HTN
Perturbated metabolites exert several physiological responses either directly through the activation of powerful humoral systems, indirectly stimulation localized target organs, or via postganglionic sympathetic nerves. The actions and interactions of insulin, angiotensin II, inflammation, vascular dysfunction, or central nervous systems lead to the pathogenesis and progression of HTN. Here, we pictured the overview of physiological processes in Figure 3.
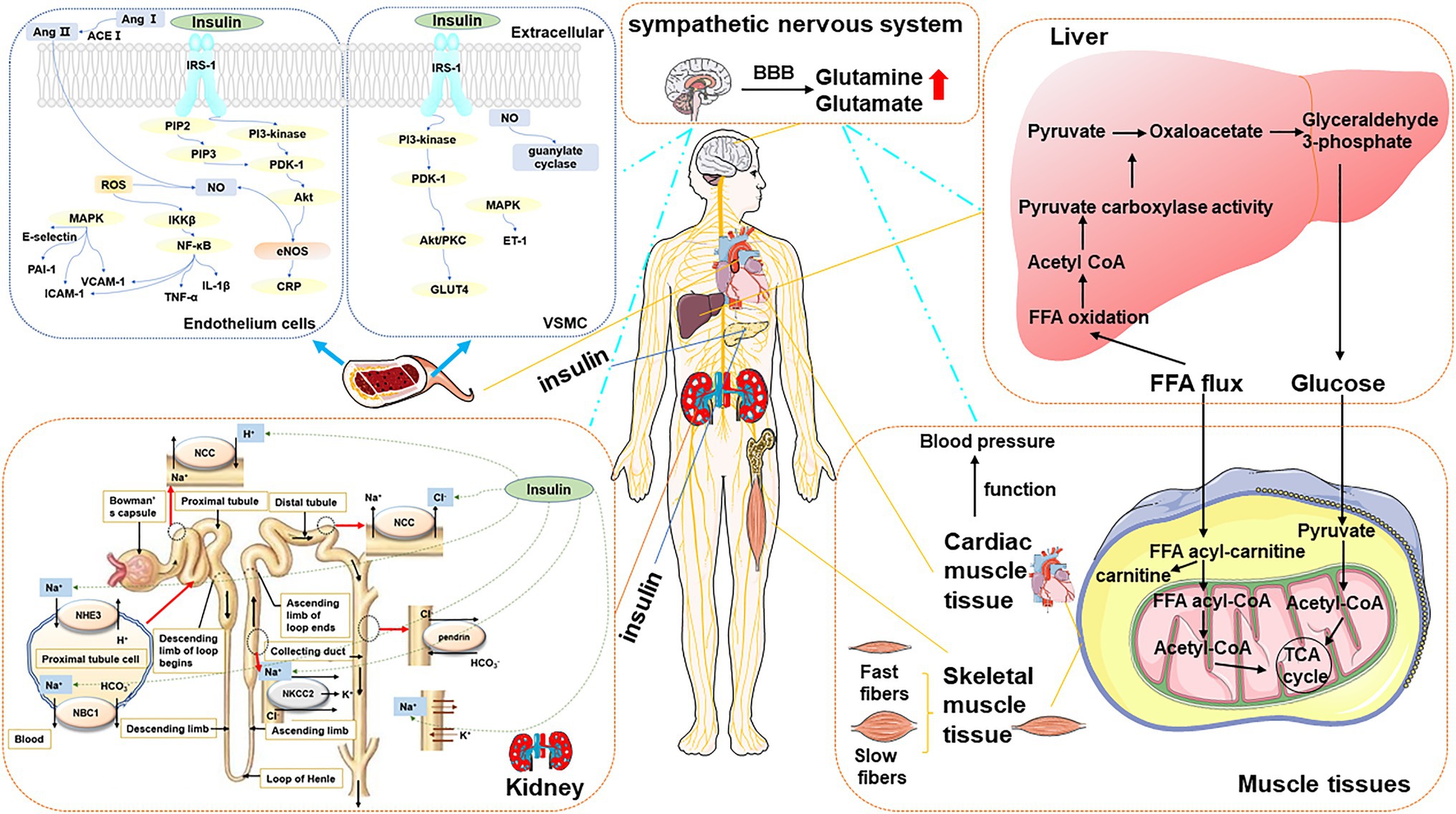
Figure 3. The overview of type 2 DM-associated metabolic perturbations potentially involved in the pathogenesis of HTN. Blood vessel The left panel presented the mechanisms of HTN in DM in the endothelium cell. The mechanism started with IRS triggered activation of PI3K and the conversion from PIP2 to PIP3. It allowed PDK-1 to access, leading to Akt activation. Akt increased eNOS activity and stimulated the production of NO. Meanwhile, Ang II and ROS are another way to produce NO. MAPK-dependent insulin signaling pathways tend to promote pro-HTN insulin metabolite. It inhibits PAI-1, E-selection, ICAM, and VCAM. The right panel presented the mechanism of HTN in DM in VSMC. The increased blood flow further facilitates glucose uptake by provoking insulin-responsive GLUT4 translocation in PI3K-dependent metabolic actions. MAPK-dependent insulin signaling pathways tend to promote ET-1. The production of NO is related to guanylate cyclase. Kidney The sodium-chloride cotransporter (NCC) has the function of reabsorbing sodium and chloride ions from the tubular fluid. Insulin promotes the resorption of chloride ions. Insulin stimulates the NHE3 on the apical membrane and upregulates the NBC1 on the basolateral membrane. In the thick ascending limb of Henle, insulin stimulates NaCl reabsorption by activating NKCC2 and Na-K-ATPase. The sodium and bicarbonate were reabsorbed. Insulin can also stimulate the Na-K-ATPase in the proximal tubule in the proximal tubule. Liver and muscular tissue FFA is the major oxidation fuel for muscular tissue, such as cardiac muscle tissue and skeletal muscle tissue, while glucose provides the remaining need. The transformation of cardiac function metabolites affects the function of the heart, which is closely related to blood pressure. The balance of FFA and glucose results in the transformation of two muscle types: slow-twitch myofibers and fast-twitch fibers. Sympathetic nervous system It controls peripheral organs, including the liver, skeletal muscle, pancreas, vascular and cardiovascular system resulting in glucose disorder, insulin resistance, insulin secretion impairment, and blood pressure increase, which are predicted to chronically contribute to the development of DM and HTN.
Insulin Sensitivity in Cells and Tissue
Reduced sensitivity to insulin in tissue is a characteristic of multiple pathological conditions (Du et al., 2006). HTN in subjects with DM was significantly related to insulin resistance (Rewers et al., 2004). Acute hyperglycemia consistently impairs endothelial function in type 2 DM patients (Wallis et al., 2005). Reducing glucose levels may inhibit vascular changes leading to HTN (The DCCT Research Group, 1988; Purnell et al., 1998).
Insulin metabolism is often mediated by insulin signaling. PI3K/Akt pathway causes atherothrombosis via multiple mechanisms. On the one hand, it produces several beneficial molecules. On the other hand, it inhibits plasminogen activator inhibitor type 1 (PAI-1), E-selection, intercellular adhesion molecule (ICAM), and vascular cell adhesion molecule (VCAM). The increased expression level of VCAM1 and ICAM1 and inflammatory cytokines, like interleukin IL6, IL18, IL1β, TNF α, and TGF-β, lead to myocyte inflammation in the DM and HTN (Rajesh et al., 2012). Glucotoxicity, lipotoxicity, and inflammation impair the PI3K-dependent insulin signaling pathway, which constructs the combination of DM and HTN (Muniyappa et al., 2008). PI3K-dependent insulin signaling pathways regulate vasodilator and insulin metabolite in skeletal muscle and vascular endothelium. MAPK-dependent insulin signaling pathways tend to promote pro-HTN insulin metabolite. Insulin resistance, hyperglycemia, oxidative stress, and attenuate inflammation and renin-angiotensin process in endothelial smooth muscle and kidney issues are predicted to make a simultaneous beneficial influence in the co-existed DM and HTN.
In vein endothelial and endothelial cells – One of the important roles of insulin is to accelerate the production of the effective vasodilator factor NO by endothelial cells (Vincent et al., 2003; Muniyappa et al., 2007). The binding of insulin binding to its receptor (a receptor tyrosine kinase) causes phosphorylation of IRS-1, which can activate PI3K. The lipid products of PI3K [PI-3,4,5-triphosphate (PIP3)] can stimulate the phosphorylation and activation of PDK1, which in turn phosphorylates and activates Akt. Akt increased the eNOS activity and stimulated the production of NO, which is calcium-independent (Montagnani et al., 2001). NO inhibits the production of ROS, which leads to endothelial dysfunction and promotes the development of atherosclerosis (Muniyappa et al., 2008). ET-1, as a potent vasoconstrictor, is stimulated by insulin and plays a crucial role in endothelial dysfunction and contributes to the development of HTN, using the MAPK-dependent signaling pathway (Marasciulo et al., 2006). And the increased expression level of PAI-1. ICAM-1, VCAM-1, and E-selectin may contribute to accelerated atherosclerosis in insulin-resistant conditions (Bonetti et al., 2003). Insulin stimulates the expression of VCAM-1, PAI-1, and E-selectin in endothelial cells through the MAPK-dependent pathway (Montagnani et al., 2002; Mukai et al., 2007). The suppression of PI3K or Akt174 increased the insulin-induced PAI-1 and adhesion molecule expression.
When endothelial cells are exposed to high concentrations of glucose, insulin-stimulated Akt and eNOS activation is significantly reduced (Du et al., 2001; Wang et al., 2006). Hyperglycemia is also believed to be associated with impaired glucose tolerance. It induces an increasing level of ROS production, post-translational O-GlcNacylation, PKC activity, and advanced glycation end products (AGEs), which is known to specifically inhibit the PI3K/Akt/eNOS pathway. Diabetes causes insulin resistance and endothelial dysfunction by increasing oxidative stress, and formatting AGEs (Du et al., 2001; Muniyappa et al., 2007). Compared with the harmful effects of the PI3K/Akt/eNOS pathway, hyperglycemia can enhance the secretion of endothelial endothelin (ET-1), thereby changing the balance between NO and ET-1, which is beneficial to endothelial dysfunction (Muniyappa et al., 2008). In vivo, acute intravenous glucosamine causes metabolic insulin resistance and impairs insulin-mediated increase in femoral arterial blood flow and capillary replacement (Wallis et al., 2005).
Elevated circulating markers of inflammation characterize insulin resistance and endothelial dysfunction. The most widely studied proinflammatory cytokine related to insulin resistance is IKKβ, TNF-α, and C-Jun N-terminal kinase (JNK) (Kim et al., 2005). IL-1β receptor-associated kinase was activated by TNF-α, which stimulated serine phosphorylation of IRS-1/2 directly or indirectly. It compounds the decreasing activation of PI3K/Akt/eNOS in endothelial cells (Zhang et al., 2003; Anderson et al., 2004; Eringa et al., 2004; Gustafson et al., 2007). TNF-α can also increase ET-1 secretion in a MAPK-dependent fashion (Sury et al., 2006). Another cytokine, IL-6, was elevated and can inhibit increased eNOS activity and increased NO production stimulated by insulin in endothelial cells (Andreozzi et al., 2007). C-reactive protein (CRP), which served as a marker of inflammation, was proved to inhibit insulin-induced NO production in endothelial cells by inhibiting the PI3K/Akt/eNOS pathway (Schwartz et al., 2007; Xu et al., 2007).
