- 1Department of Epileptology, University of Bonn Medical Centre, Bonn, Germany
- 2Helmholtz Institute for Radiation and Nuclear Physics, University of Bonn, Bonn, Germany
- 3Interdisciplinary Center for Complex Systems, University of Bonn, Bonn, Germany
The field of Network Physiology aims to advance our understanding of how physiological systems and sub-systems interact to generate a variety of behaviors and distinct physiological states, to optimize the organism's functioning, and to maintain health. Within this framework, which considers the human organism as an integrated network, vertices are associated with organs while edges represent time-varying interactions between vertices. Likewise, vertices may represent networks on smaller spatial scales leading to a complex mixture of interacting homogeneous and inhomogeneous networks of networks. Lacking adequate analytic tools and a theoretical framework to probe interactions within and among diverse physiological systems, current approaches focus on inferring properties of time-varying interactions—namely strength, direction, and functional form—from time-locked recordings of physiological observables. To this end, a variety of bivariate or, in general, multivariate time-series-analysis techniques, which are derived from diverse mathematical and physical concepts, are employed and the resulting time-dependent networks can then be further characterized with methods from network theory. Despite the many promising new developments, there are still problems that evade from a satisfactory solution. Here we address several important challenges that could aid in finding new perspectives and inspire the development of theoretic and analytical concepts to deal with these challenges and in studying the complex interactions between physiological systems.
1. Introduction
Network physiology (Bartsch et al., 2012, 2015; Bashan et al., 2012; Ivanov et al., 2016) is a novel transdisciplinary research approach that focuses on how physiological systems and subsystems interact, thereby complementing the traditional approaches from systems biology and integrative physiology. Conceptually, it considers the human organism as an evolving complex network—a radically reduced description where the full system is described by an interaction network, whose vertices represent distinct physiological subsystems and whose edges represent time-dependent, observation-derived interactions between them (see Figure 1). This reduced description has been utilized in a number of scientific disciplines, and research over the last two decades has demonstrated that the network paradigm can advance our understanding of natural and man-made complex dynamical systems (see e.g., Boccaletti et al., 2006, 2014; Arenas et al., 2008; Barthélemy, 2011; Holme and Saramäki, 2012; Bassett and Sporns, 2017; Halu et al., 2019 for an overview). Although encouraging, the data-driven network approach to the human organism faces a number of challenges. Conceptually, the inference of interactions from observation of the organism's dynamics constitutes a fundamental inverse problem, which has no unique solution (von Helmholtz, 1853). State-of-the-art reconstruction methods require access to a model of the human organism or dynamical data at a preciseness that is not available. Another and more practicable path that is often taken in the network sciences, including network physiology, encompasses (i) a time-series-analysis-based characterization of interactions between all pairs of subsystems, (ii) a derivation of a network from estimated characteristics, and (iii) a characterization of the network with methods from graph theory. In the following, we discuss important challenges of this path from pairwise interactions to interaction networks.
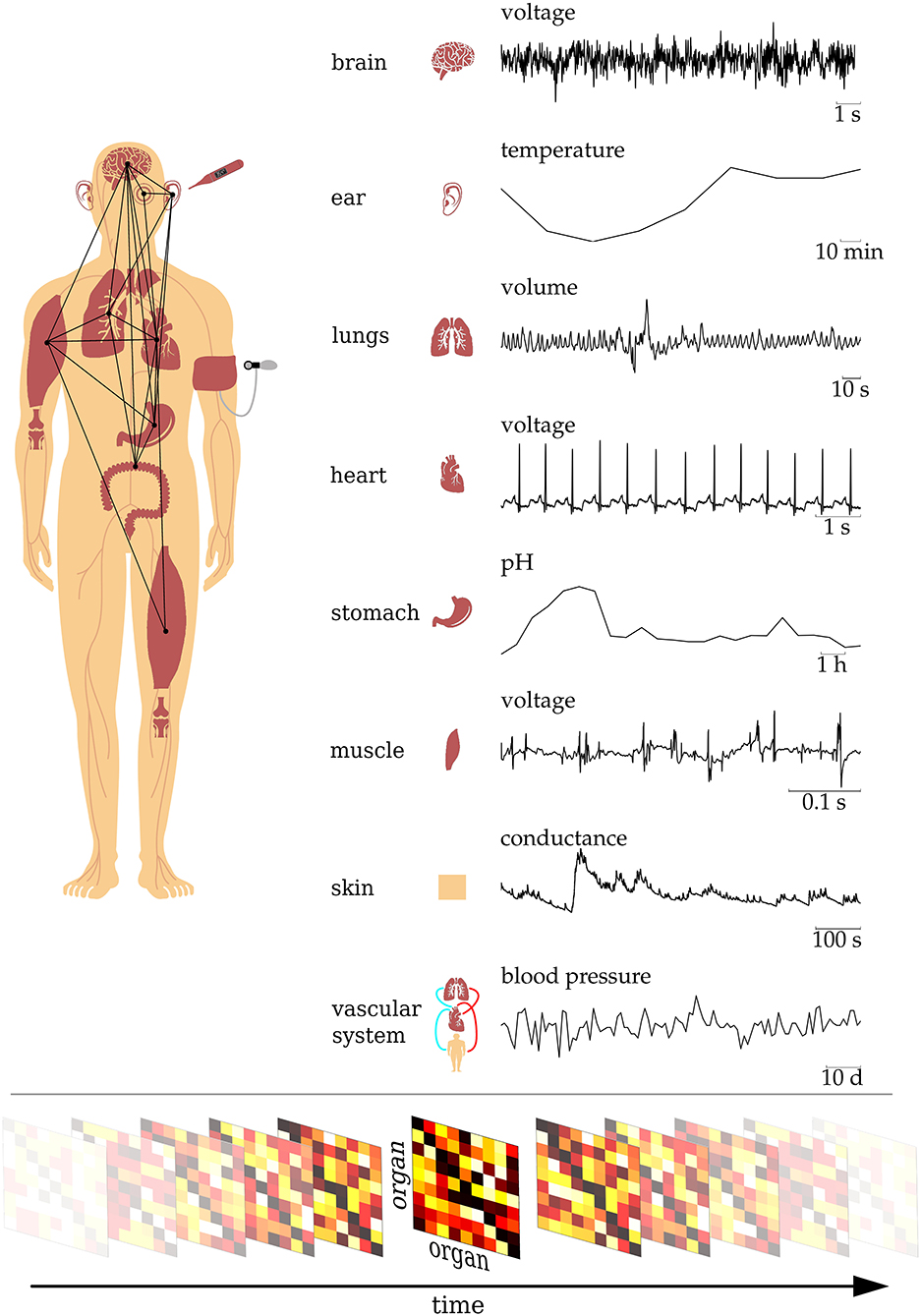
Figure 1. Schematic of the human organism as an evolving complex network of dynamical interactions between organ systems. The dynamics of different organs exhibit a broad range of timescales, and physiological observables are typically based on different physical and/or chemical quantities. Time-dependent organ-organ interaction matrices are derived from a time-resolved time-series-analysis-based characterization of interactions from all pairs of observables. These matrices represent a network that evolves in time, with nodes representing organs and edges representing time-varying interactions between them.
2. Challenges With Characterizing Interactions
The characterization of interactions between physiological systems faces several challenges:
• We often do not know exactly the systems' equations of motion;
• We lack knowledge as to how to merge/combine these equations (e.g., due to the issue of time-scale matching);
• We may have insufficient knowledge about relevant structural connections;
• We may not have direct access to interactions between systems (e.g., via probing).
Due to these (and possibly other) limitations, usually linear and non-linear time-series-analysis techniques are employed to quantify interaction properties from pairs of time series of appropriate system observables. Since interactions can manifest themselves in various aspects of the dynamics, analysis techniques originate from diverse fields such as statistics, synchronization theory, non-linear dynamics, information theory, statistical physics, and from the theory of stochastic processes (for an overview, see Pikovsky et al., 2001; Kantz and Schreiber, 2003; Reinsel, 2003; Pereda et al., 2005; Hlaváčková-Schindler et al., 2007; Marwan et al., 2007; Friedrich et al., 2011; Lehnertz, 2011; Lehnertz et al., 2014; Müller et al., 2016; Stankovski et al., 2017; Tabar, 2019). Interactions may impact on amplitudes, phases, frequencies, or even combinations thereof and for some cases it might be more efficient to consider interactions as flow of information. Beyond that, a more detailed characterization of interactions can in general be achieved with state-space-based approaches and with approaches that even allow for interactions in the stochastic (rather than the deterministic) part of the dynamics (Prusseit and Lehnertz, 2008; Rydin Gorjão et al., 2019).
2.1. Data-Driven Assessment of Pairwise Interaction Properties
Common linear time-series-analysis techniques (Carter, 1987) such as estimating the linear correlation coefficient, cross-correlation and cross-spectral functions as well as (linear) partial coherence are often used but can mostly provide information about the strength of an interaction since correlation does not imply causation. Linear indices for the direction of an interaction are usually based on the concepts of Granger causality (Seth et al., 2015) or partial directed coherence (Baccalá and Sameshima, 2001; Schelter et al., 2006b) that make use of parametric approaches to estimate (single and joint) properties of the power spectra (Lütkepohl, 2005). Note that linear approaches to characterize interactions are mostly based on amplitudes, and these approaches may not adequately account for the well-known non-linearities in physiological systems (Elbert et al., 1994; West, 2012).
