- 1School of Kinesiology and Health Studies, Queen’s University, Kingston, ON, Canada
- 2Faculty of Medicine, University of Ottawa, Ottawa, ON, Canada
Background: Many reports describe statistical approaches for estimating interindividual differences in trainability and classifying individuals as “responders” or “non-responders.” The extent to which studies in the exercise training literature have adopted these statistical approaches remains unclear.
Objectives: This systematic review primarily sought to determine the extent to which studies in the exercise training literature have adopted sound statistical approaches for examining individual responses to exercise training. We also (1) investigated the existence of interindividual differences in trainability, and (2) tested the hypothesis that less conservative thresholds inflate response rates compared with thresholds that consider error and a smallest worthwhile change (SWC)/minimum clinically important difference (MCID).
Methods: We searched six databases: AMED, CINAHL, EMBASE, Medline, PubMed, and SportDiscus. Our search spanned the aerobic, resistance, and clinical or rehabilitation training literature. Studies were included if they used human participants, employed standardized and supervised exercise training, and either: (1) stated that their exercise training intervention resulted in heterogenous responses, (2) statistically estimated interindividual differences in trainability, and/or (3) classified individual responses. We calculated effect sizes (ESIR) to examine the presence of interindividual differences in trainability. We also compared response rates (n = 614) across classification approaches that considered neither, one of, or both errors and an SWC or MCID. We then sorted response rates from studies that also reported mean changes and response thresholds (n = 435 response rates) into four quartiles to confirm our ancillary hypothesis that larger mean changes produce larger response rates.
Results: Our search revealed 3,404 studies, and 149 were included in our systematic review. Few studies (n = 9) statistically estimated interindividual differences in trainability. The results from these few studies present a mixture of evidence for the presence of interindividual differences in trainability because several ESIR values lay above, below, or crossed zero. Zero-based thresholds and larger mean changes significantly (both p < 0.01) inflated response rates.
Conclusion: Our findings provide evidence demonstrating why future studies should statistically estimate interindividual differences in trainability and consider error and an SWC or MCID when classifying individual responses to exercise training.
Systematic Review Registration: [website], identifier [registration number].
Introduction
In 1999, the seminal Health, Risk Factors, Exercise Training, and Genetics (HERITAGE) Family Study reported individual cardiorespiratory fitness responses ranging from approximately −100 to +1,000 ml/min following 20 weeks of supervised and standardized aerobic exercise training (Bouchard et al., 1999). Early studies following HERITAGE continued to examine individual responses to exercise training by (1) interpreting a wide range of observed responses as evidence that exercise training causes interindividual variability and (2) classifying individuals as “responders” or “non-responders” if their observed response was above or below zero, respectively [reviewed in Williamson et al. (2017), Ross R. et al. (2019), Bonafiglia et al. (2020)]. In 2015, biostatisticians raised concerns with these approaches and have advocated for more rigorous statistical approaches when estimating interindividual variability and classifying individual responses (Atkinson and Batterham, 2015; Hecksteden et al., 2015; Hopkins, 2015). Despite many subsequent reviews echoing these concerns (reviews listed in Supplementary Table S1), we are aware of several studies published in the past year that did not adopt these statistical approaches (Marsh et al., 2020; Thomas et al., 2020; Vellers et al., 2020; Wang et al., 2020). Because no study has systematically reviewed the approaches used to examine individual responses, it is unclear whether these recent studies are representative of the exercise training literature.
Estimating interindividual variability requires partitioning the variability in outcome measurements caused by exercise training per se, herein referred to as interindividual differences in trainability, from the variability caused by random measurement error and within-subject variability (Bonafiglia et al., 2019a). Within-subject variability refers to real physiological responses resulting from changes in behavioral or environmental factors such as diet, sleep, and physical activity outside of a standardized exercise intervention (Bonafiglia et al., 2019a). In 2015, biostatisticians recommended statistical approaches that estimate interindividual differences in trainability by partitioning error or within-subject variability (Atkinson and Batterham, 2015; Hecksteden et al., 2015; Hopkins, 2015). Since then, many reviews have used data simulations or theoretical arguments to emphasize the importance of partitioning error or within-subject variability to encourage researchers to adopt these statistical approaches (Williamson et al., 2017; Swinton et al., 2018; Atkinson et al., 2019; Bonafiglia et al., 2019a; Ross R. et al., 2019; Voisin et al., 2019; Chrzanowski-Smith et al., 2020; Dankel and Loenneke, 2020). What remains unclear is whether the exercise training literature has adopted the recommended statistical approaches of biostatisticians. If the literature has not adopted these approaches, additional lines of evidence (i.e., beyond data simulations and theoretical arguments) may be required to persuade researchers to adopt a statistical approach that partitions error or within-subject variability when attempting to examine individual response heterogeneity.
With respect to classifying individual responses, labeling someone as a “non-responder” to exercise should be avoided because individuals can: (1) demonstrate individual patterns of response across a range of outcomes [e.g., the VO2max of an individual may “respond” positively while their body fat percentage may “not respond” (Barber et al., 2021)], (2) respond differently following different exercise doses (Bonafiglia et al., 2016; Montero and Lundby, 2017; Marsh et al., 2020), or (3) respond differently to repeated exposure to the same training intervention (Del Giudice et al., 2020). Further, given the difficulty in delineating changes caused by exercise vs. behavioral or environmental factors, “responders” and “non-responders” should not be interpreted as individuals who responded or did not respond to exercise per se (Swinton et al., 2018). Instead, “responders” and “non-responders” should be interpreted as individuals who did or did not experience benefit following the completion of an exercise training intervention (Swinton et al., 2018). To reduce the risk of misclassifying individuals who did not benefit as “responders,” many reports have recommended that “responders” should be classified as individuals whose observed change in a given outcome exceeds the smallest worthwhile change (SWC) or a minimum clinically important difference (MCID) after accounting for random measurement error (Bonafiglia et al., 2018, 2019b; Hecksteden et al., 2018; Swinton et al., 2018; Ross R. et al., 2019). In support of these recommendations, we (Schulhauser et al., 2020) and others (Hecksteden et al., 2018) found that thresholds not considering error and/or a SWC or MCID inflate “response rates” compared with more conservative thresholds that consider both error and a SWC or MCID. However, recent studies have classified “responders” and “non-responders” using non-conservative thresholds (Marsh et al., 2020; Thomas et al., 2020). Whether this observation is representative of the classification approaches used in the exercise training literature is unknown. Further, given that previous reports (Hecksteden et al., 2018; Schulhauser et al., 2020) included few outcomes (n = 6 and 1) and small sample sizes (n = 40 and 84), corroborating the inverse relationship between threshold conservativeness and response rates with a larger dataset may provide more compelling evidence to convince researchers to consider error and a SWC or MCID when classifying individual responses to exercise training.
The primary purpose of the present review was to determine the extent to which studies in the exercise training literature have adopted approaches to statistically estimate interindividual differences in trainability and consider error and an SWC or MCID when classifying individual responses. We performed a systematic review that spanned the aerobic, resistance, and clinical/rehabilitation training literature. We developed our search criteria to only include studies that examined individual responses (i.e., analyzed individual responses or commented on response heterogeneity), and our analyses, therefore, do not contain data from studies that did not consider individual responses.
Materials and Methods
This systematic review followed the Preferred Reporting Items for Systematic Reviews and Meta-Analyses (PRISMA) checklist (see Supplementary Table S2). The study selection process was conducted using Covidence’s systematic review software (Veritas Health Innovation Ltd., Australia). Table 1 includes a list of definitions of individual response terms that we use throughout this review.
Eligibility Criteria
Studies were included in the systematic review if they met all of the following inclusion criteria: (1) were an original, published research study (including novel re-analyses, which refer to secondary analyses of datasets where individual responses were not examined in primary reports), (2) used human participants, (3) employed a minimum of 2 weeks (or six sessions) of standardized and supervised exercise training, and (4) examined individual responses to exercise training by (i) stating that exercise training resulted in heterogeneous responses without using a statistical approach to estimate interindividual differences in trainability (e.g., commenting on response heterogeneity or a wide range of individual responses in their results, discussion, or conclusions); (ii) adopting a statistical approach to estimate interindividual differences in trainability (approaches listed in our Supplementary Table S3) (Atkinson and Batterham, 2015; Hecksteden et al., 2015, 2018; Hopkins, 2015; Dankel and Loenneke, 2020); and/or (iii) classifying individual responses. The latter criteria are not necessarily mutually exclusive. For example, Hecksteden et al. (2018) adopted the standard deviation of individual response (SDIR) to estimate interindividual differences in trainability (criteria ii) and classified individual responses (criteria iii). Conversely, Yu et al. (2020) adopted the SDIR but did not classify (criteria ii only), and Bonafiglia et al. (2018) did not adopt the SDIR but did classify (criteria iii only). Studies were excluded if they did not meet all of the inclusion criteria, or if the manuscript was not available (i.e., conference abstract only) or not in English.
Literature Search and Study Selection
We conducted a literature search in AMED, CINAHL, EMBASE, Medline, PubMed, and SportDiscus on March 28, 2020. A second, identical up-to-date search took place on January 6, 2021. The search strategy incorporated two main concepts: exercise training and individual response. A complete list of synonyms or related terms for these two main concepts was combined with “OR” (see Supplementary Table S4 for full list), and the search strategy combined the two separate synonym lists with “AND.” Titles and abstracts were extracted from the database searches, and Covidence automatically removed duplicates.
Study selection followed a two-step process and was independently completed by JTB and NP. BJG resolved disagreements (n = 2 total). First, titles and abstracts were screened to identify studies that appeared to meet eligibility criteria. Second, full texts were downloaded for articles that passed title and abstract screening to determine their eligibility. Studies removed during full-text screening were assigned a reason for exclusion. Final analyses included studies that passed both levels of study selection. We used the Cochrane Collaboration Risk of Bias Assessment Tool (Higgins et al., 2011) to assess the risk of bias. We reviewed the protocol and/or primary publications to evaluate the risk of bias for studies that included re-analyses of previously published data.
Data Extraction
JTB and NP performed data extraction using a predetermined data collection template, which included the variables provided in our Supplementary Table S5 along with response rates, mean changes, and response thresholds. These two reviewers met to compare extracted data and resolve discrepancies. JTB and NP dichotomously categorized all included studies based on whether they did or did report using a statistical approach (Atkinson and Batterham, 2015; Hecksteden et al., 2015, 2018; Hopkins, 2015; Dankel and Loenneke, 2020) to estimate interindividual differences in trainability. Because adopting a statistical approach is needed to partition the sources of variation, this dichotomous categorization allowed us to determine how many studies may have overlooked the confounding influence of random measurement error and within-subject variability on response variability. This dichotomous categorization is further warranted because studies not adopting a statistical approach, and thus not accounting for the confounding sources of variation, risk erroneously interpreting variability in observed responses as evidence of interindividual differences in trainability. After this initial categorization, JTB and NP then sorted studies that classified individual responses into three categories based on whether they considered an error and/or an SWC or MCID (see Table 2 for details).
We extracted basic study characteristics such as training protocols, participant characteristics, and measured outcomes from all included studies (see Supplementary Table S5 for study characteristics). For studies that statistically estimated interindividual differences in trainability, we extracted sample sizes and standard deviations (SD) of baseline values and change scores. We used WebPlotDigitizer (Rohatgi, 2018) to calculate SDs of change scores from two studies (Steele et al., 2017; Hammond et al., 2019) that did not report these values but presented individual data in their figures. For studies that classified individual responses, we extracted mean changes, thresholds used to classify “responders,” and response rates. For the purposes of this review, response rate refers to the proportion of “responders” for a given outcome following a specific exercise or control condition (Table 1).
Data Analysis
In addition to our primary analysis, we conducted two analyses to demonstrate limitations with not statistically estimating interindividual differences in trainability or considering error and an SWC or MCID. First, we calculated estimates of interindividual differences in trainability from included studies that have adopted a statistical approach (Supplementary Table S3) to determine how many outcomes provided evidence of variability caused by exercise training per se. Second, we examined the impact of threshold conservativeness on response rates. Details for these two analyses are provided in the following sections.
Interindividual Differences in Trainability
For studies that statistically estimated interindividual differences in trainability, we calculated the SDIR, a statistic that estimates the presence of interindividual differences in trainability (see Bonafiglia et al., 2019a for a detailed explanation), for each outcome using the following equation (Atkinson and Batterham, 2015; Hopkins, 2015):
where SDEX and SDCTRL represent the SD of change scores from the exercise (EX) and control group (CTRL), respectively. Positive SDIR values (i.e., SDEX < SDCTRL) suggest that interindividual differences in trainability exist. An SDIR cannot be calculated if SDCTRL exceeds SDEX because you cannot take the square root of a negative number (Eq. 1). In these instances, researchers can either report not being able to calculate an SDIR, or they can switch SDCTRL with SDEX in Eq. 1 and report the value as a negative SDIR. Both incalculable and negative SDIR values should be interpreted as a lack of evidence for interindividual differences in trainability (Bonafiglia et al., 2019a). For studies that had multiple exercise groups, we amalgamated the data of the exercise groups according to chapter 7.7.3.8 in the Cochrane Handbook (Higgins et al., 2019) to calculate one SDEX value and thus one SDIR value for each outcome in each study. The standard error (SE) for each SDIR value was calculated to construct 90% confidence intervals (CIs) using the following equations (Hopkins, 2015; Hecksteden et al., 2018):
where nEX and nCTRL represent sample sizes for the EX and CTRL groups, respectively. To visually present these data from different outcomes in one figure, we standardized SDIR values by calculating unitless effect sizes (denoted as ESIR) using the following equation (Hopkins, 2015):
where SDBSL.Pooled represents the pooled SD of baseline values from the EX and CTRL groups. Upper and lower CI limits were also divided by SDBSL.Pooled to construct 90% CIs for ESIR values (Hopkins, 2015).