In vascular smooth muscle and vascular smooth muscle cells (VSMC) – Endothelial NO diffuses into adjacent VSMC, where it stimulates guanosine cyclase. The elevated levels of cGMP can give rise to vasorelaxation. NO can also modulate vascular tone by reducing monocyte adhesion, increasing the production of proinflammatory cytokines, attenuating the expression of vascular cell adhesion molecules, limiting the recruitment of leukocytes, inhibiting VSMC, avoiding platelet aggregation, and opposing apoptosis (Herman and Moncada, 2005). The increased blood flows further facilitate the glucose uptake by provoking insulin-responsive GLUT4 in PI3K-dependent metabolic actions (Zeng and Quon, 1996; Vincent et al., 2004). In human cells, three IRS subtypes (IRS-1, -2, and -4) have been identified to have a distinct role based on cell type and metabolic status. The function of IRS-1 is related to the insulin secretion mechanism, which plays a vital role in skeletal muscle (Taguchi and White, 2008). Animal models showed retarded growth of IRS-1 knockout mice especially skeletal muscle (Withers et al., 1998). Glucosamine can impair insulin and stimulate the uptake of glucose in skeletal muscle and NO production. It can also increase the blood flow in femoral arterial and capillary recruitment (Wallis et al., 2005).
In the kidney – Reduced insulin sensitivity leads to compensatory hyperinsulinemia. Maintaining normal blood sugar, glucose is taken up by skeletal muscle and adipose cells. Compensatory hyperinsulinemia with insulin resistance reinforced the salt absorption of the proximal tubule, which may lead to salt overload and HTN (Soleimani, 2015). The sodium-chloride cotransporter (NCC) has the function of reabsorbing sodium and chloride ions from the tubular fluid. Insulin promotes the resorption of chloride ions. The effect of insulin on IRS1-mediated adipocyte glucose uptake is severely attenuated, and the effect on IRS2-mediated proximal renal tubular salt reabsorption remains (Zheng et al., 2005). The kidney tubules are significant for maintaining vascular flow and blood pressure because of the sodium reabsorption regulation. Insulin was proved to promote salt reabsorption. Insulin stimulates the Na+/H+ exchanger type 3 (NHE3) on the apical membrane and upregulates the sodium-bicarbonate cotransporter (NBC1) on the basolateral membrane. The sodium and bicarbonate were reabsorbed. Insulin can also stimulate the Na-K-ATPase in the proximal tubule in the proximal tubule (Landsberg et al., 2013). In the thick ascending limb of Henle, insulin stimulates NaCl reabsorption by activating Na-K-2Cl cotransporters (NKCC2) and Na-K-ATPase (Horita et al., 2011). Insulin enhanced the epithelial sodium channel density in the distal nephron and the connecting tubule membrane (Landsberg et al., 2013). The accumulated reports showed that the stimulatory effect of insulin on with-no-lysine kinases (WNK), suggesting that sodium increases sodium reabsorption through sodium-chloride cotransporter in the distal nephron (Landsberg et al., 2013).
The Activation of Angiotensin II
DM-induced hyperglycemia can cause HTN by activating Angiotensin II (Ang II). Ang II is involved in the pathogenesis of insulin resistance through the induction of key signaling elements of the insulin AKT (also known as protein kinase B) pathway (Andreozzi et al., 2004). It also serves as an essential vasoconstrictor hormone in the renin-angiotensin system (RAS), where RAS is activated (Kobori et al., 2007). These findings suggested that the cross-action of insulin AKT and Ang II signaling pathways plays a significant role in the co-occurrence of DM and HTN. Ang II inhibits insulin metabolism signals and promotes insulin resistance by activating the mTOR/S6 kinase 1 (S6K1)-mediated IRS-1 serine phosphorylation (Pulakat et al., 2011). Ang II reduces glucose uptake by inhibiting IRS1 phosphorylation, inhibiting PI3-K function and reducing GLUT-4 to interfere with the insulin pathway (Manrique et al., 2009). Moreover, Ang II hinders the bioavailability of NO through NADPH oxidase activation and ROS generation, which impeding endothelium. And it can also enhance NF-κB, which in turn promotes the production of TNF-α and IL-6 and adhesion molecule VCAM-1, regulating inflammation (Pueyo et al., 2000).
The Activation of the Sympathetic Nervous System
The activation of the sympathetic nervous system exerts a number of physiological responses either directly through stimulation of postganglionic sympathetic nerves localized in target organs or indirectly through the activation of powerful humoral systems. The importance of sympathetic tone is readily acknowledged for blood pressure regulation and type 2 DM (Thorp and Schlaich, 2015). The sympathetic nervous system control over peripheral organs, including the liver, skeletal muscle, pancreas, and cardiovascular system, is perturbed, resulting in glucose disorder, insulin resistance, insulin secretion impairment, and blood pressure increase, which are predicted to chronically contribute to the development of DM and HTN. Otherwise, the RAS and inflammation activated by metabolites or insulin are demonstrated to increase the central sympathetic nervous system activity to elevate blood pressure.
Glutamate and glutamine, as common metabolic perturbations of both DM and HTN, are listed in Table 1. Previous research indicated that glutamate could penetrate the blood–brain barrier, excite the presympathetic neurons within the paraventricular nucleus, and increase the sympathetic outflow (Li et al., 2006). Glutamine abounds in the central nervous system, and its interstitial and cerebrospinal fluid concentration are at least one the order of magnitude higher than of any other amino acid. It is a major excitatory neurotransmitter in the vertebrate nervous system, and glutamatergic synaptic inputs innervate the presympathetic neurons located in the autonomic nucleus in the brain stem and hypothalamus. The effects of metabolites on brain regions involved in insulin and blood pressure regulation are a mechanism of DM that resulted in the pathogenesis of HTN.
The Energy Reprograming
It found that the commonality of DM and HTN among metabolites is limited. How they are different or more interestingly amalgamated in both disease phenotypes, which leads to an intermediate or tertiary state, should be explored. After summarizing the metabolic distribution in DM and HTN, amino acids, energy-related metabolites, lipids, and fatty acids were revealed to be made up the biggest percentage of the total disturbed metabolites and share a common biological function: provide energy. Here, we discussed the energy-provide metabolites transformation in the cardiac muscle and skeletal muscles.
Cardiac muscle – The transformation of cardiac function metabolites seems to disturb much more metabolites and affect the pathogenesis of HTN in type 2 DM. In the normal heart, carbohydrate and FFA oxidation mainly contribute to energy production. While type 2 DM dysregulated cardiac FFA oxidation and impaired glucose oxidation/uptake, other circulating substrates, such as ketones or BACCs, may become an alternative source of energy, which injured the heart and cause high blood pressure. Bedi et al. (2016) recently demonstrated that in the severely failing heart increased the utilization of ketone. Although the role of BCAA is less clear because of the diminishment of BCAA catabolism in heart failure, ketones and BCAAs can directly influence cardiac signaling processes. It may potentially exhibit additional beneficial effects on the heart. In the recent EMPA-REG OUTCOME trial, treatment with empagliflozin, it was observed the reduction of cardiovascular mortality and hospitalization for heart failure in patients with type 2 DM. The research that investigated the effect of empagliflozin on metabolism showed that it reduced the glucose and other sugars in the serum and degraded FFAs, amino acids, and ketone with increased levels of acetyl- and propionyl carnitine.
Skeletal muscles – Similar to the heart muscle, skeletal muscles play key roles in the regulation of systemic energy homeostasis in metabolic responses to physical activity. Excess triglycerides, FFAs, and glucose, coupled with physical inactivity, perturb the metabolism in skeletal muscle. Skeletal muscle made an influence on energy homeostasis by changing the composition of slow and fast-twitch fiber types muscles, which differ in the composition of contractile proteins, oxidative capacity, and substrate preference for ATP production (Baskin et al., 2015). Fast-twitch fibers have a higher fatigability, higher strength of contraction, lower oxidative capacity, and a preference for glucose as a substrate for ATP production through anaerobic glycolysis. Slow-twitch myofibers have a high oxidative capacity and prefer fatty acids as substrate for ATP production, while fast-twitch fibers have a lower oxidative capacity and prefer glucose.
Muscle profoundly impacts systemic energy consumption. In the pathological state of DM, the energy reprogramming changes the metabolic function of the heart and then changes the cardiac output which affects blood pressure directly.
Conclusion and Perspectives
Type 2 DM is known to be associated with HTN. The widespread of type 2 DM also poses many problems. The presence of type 2 DM increases the risk of HTN. When combined with DM, HTN has been shown to predict and promote increased risk for cardiovascular diseases, which is the leading cause of death in patients with DM. Metabolites reflect both the upstream input from the environment and the downstream output of the genome, making it possible to explore the interaction between genes and the environment. Focusing on the application of metabolomics seems to contribute to reveal the root cause of type 2 DM. Knowledge of the pathophysiological derangements involved in the occurrence of type 2 DM and HTN is critical for successful prevention and control solutions. In this review, metabolites perturbation and the underlying metabolic mechanism were summarized. Among the metabolites, amino acid, lipid metabolites, carnitines, bile acids, and other metabolites were elaborated. And the related metabolites resulted in insulin resistance, increased tissue inflammatory, ROS production, endothelial dysfunction, the distribution of the renin-angiotensin system, the activation of the sympathetic nervous system, and the energy reprograming.
There were several limitations in the present review. First, the prior mechanism studies that are relevant to the thesis might be limited because of the scope of the HTN topic. The complex influence factors are involved in the endocrine system, nervous system, and immune system. Direct influences and feedback interaction conveyed by the bloodstream from one tissue to another exacerbate the complexity of the problem. The deep mechanism researches should continue to be followed up in the future. Second, the current review is focused on how perturbated metabolites in diabetes mellitus affect the pathogenesis of hypertension. A contextual focus on vice-versa relations may need in-depth further exploration and contextual consideration.
With the development of informatics, integration of orthogonal biological approaches, and analytical technologies, it is of possibility to expand the application of metabolomics to understand the systemic function of metabolites. It seems to be promising to elaborate the mechanism in HTN in type 2 DM by regarding the metabolites as the beginning of the biological process and reveal the active role (Rinschen et al., 2019). With the review, the mechanism of HTN in type 2 DM may be investigated by gut microbial, inflammation, and energy metabolism processes.