Common non-linear time-series-analysis techniques can be subdivided into two main categories depending on the underlying concept for interaction: synchronization-based (SB) techniques (Pikovsky et al., 2001; Boccaletti et al., 2002; Stankovski et al., 2017) and information-theory-based (IB) techniques (Hlaváčková-Schindler et al., 2007; Amblard and Michel, 2013). SB techniques aim at assessing aspects of generalized synchronization (Čenys et al., 1991; Rulkov et al., 1995; Arnhold et al., 1999) or of phase synchronization (Huygens, 1673; Rosenblum et al., 1996). For generalized synchronization, at first the state spaces of the systems need to be reconstructed from time series of system observables (Kantz and Schreiber, 2003). This allows one to exploit various geometric or dynamic properties to quantify strength and direction of interactions (Arnhold et al., 1999; Pikovsky et al., 2001; Boccaletti et al., 2002; Marwan et al., 2007; Faes et al., 2008; Chicharro and Andrzejak, 2009). For phase synchronization, phase time series of the systems need to be derived from the time series of observables, and there are various approaches that allow one to extract phases from noisy broadband signals (see e.g., Bruns, 2004; Kralemann et al., 2008; Schwabedal and Kantz, 2016). The strength of interactions can then be estimated by exploiting phase differences (Tass et al., 1998; Lachaux et al., 1999; Mormann et al., 2000), and the direction of interactions can be quantified via a phase modeling approach (Rosenblum and Pikovsky, 2001; Smirnov, 2014). Recently, methods have been developed that allow the detection and reconstruction of coupling functions from measured data (Stankovski et al., 2017; Pietras and Daffertshofer, 2019; Rosenblum and Pikovsky, 2019; Bick et al., 2020). IB techniques aim at identifying common information contained in the systems' time series of observables as this would allow one to infer the direction of interaction (“causal relationships”) between systems (Schreiber, 2000; Staniek and Lehnertz, 2008; Vicente et al., 2011; Smirnov, 2014; Timme et al., 2014; Porta and Faes, 2015; Runge, 2018). Note that these techniques are only occasionally used to infer the strength of interaction (e.g., Liu, 2004; Monetti et al., 2009; Jafri et al., 2016), and we are missing techniques to detect and reconstruct coupling functions.
Despite the different concepts and the many time-series-analysis techniques, a discussion about their relative merit lasting for more than 15 years indicates that there is probably no single approach which is best suited to characterize properties of interactions between physiological systems (Smirnov and Andrzejak, 2005; Ansari-Asl et al., 2006; Kreuz et al., 2007; Paluš and Vejmelka, 2007; Smirnov et al., 2007; Osterhage et al., 2007a,b, 2008; Vejmelka and Paluš, 2008; Wendling et al., 2009; Florin et al., 2011; Wang et al., 2014; Zhou et al., 2014; Hirata et al., 2016; Stokes and Purdon, 2017; Xiong et al., 2017; Barnett et al., 2018; Beauchene et al., 2018; Dhamala et al., 2018; Krakovská et al., 2018; Bakhshayesh et al., 2019).
2.2. Current Limitations to a Data-Driven Assessment of Pairwise Interaction Properties
Conceptually, the majority of the aforementioned time-series-analysis techniques assumes the investigated systems to be stationary (or at least approximately stationary) and the interactions to be stable and persisting throughout the observation time. By their very nature, however, physiological systems are inherently non-stationary (Marmarelis, 2012) and interactions between them are mostly transient. In some cases, even multiple forms of couplings can coexist (Bartsch et al., 2014; Klimesch, 2018). So far, only a few time-series-analysis techniques were developed to characterize transient interactions between pairs of systems (Hesse et al., 2003; Andrzejak et al., 2006; Faes et al., 2008; Wagner et al., 2010; Hempel et al., 2011; Lehnertz, 2011; Martini et al., 2011; Bartsch et al., 2012; Ma et al., 2014; Liu et al., 2015; Lin et al., 2016; Kostoglou et al., 2019), and it is not yet clear whether there is one single approach that is best suited to characterize all relevant properties of transient interactions between non-stationary physiological systems.
Most physiological systems operate on very different time scales (an der Heiden, 1979; Batzel and Kappel, 2011; Gosak et al., 2018) (cf. Figure 1), and due to distance- and function-related characteristic features, delayed interactions need to be taken into account. The exact delay between physiological systems is usually not known a priori and may be time-dependent. Time-series-analysis techniques designed to characterize delayed interactions thus make use of exhaustive/brute force search methods to identify potential delay(s) (Müller et al., 2003; Silchenko et al., 2010; Dickten and Lehnertz, 2014; Faes et al., 2014; Ye et al., 2015; Lin et al., 2016; Coufal et al., 2017; Ma et al., 2017; Li et al., 2018; Rosinberg et al., 2018). The, in general, high computational burden may limit real-time analyses of delayed interactions. Addressing the issue of different time scales, methods have been proposed recently that aim at a multiscale description of interacting systems (Lungarella et al., 2007; Ahmed and Mandic, 2011; Humeau-Heurtier, 2016; Faes et al., 2017; Paluš, 2019; Jamin and Humeau-Heurtier, 2020).
Interpreting findings from pairwise interaction measurements is a challenging task. Among others, statistical fluctuations and systematic errors may impinge on findings of some interaction property. Moreover, misapplying or misinterpreting time-series-analysis techniques may lead to inappropriate conclusions. Surrogate testing is a crucial tool to ensure the reliability of the results (Schreiber and Schmitz, 2000). Nevertheless, although extensions and new development of surrogate techniques can help to avoid misinterpretations about the strength of an interaction (Andrzejak et al., 2003; Lancaster et al., 2018; Ricci et al., 2019), causal relationships are notoriously difficult to identify (Mayr, 1961; Laland et al., 2011). Although some approaches have been proposed to test the significance of directionality indices (Thiel et al., 2006; Romano et al., 2009; Faes et al., 2010; Jelfs and Chan, 2017), we still lack reliable surrogate techniques for directionality indices as well as for techniques to detect and characterize coupling functions.
3. Challenges With Deriving and Characterizing an Integrated Network of Physiological Subsystems
Network physiology considers the human organism as an integrated network, whose vertices are associated with distinct physiological subsystems (i.e., different organs) and edges represent time-varying interactions between vertices. This initial assignment of vertices and edges can have major implications on how an integrated network of interacting physiological subsystems is configured and interpreted (Butts, 2009; Bialonski et al., 2010; Hlinka et al., 2012; Timme and Casadiego, 2014; Wens, 2015; Papo et al., 2016; Nitzan et al., 2017), and a number of challenges arise when identifying and quantifying networks of diverse subsystems with different types of interactions.
3.1. Vertices
The definition of vertices of the spatially extended dynamical system human organism is notoriously difficult. Although the assignment of vertices to distinct physiological subsystems appears rather intuitive, in practice, vertices are usually associated with sensors that are assumed to be placed such that they sufficiently capture the dynamics of subsystems. This ansatz, which is often not even questioned, requires appropriate spatial and temporal sampling strategies, insights into the physical processes and the statistical properties of the system. Identifying adequate sampling strategies is closely related to issues such as accessibility and non-invasiveness and, more importantly, to what is actually a good observable for a given organ to allow insights into the relevant physical processes. Often used physiological observables range from electric and/or magnetic fields to thermodynamic properties such as temperature, pressure, or volumes as well as to chemical properties such as pH or concentration (cf. Figure 1). Observables often dictate the type of sensor, and there might be limitations concerning their size, positioning, or combinability. Due to their very nature, physiological observables can capture vastly different timescales, ranging from milliseconds to days and months, and we lack appropriate concepts and analysis techniques to match these timescales. Recordings of observables are typically noisy and prone to technical and physiological artifacts.
For single organs, there exists a large number of guidelines and recommendations for the sampling of their activities (e.g., Camm et al., 1996; Kligfield et al., 2007; Seeck et al., 2017; Harford et al., 2019; Tankisi et al., 2020). Nevertheless, with the development of novel sensing technologies (Andreu-Perez et al., 2015), guidelines and recommendations are often challenged (Trägårdh et al., 2006; Garćıa-Niebla et al., 2009; Xia et al., 2012; Grover and Venkatesh, 2016), and by now, we lack commonly accepted guidelines for the spatial and temporal sampling of interactions between different organs to allow insights into the relevant physical processes and the statistical properties of the human organism.
An alternative ansatz, which is often pursued in the neurosciences and in cardiology, would consist in replacing estimations of interaction properties in sensor-space with those in source-space (see e.g., Van Mierlo et al., 2019 and references therein). This approach requires localizing the sources of electric/magnetic activities that generate the potentials/fields that can be recorded non-invasively on the surface of the body. It constitutes another inverse problem with early explorations dating back to the 1950s using electric field theory. The lack of a unique solution to this inference problem is reflected by a large set of analysis methods that were developed since then to find an appropriate approximation (Jatoi et al., 2014).