The interpretation of interindividual differences in trainability for each outcome was based on the positions of 90% CIs: CIs laying fully above zero suggested that interindividual differences in trainability were present, whereas CIs crossing or laying fully below zero indicated a lack of evidence for interindividual differences in trainability.
Analysis of Variances on Response Rates
We performed one-way analysis of variance (ANOVA) comparing response rates across the three response classification categories outlined in Table 2. We performed an ANOVA that compared response rates across every outcome and another ANOVA on the most commonly reported outcome. The ANOVA on the most commonly reported outcome was performed to determine whether the variance introduced by comparing response rates across different outcomes impacted our ability to detect significance. We excluded studies that used quantiles because these approaches guarantee a fixed percentage of responders or non-responders and therefore do not provide estimates of response rates. To determine whether standardized mean changes impacted our ANOVA on all outcomes, we also performed an ANCOVA with a standardized mean () changes inputted as a covariate. Specifically, we first extracted available pre and post-training means and SDs for each outcome to calculate Cohen’s d values using the following equation:
where dav refers to a Cohen’s d value for a within-subject standardized mean change (Lakens, 2013), and pooled SD was calculated as the standard deviation of pre values plus the standard deviation of post values divided by two. Because many (130 out of 298) of the sample sizes included in our ANCOVA were less than 20, we then converted dav values to Hedge’s g values (gav) using the following equation:
Additionally, our ancillary hypothesis investigated whether response rates were a function of mean change relative to the response threshold. We based this hypothesis on findings from two of our recent studies: (1) larger mean changes produce larger response rates at a given response threshold (Bonafiglia et al., 2021b), and (2) smaller response thresholds produce larger response rates at a given mean change (Schulhauser et al., 2020). We tested this hypothesis using outcomes with reported response rates, mean changes, and response thresholds; outcomes could not be included if any of these three parameters were not reported. Data from both exercise and non-exercising control groups were included in this analysis. Our dependent variable was response rates (in percentage), and our independent variable was calculated as the mean change divided by the response threshold. This approach standardized our independent variable and thus allowed us to compare response rates across different outcomes. We could not include response rates from studies using zero-based thresholds because mean changes cannot be divided by a response threshold of zero. We then sorted outcomes into four response rate quartiles: response rates less than or equal to (1) 100%, (2) 75%, (3) 50%, or (4) 25%. Following this, we used a one-way ANOVA to compare standardized mean changes across these response rate quartiles. We identified outliers within each quartile as z-scores that were either >2.58 or <−2.58, and we removed these data before running our ANOVA.
For all ANOVAs, significant main effects were followed by Bonferroni post hoc tests. These statistical tests were performed on GraphPad Prism version 9 with the ANCOVA performed on SPSS version 26. All data are presented as means ± standard deviation.
Results
Study Selection
Figure 1 presents a flow diagram of the study selection process. The literature search retrieved 3,404 studies, and Covidence removed 1,120 duplicates. Two thousand two hundred eighty-four studies entered title and abstract screening, and 2,036 were deemed irrelevant and were subsequently excluded. Full texts were then downloaded for 248 studies, and 99 were excluded (reasons provided in Figure 1), leaving a total of 149 included studies. Study details, measured outcomes, analytical approaches, and participant characteristics for these 149 studies can be found in the Supplementary Table S5. Most included studies had an unclear-high risk of bias (results presented in Supplementary Table S6). For ease of viewing, in-text references for all 149 included studies are provided at the end of this manuscript (see Appendix). Table 3 presents study characteristics and analysis categories (categories outlined in Table 2) for these 149 studies.
Timeline of Studies Examining Individual Responses to Exercise Training
We created two timelines of the studies included in our analysis (Figure 2). Figure 2A includes all studies and is sorted based on whether studies used a statistical approach to estimate interindividual differences in trainability. The number of studies examining individual responses to exercise training has increased substantially since 1999, when the findings from the HERITAGE Family Study were published, with the majority (72.5%; 108/149) being published within the last 5 years (i.e., 2015 onward). The paper by Atkinson and Batterham (2015) is highlighted in Figure 2A because it describes the SDIR approach and, to our knowledge, is the first article in the exercise literature outlining how to statistically estimate the presence of interindividual differences in trainability. It was therefore unsurprising that no study before 2015 statistically estimated interindividual differences in trainability (Figure 2A). However, strikingly few studies (∼9.5%; 8/84 studies) published in 2017 onward [i.e., after Atkinson et al.’s (2019) SDIR paper] statistically estimated interindividual differences in trainability. Seven of these studies (Steele et al., 2017; Williamson et al., 2017, 2018; Bonafiglia et al., 2019a; Hammond et al., 2019; Walsh et al., 2020; Yu et al., 2020) used the SDIR, and one study explored different SDIR approaches using a variety of statistical parameters (Hecksteden et al., 2018). The 2016 study by Leifer et al. (2016) used Levene’s tests to compare variability in observed responses between control and exercise groups – an approach that follows the same principles as the SDIR (Bonafiglia et al., 2019a).
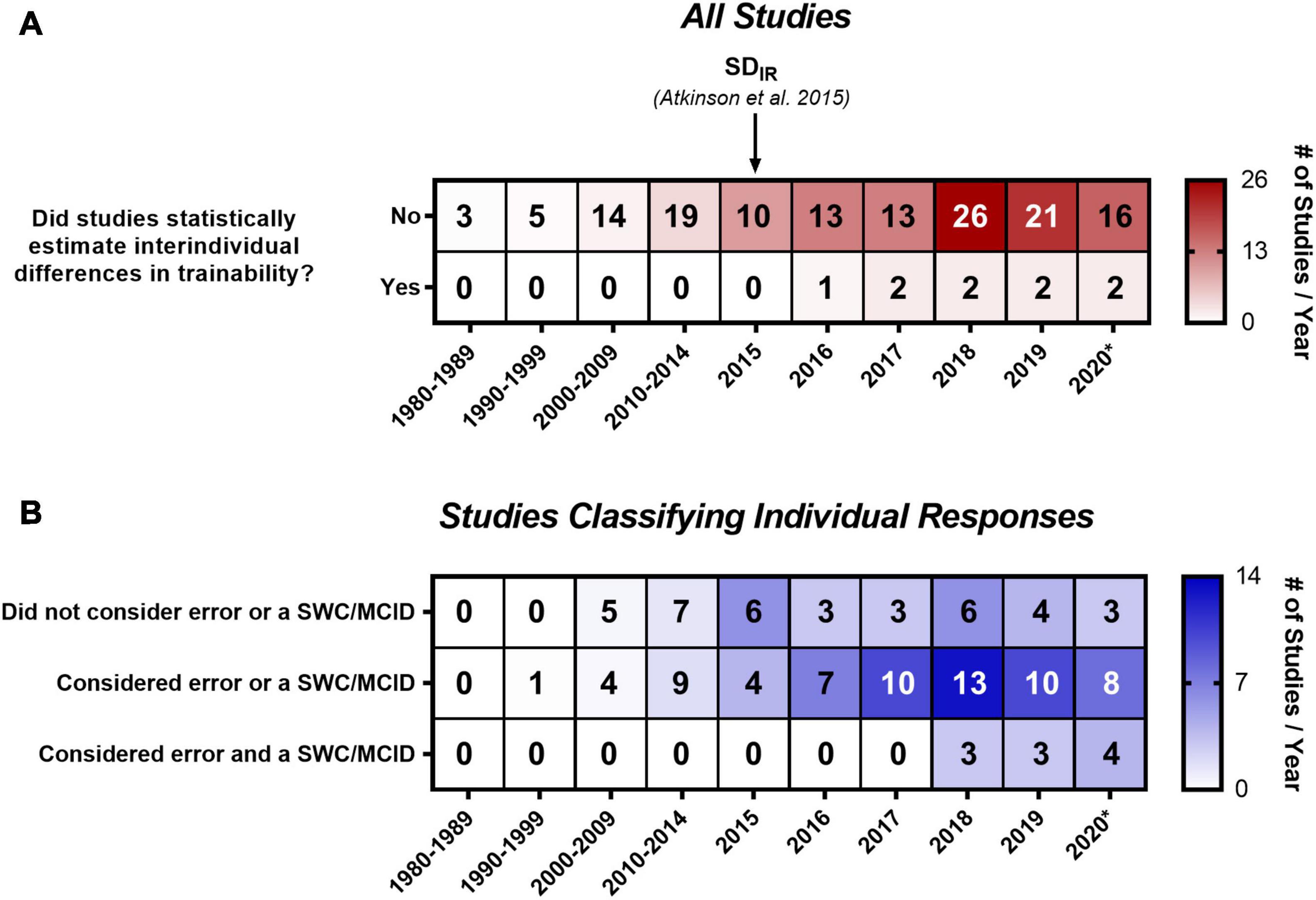
Figure 2. Timeline and heatmap of the 149 studies included in our systematic review. Numbers refer to a total number of studies in each cell, whereas shading depicts the total number of studies divided by the number of years included in that column. (A) Includes all studies sorted by whether studies used a statistical approach to estimate interindividual differences in trainability, and (B) includes the 116 studies that classified individual responses sorted by the classification categories outlined in Table 2. 2020* includes studies published in 2020 and up to the updated literature search (January 6, 2021). MCID, minimum clinically important difference; SDIR, the standard deviation of individual responses; SWC, smallest worthwhile change. (B) Only contains 113 of the 116 studies that classified individual responses because three studies did not report how individuals were classified (Hubal et al., 2005; Hagstrom and Denham, 2018; Peltonen et al., 2018).
A percentage of 77.9% (116/149) of all included studies classified individual responses, and a timeline of these studies sorted by the three categories outlined in Table 2 is presented in Figure 2B. 31.9% of studies (37/116) classified individuals using an approach that did not consider error or an SWC or MCID (e.g., zero-based thresholds or quantiles). 56.9% of studies (66/116) considered error (66.7%; 44/66) or an SWC or MCID (33.3%; 22/66), and the most common approach in this category (37.9%; 25/66) was classifying responders as individuals whose observed response exceeded a threshold of two times the typical error. Only 8.6% of studies (10/116) considered both error and an SWC or MCID. The most common approach (90%; 9/10) in this last category was classifying responders as individuals with confidence intervals, built using the typical error of measurement and constructed around observed responses, that lay fully above an SWC or MCID. Supplementary Table S5 provides more information on the specific classification approaches used in each study. Three studies (Hubal et al., 2005; Hagstrom and Denham, 2018; Peltonen et al., 2018) were not categorized because they did not report enough information on how they classified individual responses.
Studies Adopting Approaches for Estimating Interindividual Differences in Trainability
We were unable to calculate ESIR values from three of the nine studies that statically estimated interindividual differences in trainability because they did not report SD of baseline measures and/or change scores (Williamson et al., 2017, 2018; Bonafiglia et al., 2019a). All three of these studies included novel re-analyses of previously published data. Williamson et al. (2017) reported greater variability in VO2max responses in a control group compared with an exercise group, the 2018 meta-analysis of Williamson et al. (2018) demonstrated trivial interindividual differences in trainability for body weight, and we reported moderate-large variability in behavioral factors (e.g., dietary habits and sedentary time) following a controlled exercise intervention (Bonafiglia et al., 2019a).
We calculated ESIR values in the remaining six studies. These values with 90% CIs along with basic details regarding participant characteristics and training modes are presented in Figure 3. Combining data across the three aerobic training groups from Hammond et al. (2019) resulted in positive ESIR values for body mass and waist circumference. However, we can only conclude that interindividual differences in trainability were present for body mass because the 90% CI for waist circumference crossed zero. The aerobic, resistance, and aerobic plus resistance groups were also combined in the study Walsh et al. (2020). Responses in body composition and cardiometabolic health from Walsh et al. (2020) revealed mixed evidence of interindividual differences in trainability because ESIR 90% CIs lay above, below, or crossed zero. ESIR estimates from the Leifer et al. (2016). The Leifer et al. (2016) study also presented a mixture of 90% CI positions for indices of cardiometabolic health. The ESIR values from Hecksteden et al. (2018) and Yu et al. (2020), who measured fitness parameters following aerobic training, were all positive but had large 90% CIs that crossed zero. These large CIs were likely attributable to the small sample sizes of these two studies. Interestingly, only the study by Steele et al. (2017), which examined strength responses to resistance training, revealed consistent evidence of interindividual differences in trainability as all ESIR 90% CIs lay above zero.