Gut Microbial in HTN and Type 2 DM
Intestinal microbial populations contribute to energy collection, host metabolism, and disturbances in these population balances affect inflammation, glucose metabolic changes, and energy metabolomics. Furthermore, through diligent research efforts in gut microbial metabolites, the underlying mechanisms of type 2 DM and HTN are beginning to be elucidated. Microbial influenced metabolites were proved to link the microbiota and host blood pressure regulation (Shi et al., 2021). Microbial gene was proved to facilitate metabolites conversion by functional and genetic engineering studies. The microbial porA gene promotes phenylalanine change to phenylacetic acid, with the host generation fostering platelet responsiveness and thrombosis potential (Nemet et al., 2020). The Casp1−/− microbiota reduced microbiota-derived anti-inflammatory SCFAs (Brandsma et al., 2019). The gut microbiota also proved to regulate blood glucose via modulating the enteric neurons (Matheis et al., 2020).
Inflammation in HTN With Type 2 DM
In this review, inflammation is proved to be a potential common mechanism of type 2 DM and HTN. It is widely acknowledged that inflammatory disorder is the main pathological process of many diseases (Hummasti and Hotamisligil, 2010). Indeed, subclinical chronic inflammation is a common feature in their natural course. The application and development of pro-resolution therapeutic strategies for treating inflammatory pathology could lead to the revolutionary management of human ailments. Fullerton and Gilroy (2016) highlighted strive to identify pathways that target diseases that involve inflammation. Age, as a risk factor of the inflammation, underlies type 2 DM and HTN (Yandrapalli et al., 2018). Elie Metchnikoff proposed that bacterial products activated phagocytes resulting in inflammation (Metchnikoff, 2003). Aging-associated microbiota was proved to promote inflammation and reversing age-related microbiota variation for reducing inflammation and the accompanying morbidity in recent research (Thevaranjan et al., 2017). And the enhancement of autophagy and the normalization of mitochondrial function can alleviate aging-associated inflammation (Bharath et al., 2020). Novel insights into the cellular processes especially the metabolic reprogramming of immune cells driving inflammation are more or less established (Pålsson-McDermott and O’Neill, 2020). Adaptive immune memory, macrophage iron handling, epigenetic regulation of immunity, and Th2 immunity can directly affect immune function and ultimately systemic metabolism (Caslin and Hasty, 2019). The mechanism by which DM and HTN may synergistically induce macrophage metabolic dysfunction, especially during cardiac remodeling, is not fully understood (Mouton et al., 2020). It will provoke a re-think of chronic inflammation in HTN and type 2 DM therapeutically.
Energy Metabolism Switch of the Immune System in HTN With Type 2 DM
The idea that cellular metabolism affects immune function is currently being pursued. The physical activities and cellular functions related to energy were decreased owing to unmatched energy demand and supply. In return, the metabolic shift was accelerated and forming a vicious cycle. Reprogramming of energy metabolism causes a shift in energy metabolism from oxidative metabolism to glycolysis, which promotes the prevalence of aging-associated diseases, such as type 2 DM and HTN (Feng et al., 2016). These findings provide a reference for future research on the pathogenesis of type 2 DM and diabetic complications.
Author Contributions
ZN and ZS wrote the paper. CW, SP, and XW revised the paper. ZL and AL designed the outline of the paper. All authors contributed to the article and approved the submitted version.
Funding
This work was supported by the National Natural Science Foundation Committee of China (Project Nos. 82003950 and 81873009) and the Central Research Institutes of Basic Research and Public Service Special Operations (Project Nos. YZ-202045 and YZ-1912).
Conflict of Interest
The authors declare that the research was conducted in the absence of any commercial or financial relationships that could be construed as a potential conflict of interest.
Publisher’s Note
All claims expressed in this article are solely those of the authors and do not necessarily represent those of their affiliated organizations, or those of the publisher, the editors and the reviewers. Any product that may be evaluated in this article, or claim that may be made by its manufacturer, is not guaranteed or endorsed by the publisher.
References
Aa, J., Wang, G., Hao, H., Huang, Q., Lu, Y., Yan, B., et al. (2010). Differential regulations of blood pressure and perturbed metabolism by total ginsenosides and conventional antihypertensive agents in spontaneously hypertensive rats. Acta Pharmacol. Sin. 31, 930–937. doi: 10.1038/aps.2010.86
Acevedo-Calado, M., James, E. A., Morran, M. P., Pietropaolo, S. L., Ouyang, Q., Arribas-Layton, D., et al. (2017). Identification of unique antigenic determinants in the amino terminus of IA-2 (ICA512) in childhood and adult autoimmune diabetes: new biomarker development. Diabetes Care 40, 561–568. doi: 10.2337/dc16-1527
Akira, K., Imachi, M., and Hashimoto, T. (2005). Investigations into biochemical changes of genetic hypertensive rats using 1H nuclear magnetic resonance-based metabonomics. Hypertens. Res. 28, 425–430. doi: 10.1291/hypres.28.425
Akira, K., Masu, S., Imachi, M., Mitome, H., Hashimoto, M., and Hashimoto, T. (2008). 1H NMR-based metabonomic analysis of urine from young spontaneously hypertensive rats. J. Pharm. Biomed. Anal. 46, 550–556. doi: 10.1016/j.jpba.2007.11.017
Allalou, A., Nalla, A., Prentice, K. J., Liu, Y., Zhang, M., Dai, F. F., et al. (2016). A predictive metabolic signature for the transition from gestational diabetes mellitus to type 2 diabetes. Diabetes 65, 2529–2539. doi: 10.2337/db15-1720
Alston, C. L., Rocha, M. C., Lax, N. Z., Turnbull, D. M., and Taylor, R. W. (2017). The genetics and pathology of mitochondrial disease. J. Pathol. 241, 236–250. doi: 10.1002/path.4809
Anderson, H. D. I., Rahmutula, D., and Gardner, D. G. (2004). Tumor necrosis factor-alpha inhibits endothelial nitric-oxide synthase gene promoter activity in bovine aortic endothelial cells. J. Biol. Chem. 279, 963–969. doi: 10.1074/jbc.M309552200
Andreozzi, F., Laratta, E., Procopio, C., Hribal, M. L., Sciacqua, A., Perticone, M., et al. (2007). Interleukin-6 impairs the insulin signaling pathway, promoting production of nitric oxide in human umbilical vein endothelial cells. Mol. Cell. Biol. 27, 2372–2383. doi: 10.1128/MCB.01340-06
Andreozzi, F., Laratta, E., Sciacqua, A., Perticone, F., and Sesti, G. (2004). Angiotensin II impairs the insulin signaling pathway promoting production of nitric oxide by inducing phosphorylation of insulin receptor substrate-1 on Ser312 and Ser616 in human umbilical vein endothelial cells. Circ. Res. 94, 1211–1218. doi: 10.1161/01.RES.0000126501.34994.96
Arkan, M. C., Hevener, A. L., Greten, F. R., Maeda, S., Li, Z.-W., Long, J. M., et al. (2005). IKK-beta links inflammation to obesity-induced insulin resistance. Nat. Med. 11, 191–198. doi: 10.1038/nm1185
Askarpour, M., Hadi, A., Bozorg, A. D. K., Sadeghi, O., Sheikhi, A., Kazemi, M., et al. (2019). Effect of L-carnitine supplementation on blood pressure: a systematic review and meta-analysis of randomized controlled trials. J. Hum. Hypertens. 33, 725–734. doi: 10.1038/s41371-019-0248-1
Bai, Q., Peng, B., Wu, X., Cao, Y., Sun, X., Hong, M., et al. (2018). Metabolomic study for essential hypertension patients based on dried blood spot mass spectrometry approach. IUBMB Life 70, 777–785. doi: 10.1002/iub.1885
Baskin, K. K., Winders, B. R., and Olson, E. N. (2015). Muscle as “mediator” of systemic metabolism. Cell Metab. 21, 237–248. doi: 10.1016/j.cmet.2014.12.021
Bedi, L. C. Jr., Snyder, N. W., Brandimarto, J., Aziz, M., Mesaros, C., Worth, A. J., et al. (2016). Evidence for intramyocardial disruption of lipid metabolism and increased myocardial ketone utilization in advanced human heart failure. Circulation 133, 706–716. doi: 10.1161/CIRCULATIONAHA.115.017545
Bharath, L. P., Agrawal, M., McCambridge, G., Nicholas, D. A., Hasturk, H., Liu, J., et al. (2020). Metformin enhances autophagy and normalizes mitochondrial function to alleviate aging-associated inflammation. Cell Metab. 32, 44–55. doi: 10.1016/j.cmet.2020.04.015
Bonetti, P. O., Lerman, L., and Lerman, A. (2003). Endothelial dysfunction: a marker of atherosclerotic risk. Arterioscler. Thromb. Vasc. Biol. 23, 168–175. doi: 10.1161/01.ATV.0000051384.43104.FC
Brandsma, E., Kloosterhuis, N. J., Koster, M., Dekker, D. C., Gijbels, M. J. J., Velden, S. v. d., et al. (2019). A proinflammatory gut microbiota increases systemic inflammation and accelerates atherosclerosis. Circ. Res. 124, 94–100. doi: 10.1161/CIRCRESAHA.118.313234
Brindle, J. T., Nicholson, J. K., Schofield, P. M., Grainger, D. J., and Holmes, E. (2003). Application of chemometrics to 1H NMR spectroscopic data to investigate a relationship between human serum metabolic profiles and hypertension. Analyst 128, 32–36. doi: 10.1039/b209155k