3.2. Edges
A natural way to define edges of the networked human organism would be to relate them to structural connections within and between physiological subsystems (e.g., synapses, nerve tracts, or the lymph or blood stream). Since we lack non-invasive access to these structural edges and their dynamics, a widely used ansatz is to infer functional edges via a data-driven assessment of pairwise interaction properties from the subsystems' dynamics using the aforementioned time-series-analysis techniques in an attempt to elucidate the underlying coupling mechanisms (cf. Figure 1). Note that there are by now no commonly accepted genuine multivariate approaches to assess interactions properties from the dynamics of more than two physiological subsystems. Moreover, the assessment may be hampered by the as yet unsolved problem to reliably distinguish between direct and indirect interactions, with the latter being mediated by another—even unobserved—(sub-)system. This can lead to serious misinterpretations of possible causal relationships. The severity of this issue is expressed in a large number of time-series-analysis techniques—based on partialization analysis—that have been proposed over the last two decades to overcome this problem of transitivity (see e.g., Langford et al., 2001; Eichler et al., 2003; Chen et al., 2004; Schelter et al., 2006a,b; Frenzel and Pompe, 2007; Smirnov and Bezruchko, 2009; Vakorin et al., 2009; Nawrath et al., 2010; Jalili and Knyazeva, 2011; Zou et al., 2011; Runge et al., 2012; Stramaglia et al., 2012; Kugiumtzis, 2013; Leistritz et al., 2013; Ramb et al., 2013; Kralemann et al., 2014; Elsegai et al., 2015; Faes et al., 2015; Mader et al., 2015; Zhao et al., 2016; Leng et al., 2020; Marinazzo et al., 2012). All these techniques involve estimating interaction properties between two systems, holding constant the external influences of a third. Their efficiency, however, is severely limited by volume conduction effects, asymmetric signal-to-noise ratios (Albo et al., 2004; Nolte et al., 2004; Xu et al., 2006) as well as by the number of interacting subsystems and the density of connections between them (Rubido et al., 2014; Zerenner et al., 2014; Rings and Lehnertz, 2016).
Spurious indications of strength and direction of interactions can be considered as another related issue which can lead to severe misinterpretations. These indications can result from an instantaneous mixture of activities, i.e., a common source, which may be caused by, e.g., a too close spatial sampling of some organ with multiple sensors. Likewise, it may be due to an—often unavoidable—referential recording as in case of measurements of an organ's electric fields. While a number of proposed extensions to and modification of particularly phase-based time-series-analysis techniques (Stam et al., 2007; Vinck et al., 2011; Stam and van Straaten, 2012; Hardmeier et al., 2014) appear to be less affected by such influences, their general suitability, however, continues to be matter of debate (Yu and Boccaletti, 2009; Peraza et al., 2012; Gordon et al., 2013; Porz et al., 2014; Colclough et al., 2016).
3.3. Choosing the Type of Network
Once edges and vertices are defined sufficiently, they are then used to set up a binary or weighted and undirected or directed network, depending on which interaction properties between physiological subsystems have been characterized. An undirected binary network characterizes interacting physiological subsystems in terms of connected or disconnected. For such a network, a pair of subsystems is said to be connected by an edge, if an estimated strength of interaction exceeds some threshold. Despite the simplicity of this ansatz, we still lack commonly accepted criteria for the choice of the threshold (Ioannides, 2007; Kramer et al., 2009; Rubinov and Sporns, 2010; Zanin et al., 2012; Fornito et al., 2013).
An undirected weighted network characterizes interacting physiological subsystems in terms of how strongly they interact with each other. In such a network, all edges are usually considered to exist, again due to the lack of a reliable definition of a threshold to exclude edges with non-significant interaction strengths. Commonly, the weight of an edge and the estimated strength of an interaction between vertices connected by that edge are set to be equal. While many estimators for the strength of an interaction are normalized, in general, the weight matrix associated with the weighted network is not; hence, it is advisable to suitably normalize this matrix. Furthermore, the distribution of estimated strengths of interaction can have a dominant effect on network properties of interest and need to be taken into account (Ansmann and Lehnertz, 2012; Stahn and Lehnertz, 2017).
Adding information about the direction of interaction to a binary network expands this to a directed binary network. As in the undirected case, an appropriately chosen threshold may help to separate significant from non-significant indications of directionality. Even more problematic, the modulus of an estimator for the direction of an interaction typically lacks physical interpretability; often only the sign indicates the direction.
Deriving a weighted and directed network by merging both interaction properties—strength and direction—would be preferable, as such a network conveys most information about interacting physiological subsystems. As yet, this task is not solved in a conclusive manner and one needs to keep in mind that strength and direction are distinct but related properties of interactions (Elsegai et al., 2015; Lehnertz and Dickten, 2015; Dickten et al., 2016). While in some specific situations the modulus of an estimator for the direction of an interaction might be interpreted as strength of an interaction, this is not generally valid and has been shown to lead to severe misinterpretations, particularly for uncoupled and for strongly coupled systems (Osterhage et al., 2008; Lehnertz and Dickten, 2015). Both interaction properties should thus be estimated separately but using analysis techniques that based on the same concept (e.g., synchronization theory or information theory). A mixing of different concepts might be ill-advised, as it remains unclear how different concepts translate to each other (Dickten et al., 2016). Moreover, there is no commonly accepted method for how weights should be allocated to an edge's forward and backward direction. While the strength of an interaction has no directionality and is consequently invariant under exchange of vertices, the direction of an interaction is not.
3.4. Network Characterization
Graph theory provides a large spectrum of approaches that can be used to characterize an integrated network of physiological subsystems (see e.g., Boccaletti et al., 2006; Arenas et al., 2008; Fortunato, 2010; Barthélemy, 2011; Newman, 2012, for an overview). Characteristics range from local ones, which describe properties of network constituents, e.g., individual vertices or edges to global ones, which assess properties of the network as a whole. Most characteristics, however, were initially developed for binary networks, and an extension to weighted and/or directed networks is usually not straightforward. As an example, consider the shortest path between two vertices l and k in a binary network which is the smallest number of edges one has to traverse to reach vertex l from vertex k. The length of a single path between two vertices in a weighted network is oftentimes defined as the inverse of the edge weight. This definition relies on the observation that the ratio between the weights of two edges equals the ratio between their lengths; other definitions, however, might be equally valid. Influencing factors such as common sources and indirect interactions were shown to impact on the definition of shortest paths (Ioannides, 2007; Bialonski et al., 2011). Similar arguments hold for the clustering coefficient; despite several suggestions for an extension to weighted (Saramäki et al., 2007) and directed networks (Fagiolo, 2007), their suitability for the analysis of an integrated network of physiological subsystems remains to be shown.
Clustering coefficient and mean shortest path are oftentimes used to decide upon a network's small-worldness (Bassett and Bullmore, 2006), and this property has repeatedly been reported for networks from diverse scientific disciplines. Given the many factors that impact on clustering coefficient and mean shortest path, however, these findings continue to be matter of considerable debate (Bialonski et al., 2010; Gastner and Ódor, 2016; Hilgetag and Goulas, 2016; Papo et al., 2016; Hlinka et al., 2017; Zanin et al., 2018).
Since characteristics of networks (as well as of time series from which networks were derived) can be affected by a number of influencing factors, surrogate testing can be applied to eliminate or at least minimize those influences (Schreiber and Schmitz, 2000; Stahn and Lehnertz, 2017). Although such an approach is strongly recommended to avoid severe misinterpretations, we lack surrogate schemes that are appropriate for networks of interacting physiological subsystems and that address the challenges referred to here.
Eventually, an integrated network of physiological subsystems can be regarded as an evolving network, whose vertices (and/or edges) change with time. Although it is of utmost importance to understand how the network changes from time step to time step, its investigation requires appropriate methods that would allow a comparison of networks (Mheich et al., 2020). Developing such methods, however, is highly non-trivial, since a network's topological properties necessarily depend on the number of edges and the number of vertices. When both quantities change with time, an unbiased comparison between networks remains difficult.
4. Conclusion and Summary
The challenges arising on the path from pairwise interactions to interaction networks call for concerted efforts of all involved communities to advance network physiology. There is an urgent need for sensing concepts and technologies that allow time-locked recordings of relevant physiological observables thereby taking into account their various physical origins as well as their vastly different time scales. Similarly, appropriate concepts and analysis techniques need to be developed to match these time scales and to allow multimodal data fusion (Lahat et al., 2015). Time-series-analysis techniques require further improvements to allow an unambiguous characterization of properties of interactions between more than two systems and under the constraints related to investigating the human organism during (patho-)physiological conditions. Ultimately, the strong heterogeneity of organs and their dynamics calls for better suited network concepts (e.g., based on multilayer/multiplex networks, Boccaletti et al., 2014; Kivelä et al., 2014; Castellani et al., 2016) and possibly requires novel network characteristics and statistical tools. To be successful, these efforts should be scrutinized with the question whether the network framework tells us anything new about the human organism we did not knew before.
Data Availability Statement
The original contributions presented in the study are included in the article/supplementary material, further inquiries can be directed to the corresponding author/s.
Author Contributions
All authors conceived the research project and wrote the paper. All authors contributed to the article and approved the submitted version.
Funding
This work was supported by the Deutsche Forschungsgemeinschaft (Grant No: LE660/7-1) and by the Verein zur Foerderung der Epilepsieforschung e.V. (Bonn).
Conflict of Interest
The authors declare that the research was conducted in the absence of any commercial or financial relationships that could be construed as a potential conflict of interest.