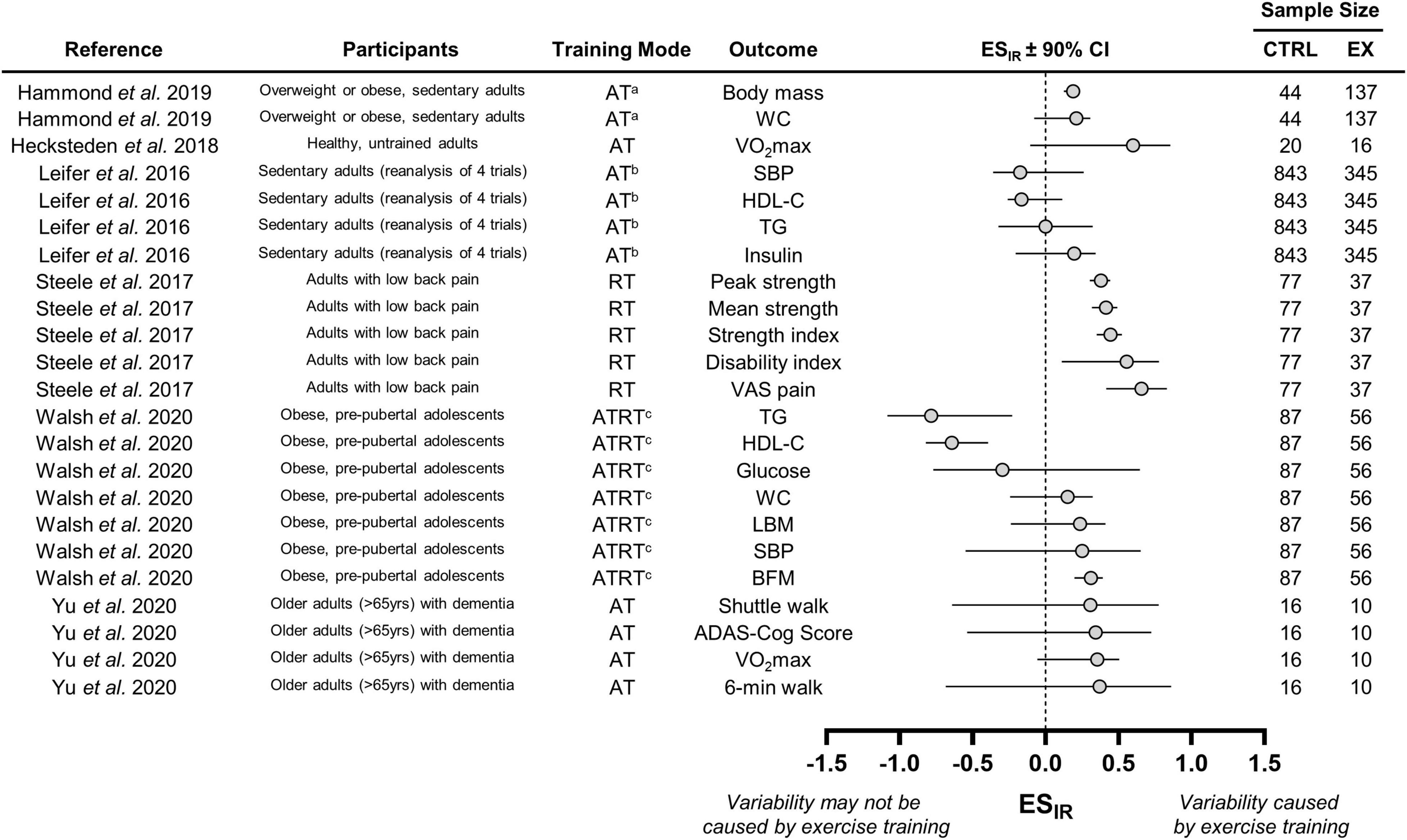
Figure 3. Forest plot of the studies that statistically estimated interindividual differences in trainability. CTRL, control group; EX, exercise training group; WC, waist circumference; VO2max; maximal oxygen uptake; SBP, systolic blood pressure; HDL-C, high-density lipoprotein cholesterol; TG, triglyceride; VAS, visual analog scale; LBM, lean body mass; BFM, body fat mass; ADAS-cog, Alzheimer’s disease assessment scale-cognitive subscale. aWe combined data from three aerobic training groups (see section “Interindividual Differences in Trainability”); bData from four trials combined by Leifer et al. (2016); cWe combined data from the three training groups (see section “Interindividual Differences in Trainability”), which included an aerobic, resistance, and combined training group.
Analysis of Variances on Response Rates
We obtained response rates for 614 outcomes from the 116 studies that classified individual responses. 71, 491, and 52 response rates were obtained from zero-based thresholds, approaches that considered error or an SWC or MCID, or both error and an SWC or MCID, respectively. Our one-way ANOVA with all 614 response rates (Figure 4A; left panel) was significant (p < 0.01) with zero-based thresholds producing a significantly (p < 0.01) higher mean response rate (71.22 ± 18.09%) compared with approaches that considered one of (50.53 ± 31.08%) or both error and an SWC or MCID (45.49 ± 20.52%). Our second ANOVA was performed on VO2max because this outcome had the most response rates (n = 75) compared with other outcomes (next three outcomes with the most response rates: various strength measures, n = 51; waist circumference, n = 20; body weight, n = 17). The one-way ANOVA on VO2max response rates (Figure 4A; right panel) was also significant (p < 0.05) with zero-based thresholds resulting in a significantly (p < 0.05) higher mean response rate (78.42 ± 12.98%) compared with approaches that considered both error and an SWC or MCID (46.50 ± 20.85%). We extracted 298 Hedge’s g values, and our ANCOVA and associated post hoc tests remained significant indicating that standardized mean changes did not confound the relationship between classification category and response rate (Figure 4A).
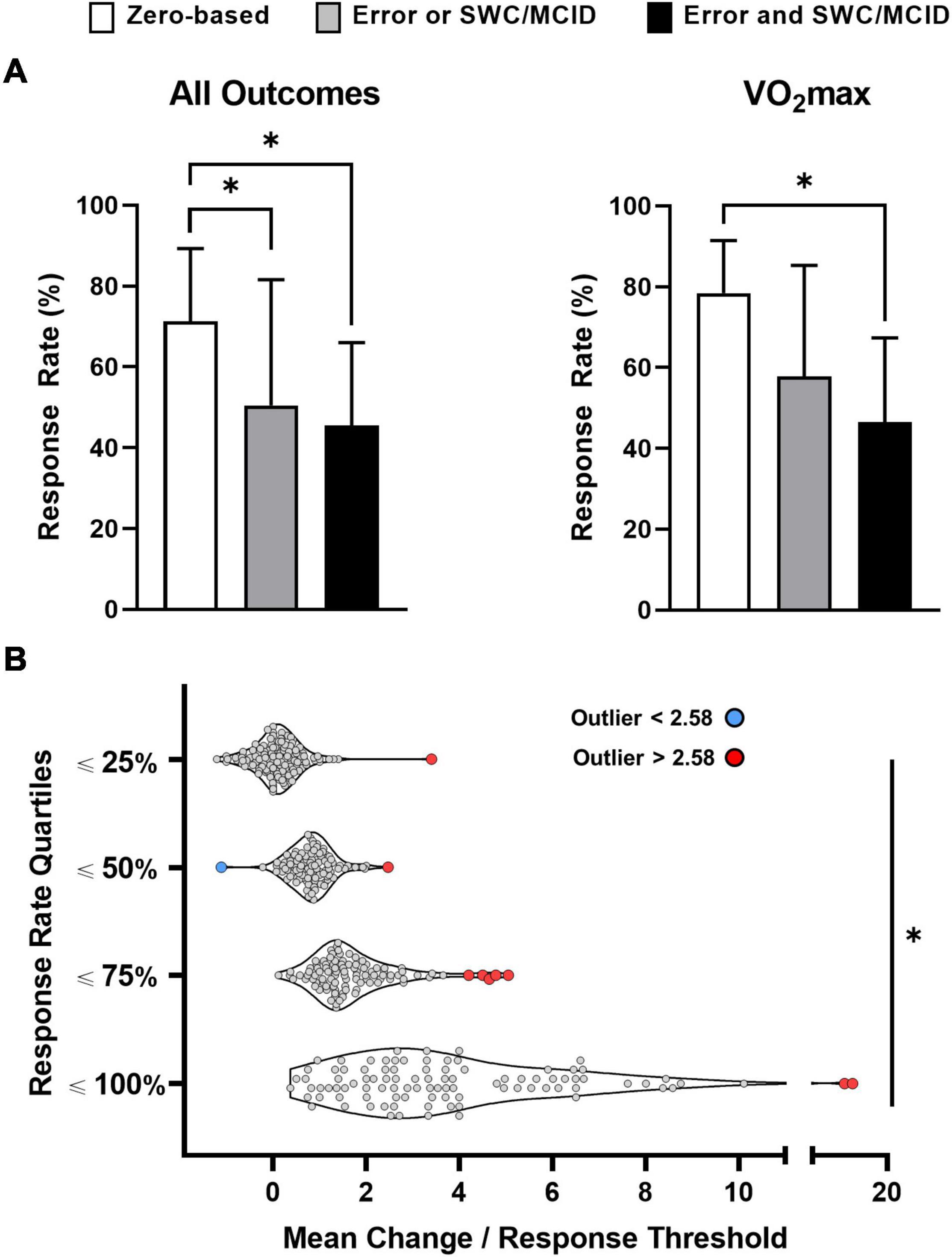
Figure 4. Impact of classification approach [A; n = 614 (all outcomes); n = 75 (maximal oxygen uptake, VO2max)] and mean change (B) on response rates. Table 2 outlines the classification categories outlined in (A). *Significant at p < 0.01.
Response rates, mean changes, and response thresholds were reported for 435 of the 614 outcomes. Our ancillary analysis (Figure 4B) on these 435 outcomes revealed significant differences in standardized mean changes (mean change divided by response threshold) across each quartile: ≤25% = 0.09 ± 0.46; ≤50% = 0.83 ± 0.41; ≤75% = 1.58 ± 0.69; ≤100% = 3.62 ± 2.19 (ANOVA and all post hoc p values < 0.01). We identified ten outlying data points, and only one lay below the mean (blue data point; all other outliers in red; Figure 4B). The two outliers in the upper quartile (response rates ≤100%) had very large standardized mean changes, and both data points represent strength gains (leg press one-repetition maximum) from two resistance training groups in the same study (Barbalho et al., 2017). The remaining eight outlying data points came from six studies (Alvarez et al., 2017b,c; Álvarez et al., 2018a,d; Delgado-Floody et al., 2019; Ramírez-Vélez et al., 2019) produced by the same research group, and homeostasis model assessment-estimated insulin resistance measurements accounted for four of these outliers.
Discussion
This is the first systematic review to investigate the approaches used to examine individual responses to exercise training. Our search revealed a large number of eligible studies (n = 149; Figure 1) that spanned the aerobic, resistance, and clinical/rehabilitation training literature. Our primary analysis revealed that few studies have statistically estimated whether exercise training causes interindividual differences in trainability (Figure 2A). This finding indicates that the majority of studies may have inappropriately interpreted variability in observed responses as evidence of interindividual differences in trainability. In support of this speculation, our review highlighted several ESIR values that either fell below zero or had a 90% CI crossing zero (Figure 3). Given that many recent studies have not adopted the statistical approaches described in previous reviews (listed in Supplementary Table S3), we hope our findings help persuade researchers to adopt these approaches when estimating the existence of interindividual differences in trainability in the future work.
Our additional analyses found that few studies considered an error and an SWC or MCID when classifying individual responses (Figure 2B). Our analyses on response rates confirmed the hypotheses that: (1) thresholds not considering error and an SWC or MCID inflate response rates (Figure 4A), and (2) larger mean changes produce larger response rates (Figure 4B). Given the disconnect between the many reviews (listed in Supplementary Table S1), highlighting the importance of considering error and an SWC or MCID and the few studies doing so (Figure 2B), our findings hopefully encourage researchers to consider error and an SWC or MCID when classifying individual responses in future studies.
Interindividual Differences in Trainability
Our ESIR calculations highlighted mixed evidence for the presence of interindividual differences in trainability (Figure 3). For instance, resistance training in the Steele et al. (2017) study appeared to cause variability in strength responses, whereas aerobic training in the Leifer et al. (2016) re-analysis may not have led to interindividual differences in cardiometabolic responses because the 90% CIs crossed zero. Interestingly, fasting insulin from the Leifer et al. (2016) study and peak strength from the Steele et al. (2017) study had similar ESIR values, but only peak strength revealed evidence of interindividual differences in trainability because its 90% CI lay fully above zero (Figure 3). Because fasting insulin had a much larger sample size than peak strength (n = 1188 vs. n = 114), its larger 90% CI may reflect how blood-based physiological outcomes have larger random measurement error compared with strength-based performance outcomes. This observation may also highlight how random measurement error for a given outcome influences interpretations of interindividual differences in trainability. Future work should therefore avoid pooling data across different outcomes when estimating interindividual differences in trainability. Moreover, these discrepancies highlight how reading one of these studies in isolation may lead to either the conclusion that interindividual differences in trainability exist or do not exist (Leifer et al., 2016; Steele et al., 2017). Figure 3 presents ESIR values across a range of outcomes and studies, highlighting how the existence of interindividual differences in trainability should be interpreted on an outcome, population, and study-specific basis.
The observation that most ESIR values (18/25) lay below or crossed zero (Figure 3) adds to a growing body of literature questioning the presence of interindividual differences in trainability following standardized exercise training (Williamson et al., 2017, 2018; Del Giudice et al., 2020; Kelley et al., 2020, 2021; Bonafiglia et al., 2021a; Islam et al., 2021). For example, we recently reported wide-ranging changes in energy intake, diet composition, and sedentary time following a controlled exercise intervention (Bonafiglia et al., 2019a). This apparent variability in behavioral changes suggests that within-subject variability contributes substantially to the variability in observed responses. Recent studies have also demonstrated that individual cardiorespiratory fitness and skeletal muscle responses appear non-reproducible following repeated exposure to an identical exercise training intervention (Lindholm et al., 2016; Del Giudice et al., 2020; Islam et al., 2021). Assuming trainability of an individual is a stable and reproducible trait, this non-reproducibility provides further evidence suggesting that within-subject variability largely comprises the variability in observed responses (Hecksteden et al., 2015). Taken together, these findings indicate that it may be erroneous to assume that variability in observed responses reflects interindividual differences in trainability. Despite this indication, also highlighted in many previous reviews (listed in Supplementary Table S1), the majority of recent studies have not adopted a statistical approach to investigate the existence of interindividual differences in trainability (Figure 2A). Future work should therefore use a statistical approach to determine whether interindividual differences in trainability are present following exercise training (Atkinson and Batterham, 2015; Hecksteden et al., 2015, 2018; Hopkins, 2015; Dankel and Loenneke, 2020).