Brufau, G., Bahr, M. J., Staels, B., Claudel, T., Ockenga, J., Böker, K. H., et al. (2010). Plasma bile acids are not associated with energy metabolism in humans. Nutr. Metab. (Lond.) 7:73. doi: 10.1186/1743-7075-7-73
Bueno, R., de Sotomayor, M. A., Perez-Guerrero, C., Gomez-Amoresb, L., Vazquez, C. M., and Dolores Herrera, M. (2005). L-carnitine and propionyl-L-carnitine improve endothelial dysfunction in spontaneously hypertensive rats: different participation of NO and COX-products. Life Sci. 77, 2082–2097. doi: 10.1016/j.lfs.2005.01.035
Bugger, H., and Abel, E. D. (2014). Molecular mechanisms of diabetic cardiomyopathy. Diabetologia 57, 660–671. doi: 10.1007/s00125-014-3171-6
Bujak, R., Yumba-Mpanga, A., Struck-Lewicka, W., Kordalewska, M., Polonis, K., Patejko, M., et al. (2018). Untargeted metabolomics provides insight into mechanisms underlying resistant hypertension. Curr. Med. Chem. 25, 1–12. doi: 10.2174/0929867324666171006122656
Calvani, M., Reda, E., and Arrigoni-Martelli, E. (2000). Regulation by carnitine of myocardial fatty acid and carbohydrate metabolism under normal and pathological congdition. Basic Res. Cardiol. 95, 75–83. doi: 10.1007/s003950050167
Cardinali, D. P., Cano-Barquilla, P., Jiménez-Ortega, V., and Esquifino, A. (2011). Melatonin and the metabolic syndrome: physiopathologic and therapeutical implications. Neuroendocrinology 93, 133–142. doi: 10.1159/000324699
Caslin, H. L., and Hasty, A. H. (2019). Extrinsic and intrinsic immunometabolism converge: perspectives on future research and therapeutic development for obesity. Curr. Obes. Rep. 8, 210–219. doi: 10.1007/s13679-019-00344-2
Chen, A., Mumick, S., Zhang, C., Lamb, J., Dai, H., Weingarth, D., et al. (2005). Diet induction of monocyte chemoattractant protein-1 and its impact on obesity. Obes. Res. 13, 1311–1320. doi: 10.1038/oby.2005.159
Cheung, B. M. Y., and Li, C. (2012). Diabetes and hypertension: is there a common metabolic pathway? Curr. Atheroscler. Rep. 14, 160–166. doi: 10.1007/s11883-012-0227-2
Cooper-DeHoff, R. M., Hou, W., Weng, L., Baillie, R. A., Beitelshees, A. L., Gong, Y., et al. (2014). Is diabetes mellitus-linked amino acid signature associated with β-blocker-induced impaired fasting glucose. Circ. Cardiovasc. Genet. 7, 199–205. doi: 10.1161/CIRCGENETICS.113.000421
de Mello, V. D., Paananen, J., Lindström, J., Lankinen, M. A., Shi, L., Kuusisto, J., et al. (2017). Indolepropionic acid and novel lipid metabolites are associated with a lower risk of type 2 diabetes in the Finnish diabetes prevention study. Sci. Rep. 7, 46337–46348. doi: 10.1038/srep46337
Dietrich, S., Floegel, A., Weikert, C., Prehn, C., Adamski, J., Pischon, T., et al. (2016). Identification of serum metabolites associated with incident hypertension in the European prospective investigation into cancer and nutrition-Potsdam study. Hypertension 68, 471–477. doi: 10.1161/HYPERTENSIONAHA.116.07292
Dodd, D., Spitzer, M. H., Treuren, W. V., Merrill, B. D., Hryckowian, A. J., Higginbottom, S. K., et al. (2017). A gut bacterial pathway metabolizes aromatic amino acids into nine circulating metabolites. Nature 551, 648–652. doi: 10.1038/nature24661
Du, X., Edelstein, D., Dimmeler, S., Ju, Q., Sui, C., and Brownlee, M. (2001). Hyperglycemia inhibits endothelial nitric oxide synthase activity by posttranslational modification at the Akt site. J. Clin. Invest. 108, 1341–1348. doi: 10.1172/JCI11235
Du, X., Edelstein, D., Obici, S., Higham, N., Zou, M., and Brownlee, M. (2006). Insulin resistance reduces arterial prostacyclin synthase and eNOS activities by increasing endothelial fatty acid oxidation. J. Clin. Invest. 116, 1071–1080. doi: 10.1172/JCI23354
Dugan, L. L., You, Y.-H., Ali, S. S., Diamond-Stanic, M., Miyamoto, S., DeCleves, A.-E., et al. (2013). AMPK dysregulation promotes diabetes-related reduction of superoxide and mitochondrial function. J. Clin. Invest. 123, 4888–4899. doi: 10.1172/JCI66218
Eringa, E. C., Stehouwer, C. D. A., van Amerongen, G. P. N., Ouwehand, L., Westerhof, N., and Sipkema, P. (2004). Vasoconstrictor effects of insulin in skeletal muscle arterioles are mediated by ERK1/2 activation in endothelium. Am. J. Physiol. Heart Circ. Physiol. 287, H2043–H2048. doi: 10.1152/ajpheart.00067.2004
Fall, T., Salihovic, S., Brandmaier, S., Nowak, C., Ganna, A., Gustafsson, S., et al. (2016). Non-target metabolomics comnined with genetic analyses identifies bile acid synthesis and phospholipid metabolism as being associated with incident type 2 diabetes. Diabetologia 59, 2114–2124. doi: 10.1007/s00125-016-4041-1
Fanelli, C., Calderone, S., Epifano, L., Vincenzo, A. D., Modarelli, F., Pampanelli, S., et al. (1993). Demonstration of a critical role for free fatty acids in mediating counterregulatory stimulation of gluconeogenesis and suppression of glucose utilization in humans. J. Clin. Invest. 92, 1617–1622. doi: 10.1172/JCI116746
Feng, Z., Hanson, R. W., Berger, N. A., and Trubitsyn, A. (2016). Reprogramming of energy metabolism as a driver of aging. Oncotarget 7, 15410–15420. doi: 10.18632/oncotarget.7645
Ferrannini, E., Natali, A., Camastra, S., Nannipieri, M., Mari, A., Adam, K.-P., et al. (2013). Early metabolic markers of the development of dysglycemia and type 2 diabetes and their physiological significance. Diabetes 62, 1730–1737. doi: 10.2337/db12-0707
Fiehn, O., Garvey, W. T., Newman, J. W., Lok, K. H., Hoppel, C. L., and Adams, S. H. (2010). Plasma metabolomic profiles reflective of glucose homeostasis in non-diabetic and type 2 diabetic obese African-American women. PLoS One 5:e15234. doi: 10.1371/journal.pone.0015234
Filla, L. A., Yuan, W., Feldman, E. L., Li, S., and Edwards, J. L. (2014). Global metabolomic and isobaric tagging capillary liquid chromatography-tandem mass spectrometry approaches for uncovering pathway dysfunction in diabetic mouse aorta. J. Proteome Res. 13, 6121–6134. doi: 10.1021/pr501030e
Floegel, A., Stefan, N., Yu, Z., Mühlenbruch, K., Drogan, D., Joost, H.-G., et al. (2013). Identification of serum metabolites associated with risk of type 2 diabetes using a targeted metabolomic approach. Diabetes 62, 639–648. doi: 10.2337/db12-0495
Flores-Guerrero, J. L., Groothof, D., Connelly, M. A., Otvos, J. D., Bakker, S. J. L., and Dullaart, R. P. F. (2019). Concentration of branched-chain amino acids is a strong risk marker for incident hypertension. Hypertension 74, 1428–1435. doi: 10.1161/HYPERTENSIONAHA.119.13735
Forouhi, N. G., and Wareham, N. J. (2014). Epidemiology of diabetes. Medicine 42, 698–702. doi: 10.1016/j.mpmed.2014.09.007
Friedrich, N., Budde, K., Suhre, K., Völker, U., John, U., Felix, S. B., et al. (2015). Sex differences in urine metabolites related with risk of diabetes using NMR spectroscopy: results of the study of health in pomerania. Metabolomics 11, 1405–1415. doi: 10.1007/s11306-015-0795-6
Frohnert, B. I., and Rewers, M. J. (2016). Metabolomics in childhood diabetes. Pediatr. Diabetes 17, 3–14. doi: 10.1111/pedi.12323
Fujiwara, M., Arifuku, K., Ando, I., and Nemoto, T. (2005). Pattern recognition analysis for classification of hypertensive model rats and diurnal variation using 1H-NMR spectroscopy of urine. Anal. Sci. 21, 1259–1262. doi: 10.2116/analsci.21.1259
Fullerton, J. N., and Gilroy, D. W. (2016). Resolution of inflammation: a new therapeutic frontier. Nat. Rev. Drug Discov. 15, 551–567. doi: 10.1038/nrd.2016.39
Gaetano, A. D., Mingrone, G., Castagneto, M., and Calvani, M. (1999). Carnitine increases glucose disposal in humans. J. Am. Coll. Nutr. 18, 289–295. doi: 10.1080/07315724.1999.10718866
Ghosh, A., Gao, L., Thakur, A., Parco, M. S., and Lai, C. W. K. (2017). Role of free fatty acids in endothelial dysfunction. J. Biomed. Sci. 24:50. doi: 10.1186/s12929-017-0357-5
Gonzalez-Calero, L., Martin-Lorenzo, M., Martínez, P. J., Baldan-Martin, M., Ruiz-Hurtado, G., Segura, J., et al. (2016). Hypertensive patients exhibit an altered metbolism. A specific metabolite signature in urine is able to predict albuminuria progression. Transl. Res. 178, 25–37. doi: 10.1016/j.trsl.2016.07.003
González-Núñez, D., Claria, J., Rivera, F., and Poch, E. (2000). Increased levels of 12(S)-HETE in patients with essential hypertension. Hypertension 37, 334–338. doi: 10.1161/01.hyp.37.2.334
Grapov, D., Johannes, F., Hwang, J., Poudel, A., Jo, J., Periwal, V., et al. (2015). Diabetes associated metabolomic perturbations in NOD mice. Metabolomics 11, 425–437. doi: 10.1007/s11306-014-0706-2
Gu, Y., Wang, X., Li, J., Zhang, Y., Zhong, H., Liu, R., et al. (2017). Analyses of gut microbiota and plasma bile acids enable stratification of patients for antidiabetic treatment. Nat. Commun. 8, 1785–1796. doi: 10.1038/s41467-017-01682-2
Guan, M. M., Xie, L. Y., Diao, C. F., Wang, N., Hu, W. Y., Zheng, Y. Q., et al. (2013). Systemic perturbations of key metabolites in diabetic rats during the evolution of diabetes studied by urine metabonomics. PLoS One 8:e60409. doi: 10.1371/journal.pone.0060409
Gustafson, B., Hammarstedt, A., Andersson, C. X., and Smith, U. (2007). Inflamed adipose tissue: a culprit underlying the metabolic syndrome and atherosclerosis. Arterioscler. Thromb. Vasc. Biol. 27, 2276–2283. doi: 10.1161/ATVBAHA.107.147835