References
Ahmed, M. U., and Mandic, D. P. (2011). Multivariate multiscale entropy: a tool for complexity analysis of multichannel data. Phys. Rev. E 84:061918. doi: 10.1103/PhysRevE.84.061918
Albo, Z., Di Prisco, G. V., Chen, Y., Rangarajan, G., Truccolo, W., Feng, J., et al. (2004). Is partial coherence a viable technique for identifying generators of neural oscillations? Biol. Cybern. 90, 318–326. doi: 10.1007/s00422-004-0475-5
Amblard, P.-O., and Michel, O. J. (2013). The relation between Granger causality and directed information theory: a review. Entropy 15, 113–143. doi: 10.3390/e15010113
Andreu-Perez, J., Leff, D. R., Ip, H. M., and Yang, G.-Z. (2015). From wearable sensors to smart implants—toward pervasive and personalized healthcare. IEEE Trans. Biomed. Eng. 62, 2750–2762. doi: 10.1109/TBME.2015.2422751
Andrzejak, R. G., Kraskov, A., Stögbauer, H., Mormann, F., and Kreuz, T. (2003). Bivariate surrogate techniques: necessity, strengths, and caveats. Phys. Rev. E 68:066202. doi: 10.1103/PhysRevE.68.066202
Andrzejak, R. G., Ledberg, A., and Deco, G. (2006). Detecting event-related time-dependent directional couplings. New J. Phys. 8:6. doi: 10.1088/1367-2630/8/1/006
Ansari-Asl, K., Senhadji, L., Bellanger, J.-J., and Wendling, F. (2006). Quantitative evaluation of linear and nonlinear methods characterizing interdependencies between brain signals. Phys. Rev. E 74:031916. doi: 10.1103/PhysRevE.74.031916
Ansmann, G., and Lehnertz, K. (2012). Surrogate-assisted analysis of weighted functional brain networks. J. Neurosci. Methods 208, 165–172. doi: 10.1016/j.jneumeth.2012.05.008
Arenas, A., Díaz-Guilera, A., Kurths, J., Moreno, Y., and Zhou, C. (2008). Synchronization in complex networks. Phys. Rep. 469, 93–153. doi: 10.1016/j.physrep.2008.09.002
Arnhold, J., Grassberger, P., Lehnertz, K., and Elger, C. E. (1999). A robust method for detecting interdependences: application to intracranially recorded EEG. Phys. D 134, 419–430.
Baccalá, L. A., and Sameshima, K. (2001). Partial directed coherence: a new concept in neural structure determination. Biol. Cybern. 84, 463–474. doi: 10.1007/PL00007990
Bakhshayesh, H., Fitzgibbon, S. P., Janani, A. S., Grummett, T. S., and Pope, K. J. (2019). Detecting connectivity in EEG: a comparative study of data-driven effective connectivity measures. Comput. Biol. Med. 111:103329. doi: 10.1016/j.compbiomed.2019.103329
Barnett, L., Barrett, A. B., and Seth, A. K. (2018). Solved problems for Granger causality in neuroscience: a response to Stokes and Purdon. Neuroimage 178, 744–748. doi: 10.1016/j.neuroimage.2018.05.067
Bartsch, R. P., Liu, K. K., Ma, Q. D., and Ivanov, P. C. (2014). “Three independent forms of cardio-respiratory coupling: transitions across sleep stages,” in Computing in Cardiology 2014 (Long Beach, CA: IEEE), 781–784
Bartsch, R. P., Liu, K. K. L., Bashan, A., and Ivanov, P. C. (2015). Network physiology: how organ systems dynamically interact. PLoS ONE 10:e0142143. doi: 10.1371/journal.pone.0142143
Bartsch, R. P., Schumann, A. Y., Kantelhardt, J. W., Penzel, T., and Ivanov, P. C. (2012). Phase transitions in physiologic coupling. Proc. Natl. Acad. Sci. U.S.A. 109, 10181–10186. doi: 10.1073/pnas.1204568109
Bashan, A., Bartsch, R. P., Kantelhardt, J. W., Havlin, S., and Ivanov, P. C. (2012). Network physiology reveals relations between network topology and physiological function. Nat. Commun. 3:702. doi: 10.1038/ncomms1705
Bassett, D. S., and Bullmore, E. (2006). Small-world brain networks. Neuroscientist 12, 512–523. doi: 10.1177/1073858406293182
Bassett, D. S., and Sporns, O. (2017). Network neuroscience. Nat. Neurosci. 20, 353–364. doi: 10.1038/nn.4502
Batzel, J. J., and Kappel, F. (2011). Time delay in physiological systems: analyzing and modeling its impact. Math. Biosci. 234, 61–74. doi: 10.1016/j.mbs.2011.08.006
Beauchene, C., Roy, S., Moran, R., Leonessa, A., and Abaid, N. (2018). Comparing brain connectivity metrics: a didactic tutorial with a toy model and experimental data. J. Neural Eng. 15:056031. doi: 10.1088/1741-2552/aad96e
Bialonski, S., Horstmann, M., and Lehnertz, K. (2010). From brain to earth and climate systems: small-world interaction networks or not? Chaos 20:013134. doi: 10.1063/1.3360561
Bialonski, S., Wendler, M., and Lehnertz, K. (2011). Unraveling spurious properties of interaction networks with tailored random networks. PLoS ONE 6:e22826. doi: 10.1371/journal.pone.0022826
Bick, C., Goodfellow, M., Laing, C. R., and Martens, E. A. (2020). Understanding the dynamics of biological and neural oscillator networks through exact mean-field reductions: a review. J. Math. Neurosci. 10:9. doi: 10.1186/s13408-020-00086-9
Boccaletti, S., Bianconi, G., Criado, R., Del Genio, C. I., Gómez-Gardenes, J., Romance, M., et al. (2014). The structure and dynamics of multilayer networks. Phys. Rep. 544, 1–122. doi: 10.1016/j.physrep.2014.07.001
Boccaletti, S., Kurths, J., Osipov, G., Valladares, D. L., and Zhou, C. S. (2002). The synchronization of chaotic systems. Phys. Rep. 366, 1–101. doi: 10.1016/S0370-1573(02)00137-0
Boccaletti, S., Latora, V., Moreno, Y., Chavez, M., and Hwang, D.-U. (2006). Complex networks: structure and dynamics. Phys. Rep. 424, 175–308. doi: 10.1016/j.physrep.2005.10.009
Bruns, A. (2004). Fourier-, Hilbert- and wavelet-based signal analysis: are they really different approaches? J. Neurosci. Methods 137, 321–332. doi: 10.1016/j.jneumeth.2004.03.002
Butts, C. T. (2009). Revisiting the foundations of network analysis. Science 325, 414–416. doi: 10.1126/science.1171022
Camm, A. J., Malik, M., Bigger, J. T., Breithardt, G., Cerutti, S., Cohen, R. J., et al. (1996). Heart rate variability. Standards of measurement, physiological interpretation, and clinical use. Task Force of the European Society of Cardiology and the North American Society of Pacing Electrophysiology. Circulation 93, 1043–1065. doi: 10.1161/01.CIR.93.5.1043
Castellani, G. C., Menichetti, G., Garagnani, P., Giulia Bacalini, M., Pirazzini, C., Franceschi, C., et al. (2016). Systems medicine of inflammaging. Brief. Bioinform. 17, 527–540. doi: 10.1093/bib/bbv062
Čenys, A., Lasiene, G., and Pyragas, K. (1991). Estimation of interrelation between chaotic observables. Phys. D Nonlinear Phenomena 52, 332–337. doi: 10.1016/0167-2789(91)90130-2
Chen, Y., Rangarajan, G., Feng, J., and Ding, M. (2004). Analyzing multiple nonlinear time series with extended Granger causality. Phys. Lett. A 324, 26–35. doi: 10.1016/j.physleta.2004.02.032
Chicharro, D., and Andrzejak, R. G. (2009). Reliable detection of directional couplings using rank statistics. Phys. Rev. E 80:026217. doi: 10.1103/PhysRevE.80.026217
Colclough, G. L., Woolrich, M. W., Tewarie, P. K., Brookes, M. J., Quinn, A. J., and Smith, S. M. (2016). How reliable are MEG resting-state connectivity metrics? Neuroimage 138, 284–293. doi: 10.1016/j.neuroimage.2016.05.070
Coufal, D., Jakubík, J., Jajcay, N., Hlinka, J., Krakovská, A., and Paluš, M. (2017). Detection of coupling delay: a problem not yet solved. Chaos 27:083109. doi: 10.1063/1.4997757
Dhamala, M., Liang, H., Bressler, S. L., and Ding, M. (2018). Granger-Geweke causality: estimation and interpretation. Neuroimage 175, 460–463. doi: 10.1016/j.neuroimage.2018.04.043
Dickten, H., and Lehnertz, K. (2014). Identifying delayed directional couplings with symbolic transfer entropy. Phys. Rev. E 90:062706. doi: 10.1103/PhysRevE.90.062706
Dickten, H., Porz, S., Elger, C. E., and Lehnertz, K. (2016). Weighted and directed interactions in evolving large-scale epileptic brain networks. Sci. Rep. 6:34824. doi: 10.1038/srep34824
Eichler, M., Dahlhaus, R., and Sandkühler, J. (2003). Partial correlation analysis for the identification of synaptic connections. Biol. Cybern. 89, 289–302. doi: 10.1007/s00422-003-0400-3
Elbert, T., Ray, W. J., Kowalik, Z. J., Skinner, J. E., Graf, K. E., and Birbaumer, N. (1994). Chaos and physiology: deterministic chaos in excitable cell assemblies. Physiol. Rev. 74, 1–47.