Although we focused on interindividual differences in trainability, other disciplines have reported a similar lack of evidence for “true response variability” following non-exercise interventions (Mills et al., 2020). For example, a recent meta-analysis of psychiatric assessment responses in patients with schizophrenia reported greater variability in control groups compared with treatment groups (Winkelbeiner et al., 2019). Rather than using the SDIR, these non-exercise studies utilized the “variability ratio” (Winkelbeiner et al., 2019; Mills et al., 2020), an approach that is similar to the SDIR because it relies on the assumption of independence to estimate true response variability. In this context, the assumption of independence refers to the assumption that random measurement error and within-subject variability are equal between groups in a randomized controlled trial (Bonafiglia et al., 2019a). However, the variability ratio divides, instead of subtracts, the variability of observed responses in the treatment group by the variability of observed responses in the control group. The variability ratio was used in recent meta-analyses (reviewed in Mills et al., 2020) across scientific disciplines other than exercise and sport science to empirically test for the existence of “true response variability.”
Classifying Individual Responses
We found that zero-based thresholds inflate response rates compared with classification approaches that consider error and an SWC or MCID (Figure 4A). These findings add to previous results derived from fewer outcomes and smaller datasets demonstrating that non-conservative thresholds increase the proportions of participants classified as “responders” (Hecksteden et al., 2018; Schulhauser et al., 2020). We hope our large dataset (i.e., 614 response rates from 116 studies) demonstrating inflated response rates with zero-based thresholds (Figure 4A) better convinces researchers to consider error and an SWC or MCID when classifying individual responses. Although considering error and an SWC or MCID will help conservatively identify individuals who experienced meaningful benefit, it is also possible these conservative thresholds increase the risk of failing to classify individuals who responded to exercise per se as “responders” (i.e., type II error). Conversely, less conservative thresholds (e.g., zero-based or considering one of error or an SWC or MCID only) increase the risk of classifying individuals who did not experience meaningful benefit as “responders” (i.e., type I error). While researchers can choose which risk of misclassification is more acceptable for their study, avoiding dichotomous classification and considering uncertainty (e.g., individual confidence intervals) can help reduce the risk of misclassifying “responders” or “non-responders” (Bonafiglia et al., 2018; Swinton et al., 2018).
Controlling for differences in response thresholds supported our ancillary hypothesis that larger mean changes produce larger response rates (Figure 4B). This finding corroborates our recent demonstration that larger mean changes explain why higher doses of exercise produce larger cardiorespiratory fitness response rates (Bonafiglia et al., 2021b). We speculate that the red outliers in the 25, 50, and 75% quartiles had large variability (or a single outlying data point), which resulted in a low response rate despite having a large standardized mean change (and vice versa for the blue data point in Figure 4B). However, analyzing these raw data is needed to confirm this speculation. Both analyses demonstrate how response rates are group statistics that highly depend on response thresholds (Figure 4A) and mean changes (Figure 4B; Atkinson et al., 2019). Future research should therefore avoid attributing lower response rates to reduced interindividual variability, an interpretation made in several studies included in our review (Wolpern et al., 2015; Dalleck et al., 2016; Byrd et al., 2019), and should recognize that response rates provide little if any, useful information at the individual level (Atkinson et al., 2019).
Although our results demonstrate how we should classify observed changes following exercise training (i.e., consider error and an SWC or MCID; Figure 4A), what remains less clear is why we should classify individuals in the first place. Although individual classification may help guide exercise prescription decision-making in clinical and applied settings, to our knowledge, only one study (Montero and Lundby, 2017) has utilized initial response classifications to guide subsequent exercise prescription decisions. A key challenge to individualizing exercise prescription is choosing a response threshold that incorporates an equipment-specific error estimate (Weatherwax et al., 2018a) and a change linked to clinical benefit (i.e., MCID). While equipment-specific errors can be easily generated using simple test re-test experiments, MCIDs are not available for many outcomes (Hopkins, 2000). For VO2max, we recently used an MCID of 1.0 MET because this change confers a ∼8–14% risk reduction in all-cause morbidity and mortality (Dorn et al., 1999; Bonafiglia et al., 2019b). When MCIDs are unavailable, researchers can use SWCs (i.e., 20% of baseline standard deviation) to estimate a threshold representing a small effect size (Swinton et al., 2018). It is important to emphasize that, unlike MCIDs, SWCs are clinically arbitrary and should not be used to gauge whether an individual has clinically benefited following exercise training. Future work is needed to examine the validity of the classification of individual responses for the purpose of optimizing exercise prescriptions and for establishing MCIDs for a wider range of outcomes.
Limitations and Future Directions
The few studies that statistically estimated the presence of interindividual differences in trainability varied substantially in participant characteristics, training modes, and outcomes assessed (Figure 3). Recent meta-analyses have reported pooled SDIR estimates indicating a lack of interindividual differences in trainability in body weight and body composition (Williamson et al., 2018; Kelley et al., 2020, 2021). Unlike the present study, these meta-analyses focused on a single outcome and used search criteria that identified all studies reporting standard deviations of change scores and not just those evaluating individual responses as we did in the present review. We did not pool ESIR values because of the heterogeneity in populations (e.g., overweight adolescents, adults with low back pain, or older adults with cognitive impairment) and outcomes assessed (e.g., VO2max, perceptions of back pain, or an Alzheimer’s cognition score) across studies (Figure 3). Nevertheless, the observation that most ESIR values (18/25) lay below or crossed zero supports conclusions from pooled estimates of single outcomes demonstrating a lack of interindividual differences in trainability. More studies should adopt the SDIR approach to allow for additional population- and outcome-specific meta-analyses on other clinically relevant outcomes (e.g., VO2max, waist circumference, grip strength, etc.). Importantly, these meta-analyses can provide robust assessments of the presence or absence of interindividual differences in trainability.
Large variation in our dataset may also explain why response rates were not significantly different between studies that considered error or SWC or MCID vs. studies that considered both (Figure 4A). Comparing response rates across different thresholds within the same group (and thus mean change) overcomes this limitation by eliminating between-study variation in participant characteristics, training modes, and random measurement error. Although we and others have explored the relationship between response rates and thresholds using VO2max and exercise performance data (Hecksteden et al., 2018; Schulhauser et al., 2020), future work should confirm that failing to account for error and an SWC or MCID inflates response rates in different outcomes.
Conclusion
The present systematic review found that despite many previous reviews (listed in Supplementary Table S1) advocating for more statistically sound approaches when examining individual responses, few studies have statistically estimated interindividual differences in trainability or used response classification thresholds that consider error and an SWC or MCID. We also presented ESIR values that question the presence of interindividual differences in trainability, which demonstrates why it is inappropriate and potentially erroneous to assume variability in observed responses reflects interindividual differences in trainability. Further, we found that zero-based thresholds inflate response rates, which demonstrates why it is important to classify responses using an approach that considers both error and an SWC or MCID. Additionally, our analysis examining mean changes supported the notion that response rates are group statistics that provide little information about an individual’s response to exercise training. We hope our findings and novel data presentations better convince researchers to statistically estimate interindividual differences in trainability and consider error and an SWC or MCID in future work.
Data Availability Statement
The original contributions presented in the study are included in the article/Supplementary Material, further inquiries can be directed to the corresponding author.
Author Contributions
JTB and NP wrote the first draft of the manuscript. All authors contributed to conception and design of the study, conducted the systematic review, statistical analysis, and manuscript revision, read, and approved the submitted version.
Funding
This project was supported by an operating grant from the Natural Sciences and Engineering Research Council of Canada (NSERC; grant number: 402635) to BJG.
Conflict of Interest
The authors declare that the research was conducted in the absence of any commercial or financial relationships that could be construed as a potential conflict of interest.
Publisher’s Note
All claims expressed in this article are solely those of the authors and do not necessarily represent those of their affiliated organizations, or those of the publisher, the editors and the reviewers. Any product that may be evaluated in this article, or claim that may be made by its manufacturer, is not guaranteed or endorsed by the publisher.
Supplementary Material
The Supplementary Material for this article can be found online at: https://www.frontiersin.org/articles/10.3389/fphys.2021.665044/full#supplementary-material
References
Ahtiainen, J. P., Sallinen, J., Häkkinen, K., and Sillanpää, E. (2020). Inter-individual variation in response to resistance training in cardiometabolic health indicators. Scand. J. Med. Sci. Sports 30, 1040–1053. doi: 10.1111/sms.13650
Ahtiainen, J. P., Walker, S., Peltonen, H., Holviala, J., Sillanpää, E., Karavirta, L., et al. (2016). Heterogeneity in resistance training-induced muscle strength and mass responses in men and women of different ages. Age 38:10. doi: 10.1007/s11357-015-9870-1
Álvarez, C., Ramírez-Campillo, R., Cano-Montoya, J., Ramírez-Vélez, R., Harridge, S. D. R., Alonso-Martínez, A. M., et al. (2018a). Exercise and glucose control in children with insulin resistance: prevalence of non-responders. Pediatr. Obes. 13, 794–802. doi: 10.1111/ijpo.12437
Álvarez, C., Ramírez-Campillo, R., Cristi-Montero, C., Ramírez-Vélez, R., and Izquierdo, M. (2018b). Prevalence of non-responders for blood pressure and cardiometabolic risk factors among prehypertensive women after long-term high-intensity interval training. Front. Physiol. 9:1443. doi: 10.3389/fphys.2018.01443
Álvarez, C., Ramírez-Campillo, R., Ramírez-Vélez, R., Martínez, C., Castro-Sepúlveda, M., Alonso-Martínez, A., et al. (2018c). Metabolic effects of resistance or high-intensity interval training among glycemic control-nonresponsive children with insulin resistance. Int. J. Obes. 42, 79–87. doi: 10.1038/ijo.2017.177
Álvarez, C., Ramírez-Vélez, R., Ramírez-Campillo, R., Ito, S., Celis-Morales, C., García-Hermoso, A., et al. (2018d). Interindividual responses to different exercise stimuli among insulin-resistant women. Scand. J. Med. Sci. Sports 28, 2052–2065. doi: 10.1111/sms.13213
Álvarez, C., Ramírez-Campillo, R., Lucia, A., Ramírez-Vélez, R., and Izquierdo, M. (2019). Concurrent exercise training on hyperglycemia and comorbidities associated: non-responders using clinical cutoff points. Scand. J. Med. Sci. Sports 29, 952–967. doi: 10.1111/sms.13413
Alvarez, C., Ramírez-Campillo, R., Ramírez-Vélez, R., and Izquierdo, M. (2017a). Effects and prevalence of non-responders after 12weeks of high-intensity interval or resistance training in adult woman with insulin resistance: a randomized trial. J. Appl. Physiol. 122, 985–996. doi: 10.1152/japplphysiol.01037.2016
Alvarez, C., Ramírez-Campillo, R., Ramírez-Vélez, R., and Izquierdo, M. (2017b). Effects of 6-weeks high-intensity interval training in schoolchildren with insulin resistance: influence of biological maturation on metabolic, body composition, cardiovascular and performance non-responses. Front. Physiol. 8:444. doi: 10.3389/fphys.2017.00444
Alvarez, C., Ramírez-Campillo, R., Ramírez-Vélez, R., and Izquierdo, M. (2017c). Prevalence of non-responders for glucose control markers after 10 weeks of high-intensity interval training in adult women with higher and lower insulin resistance. Front. Physiol. 8:479. doi: 10.3389/fphys.2017.00479
Astorino, T. A., and Schubert, M. M. (2014). Individual responses to completion of short-term and chronic interval training: a retrospective study. PLoS One 9:e97638. doi: 10.1371/journal.pone.0097638
Astorino, T. A., deRevere, J., Anderson, T., Kellogg, E., Holstrom, P., Ring, S., et al. (2018). Change in VO2maxand time trial performance in response to high-intensity interval training prescribed using ventilatory threshold. Eur. J. Appl. Physiol. 118, 1811–1820. doi: 10.1007/s00421-018-3910-3
Atkinson, G., and Batterham, A. M. (2015). True and false interindividual differences in the physiological response to an intervention. Exp. Physiol. 100, 577–588. doi: 10.1113/EP085070
Atkinson, G., Williamson, P., and Batterham, A. M. (2019). Issues in the determination of ‘responders’ and ‘non-responders’ in physiological research. Exp. Physiol. 104, 1215–1225. doi: 10.1113/EP087712
Bakker, E. A., Snoek, J. A., Meindersma, E. P., Hopman, M. T. E., Bellersen, L., Verbeek, A. L. M., et al. (2018). Absence of fitness improvement is associated with outcomes in heart failure patients. Med. Sci. Sports Exerc. 50, 196–203. doi: 10.1249/MSS.0000000000001429
Barbalho, M., de, S. M., Gentil, P., Izquierdo, M., Fisher, J., Steele, J., et al. (2017). There are no no-responders to low or high resistance training volumes among older women. Exp. Gerontol. 99, 18–26. doi: 10.1016/j.exger.2017.09.003
Barbeau, P., Gutin, B., Litaker, M. S., Ramsey, L. T., Cannady, W. E., Allison, J., et al. (2003). Influence of physical training on plasma leptin in obese youths. Can. J. Appl. Physiol. 28, 382–396. doi: 10.1139/h03-028
Barber, J. L., Ruiz-Ramie, J. J., Robbins, J. M., Gerszten, R. E., Leon, A. S., Rao, D., et al. (2021). Regular exercise and patterns of response across multiple cardiometabolic traits: the HERITAGE family study. Br. J. Sports Med. doi: 10.1136/bjsports-2020-103323 [Epub ahead of print].