Hausler, R. A., Astiarraga, B., Camastra, S., Accili, D., and Ferrannini, E. (2013). Human insulin resistance is associated with increased plasma levels of 12α-hydroxylated bile acids. Diabetes 62, 184–191. doi: 10.2337/db13-0639
Heinonen, S., Buzkova, J., Muniandy, M., Kaksonen, R., Ollikainen, M., Ismail, K., et al. (2015). Impaired mitochondrial biogenesis in adipose tissue in acquired obesity. Diabetes 64, 3135–3145. doi: 10.2337/db14-1937
Herman, A. G., and Moncada, S. (2005). Therapeutic potential of nitric oxide donors in the prevention and treatment of atherosclerosis. Eur. Heart J. 26, 1945–1955. doi: 10.1093/eurheartj/ehi333
Hernández-Alvarez, M. I., Díaz-Ramos, A., Berdasco, M., Cobb, J., Planet, E., Cooper, D., et al. (2017). Early-onset and classical forms of type 2 diabetes show impaired expression of genes involved in muscle branched-chain amino acids metabolism. Sci. Rep. 7, 13850–13861. doi: 10.1038/s41598-017-14120-6
Ho, J. E., Larson, M. G., Vasan, R. S., Ghorbani, A., Cheng, S., Rhee, E. P., et al. (2013). Metabolite profiles during oral glucose challenge. Diabetes 62, 2689–2698. doi: 10.2337/db12-0754
Hofmann, A. F. (1999). The continuing importance of bile acids in liver and intestinal disease. Arch. Intern. Med. 159, 2647–2658. doi: 10.1001/archinte.159.22.2647
Holmes, E., Loo, R. L., Stamler, J., Bictash, M., Yap, I. K. S., Chan, Q., et al. (2008). Human metabolic phenotype diversity and its association with diet and blood pressure. Nature 453, 396–400. doi: 10.1038/nature06882
Horita, S., Seki, G., Yamada, H., Suzuki, M., Koike, K., and Fujita, T. (2011). Insulin resistance, obesity, hypertension, and renal sodium transport. Int. J. Hypertens. 2011, 391762–391769. doi: 10.4061/2011/391762
Huffman, K. M., Slentz, C. A., Bateman, L. A., Thompson, D., Muehlbauer, M. J., Bain, J. R., et al. (2011). Exercise-induced changes in metabolic intermediates, hormones, and inflammatory markers associated with improvements in insulin sensitivity. Diabetes Care 34, 174–176. doi: 10.2337/dc10-0709
Hummasti, S., and Hotamisligil, G. S. (2010). Endoplasmic reticulum stress and inflammation in obesity and diabetes. Circ. Res. 107, 579–591. doi: 10.1161/CIRCRESAHA.110.225698
Hwang, G. S., Yang, J. Y., Ryu, D. H., and Kwon, T. H. (2010). Metabolic profiling of kidney and urine in rats with lithium-induced nephrogenic diabetes insipidus by (1)H-NMR-based metabonomics. Am. J. Physiol. Ren. Physiol. 298, F461–F470. doi: 10.1152/ajprenal.00389.2009
Iemitsu, M., Miyauchi, T., Maeda, S., Sakai, S., Fujii, N., Miyazaki, H., et al. (2003). Cardiac hypertrophy by hypertension and exercise training exhibits different gene expression of enzymes in energy metabolism. Hypertens. Res. 26, 829–837. doi: 10.1291/hypres.26.829
Johnson, C. H., Ivanisevic, J., and Siuzdak, G. (2016). Metabolomics: beyond biomarkers and towards mechanisms. Nat. Rev. Mol. Cell Biol. 17, 451–459. doi: 10.1038/nrm.2016.25
KanterEmail, M., Uysal, H., Karaca, T., and Sagmanligil, H. O. (2006). Depression of glucose levels and partial restoration of pancreatic beta-cell damage by melatonin in streptozotocin-induced diabetic rats. Arch. Toxicol. 80, 362–369. doi: 10.1007/s00204-005-0055-z
Kappel, B. A., Lehrke, M., Schütt, K., Artati, A., Adamski, J., Lebherz, C., et al. (2017). Effect of empagliflozin on the metabolic signature of patients with type 2 diabetes mellitus and cardiovascular disease. Circulation 136, 969–972. doi: 10.1161/CIRCULATIONAHA.117.029166
Khitan, Z., and Kim, D. H. (2013). Fructose: a key factor in the development of metabolic syndrome and hypertension. J. Nutr. Metab. 2013, 1–12. doi: 10.1155/2013/682673
Kim, F., Tysseling, K. A., Rice, J., Pham, M., Haji, L., Gallis, B. M., et al. (2005). Free fatty acid impairment of nitric oxide production in endothelial cells is mediated by IKKbeta. Arterioscler. Thromb. Vasc. Biol. 25, 989–994. doi: 10.1161/01.ATV.0000160549.60980.a8
Kitai, T., and Tang, W. H. W. (2017). The role and impact of gut microbiota in cardiovascular disease. Rev. Esp. Cardiol. 70, 799–800. doi: 10.1016/j.rec.2017.04.007
Kobori, H., Nangaku, M., Navar, L. G., and Nishiyama, A. (2007). The intrarenal renin-angiotensin system: from physiology to the pathobiology of hypertension and kidney disease. Pharmacol. Rev. 59, 251–287. doi: 10.1124/pr.59.3.3
Koeth, R. A., Wang, Z., Levison, B. S., Buffa, J. A., Org, E., Sheehy, B. T., et al. (2013). Intestinal microbiota metabolism of L-carnitine, a nutrient in red meat, promotes atherosclerosis. Nat. Med. 19, 576–585. doi: 10.1038/nm.3145
Konstantinova, S. V., Tell, G. S., Vollset, S. E., Nygård, O., Bleie, Ø., and Ueland, P. M. (2008). Divergent associations of plasma choline and betaine with components of metabolic syndrome in middle age and elderly men and women. J. Nutr. 138, 914–920. doi: 10.1093/jn/138.5.914
Kotronen, A., Velagapudi, V. R., Yetukrti, L., Makkonen, J., Taskinen, M. R., Orešič, M., et al. (2009). Serum saturated fatty acids containing triacylglycerols are better markers of insulin resistance than total serum triacylglycerol concentrations. Diabetologia 52, 684–690. doi: 10.1007/s00125-009-1282-2
Landsberg, L., Aronne, L. J., Beilin, L. J., Burke, V., Igel, L. I., Lloyd-Jones, D., et al. (2013). Obesity-related hypertension: pathogenesis, cardiovascular risk, and treatment: a position paper of The Obesity Society and the American Society of Hypertension. J. Clin. Hyperten. 15, 14–33. doi: 10.1111/jch.12049
Lastra, G., Syed, S., Kurukulasuriya, L. R., Manrique, C., and Sowers, J. R. (2014). Type 2 diabetes mellitus and hypertension: an update. Endocrinol. Metab. Clin. N. Am. 43, 103–122. doi: 10.1016/j.ecl.2013.09.005
Lazar, A. G., Romanciuc, F., Socaciu, M. A., and Socaciu, C. (2015). Bioinformatics tools for metabolomic data processing and analysis using untargeted liquid chromatography couple with mass spectrometry. Bull. UASVM Ani. Sci. Biotech. 72, 103–115. doi: 10.15835/buasvmcn-asb:11536
Li, Y. F., Jackson, K. L., Stern, J. E., Rabeler, B., and Patal, K. P. (2006). Interaction between glutamate and GABA systems in the integration of sympathetic outflow by the paraventricular nucleus of the hypothalamus. Am. J. Physiol. Heart Circ. Physiol. 291, H2847–H2856. doi: 10.1152/ajpheart.00625.2005
Li, B., Selmi, C., Tang, R., Gershwin, M., and Ma, X. (2018a). The microbiome and autoimmunity: a paradigm from the gut-liver axis. Cell. Mol. Immunol. 15, 595–609. doi: 10.1038/cmi.2018.7
Li, M., Wang, X., Aa, J., Qin, W., Zha, W., Ge, Y., et al. (2013). GC/TOFMS analysis of metabolites in serum and urine reveals metabolic perturbation of TCA cycle in db/db mice involved in diabetic nephropathy. Am. J. Physiol. Ren. Physiol. 304, F1317–F1324. doi: 10.1152/ajprenal.00536.2012
Li, X. S., Wang, Z., Cajka, T., Buffa, J. A., Nemet, I., Hurd, A. G., et al. (2018b). Untargeted metabolomics identifies trimethyllysine, a TMAO-producing nutrient precursor, as a predictor of incident cardiovascular disease risk. JCI Insight 3, e99096–e99113. doi: 10.1172/jci.insight.99096
Liu, Y., Chen, T., Qiu, Y., Cheng, Y., Cao, Y., Zhao, A., et al. (2011). An ultrasonication-assisted extraction and derivatization protocol for GC/TOFMS-based metabolite profiling. Anal. Bioanal. Chem. 400, 1405–1417. doi: 10.1007/s00216-011-4880-z
Liu, A., Chu, Y., Wang, X., Yu, R., Jiang, H., Li, Y., et al. (2018). Serum metabolomics study based on LC-MS and antihypertensive effect of Uncaria on spontaneously hypertensive rats. Evid. Based Complement. Alternat. Med. 2018:9281946. doi: 10.1155/2018/9281946
Liu, R., Hong, J., Xu, X., Feng, Q., Zhang, D., Gu, Y., et al. (2017b). Gut microbiome and serum metabolome alterations in obesity and after weight-loss intervention. Nat. Med. 23, 859–868. doi: 10.1038/nm.4358
Liu, J., Semiz, S., van der Lee, S. J., van der Spek, A., Verhoeven, A., van Klinken, J. B., et al. (2017a). Metabolomics based markers predict type 2 diabetes in a 14-year follow-up study. Metabolomics 13:104. doi: 10.1007/s11306-017-1239-2
Liu, X., Wang, Y., Gao, R., Xing, Y., Li, X., and Wang, Z. (2017c). Serum metabolomic response to exercise training in spontaneously hypertensive rats. J. Am. Soc. Hypertens. 11, 428–436. doi: 10.1016/j.jash.2017.05.003
Liu, L., Wang, M., Yang, X., Bi, M., Na, L., Niu, Y., et al. (2013). Fasting serum lipid and dehydroepiandrosterone sulfate as important metabolites for detecting isolated postchallenge diabetes: serum metabolomics via ultra-high-performance LC-MS. Clin. Chem. 59, 1338–1348. doi: 10.1373/clinchem.2012.200527
Lu, Y., Jiye, A., Wang, G., Hao, H., Huang, Q., Yan, B., et al. (2008). Gas chromatography/time-of-flight mass spectrometry based metabonomic approach to differentiating hypertension- and age-related metabolic variation in spontaneously hypertensive rats. Rapid Commun. Mass Spectrom. 22, 2882–2888. doi: 10.1002/rcm.3670
Mahony, S. M. O., Clarke, G., Borre, Y. E., Dinan, T. G., and Cryan, J. F. (2015). Serotonin, tryptophan metabolism and the brain-gut-microbiome axis. Behav. Brain Res. 277, 32–48. doi: 10.1016/j.bbr.2014.07.027
Mäkinen, V.-P., Tnkkynen, T., Soininen, P., Peltola, T., Kangas, A. J., Forsblom, C., et al. (2012). Metabolic diversity of progressive kidney disease in 325 patients with type 1 diabetes (the FinnDiane Study). J. Proteome Res. 11, 1782–1790. doi: 10.1021/pr201036j