Elsegai, H., Shiells, H., Thiel, M., and Schelter, B. (2015). Network inference in the presence of latent confounders: The role of instantaneous causalities. J. Neurosci. Methods 245, 91–106. doi: 10.1016/j.jneumeth.2015.02.015
Faes, L., Kugiumtzis, D., Nollo, G., Jurysta, F., and Marinazzo, D. (2015). Estimating the decomposition of predictive information in multivariate systems. Phys. Rev. E 91:032904. doi: 10.1103/PhysRevE.91.032904
Faes, L., Marinazzo, D., Montalto, A., and Nollo, G. (2014). Lag-specific transfer entropy as a tool to assess cardiovascular and cardiorespiratory information transfer. IEEE Trans. Biomed. Eng. 61, 2556–2568. doi: 10.1109/TBME.2014.2323131
Faes, L., Nollo, G., Stramaglia, S., and Marinazzo, D. (2017). Multiscale Granger causality. Phys. Rev. E 96:042150. doi: 10.1103/PhysRevE.96.042150
Faes, L., Porta, A., and Nollo, G. (2008). Mutual nonlinear prediction as a tool to evaluate coupling strength and directionality in bivariate time series: comparison among different strategies based on k nearest neighbors. Phys. Rev. E 78:026201. doi: 10.1103/PhysRevE.78.026201
Faes, L., Porta, A., and Nollo, G. (2010). Testing frequency-domain causality in multivariate time series. IEEE Trans. Biomed. Eng. 57, 1897–1906. doi: 10.1109/TBME.2010.2042715
Fagiolo, G. (2007). Clustering in complex directed networks. Phys. Rev. E 76:026107. doi: 10.1103/PhysRevE.76.026107
Florin, E., Gross, J., Pfeifer, J., Fink, G. R., and Timmermann, L. (2011). Reliability of multivariate causality measures for neural data. J. Neurosci. Methods 198, 344–358. doi: 10.1016/j.jneumeth.2011.04.005
Fornito, A., Zalesky, A., and Breakspear, M. (2013). Graph analysis of the human connectome: promise, progress, and pitfalls. Neuroimage 80, 426–444. doi: 10.1016/j.neuroimage.2013.04.087
Fortunato, S. (2010). Community detection in graphs. Phys. Rep. 486, 75–174. doi: 10.1016/j.physrep.2009.11.002
Frenzel, S., and Pompe, B. (2007). Partial mutual information for coupling analysis of multivariate time series. Phys. Rev. Lett. 99:204101. doi: 10.1103/PhysRevLett.99.204101
Friedrich, R., Peinke, J., Sahimi, M., and Tabar, M. R. R. (2011). Approaching complexity by stochastic methods: from biological systems to turbulence. Phys. Rep. 506, 87–162. doi: 10.1016/j.physrep.2011.05.003
García-Niebla, J., Llontop-García, P., Valle-Racero, J. I., Serra-Autonell, G., Batchvarov, V. N., and De Luna, A. B. (2009). Technical mistakes during the acquisition of the electrocardiogram. Ann. Noninvas. Electro. 14, 389–403. doi: 10.1111/j.1542-474X.2009.00328.x
Gastner, M. T., and Ódor, G. (2016). The topology of large open connectome networks for the human brain. Sci. Rep. 6:27249. doi: 10.1038/srep27249
Gordon, S. M., Franaszczuk, P. J., Hairston, W. D., Vindiola, M., and McDowell, K. (2013). Comparing parametric and nonparametric methods for detecting phase synchronization in EEG. J. Neurosci. Methods 212, 247–258. doi: 10.1016/j.jneumeth.2012.10.002
Gosak, M., Markovič, R., Dolenšek, J., Rupnik, M. S., Marhl, M., Stožer, A., et al. (2018). Network science of biological systems at different scales: a review. Phys. Life Rev. 24, 118–135. doi: 10.1016/j.plrev.2017.11.003
Grover, P., and Venkatesh, P. (2016). An information-theoretic view of EEG sensing. Proc. IEEE 105, 367–384. doi: 10.1109/JPROC.2016.2615179
Halu, A., De Domenico, M., Arenas, A., and Sharma, A. (2019). The multiplex network of human diseases. NPJ Syst. Biol. Appl. 5, 1–12. doi: 10.1038/s41540-019-0092-5
Hardmeier, M., Hatz, F., Bousleiman, H., Schindler, C., Stam, C. J., and Fuhr, P. (2014). Reproducibility of functional connectivity and graph measures based on the Phase Lag Index (PLI) and Weighted Phase Lag Index (wPLI) derived from high resolution EEG. PLoS ONE 9:e108648. doi: 10.1371/journal.pone.0108648
Harford, M., Catherall, J., Gerry, S., Young, J., and Watkinson, P. (2019). Availability and performance of image-based, non-contact methods of monitoring heart rate, blood pressure, respiratory rate, and oxygen saturation: a systematic review. Physiol. Meas. 40:06TR01. doi: 10.1088/1361-6579/ab1f1d
Hempel, S., Koseska, A., Kurths, J., and Nikoloski, Z. (2011). Inner composition alignment for inferring directed networks from short time series. Phys. Rev. Lett. 107:054101. doi: 10.1103/PhysRevLett.107.054101
Hesse, W., Möller, E., Arnold, M., and Schack, B. (2003). The use of time-variant {EEG} granger causality for inspecting directed interdependencies of neural assemblies. J. Neurosci. Methods 124, 27–44. doi: 10.1016/S0165-0270(02)00366-7
Hilgetag, C. C., and Goulas, A. (2016). Is the brain really a small-world network? Brain Struct. Funct. 221, 2361–2366. doi: 10.1007/s00429-015-1035-6
Hirata, Y., Amigó, J. M., Matsuzaka, Y., Yokota, R., Mushiake, H., and Aihara, K. (2016). Detecting causality by combined use of multiple methods: Climate and brain examples. PLoS ONE 11:e0158572. doi: 10.1371/journal.pone.0158572
Hlaváčková-Schindler, K., Paluš, M., Vejmelka, M., and Bhattacharya, J. (2007). Causality detection based on information-theoretic approaches in time series analysis. Phys. Rep. 441, 1–46. doi: 10.1016/j.physrep.2006.12.004
Hlinka, J., Hartman, D., Jajcay, N., Tomeček, D., Tintěra, J., and Paluš, M. (2017). Small-world bias of correlation networks: from brain to climate. Chaos 27:035812. doi: 10.1063/1.4977951
Hlinka, J., Hartman, D., and Paluš, M. (2012). Small-world topology of functional connectivity in randomly connected dynamical systems. Chaos 22:033107. doi: 10.1063/1.4732541
Holme, P., and Saramäki, J. (2012). Temporal networks. Phys. Rep. 519, 97–125. doi: 10.1016/j.physrep.2012.03.001
Humeau-Heurtier, A. (2016). Multivariate generalized multiscale entropy analysis. Entropy 18:411. doi: 10.3390/e18110411
Ioannides, A. A. (2007). Dynamic functional connectivity. Curr. Opin. Neurobiol. 17, 161–170. doi: 10.1016/j.conb.2007.03.008
Ivanov, P. C., Liu, K. K., and Bartsch, R. P. (2016). Focus on the emerging new fields of network physiology and network medicine. New J. Phys. 18:100201. doi: 10.1088/1367-2630/18/10/100201
Jafri, H. H., Singh, R. K. B., and Ramaswamy, R. (2016). Generalized synchrony of coupled stochastic processes with multiplicative noise. Phys. Rev. E 94:052216. doi: 10.1103/PhysRevE.94.052216
Jalili, M., and Knyazeva, M. G. (2011). Constructing brain functional networks from EEG: partial and unpartial correlations. J. Integr. Neurosci. 10, 213–232. doi: 10.1142/S0219635211002725
Jamin, A., and Humeau-Heurtier, A. (2020). (multiscale) cross-entropy methods: a review. Entropy 22:45. doi: 10.3390/e22010045
Jatoi, M. A., Kamel, N., Malik, A. S., Faye, I., and Begum, T. (2014). A survey of methods used for source localization using EEG signals. Biomed. Sig. Proc. Contr. 11, 42–52. doi: 10.1016/j.bspc.2014.01.009
Jelfs, B., and Chan, R. H. M. (2017). Directionality indices: testing information transfer with surrogate correction. Phys. Rev. E 96:052220. doi: 10.1103/PhysRevE.96.052220
Kantz, H., and Schreiber, T. (2003). Nonlinear Time Series Analysis, 2nd Edn. Cambridge, UK: Cambridge University Press. doi: 10.1017/CBO9780511755798
Kivelä, M., Arenas, A., Barthelemy, M., Gleeson, J. P., Moreno, Y., and Porter, M. A. (2014). Multilayer networks. J. Complex Netw. 2, 203–271. doi: 10.1093/comnet/cnu016
Kligfield, P., Gettes, L. S., Bailey, J. J., Childers, R., Deal, B. J., Hancock, E. W., et al. (2007). Recommendations for the standardization and interpretation of the electrocardiogram: Part I: the electrocardiogram and its technology a scientific statement from the American Heart Association Electrocardiography and Arrhythmias Committee, Council on Clinical Cardiology; the American College of Cardiology Foundation; and the Heart Rhythm Society endorsed by the International Society for computerized electrocardiology. J. Am. Coll. Cardiol. 49, 1109–1127. doi: 10.1016/j.jacc.2007.01.024