Bonafiglia, J. T., Brennan, A. M., Ross, R., and Gurd, B. J. (2019a). An appraisal of the SD IR as an estimate of true individual differences in training responsiveness in parallel-arm exercise randomized controlled trials. Physiol. Rep. 7:e14163. doi: 10.14814/phy2.14163
Bonafiglia, J. T., Ross, R., and Gurd, B. J. (2019b). The application of repeated testing and monoexponential regressions to classify individual cardiorespiratory fitness responses to exercise training. Eur. J. Appl. Physiol. 119, 889–900. doi: 10.1007/s00421-019-04078-w
Bonafiglia, J. T., Islam, H., Eynon, N., and Gurd, B. J. (2020). “Statistical considerations and biological mechanisms underlying individual differences in adaptations to exercise training,” in The Routledge Handbook on Biochemistry of Exercise, eds P. M. Tiidus, R. E. K. MacPherson, P. J. LeBlanc, and A. R. Josse (Abingdon-on-Thames: Routledge), 242–259. doi: 10.4324/9781003123835-16
Bonafiglia, J. T., Nelms, M. W., Preobrazenski, N., LeBlanc, C., Robins, L., Lu, S., et al. (2018). Moving beyond threshold-based dichotomous classification to improve the accuracy in classifying non-responders. Physiol. Rep. 6:e13928. doi: 10.14814/phy2.13928
Bonafiglia, J. T., Islam, H., Preobrazenski, N., Ma, A., Deschenes, M., Erlich, A. T., et al. (2021a). Examining interindividual differences in select muscle and whole-body adaptations to continuous endurance training. Exp. Physiol. doi: 10.1113/EP089421 [Epub ahead of print].
Bonafiglia, J. T., Preobrazenski, N., Islam, H., Walsh, J. J., Ross, R., Johannsen, N. M., et al. (2021b). Exploring differences in cardiorespiratory fitness response rates across varying doses of exercise training: a retrospective analysis of eight randomized controlled trials. Sports Med. 51, 1785–1797. doi: 10.1007/s40279-021-01442-9
Bonafiglia, J. T., Rotundo, M. P., Whittall, J. P., Scribbans, T. D., Graham, R. B., and Gurd, B. J. (2016). Inter-individual variability in the adaptive responses to endurance and sprint interval training: a randomized crossover study. PLoS One 11:e0167790. doi: 10.1371/journal.pone.0167790
Bouchard, C., An, P., Rice, T., Skinner, J. S., Wilmore, J. H., Gagnon, J., et al. (1999). Familial aggregation of VO2max response to exercise training: results from the HERITAGE family study. J. Appl. Physiol. 87, 1003–1008. doi: 10.1152/jappl.1999.87.3.1003
Bouchard, C., Blair, S. N., Church, T. S., Earnest, C. P., Hagberg, J. M., Häkkinen, K., et al. (2012). Adverse metabolic response to regular exercise: is it a rare or common occurrence? PLoS One 7:e37887. doi: 10.1371/journal.pone.0037887
Bouchard, C., Tremblay, A., Després, J.-P., Thériault, G., Nadeauf, A., Lupien, P. J., et al. (1994). The response to exercise with constant energy intake in identical twins. Obes. Res. 2, 400–410. doi: 10.1002/j.1550-8528.1994.tb00087.x
Bouchard, C., Tremblay, A., Nadeau, A., Dussault Despres, J. J. P., Theriault, G., Lupien, P. J., et al. (1990). Long-term exercise training with constant energy intake. 1: effect on body composition and selected metabolic variables. Int. J. Obes. 14, 57–73.
Boule, N. G., Weisnagel, S. J., Lakka, T. A., Tremblay, A., Bergman, R. N., Rankinen, T., et al. (2005). Effects of exercise training on glucose homeostasis. Diabetes Care 28, 108–114.
Brennan, A. M., Day, A. G., Cowan, T. E., Clarke, G. J., Lamarche, B., and Ross, R. (2019). Individual response to standardized exercise: total and abdominal adipose tissue. Med. Sci. Sports Exerc. 52:1. doi: 10.1249/MSS.0000000000001930
Byrd, B. R., Keith, J., Keeling, S. M., Weatherwax, R. M., Nolan, P. B., Ramos, J. S., et al. (2019). Personalized moderate-intensity exercise training combined with high-intensity interval training enhances training responsiveness. Int. J. Environ. Res. Public Health 16:2088. doi: 10.3390/ijerph16122088
Cadore, E. L., Pinto, R. S., Teodoro, J. L., da Silva, L. X. N., Menger, E., Alberton, C. L., et al. (2018). Cardiorespiratory adaptations in elderly men following different concurrent training regimes. J. Nutr. Heal. Aging 22, 483–490. doi: 10.1007/s12603-017-0958-4
Castro, A., Duft, R. G., Ferreira, M. L. V., de Andrade, A. L. L., Gáspari, A. F., Silva, L. D. M., et al. (2019). Association of skeletal muscle and serum metabolites with maximum power output gains in response to continuous endurance or high-intensity interval training programs: the TIMES study – a randomized controlled trial. PLoS One 14:e0212115. doi: 10.1371/journal.pone.0212115
Caudwell, P., Hopkins, M., King, N. A., Stubbs, R. J., and Blundell, J. E. (2009). Exercise alone is not enough: weight loss also needs a healthy (Mediterranean) diet? Public Health Nutr. 12, 1663–1666. doi: 10.1017/S1368980009990528
Chen, Y.-W., Gregory, C., Ye, F., Harafuji, N., Lott, D., Lai, S.-H., et al. (2017). Molecular signatures of differential responses to exercise trainings during rehabilitation. Biomed. Genet. Genomics 2, 1–22. doi: 10.15761/bgg.1000127
Chmelo, E. A., Crotts, C. I., Newman, J. C., Brinkley, T. E., Lyles, M. F., Leng, X., et al. (2015). Heterogeneity of physical function responses to exercise training in older adults. J. Am. Geriatr. Soc. 63, 462–469. doi: 10.1111/jgs.13322
Chrzanowski-Smith, O. J., Piatrikova, E., Betts, J. A., Williams, S., and Gonzalez, J. T. (2020). Variability in exercise physiology: can capturing intra-individual variation help better understand true inter-individual responses? Eur. J. Sports Sci. 20, 452–460. doi: 10.1080/17461391.2019.1655100
Churchward-Venne, T. A., Tieland, M., Verdijk, L. B., Leenders, M., Dirks, M. L., de Groot, L. C. P. G. M., et al. (2015). There are no nonresponders to resistance-type exercise training in older men and women. J. Am. Med. Dir. Assoc. 16, 400–411. doi: 10.1016/j.jamda.2015.01.071
Croymans, D. M., Paparisto, E., Lee, M. M., Brandt, N., Le, B. K., Lohan, D., et al. (2013). Resistance training improves indices of muscle insulin sensitivity and β-cell function in overweight/obese, sedentary young men. J. Appl. Physiol. 115, 1245–1253. doi: 10.1152/japplphysiol.00485.2013
da Cunha Nascimento, D., da Silva, C. R., Valduga, R., Saraiva, B., de Sousa Neto, I. V., Vieira, A., et al. (2018). Blood pressure response to resistance training in hypertensive and normotensive older women. Clin. Interv. Aging 13, 541–553. doi: 10.2147/CIA.S157479
da Cunha Nascimento, D., de Sousa Neto, I. V., Saraiva, B., Lima, A. D. S., Navalta, J. W., Pereira, G. B., et al. (2020). Advancements and critical steps for statistical analyses in blood pressure response to resistance training in hypertensive older women. Blood Press. Monit. 26, 135–145. doi: 10.1097/MBP.0000000000000505
Dalleck, L. C., Haney, D. E., Buchanan, C. A., and Weatherwax, R. M. (2016). Does a personalised exercise prescription enhance training efficacy and limit training unresponsiveness? A randomised controlled trial. Fit. Res. 5, 15–27.
Dalleck, L. C., Van Guilder, G. P., Richardson, T. B., and Vella, C. A. (2015). The prevalence of adverse cardiometabolic responses to exercise training with evidence-based practice is low. Diabetes Metab. Syndr. Obes. Targets Ther. 8, 73–78. doi: 10.2147/DMSO.S76880
Damas, F., Barcelos, C., Nóbrega, S. R., Ugrinowitsch, C., Lixandrão, M. E., Santos, L. M. E. D., et al. (2019b). Individual muscle hypertrophy and strength responses to high vs. low resistance training frequencies. J. Strength Cond. Res. 33, 897–901. doi: 10.1519/JSC.0000000000002864
Damas, F., Angleri, V., Phillips, S. M., Witard, O. C., Ugrinowitsch, C., Santanielo, N., et al. (2019a). Myofibrillar protein synthesis and muscle hypertrophy individualized responses to systematically changing resistance training variables in trained young men. J. Appl. Physiol. 127, 806–815. doi: 10.1152/japplphysiol.00350.2019
Dankel, S. J., and Loenneke, J. P. (2020). A method to stop analyzing random error and start analyzing differential responders to exercise. Sports Med. 50, 231–238. doi: 10.1007/s40279-019-01147-0
Davidsen, P. K., Gallagher, I. J., Hartman, J. W., Tarnopolsky, M. A., Dela, F., Helge, J. W., et al. (2011). High responders to resistance exercise training demonstrate differential regulation of skeletal muscle microRNA expression. J. Appl. Physiol. 110, 309–317. doi: 10.1152/japplphysiol.00901.2010
de Lannoy, L., Clarke, J., Stotz, P. J., and Ross, R. (2017). Effects of intensity and amount of exercise on measures of insulin and glucose: analysis of inter-individual variability. PLoS One 12:e0177095. doi: 10.1371/journal.pone.0177095
Del Giudice, M., Bonafiglia, J. T., Islam, H., Preobrazenski, N., Amato, A., and Gurd, B. J. (2020). Investigating the reproducibility of maximal oxygen uptake responses to high-intensity interval training. J. Sci. Med. Sports 23, 94–99. doi: 10.1016/j.jsams.2019.09.007
Delgado-Floody, P., Álvarez, C., Lusa Cadore, E., Flores-Opazo, M., Caamaño-Navarrete, F., and Izquierdo, M. (2019). Preventing metabolic syndrome in morbid obesity with resistance training: reporting interindividual variability. Nutr. Metab. Cardiovasc. Dis. 29, 1368–1381. doi: 10.1016/j.numecd.2019.07.002
Denham, J. (2019). The association between sperm telomere length, cardiorespiratory fitness and exercise training in humans. Biomed. J. 42, 430–433. doi: 10.1016/j.bj.2019.07.003
Díaz-Vegas, A., Espinoza, A., Cofré, C., and Sánchez-Aguilera, P. (2018). Eccentric resistance training reduces both non-response to exercise and cardiovascular risk factors in adult with overweight or obesity. Sci. Sports 33, 245–252. doi: 10.1016/j.scispo.2017.12.002
Dionne, F. T., Turcotte, L., Thibault, M. C., Boulay, M. R., Skinner, J. S., and Bouchard, C. (1991). Mitochondrial DNA sequence polymorphism, VO2max, and response to endurance training. Med. Sci. Sports Exerc. 23, 177–185. doi: 10.1249/00005768-199102000-00006
Dorn, J., Naughton, J., Imamura, D., and Trevisan, M. (1999). Results of a multicenter randomized clinical trial of exercise and long-term survival in myocardial infarction patients. Circulation 100, 1764–1769. doi: 10.1161/01.CIR.100.17.1764
Edgett, B. A., Bonafiglia, J. T., Raleigh, J. P., Rotundo, M. P., Giles, M. D., Whittall, J. P., et al. (2018). Reproducibility of peak oxygen consumption and the impact of test variability on classification of individual training responses in young recreationally active adults. Clin. Physiol. Funct. Imaging 38, 630–638. doi: 10.1111/cpf.12459
Erskine, R. M., Jones, D. A., Williams, A. G., Stewart, C. E., and Degens, H. (2010). Inter-individual variability in the adaptation of human muscle specific tension to progressive resistance training. Eur. J. Appl. Physiol. 110, 1117–1125. doi: 10.1007/s00421-010-1601-9
Garcia, P., Nascimento, D., da, C., Tibana, R. A., Barboza, M. M., Willardson, J. M., et al. (2016). Comparison between the multiple-set plus 2 weeks of tri-set and traditional multiple-set method on strength and body composition in trained women: a pilot study. Clin. Physiol. Funct. Imaging 36, 47–52. doi: 10.1111/cpf.12192
Goddard, N., Baker, M., Higgins, T., and Cobbold, C. (2014). The effect of angiotensin converting enzyme genotype on aerobic capacity following high intensity interval training. Int. J. Exerc. Sci. 7, 250–259.