Manrique, C., Lastra, G., Gardner, M., and Sowers, J. R. (2009). The renin angiotensin aldosterone system in hypertension: roles of insulin resistance and oxidative stress. Med. Clin. North Am. 93, 569–582. doi: 10.1016/j.mcna.2009.02.014
Marasciulo, F. L., Montagnani, M., and Potenza, M. A. (2006). Endothelin-1: the yin and yang on vascular function. Curr. Med. Chem. 13, 1655–1665. doi: 10.2174/092986706777441968
Martin-Lorenzo, M., Martinez, P. J., Baldan-Martin, M., Ruiz-Hurtado, G., Prado, J. C., Segura, J., et al. (2017). Citric acid metabolism in resistant hypertension underlying mechanisms and metabolic prediction of treatment response. Hypertension 70, 1049–1056. doi: 10.1161/HYPERTENSIONAHA.117.09819
Matheis, P. A. M. F., Schneeberger, M., Kerner, Z., Jové, V., and Mucida, D. (2020). Microbiota-modulated CART+ enteric neurons autonomously regulate blood glucose. Science 370, 314–321. doi: 10.1126/science.abd6176
Mazepa, R. C., Cuevas, M. J., Collado, P. S., and González-Gallego, J. (1999). Melatonin increases muscle and liver glycogen content in nonexercised and exercised rats. Life Sci. 66, 153–160. doi: 10.1016/S0024-3205(99)00573-1
Mell, B., Jala, V. R., Mathew, A. V., Byun, J., Waghulde, H., Zhang, Y., et al. (2015). Evidence for a link between gut microbiota and hypertension in the Dahl rat. Physiol. Genomics 47, 187–197. doi: 10.1152/physiolgenomics.00136.2014
Menni, C., Fauman, E., Erte, I., Perry, J. R., Kastenmuller, G., Shin, S. Y., et al. (2013). Biomarkers for type 2 diabetes and impaired fasting glucose using a nontargeted metabolomics approach. Diabetes 62, 4270–4276. doi: 10.2337/db13-0570
Michaliszyn, S. F., Sjaarda, L. A., Mihalik, S. J., Lee, S., Bacha, F., Chace, D. H., et al. (2012). Metabolomic profiling of amino acids and β-cell function relative to insulin sensitivity in youth. J. Clin. Endocrinol. Metab. 97, E2119–E2124. doi: 10.1210/jc.2012-2170
Mihalik, S. J., Michaliszyn, S. F., Heras, J. D. L., Bacha, F., Lee, S., Chace, D. H., et al. (2012). Metabolomic profiling of fatty acid and amino acid metabolism in youth with obesity and type 2 diabetes: evidence for enhanced mitochondrial oxidation. Diabetes Care 35, 605–611. doi: 10.2337/DC11-1577
Mingrone, G. (2004). Carnitine in type 2 diabetes. Ann. N. Y. Acad. Sci. 1033, 99–107. doi: 10.1196/annals.1320.009
Montagnani, M., Chen, H., Barr, V. A., and Quon, M. J. (2001). Insulin-stimulated activation of eNOS is independent of Ca2+ but requires phosphorylation by Akt at Ser(1179). J. Biol. Chem. 276, 30392–30398. doi: 10.1074/jbc.M103702200
Montagnani, M., Golovchenko, I., Kim, I., Koh, G. Y., Goalstone, M. L., Mundhekar, A. N., et al. (2002). Inhibition of phosphatidylinositol 3-kinase enhances mitogenic actions of insulin in endothelial cells. J. Biol. Chem. 277, 1794–1799. doi: 10.1074/jbc.M103728200
Monteiro, M. S., Carvalho, M., Bastos, M. L., and Guedes de Pinho, P. (2013). Metabolomics analysis for biomarker discoverty: advances and challenges. Curr. Med. Chem. 20, 257–271. doi: 10.2174/092986713804806621
Mouton, A. J., Li, X., Hall, M. E., and Hall, J. E. (2020). Obesity, hypertension, and cardiac dysfunction: novel roles of immunometabolism in macrophage activation and inflammation. Circ. Res. 126, 789–806. doi: 10.1161/CIRCRESAHA.119.312321
Mukai, Y., Wang, C.-Y., Rikitake, Y., and Liao, J. K. (2007). Phosphatidylinositol 3-kinase/protein kinase Akt negatively regulates plasminogen activator inhibitor type 1 expression in vascular endothelial cells. Am. J. Physiol. Heart Circ. Physiol. 292, H1937–H1942. doi: 10.1152/ajpheart.00868.2006
Muniyappa, R., Iantorno, M., and Quon, M. J. (2008). An integrated view of insulin resistance and endothelial dysfunction. Endocrinol. Metab. Clin. N. Am. 37, 685–711. doi: 10.1016/j.ecl.2008.06.001
Muniyappa, R., Montagnani, M., Koh, K. K., and Quon, M. J. (2007). Cardiovascular actions of insulin. Endocr. Rev. 28, 463–491. doi: 10.1210/er.2007-0006
Muoio, D. M., and Newgard, C. B. (2008). Molecular and metabolic mechanisms of insulin resistance and β-cell failure in type 2 diabetes. Nat. Rev. Mol. Cell Biol. 9, 193–205. doi: 10.1038/nrm2327
Murfitt, S. A., Zaccone, P., Wang, X., Acharjee, A., Sawyer, Y., Koulman, A., et al. (2017). A metabolomics and lipidomics study of mouse models of type 1 diabetes highlights divergent metabolism in purine and tryptophan metabolism prior to disease on-set. J. Proteome Res. 17, 946–960. doi: 10.1021/acs.jproteome.7b00489
Neis, E. P. J. G., Dejong, C. H. C., and Rensen, S. S. (2015). The role of microbial amino acid metabolism in host metabolism. Nutrients 7, 2930–2946. doi: 10.3390/nu7042930
Nemet, I., Saha, P. P., Gupta, N., Zhu, W., Romano, K. A., Skye, S. M., et al. (2020). A cardiovascular disease-linked gut microbial metabolite acts via adrenergic receptors. Cell 180, 862–877. doi: 10.1016/j.cell.2020.02.016
Newgard, C. B. (2012). Interplay between lipids and branched-chain amino acids in development of insulin resistance. Cell Metab. 15, 606–614. doi: 10.1016/j.cmet.2012.01.024
Nicholson, J. K., Holmes, E., Kinross, J., Burcelin, R., Gibson, G., and Pettersson, W. J. S. (2012). Host-gut microbiota metabolic interactions. Science 336, 1262–1267. doi: 10.1126/science.1223813
Nikolic, S. B., Edwards, L. M., Karpievitch, Y. V., Wilson, R., Horne, J., Adams, M. J., et al. (2016). Serum metabolic profile predicts adverse central haemodynamics in patients with type 2 diabetes mellitus. Acta Diabetol. 53, 367–375. doi: 10.1007/s00592-015-0802-4
O’Connell, T. M. (2013). The complex role of branched chain amino acids in diabetes and cancer. Meta 3, 931–945. doi: 10.3390/metabo3040931
Ordway, R. W., Singer, J. J., and Walsh, J. V. Jr. (1991). Direct regulation of ion channels by fatty acids. Trends Neurosci. 14, 96–100. doi: 10.1016/0166-2236(91)90069-7
Palmer, N. D., Stevens, R. D., Antinozzi, P. A., Anderson, A., Bergman, R. N., Wagenknecht, L. E., et al. (2015). Metabolomic profile associated with insulin resistance and conversion to diabetes in the insulin resistance atherosclerosis study. J. Clin. Endocrinol. Metab. 100, E463–E468. doi: 10.1210/jc.2014-2357
Pålsson-McDermott, E. M., and O’Neill, L. A. J. (2020). Targeting immunometabolism as an anti-inflammatory strategy. Cell Res. 30, 300–314. doi: 10.1038/s41422-020-0291-z
Pedersen, H. K., Gudmundsdottir, V., Nielsen, H. B., Hyotylainen, T., Nielsen, T., Jensen, B. A. H., et al. (2016). Human gut microbes impact host serum metabolome and insulin sensitivity. Nature 535, 376–381. doi: 10.1038/nature18646
Pena, M. J., Heerspink, H. J. L., Hellemons, M. E., Friedrich, T., Dallmann, G., Lajer, M., et al. (2014). Urine and plasma metabolites predict the development of diabetic nephropathy in individuals with type 2 diabetes mellitus. Diabet. Med. 31, 1138–1147. doi: 10.1111/dme.12447
Petrie, J. R., Guzik, T. J., and Touyz, R. M. (2018). Diabetes, hypertension, and cardiovascular disease: clinical insights and vascular mechanisms. Can. J. Cardiol. 34, 575–584. doi: 10.1016/j.cjca.2017.12.005
Pinti, M. V., Fink, G. K., Hathaway, Q. A., Durr, A. J., Kunovac, A., and Hollander, J. M. (2019). Mitochondrial dysfunction in type 2 diabetes mellitus: an organ-based analysis. Am. J. Physiol. Endocrinol. Metab. 316, E268–E285. doi: 10.1152/ajpendo.00314.2018
Pluznick, J. L. (2013). Renal and cardiovascular sensory receptors and blood pressure regulation. Am. J. Physiol. Ren. Physiol. 305, F439–F444. doi: 10.1152/ajprenal.00252.2013
Polak-Iwaniuk, A., Harasim-Symbor, E., Gołaszewska, K., and Chabowski, A. (2019). How hypertension affects heart metabolism. Front. Physiol. 10:435. doi: 10.3389/fphys.2019.00435
Psichas, A., Sleeth, M., Murphy, K., Brooks, L., Bewick, G., Hanyaloglu, A., et al. (2015). The short chain fatty acid propionate stimulates GLP-1 and PYY secretion via free fatty acid receptor 2 in rodents. Int. J. Obes. 39, 424–429. doi: 10.1038/ijo.2014.153
Pueyo, M. E., Gonzalez, W., Nicoletti, A., Savoie, F., Arnal, J.-F., and Michel, J.-B. (2000). Angiotensin II stimulates endothelial vascular cell adhesion molecule-1 via nuclear factor-kappaB activation induced by intracellular oxidative stress. Arterioscler. Thromb. Vasc. Biol. 20, 645–651. doi: 10.1161/01.ATV.20.3.645
Pulakat, L., DeMarco, V. G., Whaley-Connell, A., and Sowers, J. R. (2011). The impact of overnutrition on insulin metabolic signaling in the heart and the kidney. Cardiorenal Med. 1, 102–112. doi: 10.1159/000327140
Purnell, J. Q., Hokanson, J. E., Marcovina, S. M., Steffes, M. W., Cleary, P. A., and Brunzell, J. D. (1998). Effect of excessive weight gain with intensive therapy of type 1 diabetes on lipid levels and blood pressure: results from the DCCT. Diabetes control and complications trial. JAMA 280, 140–146. doi: 10.1001/jama.280.2.140
Qi, Y., Aranda, J. M., Rodriguez, V., Raizada, M. K., and Pepine, C. J. (2015). Impact of antibiotics on arterial blood pressure in a patient with resistant hypertension - A case report. Int. J. Cardiol. 201, 157–158. doi: 10.1016/j.ijcard.2015.07.078