Klimesch, W. (2018). The frequency architecture of brain and brain body oscillations: an analysis. Eur. J. Neurosci. 48, 2431–2453. doi: 10.1111/ejn.14192
Kostoglou, K., Schondorf, R., and Mitsis, G. D. (2019). Modeling of multiple-input, time-varying systems with recursively estimated basis expansions. Signal Proc. 155, 287–300. doi: 10.1016/j.sigpro.2018.09.040
Krakovská, A., Jakubík, J., Chvosteková, M., Coufal, D., Jajcay, N., and Paluš, M. (2018). Comparison of six methods for the detection of causality in a bivariate time series. Phys. Rev. E 97:042207. doi: 10.1103/PhysRevE.97.042207
Kralemann, B., Cimponeriu, L., Rosenblum, M. G., Pikovsky, A. S., and Mrowka, R. (2008). Phase dynamics of coupled oscillators reconstructed from data. Phys. Rev. E 77:066205. doi: 10.1103/PhysRevE.77.066205
Kralemann, B., Pikovsky, A., and Rosenblum, M. (2014). Reconstructing effective phase connectivity of oscillator networks from observations. New J. Phys. 16:085013. doi: 10.1088/1367-2630/16/8/085013
Kramer, M. A., Eden, U. T., Cash, S. S., and Kolaczyk, E. D. (2009). Network inference with confidence from multivariate time series. Phys. Rev. E 79:061916. doi: 10.1103/PhysRevE.79.061916
Kreuz, T., Mormann, F., Andrzejak, R. G., Kraskov, A., Lehnertz, K., and Grassberger, P. (2007). Measuring synchronization in coupled model systems: a comparison of different approaches. Phys. D 225, 29–42. doi: 10.1016/j.physd.2006.09.039
Kugiumtzis, D. (2013). Partial transfer entropy on rank vectors. Eur. Phys. J. Spec. Top. 222, 401–420. doi: 10.1140/epjst/e2013-01849-4
Lachaux, J. P., Rodriguez, E., Martinerie, J., and Varela, F. J. (1999). Measuring phase synchrony in brain signals. Hum. Brain Mapp. 8, 194–208. doi: 10.1002/(SICI)1097-0193(1999)8:4<194::AID-HBM4>3.0.CO;2-C
Lahat, D., Adali, T., and Jutten, C. (2015). Multimodal data fusion: an overview of methods, challenges, and prospects. Proc. IEEE 103, 1449–1477. doi: 10.1109/JPROC.2015.2460697
Laland, K. N., Sterelny, K., Odling-Smee, J., Hoppitt, W., and Uller, T. (2011). Cause and effect in biology revisited: is Mayr's proximate-ultimate dichotomy still useful? Science 334, 1512–1516. doi: 10.1126/science.1210879
Lancaster, G., Iatsenko, D., Pidde, A., Ticcinelli, V., and Stefanovska, A. (2018). Surrogate data for hypothesis testing of physical systems. Phys. Rep. 748, 1–60. doi: 10.1016/j.physrep.2018.06.001
Langford, E., Schwertman, N., and Owens, M. (2001). Is the property of being positively correlated transitive? Am. Stat. 55, 322–325. doi: 10.1198/000313001753272286
Lehnertz, K. (2011). Assessing directed interactions from neurophysiological signals – an overview. Physiol. Meas. 32, 1715–1724. doi: 10.1088/0967-3334/32/11/R01
Lehnertz, K., Ansmann, G., Bialonski, S., Dickten, H., Geier, C., and Porz, S. (2014). Evolving networks in the human epileptic brain. Phys. D 267, 7–15. doi: 10.1016/j.physd.2013.06.009
Lehnertz, K., and Dickten, H. (2015). Assessing directionality and strength of coupling through symbolic analysis: an application to epilepsy patients. Philos. Trans. R. Soc. A 373:20140094. doi: 10.1098/rsta.2014.0094
Leistritz, L., Pester, B., Doering, A., Schiecke, K., Babiloni, F., Astolfi, L., et al. (2013). Time-variant partial directed coherence for analysing connectivity: a methodological study. Philos. Trans. R. Soc. A 371:20110616. doi: 10.1098/rsta.2011.0616
Leng, S., Ma, H., Kurths, J., Lai, Y.-C., Lin, W., Aihara, K., et al. (2020). Partial cross mapping eliminates indirect causal influences. Nat. Commun. 11:2632. doi: 10.1038/s41467-020-16238-0
Li, S., Xiao, Y., Zhou, D., and Cai, D. (2018). Causal inference in nonlinear systems: Granger causality versus time-delayed mutual information. Phys. Rev. E 97:052216. doi: 10.1103/PhysRevE.97.052216
Lin, A., Liu, K. K., Bartsch, R. P., and Ivanov, P. C. (2016). Delay-correlation landscape reveals characteristic time delays of brain rhythms and heart interactions. Philos. Transa. R. Soc. A Math. Phys. Eng. Sci. 374:20150182. doi: 10.1098/rsta.2015.0182
Liu, K. K., Bartsch, R. P., Lin, A., Mantegna, R. N., and Ivanov, P. C. (2015). Plasticity of brain wave network interactions and evolution across physiologic states. Front. Neural Circ. 9:62. doi: 10.3389/fncir.2015.00062
Liu, Z. (2004). Measuring the degree of synchronization from time series data. Europhys. Lett. 68, 19–25. doi: 10.1209/epl/i2004-10173-x
Lungarella, M., Pitti, A., and Kuniyoshi, Y. (2007). Information transfer at multiple scales. Phys. Rev. E 76:056117. doi: 10.1103/PhysRevE.76.056117
Lütkepohl, H. (2005). New Introduction to Multiple Time Series Analysis. Berlin; Heidelberg: Springer Science & Business Media.
Ma, H., Aihara, K., and Chen, L. (2014). Detecting causality from nonlinear dynamics with short-term time series. Sci. Rep. 4:7464. doi: 10.1038/srep07464
Ma, H., Leng, S., Tao, C., Ying, X., Kurths, J., Lai, Y.-C., et al. (2017). Detection of time delays and directional interactions based on time series from complex dynamical systems. Phys. Rev. E 96:012221. doi: 10.1103/PhysRevE.96.012221
Mader, W., Mader, M., Timmer, J., Thiel, M., and Schelter, B. (2015). Networks: On the relation of bi-and multivariate measures. Sci. Rep. 5:10805. doi: 10.1038/srep10805
Marinazzo, D., Pellicoro, M., and Stramaglia, S. (2012). Causal information approach to partial conditioning in multivariate data sets. Comput. Math. Method Med. 303601, 1–8. doi: 10.1155/2012/303601
Marmarelis, V. (2012). Analysis of Physiological Systems: The White-Noise Approach. New York, NY: Springer Science & Business Media.
Martini, M., Kranz, T. A., Wagner, T., and Lehnertz, K. (2011). Inferring directional interactions from transient signals with symbolic transfer entropy. Phys. Rev. E 83:011919. doi: 10.1103/PhysRevE.83.011919
Marwan, N., Romano, M. C., Thiel, M., and Kurths, J. (2007). Recurrence plots for the analysis of complex systems. Phys. Rep. 438, 237–329. doi: 10.1016/j.physrep.2006.11.001
Mheich, A., Wendling, F., and Hassan, M. (2020). Brain network similarity: methods and applications. Network Neurosci. 4, 507–527. doi: 10.1162/netn_a_00133
Monetti, R., Bunk, W., Aschenbrenner, T., and Jamitzky, F. (2009). Characterizing synchronization in time series using information measures extracted from symbolic representations. Phys. Rev. E 79:046207. doi: 10.1103/PhysRevE.79.046207
Mormann, F., Lehnertz, K., David, P., and Elger, C. E. (2000). Mean phase coherence as a measure for phase synchronization and its application to the EEG of epilepsy patients. Phys. D 144, 358–369. doi: 10.1016/S0167-2789(00)00087-7
Müller, A., Kraemer, J. F., Penzel, T., Bonnemeier, H., Kurths, J., and Wessel, N. (2016). Causality in physiological signals. Physiol. Meas. 37:R46. doi: 10.1088/0967-3334/37/5/R46
Müller, T., Lauk, M., Reinhard, M., Hetzel, A., Lücking, C. H., and Timmer, J. (2003). Estimation of delay times in biological systems. Ann. Biomed. Eng. 31, 1423–1439. doi: 10.1114/1.1617984
Nawrath, J., Romano, M. C., Thiel, M., Kiss, I. Z., Wickramasinghe, M., Timmer, J., et al. (2010). Distinguishing direct from indirect interactions in oscillatory networks with multiple time scales. Phys. Rev. Lett. 104:038701. doi: 10.1103/PhysRevLett.104.038701
Newman, M. E. J. (2012). Communities, modules and large-scale structure in networks. Nat. Phys. 8, 25–31. doi: 10.1038/NPHYS2162
Nitzan, M., Casadiego, J., and Timme, M. (2017). Revealing physical interaction networks from statistics of collective dynamics. Sci. Adv. 3:e1600396. doi: 10.1126/sciadv.1600396
Nolte, G., Bai, O., Wheaton, L., Mari, Z., Vorbach, S., and Hallett, M. (2004). Identifying true brain interaction from EEG data using the imaginary part of coherency. Clin. Neurophysiol. 115, 2292–2307. doi: 10.1016/j.clinph.2004.04.029
Osterhage, H., Mormann, F., Staniek, M., and Lehnertz, K. (2007a). Measuring synchronization in the epileptic brain: A comparison of different approaches. Int. J. Bifurcation Chaos Appl. Sci. Eng. 17, 3539–3544.