Gray, S. R., Aird, T. P., Farquharson, A. J., Horgan, G. W., Fisher, E., Wilson, J., et al. (2018). Inter-individual responses to sprint interval training, a pilot study investigating interactions with the sirtuin system. Appl. Physiol. Nutr. Metab. 43, 84–93. doi: 10.1139/apnm-2017-0224
Gross, M., Swensen, T., and King, D. (2007). Nonconsecutive- versus consecutive-day high-intensity interval training in cyclists. Med. Sci. Sports Exerc. 39, 1666–1671. doi: 10.1249/mss.0b013e3180cac209
Gurd, B. J., Giles, M. D., Bonafiglia, J. T., Raleigh, J. P., Boyd, J. C., Ma, J. K., et al. (2016). Incidence of nonresponse and individual patterns of response following sprint interval training. Appl. Physiol. Nutr. Metab. 41, 229–234. doi: 10.1139/apnm-2015-0449
Hagstrom, A. D., and Denham, J. (2018). MicroRNAs in high and low responders to resistance training in breast cancer survivors. Int. J. Sports Med. 39, 482–489. doi: 10.1055/a-0592-7691
Hammond, B. P., Stotz, P. J., Brennan, A. M., Lamarche, B., Day, A. G., and Ross, R. (2019). Individual variability in waist circumference and body weight in response to exercise. Med. Sci. Sports Exerc. 51, 315–322. doi: 10.1249/MSS.0000000000001784
Haun, C. T., Vann, C. G., Mobley, C. B., Osburn, S. C., Mumford, P. W., Roberson, P. A., et al. (2019). Pre-training skeletal muscle fiber size and predominant fiber type best predict hypertrophic responses to 6 weeks of resistance training in to previously trained young men. Front. Physiol. 10:297. doi: 10.3389/fphys.2019.00297
Hauser, A., Schmitt, L., Troesch, S., Saugy, J. J., Cejuela-Anta, R., Faiss, R., et al. (2016). Similar hemoglobin mass response in hypobaric and normobaric hypoxia in athletes. Med. Sci. Sports Exerc. 48, 734–741. doi: 10.1249/MSS.0000000000000808
Hautala, A. J., Kiviniemi, A. M., Mäkikallio, T. H., Kinnunen, H., Nissilä, S., Huikuri, H. V., et al. (2006). Individual differences in the responses to endurance and resistance training. Eur. J. Appl. Physiol. 96, 535–542. doi: 10.1007/s00421-005-0116-2
Hecksteden, A., Kraushaar, J., Scharhag-Rosenberger, F., Theisen, D., Senn, S., and Meyer, T. (2015). Individual response to exercise training – a statistical perspective. J. Appl. Physiol. 118, 1450–1459. doi: 10.1152/japplphysiol.00714.2014
Hecksteden, A., Pitsch, W., Rosenberger, F., and Meyer, T. (2018). Repeated testing for the assessment of individual response to exercise training. J. Appl. Physiol. 124, 1567–1579. doi: 10.1152/japplphysiol.00896.2017
Higgins, J. P. T., Altman, D. G., Gøtzsche, P. C., Jüni, P., Moher, D., Oxman, A. D., et al. (2011). The Cochrane Collaboration’s tool for assessing risk of bias in randomised trials. BMJ 343, 1–9. doi: 10.1136/bmj.d5928
Higgins, J. P. T., Thomas, J., Chandler, J., Cumpston, M., Li, T., Page, M. J., et al. (2019). Cochrane Handbook for Systematic Reviews of Interventions, 2nd Edn. Hoboken, NJ: Wiley. doi: 10.1002/9781119536604
Hopkins, W. G. (2000). Measures of reliability in sports medicine and science. Sports Med. 30, 375–381. doi: 10.2165/00007256-200030050-00006
Hopkins, W. G. (2015). Individual responses made easy. J. Appl. Physiol. 118, 1444–1446. doi: 10.1152/japplphysiol.00098.2015
Horak, M., Zlamal, F., Iliev, R., Kucera, J., Cacek, J., Svobodova, L., et al. (2018). Exercise-induced circulating microRNA changes in athletes in various training scenarios. PLoS One 13:e0191060. doi: 10.1371/journal.pone.0191060
Hubal, M. J., Gordish Dressman, H., Thompson, P. D., Price, T. B., Hoffman, E. P., Angelopoulos, T. J., et al. (2005). Variability in muscle size and strength gain after unilateral resistance training. Med. Sci. Sports Exerc. 37, 964–972. doi: 10.1249/01.mss.0000170469.90461.5f
Hvid, L. G., Nielsen, M. K. F., Simonsen, C., Caserotti, P., Andersen, M., and Caserotti, P. (2017). Brain-derived neurotrophic factor (BDNF) serum basal levels is not affected by power training in mobility-limited older adults — a randomized controlled trial. Exp. Gerontol. 93, 29–35. doi: 10.1016/j.exger.2017.03.019
Islam, H., Bonafiglia, J. T., Del Giudice, M., Pathmarajan, R., Simpson, C. A., Quadrilatero, J., et al. (2021). Repeatability of training-induced skeletal muscle adaptations in active young males. J. Sci. Med. Sports 24, 494–498. doi: 10.1016/j.jsams.2020.10.016
Islam, H., Siemens, T. L., Matusiak, J. B. L., Sawula, L., Bonafiglia, J. T., Preobrazenski, N., et al. (2019). Cardiorespiratory fitness and muscular endurance responses immediately and two-months after a whole-body Tabata or vigorous-intensity continuous training intervention. Appl. Physiol. Nutr. Metab. 45, 650–658. doi: 10.1139/apnm-2019-0492
Johannsen, N. M., Swift, D. L., Lavie, C. J., Earnest, C. P., Blair, S. N., and Church, T. S. (2013). Categorical analysis of the impact of aerobic and resistance exercise training, alone and in combination, on cardiorespiratory fitness levels in patients with type 2 diabetes. Diabetes Care 36, 3305–3312. doi: 10.2337/dc12-2194
Kacerovsky-Bielesz, G., Kacerovsky, M., Chmelik, M., Farukuoye, M., Ling, C., Pokan, R., et al. (2012). A single nucleotide polymorphism associates with the response of muscle ATP synthesis to long-term exercise training in relatives of type 2 diabetic humans. Diabetes Care 35, 350–357. doi: 10.2337/dc11-1426
Karavirta, L., Costa, M. D., Goldberger, A. L., Tulppo, M. P., Laaksonen, D. E., Nyman, K., et al. (2013). Heart rate dynamics after combined strength and endurance training in middle-aged women: heterogeneity of responses. PLoS One 8:e72664. doi: 10.1371/journal.pone.0072664
Karavirta, L., Häkkinen, K., Kauhanen, A., Arija-Blázquez, A., Sillanpää, E., Rinkinen, N., et al. (2011). Individual responses to combined endurance and strength training in older adults. Med. Sci. Sports Exerc. 43, 484–490. doi: 10.1249/MSS.0b013e3181f1bf0d
Keller, P., Vollaard, N. B. J. J., Gustafsson, T., Gallagher, I. J., Sundberg, C. J., Rankinen, T., et al. (2011). A transcriptional map of the impact of endurance exercise training on skeletal muscle phenotype. J. Appl. Physiol. 110, 46–59. doi: 10.1152/japplphysiol.00634.2010
Kelley, G. A., Kelley, K. S., and Pate, R. R. (2020). Are there inter-individual differences in fat mass and percent body fat as a result of aerobic exercise training in overweight and obese children and adolescents? A meta-analytic perspective. Child. Obes. 16, 301–306. doi: 10.1089/chi.2020.0056
Kelley, G. A., Kelley, K. S., and Pate, R. R. (2021). Inter-individual differences in body mass index were not observed as a result of aerobic exercise in children and adolescents with overweight and obesity. Pediatr. Obes. 16, 1–9. doi: 10.1111/ijpo.12692
King, N. A., Hopkins, M., Caudwell, P., Stubbs, R. J., and Blundell, J. E. (2008). Individual variability following 12 weeks of supervised exercise: identification and characterization of compensation for exercise-induced weight loss. Int. J. Obes. 32, 177–184. doi: 10.1038/sj.ijo.0803712
Kneppers, A. E. M., Haast, R. A. M., Langen, R. C. J., Verdijk, L. B., Leermakers, P. A., Gosker, H. R., et al. (2019). Distinct skeletal muscle molecular responses to pulmonary rehabilitation in chronic obstructive pulmonary disease: a cluster analysis. J. Cachexia Sarcopenia Muscle 10, 311–322. doi: 10.1002/jcsm.12370
Koufaki, P., Nash, P. F., and Mercer, T. H. (2002). Assessing the efficacy of exercise training in patients with chronic disease. Med. Sci. Sports Exerc. 34, 1234–1241. doi: 10.1097/00005768-200208000-00002
Krusnauskas, R., Eimantas, N., Baranauskiene, N., Venckunas, T., Snieckus, A., Brazaitis, M., et al. (2020). Response to three weeks of sprint interval training cannot be explained by the exertional level. Medicina 56:395. doi: 10.3390/medicina56080395
Lakens, D. (2013). Calculating and reporting effect sizes to facilitate cumulative science: a practical primer for t-tests and ANOVAs. Front. Psychol. 4:863. doi: 10.3389/fpsyg.2013.00863
Leddy, A. L., Connolly, M., Holleran, C. L., Hennessy, P. W., Woodward, J., Arena, R. A., et al. (2016). Alterations in aerobic exercise performance and gait economy following high-intensity dynamic stepping training in persons with subacute stroke. J. Neurol. Phys. Ther. 40, 239–248. doi: 10.1097/NPT.0000000000000147
Leifer, E. S., Mikus, C. R., Karavirta, L., Resnick, B. D., Kraus, W. E., Hakkinen, K., et al. (2016). Adverse cardiovascular response to aerobic exercise training: is this a concern? Med. Sci. Sports Exerc. 48, 20–25. doi: 10.1249/MSS.0000000000000752
Leon, A. S., Gaskill, S. E., Rice, T., Bergeron, J., Gagnon, J., Rao, D. C., et al. (2002). Variability in the response of HDL cholesterol to exercise training in the HERITAGE family study. Int. J. Sports Med. 23, 1–9. doi: 10.1055/s-2002-19270
Leoñska-Duniec, A., Jastrzêbski, Z., Jażdżewska, A., Moska, W., Luliñska-Kuklik, E., Sawczuk, M., et al. (2018). Individual responsiveness to exercise-induced fat loss and improvement of metabolic profile in young women is associated with polymorphisms of adrenergic receptor genes. J. Sports Sci. Med. 17, 134–144.