Qiu, G., Zheng, Y., Wang, H., Sun, J., Ma, H., Xiao, Y., et al. (2016). Plasma metabolomics identified novel metabolites associated with risk of type 2 diabetes in two prospective cohorts of Chinese adults. Int. J. Epidemiol. 45, 1507–1516. doi: 10.1093/ije/dyw221
Quan, X., Wang, J., Liang, C., Zheng, H., and Zhang, L. (2015). Melatonin inhibits tunicamycin-induced endoplasmic reticulum stress and insulin resistance in skeletal muscle cells. Biochem. Biophys. Res. Commun. 463, 1102–1107. doi: 10.1016/j.bbrc.2015.06.065
Rajesh, M., Bátkai, S., Kechrid, M., Mukhopadhyay, P., Lee, W.-S., Horváth, B., et al. (2012). Cannabinoid 1 receptor promotes cardiac dysfunction, oxidative stress, inflammation, and fibrosis in diabetic cardiomyopathy. Diabetes 61, 716–727. doi: 10.2337/db11-0477
Rewers, M. F. S. M., Selby, J., Howard, G., Jinagouda, S., Fahmi, S., Zaccaro, D., et al. (2004). Insulin resistance and hypertension. Hypertension 43, 1324–1331. doi: 10.3109/10641969909061017
Rinschen, M. M., Ivanisevic, J., Giera, M., and Siuzdak, G. (2019). Identification of bioactive metabolites using activity metabolomics. Nat. Rev. Mol. Cell Biol. 20, 353–367. doi: 10.1038/s41580-019-0108-4
Roman, R. J., and Fan, F. (2018). 20-HETE hypertension and beyond. Hypertension 72, 12–18. doi: 10.1161/HYPERTENSIONAHA.118.10269
Rotroff, D., Shahin, M., Gurley, S., Zhu, H., Motsinger-Reif, A., Meisner, M., et al. (2015). Pharmacometabolomic assessments of atenolol and hydrochlorothiazide treatment reveal novel drug response phenotypes. CPT Pharmacometrics Syst. Pharmacol. 4, 669–679. doi: 10.1002/psp4.12017
Ruiz-Canela, M., Guasch-Ferré, M., Toledo, E., Clish, C. B., Razquin, C., Liang, L., et al. (2018). Plasma branched chain/aromatic amino acids, enriched Mediterranean diet and risk of type 2 diabetes: case-cohort study within the PREDIMED trial. Diabetologia 61, 1560–1571. doi: 10.1007/s00125-018-4611-5
Russell, D. W. (2003). The enzymes, regulation, and genetics of bile acid synthesis. Annu. Rev. Biochem. 72, 137–174. doi: 10.1146/annurev.biochem.72.121801.161712
Salek, R. M., Maguire, M. L., Bentley, E., Rubtsov, D. V., Hough, T., Cheeseman, M., et al. (2007). A metabolomic comparison of urinary changes in type 2 diabetes in mouse, rat, and human. Physiol. Genomics 29, 99–108. doi: 10.1152/physiolgenomics.00194.2006
Samuel, B. S., Shaito, A., Motoike, T., Rey, F. E., Backhed, F., Manchester, J. K., et al. (2008). Effects of the gut microbiota on host adiposity are modulated by the short-chain fatty-acid binding G protein-coupled receptor, Gpr41. Proc. Natl. Acad. Sci. U. S. A. 105, 16767–16772. doi: 10.1073/pnas.0808567105
Savolainen, O., Fagerberg, B., Vendelbo Lind, M., Sandberg, A. S., Ross, A. B., and Bergstrom, G. (2017a). Biomarkers for predicting type 2 diabetes development-can metabolomics improve on existing biomarkers? PLoS One 12:e0177738. doi: 10.1371/journal.pone.0177738
Savolainen, O., Lind, M. V., Bergström, G., Fagerberg, B., Sandberg, A.-S., and Ross, A. (2017b). Biomarkers of food intake and nutrient status are associated with glucose tolerance status and development of type 2 diabetes in older Swedish women. Am. J. Clin. Nutr. 106, 1302–1310. doi: 10.3945/ajcn.117.152850
Sayin, S. I., Wahlström, A., Felin, J., Jäntti, S., Marschall, H.-U., Bamberg, K., et al. (2013). Gut microbiota regulates bile acid metabolism by reducing the levels of tauro-beta-muricholic acid, a naturally occurring FXR antagonist. Cell Metab. 17, 225–235. doi: 10.1016/j.cmet.2013.01.003
Schutta, M. H. (2007). Diabetes and hypertension: epidemiology of the relationship and pathophysiology of factors associated with these comorbid conditions. Can. J. Cardiol. 2, 124–130. doi: 10.1016/j.cjca.2017.12.005
Schwartz, R., Osborne-Lawrence, S., Hahner, L., Gibson, L. L., Gormley, A. K., Vongpatanasin, W., et al. (2007). C-reactive protein downregulates endothelial NO synthase and attenuates reendothelialization in vivo in mice. Circ. Res. 100, 1452–1459. doi: 10.1161/01.RES.0000267745.03488.47
Sekhar, R. V., McKay, S. V., Patel, S. G., Guthikonda, A. P., Reddy, V. T., Balasubramanyam, A., et al. (2011). Glutathione synthesis is diminished in patients with uncontrolled diabetes and restored by dietary supplementation with cysteine and glycine. Diabetes Care 34, 162–167. doi: 10.2337/dc10-1006
She, M., Deng, X., Guo, Z., Laudon, M., Hu, Z., Liao, D., et al. (2009). NEU-P11, a novel melatonin agonist, inhibits weight gain and improves insulin sensitivity in high-fat/high-sucrose-fed rats. Pharmacol. Res. 59, 248–253. doi: 10.1016/j.phrs.2009.01.005
She, M., Hou, H., Wang, Z., Zhang, C., Laudon, M., and Yin, W. (2014). Melatonin rescues 3T3-L1 adipocytes from FFA-induced insulin resistance by inhibiting phosphorylation of IRS-1 on Ser307. Biochimie 103, 126–130. doi: 10.1016/j.biochi.2014.05.001
Shi, H., Zhang, B., Abo-Hamzy, T., Nelson, J. W., Ambati, C. S. R., Petrosino, J. F., et al. (2021). Restructuring the gut microbiota by intermittent fasting lowers blood pressure. Circ. Res. 128, 1240–1254. doi: 10.1161/CIRCRESAHA.120.318155
Shieh, J. M., Wu, H. T., Cheng, K. C., and Cheng, J. T. (2009). Melatonin ameliorates high fat diet-induced diabetes and stimulates glycogen synthesis via a PKCzeta-Akt-GSK3beta pathway in hepatic cells. J. Pineal Res. 47, 339–344. doi: 10.1111/j.1600-079X.2009.00720.x
Shulaev, V. (2006). Metabolomics technology and bioinformatics. Brief. Bioinform. 7, 128–139. doi: 10.1093/bib/bbl012
Soleimani, M. (2015). Insulin resistance and hypertension: new insights. Kidney Int. 87, 497–499. doi: 10.1038/ki.2014.392
Sowers, J. R., Epstein, M., and Frohlich, E. D. (2001). Diabetes, hypertension and vardiovascular disease. Hypertension 37, 1053–1059. doi: 10.1161/01.HYP.37.4.1053
Stančáková, A., Civelek, M., Saleem, N. K., Soininen, P., Kangas, A. J., Cederberg, H., et al. (2012). Hyperglycemia and a common variant of GCKR are associated with the levels of eight amino acids in 9369 finnish men. Diabetes 61, 1895–1902. doi: 10.2337/db11-1378
Stanley, W. C., Lopaschuk, G. D., and McCormack, J. G. (1997). Regulation of energy substrate metabolism in the diabetic heart. Cardiovasc. Res. 34, 25–33. doi: 10.1016/S0008-6363(97)00047-3
Suhre, K., Meisinger, C., Doring, A., Altmaier, E., Belcredi, P., Gieger, C., et al. (2010). Metabolic footprint of diabetes: a multiplatform metabolomics study in an epidemiological setting. PLoS One 5:e13953. doi: 10.1371/journal.pone.0013953
Sury, M. D., Frese-Schaper, M., Mühlemann, M. K., Schulthess, F. T., Blasiga, I. E., Täuber, M. G., et al. (2006). Evidence that N-acetylcysteine inhibits TNF-alpha-induced cerebrovascular endothelin-1 upregulation via inhibition of mitogen- and stress-activated protein kinase. Free Radic. Biol. Med. 41, 1372–1383. doi: 10.1016/j.freeradbiomed.2006.07.016
Taguchi, A., and White, M. F. (2008). Insulin-like signaling, nutrient homeostasis, and life span. Annu. Rev. Physiol. 70, 191–212. doi: 10.1146/annurev.physiol.70.113006.100533
Tang, W. H. W., and Hazen, S. L. (2017). The gut microbiome and its role in cardiovascular diseases. Curr. Opin. Cardiol. 135, 1008–1010. doi: 10.1097/HCO.0000000000000445
Tang, W., Zhang, B., Wang, H., Li, M., Wang, H., Liu, F., et al. (2019). Improved skeletal muscle energy metabolism relates to the recovery of β cell function by intensive insulin therapy in drug naïve type 2 diabtes. Diabetes Metab. Res. Rev. 35:e3177. doi: 10.1002/dmrr.3177
Teodoro, B. G., Baraldi, F. G., Sampaio, I. H., Bomfim, L. H. M., Queiroz, A. L., Passos, M. A., et al. (2014). Melatonin prevents mitochondrial dysfunction and insulin resistance in rat skeletal muscle. J. Pineal Res. 57, 155–167. doi: 10.1111/jpi.12157
Teymoori, F., Asghari, G., Mirmiran, P., and Azizi, F. (2018). High dietary intake of aromatic amino acids increases risk of hypertension. J. Am. Soc. Hypertens. 12, 25–33. doi: 10.1016/j.jash.2017.11.004
The DCCT Research Group (1988). Weight gain associated with intensive therapy in the diabetes control and complications trial. Diabetes Care 11, 567–573. doi: 10.2337/diacare.11.7.567
Thevaranjan, N., Puchta, A., Schulz, C., Naidoo, A., Szamosi, J. C., Verschoor, C. P., et al. (2017). Age-associated microbial dysbiosis promotes intestinal permeability, systemic inflammation, and macrophage dysfunction. Cell Host Microbe 21, 455–466. doi: 10.1016/j.chom.2017.03.002
Thomas, C., Pellicciari, R., Pruzanski, M., Auwerx, J., and Schoonjans, K. (2008). Targeting bile-acid signalling for metabolic diseases. Nat. Rev. Drug Discov. 7, 678–693. doi: 10.1038/nrd2619
Thorp, A. A., and Schlaich, M. P. (2015). Relevance of sympathetic nervous system activation in obesity and metabolic syndrome. J. Diabetes Res. 2015:341583. doi: 10.1155/2015/341583
Tian, Y., Jiang, F., Li, Y., Jiang, H., Chu, Y., Zhu, L., et al. (2018). Evaluation of the anti-hypertensive effect of Tengfu Jiangya tablet by combination of UPLC-Q-exactive-MS-based metabolomics and iTRAQ-based proteomics technology. Biomed. Pharmacother. 100, 324–334. doi: 10.1016/j.biopha.2018.02.025
Tolhurst, G., Heffron, H., Lam, Y. S., Parker, H. E., Habib, A. M., Diakoqiannaki, E., et al. (2012). Short-chain fatty acids stimulate glucagon-like peptide-1 secretion via the G-protein-coupled receptor FFAR2. Diabetes 61, 364–371. doi: 10.2337/db11-1019