Osterhage, H., Mormann, F., Wagner, T., and Lehnertz, K. (2007b). Measuring the directionality of coupling: phase versus state space dynamics and application to EEG time series. Int. J. Neural Syst. 17, 139–148. doi: 10.1142/S0129065707001019
Osterhage, H., Mormann, F., Wagner, T., and Lehnertz, K. (2008). Detecting directional coupling in the human epileptic brain: Limitations and potential pitfalls. Phys. Rev. E 77:011914. doi: 10.1103/PhysRevE.77.011914
Paluš, M. (2019). Coupling in complex systems as information transfer across time scales. Philos. Trans. R. Soc. A 377:20190094. doi: 10.1098/rsta.2019.0094
Paluš, M., and Vejmelka, M. (2007). Directionality of coupling from bivariate time series: How to avoid false causalities and missed connections. Phys. Rev. E 75:056211. doi: 10.1103/PhysRevE.75.056211
Papo, D., Zanin, M., Martínez, J. H., and Buldú, J. M. (2016). Beware of the small-world neuroscientist! Front. Hum. Neurosci. 10:96. doi: 10.3389/fnhum.2016.00096
Peraza, L. R., Asghar, A. U. R., Green, G., and Halliday, D. M. (2012). Volume conduction effects in brain network inference from electroencephalographic recordings using phase lag index. J. Neurosci. Methods 207, 189–199. doi: 10.1016/j.jneumeth.2012.04.007
Pereda, E., Quian Quiroga, R., and Bhattacharya, J. (2005). Nonlinear multivariate analysis of neurophysiological signals. Prog. Neurobiol. 77, 1–37. doi: 10.1016/j.pneurobio.2005.10.003
Pietras, B., and Daffertshofer, A. (2019). Network dynamics of coupled oscillators and phase reduction techniques. Phys. Rep. 819, 1–105. doi: 10.1016/j.physrep.2019.06.001
Pikovsky, A. S., Rosenblum, M. G., and Kurths, J. (2001). Synchronization: A Universal Concept in Nonlinear Sciences. Cambridge, UK: Cambridge University Press. doi: 10.1017/CBO9780511755743
Porta, A., and Faes, L. (2015). Wiener–Granger causality in network physiology with applications to cardiovascular control and neuroscience. Proc. IEEE 104, 282–309. doi: 10.1109/JPROC.2015.2476824
Porz, S., Kiel, M., and Lehnertz, K. (2014). Can spurious indications for phase synchronization due to superimposed signals be avoided? Chaos 24:033112. doi: 10.1063/1.4890568
Prusseit, J., and Lehnertz, K. (2008). Measuring interdependences in dissipative dynamical systems with estimated Fokker-Planck coefficients. Phys. Rev. E 77:041914. doi: 10.1103/PhysRevE.77.041914
Ramb, R., Eichler, M., Ing, A., Thiel, M., Weiller, C., Grebogi, C., et al. (2013). The impact of latent confounders in directed network analysis in neuroscience. Philos. Trans. R. Soc. A 371:20110612. doi: 10.1098/rsta.2011.0612
Reinsel, G. C. (2003). Elements of Multivariate Time Series Analysis, 2nd Edn. New York, NY: Springer.
Ricci, L., Castelluzzo, M., Minati, L., and Perinelli, A. (2019). Generation of surrogate event sequences via joint distribution of successive inter-event intervals. Chaos 29:121102. doi: 10.1063/1.5138250
Rings, T., and Lehnertz, K. (2016). Distinguishing between direct and indirect directional couplings in large oscillator networks: partial or non-partial phase analyses? Chaos 26:093106. doi: 10.1063/1.4962295
Romano, M. C., Thiel, M., Kurths, J., Mergenthaler, K., and Engbert, R. (2009). Hypothesis test for synchronization: twin surrogates revisited. Chaos 19:015108. doi: 10.1063/1.3072784
Rosenblum, M., and Pikovsky, A. (2019). Nonlinear phase coupling functions: a numerical study. Philos. Trans. R. Soc. A 377:20190093. doi: 10.1098/rsta.2019.0093
Rosenblum, M. G., and Pikovsky, A. S. (2001). Detecting direction of coupling in interacting oscillators. Phys. Rev. E 64:045202. doi: 10.1103/PhysRevE.64.045202
Rosenblum, M. G., Pikovsky, A. S., and Kurths, J. (1996). Phase synchronization of chaotic oscillators. Phys. Rev. Lett. 76, 1804–1807. doi: 10.1103/PhysRevLett.76.1804
Rosinberg, M. L., Tarjus, G., and Munakata, T. (2018). Influence of time delay on information exchanges between coupled linear stochastic systems. Phys. Rev. E 98:032130. doi: 10.1103/PhysRevE.98.032130
Rubido, N., Marti, A. C., Bianco-Martinez, E., Grebogi, C., Baptista, M. S., and Masoller, C. (2014). Exact detection of direct links in networks of interacting dynamical units. New J. Phys. 16:093010. doi: 10.1088/1367-2630/16/9/093010
Rubinov, M., and Sporns, O. (2010). Complex network measures of brain connectivity: uses and interpretations. Neuroimage 52, 1059–1069. doi: 10.1016/j.neuroimage.2009.10.003
Rulkov, N. F., Sushchik, M. M., Tsimring, L. S., and Abarbanel, H. D. I. (1995). Generalized synchronization of chaos in directionally coupled chaotic systems. Phys. Rev. E 51, 980–994. doi: 10.1103/PhysRevE.51.980
Runge, J. (2018). Causal network reconstruction from time series: from theoretical assumptions to practical estimation. Chaos 28:075310. doi: 10.1063/1.5025050
Runge, J., Heitzig, J., Petoukhov, V., and Kurths, J. (2012). Escaping the curse of dimensionality in estimating multivariate transfer entropy. Phys. Rev. Lett. 108:258701. doi: 10.1103/PhysRevLett.108.258701
Rydin Gorjão, L., Heysel, J., Lehnertz, K., and Tabar, M. R. R. (2019). Analysis and data-driven reconstruction of bivariate jump-diffusion processes. Phys. Rev. E 100:062127. doi: 10.1103/PhysRevE.100.062127
Saramäki, J., Kivelä, M., Onnela, J. P., Kaski, K., and Kertész, J. (2007). Generalizations of the clustering coefficient to weighted complex networks. Phys. Rev. E 75:027105. doi: 10.1103/PhysRevE.75.027105
Schelter, B., Winterhalder, M., Dahlhaus, R., Kurths, J., and Timmer, J. (2006a). Partial phase synchronization for multivariate synchronizing systems. Phys. Rev. Lett. 96:208103. doi: 10.1103/PhysRevLett.96.208103
Schelter, B., Winterhalder, M., Eichler, M., Peifer, M., Hellwig, B., Guschlbauer, B., et al. (2006b). Testing for directed influences among neural signals using partial directed coherence. J. Neurosci. Methods 152, 210–219. doi: 10.1016/j.jneumeth.2005.09.001
Schreiber, T. (2000). Measuring information transfer. Phys. Rev. Lett. 85, 461–464. doi: 10.1103/PhysRevLett.85.461
Schreiber, T., and Schmitz, A. (2000). Surrogate time series. Phys. D 142, 346–382. doi: 10.1016/S0167-2789(00)00043-9
Schwabedal, J. T. C., and Kantz, H. (2016). Optimal extraction of collective oscillations from unreliable measurements. Phys. Rev. Lett. 116:104101. doi: 10.1103/PhysRevLett.116.104101
Seeck, M., Koessler, L., Bast, T., Leijten, F., Michel, C., Baumgartner, C., et al. (2017). The standardized EEG electrode array of the IFCN. Clin. Neurophysiol. 128, 2070–2077. doi: 10.1016/j.clinph.2017.06.254
Seth, A. K., Barrett, A. B., and Barnett, L. (2015). Granger causality analysis in neuroscience and neuroimaging. J. Neurosci. 35, 3293–3297.