Lindholm, M. E., Giacomello, S., Werne Solnestam, B., Fischer, H., Huss, M., Kjellqvist, S., et al. (2016). The impact of endurance training on human skeletal muscle memory, global isoform expression and novel transcripts. PLoS Genet. 12:e1006294. doi: 10.1371/journal.pgen.1006294
Lortie, G., Simoneau, J. A., Hamel, P., Boulay, M. R., Landry, F., and Bouchard, C. (1984). Responses of maximal aerobic power and capacity to aerobic training. Int. J. Sports Med. 5, 232–236. doi: 10.1055/s-2008-1025911
Marsh, C. E., Thomas, H. J., Naylor, L. H., Scurrah, K. J., and Green, D. J. (2020). Fitness and strength responses to distinct exercise modes in twins: studies of twin responses to understand exercise as a therapy (STRUETH) study. J. Physiol. 598, 3845–3858. doi: 10.1113/JP280048
Massie, R., Smallcombe, J., and Tolfrey, K. (2019). Effects of a 12-week exercise intervention on subsequent compensatory behaviors in adolescent girls: an exploratory study. Pediatr. Exerc. Sci. 31, 495–504. doi: 10.1123/pes.2019-0012
Mclaren, S. J., Smith, A., Bartlett, J. D., Spears, I. R., Weston, M., Mclaren, S. J., et al. (2018). Differential training loads and individual fitness responses to pre-season in professional rugby union players. J. Sports Sci. 36, 2438–2446. doi: 10.1080/02640414.2018.1461449
McPhee, J. S., Williams, A. G., Degens, H., and Jones, D. A. (2010). Inter-individual variability in adaptation of the leg muscles following a standardised endurance training programme in young women. Eur. J. Appl. Physiol. 109, 1111–1118. doi: 10.1007/s00421-010-1454-2
McPhee, J. S., Williams, A. G., Perez-Schindler, J., Degens, H., Baar, K., and Jones, D. A. (2011). Variability in the magnitude of response of metabolic enzymes reveals patterns of co-ordinated expression following endurance training in women. Exp. Physiol. 96, 699–707. doi: 10.1113/expphysiol.2011.057729
Medrano, M., Arenaza, L., Ramírez-Vélez, R., Ortega, F. B., Ruiz, J. R., and Labayen, I. (2020). Prevalence of responders for hepatic fat, adiposity and liver enzyme levels in response to a lifestyle intervention in children with overweight/obesity: EFIGRO randomized controlled trial. Pediatr. Diabetes 21, 215–223. doi: 10.1111/pedi.12949
Metcalfe, R. S., Tardif, N., Thompson, D., and Vollaard, N. B. J. (2016). Changes in aerobic capacity and glycaemic control in response to reduced-exertion high-intensity interval training (REHIT) are not different between sedentary men and women. Appl. Physiol. Nutr. Metab. 41, 1117–1123. doi: 10.1139/apnm-2016-0253
Mills, H., Higgins, J. P., Morris, R., Kessler, D., Heron, J., Wiles, N., et al. (2020). Detecting heterogeneity of intervention effects using analysis and meta-analysis of differences in variance between arms of a trial. MedRxiv [Preprint] doi: 10.1101/2020.03.07.20032516
Mobley, C. B., Haun, C. T., Roberson, P. A., Mumford, P. W., Kephart, W. C., Romero, M. A., et al. (2018). Biomarkers associated with low, moderate, and high vastus lateralis muscle hypertrophy following 12 weeks of resistance training. PLoS One 13:e0195203. doi: 10.1371/journal.pone.0195203
Montero, D., and Lundby, C. (2017). Refuting the myth of non-response to exercise training: ‘non-responders’ do respond to higher dose of training. J. Physiol. 595, 3377–3387. doi: 10.1113/JP273480
Morton, R. W., Sato, K., Gallaugher, M. P. B., Oikawa, S. Y., McNicholas, P. D., Fujita, S., et al. (2018). Muscle androgen receptor content but not systemic hormones is associated with resistance training-induced skeletal muscle hypertrophy in healthy, young men. Front. Physiol. 9:1373. doi: 10.3389/fphys.2018.01373
Mueller, K., Moller, H. E., Horstmann, A., Busse, F., Lepsien, J., Bluher, M., et al. (2015). Physical exercise in overweight to obese individuals induces metabolic-and neurotrophic-related structural brain plasticity. Front. Hum. Neurosci. 9:372. doi: 10.3389/fnhum.2015.00372
Mulroy, S. J., Klassen, T., Gronley, J. K., Eberly, V. J., Brown, D. A., and Sullivan, K. J. (2010). Gait parameters associated with responsiveness to treadmill training with body-weight support after stroke: an exploratory study. Phys. Ther. 90, 209–223. doi: 10.2522/ptj.20090141
Nunes, J. P., Pina, F. L. C., Ribeiro, A. S., Cunha, P. M., Kassiano, W., Costa, B. D. V., et al. (2020). Responsiveness to muscle mass gain following 12 and 24 weeks of resistance training in older women. Aging Clin. Exp. Res. 33, 1071–1078. doi: 10.1007/s40520-020-01587-z
Ogasawara, R., Akimoto, T., Umeno, T., Sawada, S., Hamaoka, T., and Fujita, S. (2016). MicroRNA expression profiling in skeletal muscle reveals different regulatory patterns in high and low responders to resistance training. Physiol. Genomics 48, 320–324. doi: 10.1152/physiolgenomics.00124.2015
Orssatto, L. B. R., Bezerra, E. S., Zwaal, G. I., Moreira, J. D., Moro, A. R. P., de Moura, B. M., et al. (2020). Is responsiveness of elderly individuals to resistance training related to habitual nutritional intake? An exploratory analysis of a randomized controlled trial. Sports Sci. Health 16, 355–364. doi: 10.1007/s11332-020-00628-4
Pandey, A., Swift, D. L., Mcguire, D. K., Ayers, C. R., Neeland, I. J., Blair, S. N., et al. (2015). Metabolic effects of exercise training among fitness- nonresponsive patients with type 2 diabetes: the HART-D study. Diabetes Care 38, 1494–1501. doi: 10.2337/dc14-2378
Park, J. Y., Farrance, I. K. G., Fenty, N. M., Hagberg, J. M., Roth, S. M., Mosser, D. M., et al. (2007). NFKB1 promoter variation implicates shear-induced NOS3 gene expression and endothelial function in prehypertensives and stage I hypertensives. Am. J. Physiol. Hear. Circ. Physiol. 293, 2320–2327. doi: 10.1152/ajpheart.00186.2007
Patel, K., Polonsky, T. S., Kibbe, M. R., Guralnik, J. M., Tian, L., Ferrucci, L., et al. (2021). Clinical characteristics and response to supervised exercise therapy of people with lower extremity peripheral artery disease. J. Vasc. Surg. 73, 608–625. doi: 10.1016/j.jvs.2020.04.498
Peltonen, H., Walker, S., Hackney, A. C., Avela, J., and Häkkinen, K. (2018). Increased rate of force development during periodized maximum strength and power training is highly individual. Eur. J. Appl. Physiol. 118, 1033–1042. doi: 10.1007/s00421-018-3836-9
Prestes, J., da Cunha Nascimento, D., Tibana, R. A., Teixeira, T. G., Vieira, D. C. L., Tajra, V., et al. (2015). Understanding the individual responsiveness to resistance training periodization. Age 37:9793. doi: 10.1007/s11357-015-9793-x
Prestes, J., Nascimento, D. D. C., Neto, I. V. D. S., Tibana, R. A., Shiguemoto, G. E., de Andrade Perez, S. E., et al. (2018). The effects of muscle strength responsiveness to periodized resistance training on resistin, leptin, and cytokine in elderly postmenopausal women. J. Strength Cond. Res. 32, 113–120. doi: 10.1519/JSC.0000000000001718
Prud’homme, D., Bouchard, C., Leblanc, C., Landry, F., and Fontaine, E. (1984). Sensitivity of maximal aerobic power to training is genotype-dependent. Med. Sci. Sports Exerc. 16, 489–493. doi: 10.1249/00005768-198410000-00012
Radnor, J. M., Lloyd, R. S., and Oliver, J. L. (2017). Individual response to different forms of resistance training in school-aged boys. J. Strength Cond. Res. 31, 787–797. doi: 10.1519/JSC.0000000000001527
Raleigh, J. P., Giles, M. D., Islam, H., Nelms, M., Bentley, R. F., Jones, J. H., et al. (2018). Contribution of central and peripheral adaptations to changes in maximal oxygen uptake following 4 weeks of sprint interval training. Appl. Physiol. Nutr. Metab. 43, 1059–1068. doi: 10.1139/apnm-2017-0864
Raleigh, J. P., Giles, M. D., Scribbans, T. D., Edgett, B. A., Sawula, L. J., Bonafiglia, J. T., et al. (2016). The impact of work-matched interval training on VO2peak and VO2 kinetics: diminishing returns with increasing intensity. Appl. Physiol. Nutr. Metab. 41, 706–713. doi: 10.1139/apnm-2015-0614
Ramírez-Vélez, R., Hernández-Quiñones, P. A., Tordecilla-Sanders, A., Álvarez, C., Ramírez-Campillo, R., Izquierdo, M., et al. (2019). Effectiveness of HIIT compared to moderate continuous training in improving vascular parameters in inactive adults. Lipids Health Dis. 18, 1–10. doi: 10.1186/s12944-019-0981-z
Ramírez-Vélez, R., Izquierdo, M., Castro-Astudillo, K., Medrano-Mena, C., Monroy-Díaz, A. L., Castellanos-Vega, R. D. P., et al. (2020). Weight loss after 12 weeks of exercise and/or nutritional guidance is not obligatory for induced changes in local fat/lean mass indexes in adults with excess of adiposity. Nutrients 12:2231. doi: 10.3390/nu12082231
Riesco, E., Aubertin-Leheudre, M., Maltais, M. L., Audet, M., and Dionne, I. J. (2010). Synergic effect of phytoestrogens and exercise training on cardiovascular risk profile in exercise-responder postmenopausal women: a pilot study. Menopause 17, 1035–1039. doi: 10.1097/gme.0b013e3181da7915
Rohatgi, A. (2018). WebPlotDigitizer. Available online at: https://automeris.io/WebPlotDigitizer (accessed November 18, 2018).
Ross, L. M., Slentz, C. A., and Kraus, W. E. (2019). Evaluating individual level responses to exercise for health outcomes in overweight or obese adults. Front. Physiol. 10:1401. doi: 10.3389/fphys.2019.01401
Ross, R., Blair, S. N., Arena, R., Church, T. S., Després, J.-P., Franklin, B. A., et al. (2016). Importance of assessing cardiorespiratory fitness in clinical practice: a case for fitness as a clinical vital sign: a scientific statement from the American heart association. Circulation 134, e653–e699. doi: 10.1161/CIR.0000000000000461
Ross, R., de Lannoy, L., and Stotz, P. J. (2015). Separate effects of intensity and amount of exercise on interindividual cardiorespiratory fitness response. Mayo Clin. Proc. 90, 1506–1514. doi: 10.1016/j.mayocp.2015.07.024
Ross, R., Goodpaster, B. H., Koch, L. G., Sarzynski, M. A., Kohrt, W. M., Johannsen, N. M., et al. (2019). Precision exercise medicine: understanding exercise response variability. Br. J. Sports Med. 53, 1141–1153. doi: 10.1136/bjsports-2018-100328
Sandroff, B. M., Baird, J. F., Silveira, S. L., and Motl, R. W. (2019). Response heterogeneity in fitness, mobility and cognition with exercise-training in MS. Acta Neurol. Scand. 139, 183–191. doi: 10.1111/ane.13041
Saric, J., Lisica, D., Orlic, I., Grgic, J., Krieger, J. W., Vuk, S., et al. (2019). Resistance training frequencies of 3 and 6 times per week produce similar muscular adaptations in resistance-trained men. J. Strength Cond. Res. 33, S122–S129. doi: 10.1519/JSC.0000000000002909
Sawyer, B. J., Bhammar, D. M., Angadi, S. S., Ryan, D. M., Ryder, J. R., Sussman, E. J., et al. (2015). Predictors of fat mass changes in response to aerobic exercise training in women. J. Strength Cond. Res. 29, 297–304. doi: 10.1519/JSC.0000000000000726
Scharhag-Rosenberger, F., Walitzek, S., Kindermann, W., and Meyer, T. (2012). Differences in adaptations to 1 year of aerobic endurance training: individual patterns of nonresponse. Scand. J. Med. Sci. Sports 22, 113–118. doi: 10.1111/j.1600-0838.2010.01139.x
Schmid, J. P., Zurek, M., and Saner, H. (2013). Chronotropic incompetence predicts impaired response to exercise training in heart failure patients with sinus rhythm. Eur. J. Prev. Cardiol. 20, 585–592. doi: 10.1177/2047487312444368
Schubert, M. M., Palumbo, E., Seay, R. F., Spain, K. K., and Clarke, H. E. (2017). Energy compensation after sprint- and high-intensity interval training. PLoS One 12:e0189590. doi: 10.1371/journal.pone.0189590
Schulhauser, K. T., Bonafiglia, J. T., McKie, G. L., McCarthy, S. F., Islam, H., Townsend, L. K., et al. (2020). Individual patterns of response to traditional and modified sprint interval training. J. Sports Sci. 39, 1077–1087. doi: 10.1080/02640414.2020.1857507
Seward, S., Ramos, J., Drummond, C., Dalleck, A., Byrd, B., Kehmeier, M., et al. (2019). Inter-individual variability in metabolic syndrome severity score and VO2max changes following personalized, community-based exercise programming. Int. J. Environ. Res. Public Health 16:4855. doi: 10.3390/ijerph16234855
Simoneau, J. A., Lortie, G., Boulay, M. R., Marcotte, M., Thibault, M. C., and Bouchard, C. (1986). Inheritance of human skeletal muscle and anaerobic capacity adaptation to high-intensity intermittent training. Int. J. Sports Med. 7, 167–171.