Tremaroli, V., Karlsson, F., Werling, M., Ståhlman, M., Kovatcheva-Datchary, P., Olbers, T., et al. (2015). Roux-en-Y gastric bypass and vertical banded gastroplasty induce long-term changes on the human gut microbiome contributing to fat mass regulation. Cell Metab. 22, 228–238. doi: 10.1016/j.cmet.2015.07.009
Tripathi, A., Debelius, J., Brenner, D. A., Karin, M., Loomba, R., Schnabl, B., et al. (2018). The gut-liver axis and the intersection with the microbiome. Nat. Rev. Gastroenterol. Hepatol. 15, 397–411. doi: 10.1038/s41575-018-0011-z
van Deventer, C. A., Lindeque, Z., Rensburg, P. J. J., Malan, L., van der Westhuizen, F. H., and Louw, R. (2015). Use of metabolomics to elucidate the metabolic perturbation associated with hypertension in a black south African male cohort: the SABPA study. J. Am. Soc. Hypertens. 9, 104–114. doi: 10.1016/j.jash.2014.11.007
Vangaveti, V., Baune, B. T., and Kennedy, R. L. (2010). Hydroxyoctadecadienoic acids: novel regulators of macrophage differentiation and atherogenesis. Ther. Adv. Endocrinol. Metab. 1, 51–60. doi: 10.1177/2042018810375656
Vincent, M. A., Clerk, L. H., Lindner, J. R., Klibanov, A. L., Clark, M. G., Rattigan, S., et al. (2004). Microvascular recruitment is an early insulin effect that regulates skeletal muscle glucose uptake in vivo. Diabetes 53, 1418–1423. doi: 10.2337/diabetes.53.6.1418
Vincent, M. A., Montagnani, M., and Quon, M. J. (2003). Molecular and physiologic actions of insulin related to production of nitric oxide in vascular endothelium. Curr. Diab. Rep. 3, 279–288. doi: 10.1007/s11892-003-0018-9
Voss, T. S., Vendelbo, M. H., Kampmann, U., Pedersen, S. B., Nielsen, T. S., Johannsen, M., et al. (2017). Effects of insulin-induced hypoglycaemia on lipolysis rate, lipid oxidation and adipose tissue signalling in human volunteers: a randomised clinical study. Diabetologia 60, 143–152. doi: 10.1007/s00125-016-4126-x
Walford, G. A., Davis, J., Warner, A. S., Ackerman, R. J., Billings, L. K., Chamarthi, B., et al. (2013). Branched chain and aromatic amino acids change acutely following two medical therapies for type 2 diabetes mellitus. Metabolism 62, 1–13. doi: 10.1016/j.metabol.2013.07.003
Wallis, M. G., Smith, M. E., Kolka, C. M., Zhang, L., Richards, S. M., Rattigan, S., et al. (2005). Acute glucosamine-induced insulin resistance in muscle in vivo is associated with impaired capillary recruitment. Diabetologia 48, 2131–2139. doi: 10.1007/s00125-005-1887-z
Wang, Y., Deng, G., and Davies, K. (2016). Metabolomics reveals differential changes in the energy generating pathways of the detrusor and urothelium caused by diabetes. J. Urol. 195, E414–E414. doi: 10.1016/j.juro.2016.02.1240
Wang, T. J., Larson, M. G., Vasan, R. S., Cheng, S., Rhee, E. P., McCabe, E., et al. (2011). Metabolite profiles and the risk of developing diabetes. Nat. Med. 17, 448–453. doi: 10.1038/nm.2307
Wang, X., Zhang, L., Youker, K., Zhang, M., Wang, J., LeMaire, S. A., et al. (2006). Free fatty acids inhibit insulin signaling-stimulated endothelial nitric oxide synthase activation through upregulating PTEN or inhibiting Akt kinase. Diabetes 55, 2301–2310. doi: 10.2337/db05-1574
Weiss, G. A., and Hennet, T. (2017). Mechanisms and consequences of intestinal dysbiosis. Cell. Mol. Life Sci. 74, 2959–2977. doi: 10.1007/s00018-017-2509-x
Wewalka, M., Patti, M.-E., Barbato, C., Houten, S. M., and Goldfine, A. B. (2014). Fasting serum taurine-conjugated bile acids are elevated in type 2 diabetes and do not change with intensification of insulin. J. Clin. Endocrinol. Metab. 99, 1442–1451. doi: 10.1210/jc.2013-3367
Wishart, D. S. (2016). Emerging applications of metabolomics in drug discovery and precision medicine. Nat. Rev. Drug Discov. 15, 473–484. doi: 10.1038/nrd.2016.32
Withers, D. J., Gutierrez, J. S., Towery, H., Burks, D. J., Previs, J.-M. R. S., Zhang, Y., et al. (1998). Disruption of IRS-2 causes type 2 diabetes in mice. Nature 391, 900–904. doi: 10.1038/36116
Wu, T., Xie, G., Ni, Y., Liu, T., Yang, M., Wei, H., et al. (2015). Serum metabolite signatures of type 2 diabetes mellitus complications. J. Proteome Res. 14, 447–456. doi: 10.1186/s12967-019-2096-8
Würtz, P., Soininen, P., Soininen, P., Rönnemaa, T., Lehtimäki, T., Kähönen, M., et al. (2013). Branched-chain and aromatic amino acids are predictors of insulin resistance in young adults. Diabetes Care 36, 648–655. doi: 10.2337/dc12-0895
Xu, J., Morita, I., Ikeda, K., Miki, T., and Yamori, Y. (2007). C-reactive protein suppresses insulin signaling in endothelial cells: role of spleen tyrosine kinase. Mol. Endocrinol. 21, 564–573. doi: 10.1210/me.2006-0354
Yandrapalli, S., Pal, S., Nabors, C., and Aronow, W. S. (2018). Drug treatment of hypertension in older patients with diabetes mellitus. Expert. Opin. Pharmacother. 19, 633–642. doi: 10.1080/14656566.2018.1456529
Yang, Q., Graham, T. E., Mody, N., Preitner, F., Peroni, O. D., Zabolotny, J. M., et al. (2005). Serum retinol binding protein 4 contributes to insulin resistance in obesity and type 2 diabetes. Nature 436, 356–362. doi: 10.1038/nature03711
Yang, T., Richards, E. M., Pepine, C. J., and Raizada, M. K. (2018). The gut microbiota and the brain-gut-kidney axis in hypertension and chronic kidney disease. Nat. Rev. Nephrol. 14, 442–456. doi: 10.1038/s41581-018-0018-2
Yang, T., Santisteban, M. M., Rodriquez, V., Li, E., Ahmari, N., Carvajal, J. M., et al. (2015). Gut dysbiosis is linked to hypertension. Hypertension 65, 1331–1340. doi: 10.1161/HYPERTENSIONAHA.115.05315
Yokoi, N., Beppu, M., Yoshida, E., Hoshikawa, R., Hidaka, S., Matsubara, T., et al. (2015). Identification of putative biomarkers for prediabetes by metabolome analysis of rat models of type 2 diabetes. Metabolomics 11, 1277–1286. doi: 10.1007/s11306-015-0784-9
Yu, D., Moore, S. C., Matthews, C. E., Xiang, Y., Zhang, X., Gao, Y., et al. (2016). Plasma metabolomic profiles in association with type 2 diabetes risk and prevalence in Chinese adults. Metabolomics 12, 1–13. doi: 10.1007/s11306-015-0890-8
Zeng, G., and Quon, M. J. (1996). Insulin-stimulated production of nitric oxide is inhibited by wortmannin. Direct measurement in vascular endothelial cells. J. Clin. Invest. 98, 894–898. doi: 10.1172/JCI118871
Zhang, B., Hu, S., Baskin, E., Patt, A., Siddiqui, J. K., and Mathé, E. A. (2018). RaMP: a comprehensive relational database of metabolomics pathways for pathway enrichment analysis of genes and metabolites. Meta 8:16. doi: 10.3390/metabo8010016
Zhang, L., Wheatley, C. M., Richards, S. M., Barrett, E. J., Clark, M. G., and Rattigan, S. (2003). TNF-α acutely inhibits vascular effects of physiological but not high insulin or contraction. Am. J. Physiol. Endocrinol. Metab. 285, E654–E660. doi: 10.1152/ajpendo.00119.2003
Zhao, L., Zhang, F., Ding, X., Wu, G., Lam, Y. Y., Wang, X., et al. (2018). Gut bacteria selectively promoted by dietary fibers alleviate type 2 diabetes. Science 359, 1151–1156. doi: 10.1126/science.aao5774
Zheng, Y., Yamada, H., Sakamoto, K., Horita, S., Kunimi, M., Endo, Y., et al. (2005). Roles of insulin receptor substrates in insulin-induced stimulation of renal proximal bicarbonate absorption. J. Am. Soc. Nephrol. 16, 2288–2295. doi: 10.1681/ASN.2005020193
Zheng, Y., Yu, B., Alexander, D., Mosley, T. H., Heiss, G., Nettleton, J. A., et al. (2013). Metabolomics and incident hypertension among blacks: the atherosclerosis risk in communities study. Hypertension 62, 398–403. doi: 10.1161/HYPERTENSIONAHA.113.01166
Zhu, W., Gregory, J. C., Org, E., Buffa, J. A., Gupta, N., Wang, Z., et al. (2016). Gut microbial metabolite TMAO enhances platelet hyperreactivity and thrombosis risk. Cell 165, 111–124. doi: 10.1016/j.cell.2016.02.011
Zubcevic, J., Jun, J. Y., Kim, S., Perez, P. D., Afzal, A., Shan, Z., et al. (2014). Altered inflammatory response is associated with an impaired autonomic input to the bone marrow in the SHR. Hypertension 63, 542–550. doi: 10.1161/HYPERTENSIONAHA.113.02722
Glossary
Keywords: diabetes mellitus, the pathogenesis of hypertension, perturbated metabolites, metabolic function, connected metabolic mechanism
Citation: Ning Z, Song Z, Wang C, Peng S, Wan X, Liu Z and Lu A (2021) How Perturbated Metabolites in Diabetes Mellitus Affect the Pathogenesis of Hypertension? Front. Physiol. 12:705588. doi: 10.3389/fphys.2021.705588
Edited by:
Hanjun Wang, University of Nebraska Medical Center, United StatesReviewed by:
Shyam Sundar Nandi, University of Nebraska Medical Center, United StatesBaojian Xue, The University of Iowa, United States
Copyright © 2021 Ning, Song, Wang, Peng, Wan, Liu and Lu. This is an open-access article distributed under the terms of the Creative Commons Attribution License (CC BY). The use, distribution or reproduction in other forums is permitted, provided the original author(s) and the copyright owner(s) are credited and that the original publication in this journal is cited, in accordance with accepted academic practice. No use, distribution or reproduction is permitted which does not comply with these terms.
*Correspondence: Aiping Lu, bGFwNjQwNjc2MTFAMTI2LmNvbQ==; Zhenli Liu, emhlbmxpX2xpdUBzaW5hLmNvbQ==
†These authors have contributed equally to this work