Silchenko, A. N., Adamchic, I., Pawelczyk, N., Hauptmann, C., Maarouf, M., Sturm, V., et al. (2010). Data-driven approach to the estimation of connectivity and time delays in the coupling of interacting neuronal subsystems. J. Neurosci. Methods 191, 32–44. doi: 10.1016/j.jneumeth.2010.06.004
Smirnov, D., Schelter, B., Winterhalder, M., and Timmer, J. (2007). Revealing direction of coupling between neuronal oscillators from time series: Phase dynamics modeling versus partial directed coherence. Chaos 17:013111. doi: 10.1063/1.2430639
Smirnov, D. A. (2014). Quantification of causal couplings via dynamical effects: A unifying perspective. Phys. Rev. E 90:062921. doi: 10.1103/PhysRevE.90.062921
Smirnov, D. A., and Andrzejak, R. G. (2005). Detection of weak directional coupling: phase dynamics approach versus state-space approach. Phys. Rev. E 61:036207. doi: 10.1103/PhysRevE.71.036207
Smirnov, D. A., and Bezruchko, B. P. (2009). Detection of couplings in ensembles of stochastic oscillators. Phys. Rev. E 79:046204. doi: 10.1103/PhysRevE.79.046204
Stahn, K., and Lehnertz, K. (2017). Surrogate-assisted identification of influences of network construction on evolving weighted functional networks. Chaos 27:123106. doi: 10.1063/1.4996980
Stam, C. J., Nolte, G., and Daffertshofer, A. (2007). Phase lag index: assessment of functional connectivity from multi channel EEG and MEG with diminished bias from common sources. Hum. Brain Mapp. 28, 1178–1193. doi: 10.1002/hbm.20346
Stam, C. J., and van Straaten, E. C. W. (2012). Go with the flow: Use of a directed phase lag index (dPLI) to characterize patterns of phase relations in a large-scale model of brain dynamics. Neuroimage 62, 1415–1428. doi: 10.1016/j.neuroimage.2012.05.050
Staniek, M., and Lehnertz, K. (2008). Symbolic transfer entropy. Phys. Rev. Lett. 100:158101. doi: 10.1103/PhysRevLett.100.158101
Stankovski, T., Pereira, T., McClintock, P. V. E., and Stefanovska, A. (2017). Coupling functions: universal insights into dynamical interaction mechanisms. Rev. Mod. Phys. 89:045001. doi: 10.1103/RevModPhys.89.045001
Stokes, P. A., and Purdon, P. L. (2017). A study of problems encountered in Granger causality analysis from a neuroscience perspective. Proc. Natl Acad. Sci. U.S.A. 114, E7063–E7072. doi: 10.1073/pnas.1704663114
Stramaglia, S., Wu, G.-R., Pellicoro, M., and Marinazzo, D. (2012). Expanding the transfer entropy to identify information circuits in complex systems. Phys. Rev. E 86:066211. doi: 10.1103/PhysRevE.86.066211
Tabar, M. R. R. (2019). Analysis and Data-Based Reconstruction of Complex Nonlinear Dynamical Systems: Using the Methods of Stochastic Processes. Cham: Springer. doi: 10.1007/978-3-030-18472-8
Tankisi, H., Burke, D., Cui, L., de Carvalho, M., Kuwabara, S., Nandedkar, S. D., et al. (2020). Standards of instrumentation of EMG. Clin. Neurophysiol. 131, 243–258. doi: 10.1016/j.clinph.2019.07.025
Tass, P. A., Rosenblum, M. G., Weule, J., Kurths, J., Pikovsky, A., Volkmann, J., et al. (1998). Detection of n:m phase locking from noisy data: application to magnetoencephalography. Phys. Rev. Lett. 81, 3291–3294. doi: 10.1103/PhysRevLett.81.3291
Thiel, M., Romano, M. C., Kurths, J., Rolfs, M., and Kliegl, R. (2006). Twin surrogates to test for complex synchronisation. Europhys. Lett. 75, 535–541. doi: 10.1209/epl/i2006-10147-0
Timme, M., and Casadiego, J. (2014). Revealing networks from dynamics: an introduction. J. Phys. A 47:343001. doi: 10.1088/1751-8113/47/34/343001
Timme, N., Alford, W., Flecker, B., and Beggs, J. M. (2014). Synergy, redundancy, and multivariate information measures: an experimentalis's perspective. J. Comput. Neurosci. 36, 119–140. doi: 10.1007/s10827-013-0458-4
Trägårdh, E., Engblom, H., and Pahlm, O. (2006). How many ECG leads do we need? Cardiol. Clin. 24, 317–330. doi: 10.1016/j.ccl.2006.04.005
Vakorin, V. A., Krakovska, O. A., and McIntosh, A. R. (2009). Confounding effects of indirect connections on causality estimation. J. Neurosci. Methods 184, 152–160. doi: 10.1016/j.jneumeth.2009.07.014
Van Mierlo, P., Höller, Y., Focke, N. K., and Vulliemoz, S. (2019). Network perspectives on epilepsy using EEG/MEG source connectivity. Front. Neurol. 10:721. doi: 10.3389/fneur.2019.00721
Vejmelka, M., and Paluš, M. (2008). Inferring the directionality of coupling with conditional mutual information. Phys. Rev. E 77:026214. doi: 10.1103/PhysRevE.77.026214
Vicente, R., Wibral, M., Lindner, M., and Pipa, G. (2011). Transfer entropy–a model-free measure of effective connectivity for the neurosciences. J. Comput. Neurosci. 30, 45–67. doi: 10.1007/s10827-010-0262-3
Vinck, M., Oostenveld, R., van Wingerden, M., Battaglia, F., and Pennartz, C. M. A. (2011). An improved index of phase-synchronization for electrophysiological data in the presence of volume-conduction, noise and sample-size bias. Neuroimage 55, 1548–1565. doi: 10.1016/j.neuroimage.2011.01.055
von Helmholtz, H. (1853). Ueber einige Gesetze der Vertheilung elektrischer Ströme in körperlichen Leitern, mit Anwendung auf die thierisch-elektrischen Versuche (Schluss.). Ann. Phys. 165, 353–377.
Wagner, T., Fell, J., and Lehnertz, K. (2010). The detection of transient directional couplings based on phase synchronization. New J. Phys. 12:053031. doi: 10.1088/1367-2630/12/5/053031
Wang, H. E., Bénar, C. G., Quilichini, P. P., Friston, K. J., Jirsa, V., and Bernard, C. (2014). A systematic framework for functional connectivity measures. Front. Neurosci. 8:405. doi: 10.3389/fnins.2014.00405
Wendling, F., Ansari-Asl, K., Bartolomei, F., and Senhadji, L. (2009). From EEG signals to brain connectivity: a model-based evaluation of interdependence measures. J. Neurosci. Methods 183, 9–18. doi: 10.1016/j.jneumeth.2009.04.021
Wens, V. (2015). Investigating complex networks with inverse models: analytical aspects of spatial leakage and connectivity estimation. Phys. Rev. E 91:012823. doi: 10.1103/PhysRevE.91.012823
Xia, H., Garcia, G. A., and Zhao, X. (2012). Automatic detection of ECG electrode misplacement: a tale of two algorithms. Physiol. Meas. 33:1549. doi: 10.1088/0967-3334/33/9/1549
Xiong, W., Faes, L., and Ivanov, P. C. (2017). Entropy measures, entropy estimators, and their performance in quantifying complex dynamics: effects of artifacts, nonstationarity, and long-range correlations. Phys. Rev. E 95:062114. doi: 10.1103/PhysRevE.95.062114
Xu, L., Chen, Z., Hu, K., Stanley, H. E., and Ivanov, P. C. (2006). Spurious detection of phase synchronization in coupled nonlinear oscillators. Phys. Rev. E 73:065201. doi: 10.1103/PhysRevE.73.065201
Ye, H., Deyle, E. R., Gilarranz, L. J., and Sugihara, G. (2015). Distinguishing time-delayed causal interactions using convergent cross mapping. Sci. Rep. 5:14750. doi: 10.1038/srep14750
Yu, D., and Boccaletti, S. (2009). Real-time estimation of interaction delays. Phys. Rev. E 80:036203. doi: 10.1103/PhysRevE.80.036203
Zanin, M., Belkoura, S., Gomez, J., Alfaro, C., and Cano, J. (2018). Topological structures are consistently overestimated in functional complex networks. Sci. Rep. 8:11980. doi: 10.1038/s41598-018-30472-z
Zanin, M., Sousa, P., Papo, D., Bajo, R., Garcia-Prieto, J., del Pozo, F., et al. (2012). Optimizing functional network representation of multivariate time series. Sci. Rep. 2:630. doi: 10.1038/srep00630
Zerenner, T., Friederichs, P., Lehnertz, K., and Hense, A. (2014). A Gaussian graphical model approach to climate networks. Chaos 24:023103. doi: 10.1063/1.4870402
Zhao, J., Zhou, Y., Zhang, X., and Chen, L. (2016). Part mutual information for quantifying direct associations in networks. Proc. Natl. Acad. Sci. U.S.A. 113, 5130–5135. doi: 10.1073/pnas.1522586113
Zhou, D., Zhang, Y., Xiao, Y., and Cai, D. (2014). Reliability of the Granger causality inference. New J. Phys. 16:043016. doi: 10.1088/1367-2630/16/4/043016
Keywords: complex networks, time-series-analysis techniques, surrogate concepts, inverse problem, physiological systems, organ communications, network physiology
Citation: Lehnertz K, Bröhl T and Rings T (2020) The Human Organism as an Integrated Interaction Network: Recent Conceptual and Methodological Challenges. Front. Physiol. 11:598694. doi: 10.3389/fphys.2020.598694
Received: 25 August 2020; Accepted: 30 November 2020;
Published: 21 December 2020.
Edited by:
Plamen Ch. Ivanov, Boston University, United StatesReviewed by:
Sebastiano Stramaglia, University of Bari Aldo Moro, ItalyLuca Faes, University of Palermo, Italy
Ronny P. Bartsch, Bar-Ilan University, Israel
Copyright © 2020 Lehnertz, Bröhl and Rings. This is an open-access article distributed under the terms of the Creative Commons Attribution License (CC BY). The use, distribution or reproduction in other forums is permitted, provided the original author(s) and the copyright owner(s) are credited and that the original publication in this journal is cited, in accordance with accepted academic practice. No use, distribution or reproduction is permitted which does not comply with these terms.
*Correspondence: Klaus Lehnertz, a2xhdXMubGVobmVydHpAdWtib25uLmRl