Sisson, S. B., Katzmarzyk, P. T., Earnest, C. P., Bouchard, C., Blair, S. N., and Church, T. S. (2009). Volume of exercise and fitness nonresponse in sedentary, postmenopausal women. Med. Sci. Sports Exerc. 41, 539–545. doi: 10.1249/MSS.0b013e3181896c4e
Stec, M. J., Kelly, N. A., Many, G. M., Windham, S. T., Tuggle, S. C., and Bamman, M. M. (2016). Ribosome biogenesis may augment resistance training-induced myofiber hypertrophy and is required for myotube growth in vitro. Am. J. Physiol. Metab. 310, E652–E661. doi: 10.1152/ajpendo.00486.2015
Stec, M. J., Thalacker-Mercer, A., Mayhew, D. L., Kelly, N. A., Tuggle, C. S., Merritt, E. K., et al. (2017). Randomized, four-arm, dose-response clinical trial to optimize resistance exercise training for older adults with age-related muscle atrophy. Exp. Gerontol. 99, 98–109. doi: 10.1016/j.exger.2017.09.018
Steele, J., Fisher, J., Bruce-Low, S., Smith, D., Osborne, N., and Newell, D. (2017). Variability in strength, pain, and disability changes in response to an isolated lumbar extension resistance training intervention in participants with chronic low back pain. Healthcare 5:75. doi: 10.3390/healthcare5040075
Stephens, N. A., Brouwers, B., Eroshkin, A. M., Yi, F., Cornnell, H. H., Meyer, C., et al. (2018). Exercise response variations in skeletal muscle PCR recovery rate and insulin sensitivity relate to muscle epigenomic profiles in individuals with type 2 diabetes. Diabetes Care 41, 2245–2254. doi: 10.2337/dc18-0296
Stephens, N. A., Xie, H., Johannsen, N. M., Church, T. S., Smith, S. R., and Sparks, L. M. (2015). A transcriptional signature of “exercise resistance” in skeletal muscle of individuals with type 2 diabetes mellitus. Metabolism 64, 999–1004. doi: 10.1016/j.metabol.2015.06.008
Stuart, C. A., Lee, M. L., South, M. A., Howell, M. E. A., Cartwright, B. M., Ramsey, M. W., et al. (2017). Pre-training muscle characteristics of subjects who are obese determine how well exercise training will improve their insulin responsiveness. J. Strength Cond. Res. 31, 798–808. doi: 10.1519/JSC.0000000000001530
Swinton, P. A., Hemingway, B. S., Saunders, B., Gualano, B., and Dolan, E. (2018). A statistical framework to interpret individual response to intervention: paving the way for personalised nutrition and exercise prescription. Front. Nutr. 5:41. doi: 10.3389/FNUT.2018.00041
Taaffe, D. R., Newton, R. U., Spry, N., Joseph, D. J., and Galvão, D. A. (2019). Responsiveness to resistance-based multimodal exercise among men with prostate cancer receiving androgen deprivation therapy. J. Natl. Compr. Cancer Netw. 17, 1211–1220. doi: 10.6004/jnccn.2019.7311
Thomaes, T., Thomis, M., Onkelinx, S., Goetschalckx, K., Fagard, R., Lambrechts, D., et al. (2013). Genetic predisposition scores associate with muscular strength, size, and trainability. Med. Sci. Sports Exerc. 45, 1451–1459. doi: 10.1249/MSS.0b013e31828983f7
Thomas, H. J., Marsh, C. E., Maslen, B. A., Scurrah, K. J., Naylor, L. H., and Green, D. J. (2020). Studies of twin responses to understand exercise therapy (STRUETH). Med. Sci. Sports Exerc. 53, 58–67. doi: 10.1249/MSS.0000000000002461
Timmons, J. A., Jansson, E., Fischer, H., Gustafsson, T., Greenhaff, P. L., Ridden, J., et al. (2005). Modulation of extracellular matrix genes reflects the magnitude of physiological adaptation to aerobic exercise training in humans. BMC Biol. 3:19. doi: 10.1186/1741-7007-3-19
Valenzuela, P. L., de Alba, A., Pedrero-Chamizo, R., Morales, J. S., Cobo, F., Botella, A., et al. (2018). Intradialytic exercise: one size doesn’t fit all. Front. Physiol. 9:844. doi: 10.3389/fphys.2018.00844
Valenzuela, P. L., Ortiz-Alonso, J., Bustamante-Ara, N., Vidán, M. T., Rodríguez-Romo, G., Mayordomo-Cava, J., et al. (2020). Individual responsiveness to physical exercise intervention in acutely hospitalized older adults. J. Clin. Med. 9:797. doi: 10.3390/jcm9030797
Vellers, H. L., Verhein, K. C., Burkholder, A. B., Lee, J., Kim, Y., Lightfoot, J. T., et al. (2020). Association between mitochondrial DNA sequence variants and VO2 max trainability. Med. Sci. Sports Exerc. 52, 2303–2309. doi: 10.1249/MSS.0000000000002390
Vincent, H. K., Vincent, K. R., Seay, A. N., Conrad, B. P., Hurley, R. W., and George, S. Z. (2014). Back strength predicts walking improvement in obese, older adults with chronic low back pain. PM R 6, 418–426. doi: 10.1016/j.pmrj.2013.11.002
Voisin, S., Jacques, M., Lucia, A., Bishop, D. J., and Eynon, N. (2019). Statistical Considerations for exercise protocols aimed at measuring trainability. Exerc. Sports Sci. Rev. 47, 37–45. doi: 10.1249/JES.0000000000000176
Vollaard, N. B. J., Constantin-Teodosiu, D., Fredriksson, K., Rooyackers, O., Jansson, E., Greenhaff, P. L., et al. (2009). Systematic analysis of adaptations in aerobic capacity and submaximal energy metabolism provides a unique insight into determinants of human aerobic performance. J. Appl. Physiol. 106, 1479–1486. doi: 10.1152/japplphysiol.91453.2008
Wadell, K., Sundelin, G., Lundgren, R., Henriksson-Larsén, K., and Lindström, B. (2005). Muscle performance in patients with chronic obstructive pulmonary disease – effects of a physical training programme. Adv. Physiother. 7, 51–59. doi: 10.1080/14038190510010269
Walsh, J. J., Bonafiglia, J. T., Goldfield, G. S., Sigal, R. J., Kenny, G. P., Doucette, S., et al. (2020). Interindividual variability and individual responses to exercise training in adolescents with obesity. Appl. Physiol. Nutr. Metab. 45, 45–54. doi: 10.1139/apnm-2019-0088
Wang, X., Breneman, C. B., Sparks, J. R., and Blair, S. N. (2020). Sedentary time and physical activity in older women undergoing exercise training. Med. Sci. Sports Exerc. 52, 2590–2598. doi: 10.1249/MSS.0000000000002407
Weatherwax, R. M., Harris, N. K., Kilding, A. E., and Dalleck, L. C. (2018a). Using a site-specific technical error to establish training responsiveness: a preliminary explorative study. Open Access J. Sports Med. 9, 47–53. doi: 10.2147/OAJSM.S155440
Weatherwax, R. M., Harris, N. K., Kilding, A. E., and Dalleck, L. C. (2019). Incidence of VO2max responders to personalized versus standardized exercise prescription. Med. Sci. Sports Exerc. 51, 681–691. doi: 10.1249/MSS.0000000000001842
Weatherwax, R. M., Ramos, J. S., Harris, N. K., Kilding, A. E., and Dalleck, L. C. (2018b). Changes in metabolic syndrome severity following individualized versus standardized exercise prescription: a feasibility study. Int. J. Environ. Res. Public Health 15, 1–14. doi: 10.3390/ijerph15112594
Williams, C. J., Gurd, B. J., Bonafiglia, J. T., Voisin, S., Li, Z., Harvey, N., et al. (2019). A multi-center comparison of VO2peak trainability between interval training and moderate intensity continuous training. Front. Physiol. 10:19. doi: 10.3389/fphys.2019.00019
Williamson, P. J., Atkinson, G., and Batterham, A. M. (2017). Inter-individual responses of maximal oxygen uptake to exercise training: a critical review. Sports Med. 47, 1501–1513. doi: 10.1007/s40279-017-0680-8
Williamson, P. J., Atkinson, G., and Batterham, A. M. (2018). Inter-individual differences in weight change following exercise interventions?: a systematic review and meta- analysis of randomized controlled trials. Obes. Rev. 19, 960–975. doi: 10.1111/obr.12682
Wilson, J. R., Groves, J., and Rayos, G. (1996). Circulatory status and response to cardiac rehabilitation in patients with heart failure. Circulation 94, 1567–1572. doi: 10.1161/01.CIR.94.7.1567
Winkelbeiner, S., Leucht, S., Kane, J. M., and Homan, P. (2019). Evaluation of differences in individual treatment response in schizophrenia spectrum disorders. JAMA Psychiatry 76:1063. doi: 10.1001/jamapsychiatry.2019.1530
Witvrouwen, I., Pattyn, N., Gevaert, A. B., Possemiers, N., Van Craenenbroeck, A. H., Cornelissen, V. A., et al. (2019). Predictors of response to exercise training in patients with coronary artery disease – a subanalysis of the SAINTEX-CAD study. Eur. J. Prev. Cardiol. 26, 1158–1163. doi: 10.1177/2047487319828478
Wolpern, A. E., Burgos, D. J., Janot, J. M., and Dalleck, L. C. (2015). Is a threshold-based model a superior method to the relative percent concept for establishing individual exercise intensity? A randomized controlled trial. BMC Sports Sci. Med. Rehabil. 7:16. doi: 10.1186/s13102-015-0011-z
Wormgoor, S. G., Dalleck, L. C., Zinn, C., Borotkanics, R., and Harris, N. K. (2018). High-intensity interval training is equivalent to moderate-intensity continuous training for short- and medium-term outcomes of glucose control, cardiometabolic risk, and microvascular complication markers in men with type 2 diabetes. Front. Endocrinol. 9:475. doi: 10.3389/fendo.2018.00475
Yalamanchi, S. V., Stewart, K. J., Ji, N., Golden, S. H., Dobs, A., Becker, D. M., et al. (2016). The relationship of fasting hyperglycemia to changes in fat and muscle mass after exercise training in type 2 diabetes. Diabetes Res. Clin. Pract. 122, 154–161. doi: 10.1016/j.diabres.2016.09.026
Yan, X., Eynon, N., Papadimitriou, I. D., Kuang, J., Munson, F., Tirosh, O., et al. (2017). The gene SMART study: method, study design, and preliminary findings. BMC Genomics 18:821. doi: 10.1186/s12864-017-4186-4
Yoo, J., Kim, B. H., Kim, S. H., Kim, Y., and Yim, S. V. (2016). Genetic polymorphisms to predict gains in maximal O2 uptake and knee peak torque after a high intensity training program in humans. Eur. J. Appl. Physiol. 116, 947–957. doi: 10.1007/s00421-016-3353-7
Yu, F., Salisbury, D., and Mathiason, M. A. (2020). Interindividual differences in the responses to aerobic exercise in Alzheimer’s disease: findings from the FIT-AD trial. J. Sports Heal. Sci. 10, 65–72. doi: 10.1016/j.jshs.2020.05.007
Appendix
In-Text Citations for the 149 Included Studies
References A-I:
(Bouchard et al., 1990, 1994, 1990, 2012; Dionne et al., 1991; Barbeau et al., 2003; Boule et al., 2005; Hubal et al., 2005; Hautala et al., 2006; Gross et al., 2007; Caudwell et al., 2009; Erskine et al., 2010; Davidsen et al., 2011; Croymans et al., 2013; Astorino and Schubert, 2014; Goddard et al., 2014; Chmelo et al., 2015; Churchward-Venne et al., 2015; Dalleck et al., 2015, 2016; Ahtiainen et al., 2016, 2020; Bonafiglia et al., 2016, 2018, 2021a,b; Garcia et al., 2016; Gurd et al., 2016; Hauser et al., 2016; Alvarez et al., 2017a,b,c; Barbalho et al., 2017; Chen et al., 2017; de Lannoy et al., 2017; Hvid et al., 2017; Astorino et al., 2018; Álvarez et al., 2018a,b,c,d, 2019; Bakker et al., 2018; Cadore et al., 2018; Díaz-Vegas et al., 2018; Edgett et al., 2018; Gray et al., 2018; Hagstrom and Denham, 2018; Hecksteden et al., 2018; Horak et al., 2018; Brennan et al., 2019; Byrd et al., 2019; Castro et al., 2019; Damas et al., 2019a,b; Delgado-Floody et al., 2019; Denham, 2019; Hammond et al., 2019; Haun et al., 2019; Islam et al., 2019; Del Giudice et al., 2020).
References I-R:
(Lortie et al., 1984; Prud’homme et al., 1984; Koufaki et al., 2002; Leon et al., 2002; Park et al., 2007; King et al., 2008; McPhee et al., 2010, 2011; Mulroy et al., 2010; Riesco et al., 2010; Karavirta et al., 2011, 2013; Keller et al., 2011; Kacerovsky-Bielesz et al., 2012; Johannsen et al., 2013; Mueller et al., 2015; Pandey et al., 2015; Prestes et al., 2015, 2018; Ross et al., 2015; Leddy et al., 2016; Leifer et al., 2016; Metcalfe et al., 2016; Ogasawara et al., 2016; Raleigh et al., 2016, 2018; Montero and Lundby, 2017; Radnor et al., 2017; da Cunha Nascimento et al., 2018, 2020; Leoñska-Duniec et al., 2018; Mclaren et al., 2018; Mobley et al., 2018; Morton et al., 2018; Peltonen et al., 2018; Kneppers et al., 2019; Massie et al., 2019; Ramírez-Vélez et al., 2019, 2020; Ross L. M. et al., 2019; Krusnauskas et al., 2020; Marsh et al., 2020; Medrano et al., 2020; Nunes et al., 2020; Orssatto et al., 2020; Patel et al., 2021).
References S-Z:
(Simoneau et al., 1986; Wilson et al., 1996; Timmons et al., 2005; Wadell et al., 2005; Sisson et al., 2009; Vollaard et al., 2009; Scharhag-Rosenberger et al., 2012; Schmid et al., 2013; Thomaes et al., 2013; Vincent et al., 2014; Sawyer et al., 2015; Stephens et al., 2015, 2018; Wolpern et al., 2015; Stec et al., 2016, 2017; Yalamanchi et al., 2016; Yoo et al., 2016; Schubert et al., 2017; Steele et al., 2017; Stuart et al., 2017; Williamson et al., 2017, 2018; Yan et al., 2017; Valenzuela et al., 2018, 2020; Weatherwax et al., 2018a,b, 2019; Wormgoor et al., 2018; Sandroff et al., 2019; Saric et al., 2019; Seward et al., 2019; Taaffe et al., 2019; Williams et al., 2019; Witvrouwen et al., 2019; Schulhauser et al., 2020; Thomas et al., 2020; Vellers et al., 2020; Walsh et al., 2020; Wang et al., 2020; Yu et al., 2020).
Keywords: individual response, interindividual variability, trainability, exercise training, responders, non-responder analysis
Citation: Bonafiglia JT, Preobrazenski N and Gurd BJ (2021) A Systematic Review Examining the Approaches Used to Estimate Interindividual Differences in Trainability and Classify Individual Responses to Exercise Training. Front. Physiol. 12:665044. doi: 10.3389/fphys.2021.665044
Received: 06 February 2021; Accepted: 05 October 2021;
Published: 08 November 2021.
Edited by:
Stefano Ramat, University of Pavia, ItalyReviewed by:
Brendan Egan, Dublin City University, IrelandDaniel Muniz, University of Hertfordshire, United Kingdom
Copyright © 2021 Bonafiglia, Preobrazenski and Gurd. This is an open-access article distributed under the terms of the Creative Commons Attribution License (CC BY). The use, distribution or reproduction in other forums is permitted, provided the original author(s) and the copyright owner(s) are credited and that the original publication in this journal is cited, in accordance with accepted academic practice. No use, distribution or reproduction is permitted which does not comply with these terms.
*Correspondence: Brendon J. Gurd, gurdb@queensu.ca
†These authors have contributed equally to this work and share first authorship