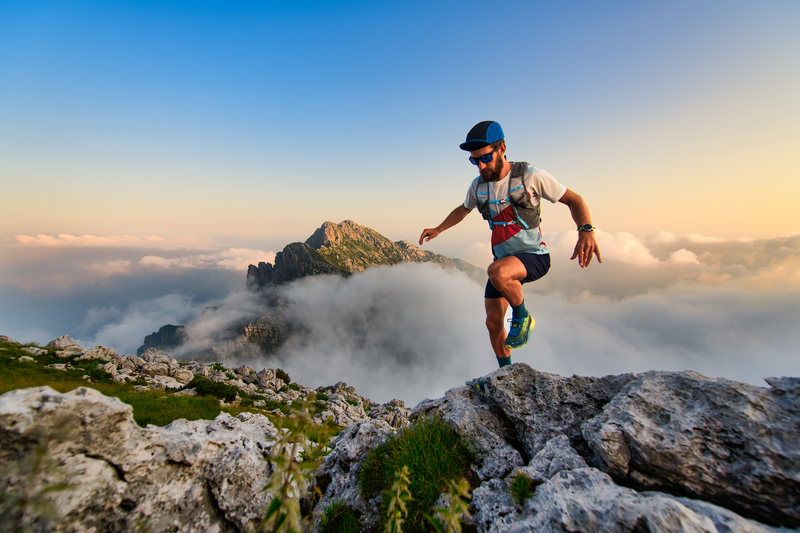
95% of researchers rate our articles as excellent or good
Learn more about the work of our research integrity team to safeguard the quality of each article we publish.
Find out more
ORIGINAL RESEARCH article
Front. Physiol. , 05 February 2021
Sec. Exercise Physiology
Volume 11 - 2020 | https://doi.org/10.3389/fphys.2020.597697
The purposes of this study were (i) to analyze the variations in maximal oxygen consumption (VO2max), maximal heart rate (HRmax), heart rate at rest, acceleration, maximal speed, agility, anaerobic sprint test (RAST) of peak power (RPP), RAST of minimum power, RAST of average power (RAP), and RAST of fatigue index (RFI) during the competitive season, using maturation status and accumulated training load as covariates, and (ii) to describe the differences between responders and non-responders in relation to baseline levels. Twenty-three elite players from the same team competing in the national under-16 competitions were evaluated for 20 weeks in period 1 (before league), middle (mid league), and period 2 (after league). The VO2max (p = 0.009), maximal speed (p = 0.001), RPP (p < 0.001), RAP (p < 0.001), and RFI (p < 0.001) significantly changed across the assessment periods. Interestingly, using accumulated training load and maturation status as covariates revealed no statistical significance (p > 0.05). When analyzing responders and non-responders, only HRmax (between periods 1 and 2) showed no differences between the groups. As a conclusion, it can be seen that accumulated training load and maturation status play an important role in the differences observed across the season. Thus, coaches should consider the importance of these two factors to carefully interpret fitness changes in their players and possibly adjust training decisions according to the maturation level of the players.
Successful soccer performance depends on multiple, complex, and interdependent factors, including anthropometric traits, maximal speed, change-of-direction ability (COD), and aerobic and anaerobic capacities (Stolen et al., 2005; Hulse et al., 2012). In fact, soccer is an intermittent high-intensity sport, which includes various types of running with rapid changes of directions, starts, stops, jumps, and kicks (Alfredson et al., 1996). Therefore, physical performance is commonly assessed and monitored as the outcome of standardized motor tasks requiring maximal speed, COD ability, balance, flexibility, explosive strength (Malina et al., 2004), local muscular endurance, and static muscular strength, particularly for the purpose of long-term athletic training (Carling, 2013).
The energy demand in soccer players is mainly dependent on aerobic capacity (Stolen et al., 2005). Adults soccer players usually cover between ∼10 and 12 km, while in young soccer players the distances covered during competitions is lower: 6.5, 7.4, and 8.1 km (for under −13, −14, and −15, respectively) (Buchheit et al., 2010). Moreover, higher levels of aerobic capacity enhance recovery from high-intensity interval loads (Svensson and Drust, 2005). This capacity also seems to be a prerequisite to improve the efficiency of anaerobic capacity when performing high-intensity intermittent efforts (Tomlin and Wenger, 2001). Also, when performing repetitive sprints tests, the best players showed more tolerance to fatigue (Reilly et al., 2000b). Finally, change-of-direction ability is also able to discriminate recreational and non-soccer players matched for intermittent endurance capacity (Coratella et al., 2016).
Maximal speed and COD ability tests are commonly used in talent identification programs (Rommers et al., 2019), as during the match activities involving accelerations (ACC), maximal speed, and COD ability number in the range of 150–250 n (Bangsbo et al., 2007). Although the physiological measures (e.g., absolute maximal oxygen uptake—VO2max) were generally more discriminative than anthropometry (Reilly et al., 2000a), positive correlations between body mass, fat-free mass, and skeletal muscle mass have been observed (Boraczyński et al., 2015). Indeed, fat-free mass has been shown to be a significant predictor of maximal speed, endurance, and jump capacity (Gil et al., 2014). Nevertheless, improvements in performance are also dependent on increases in anthropometric traits, body mass, skeletal muscle mass, heart and lung mass, hemoglobin level, and blood volume as well as maturation of the nervous system (Stolen et al., 2005). This highlights the importance/influence of growth and maturity in performance. In fact, the optimal period (window of opportunity) to improve physical, technical, and physiological capacities has been postulated to occur between the ages of 12 and 16 years, during maturation (Lloyd and Oliver, 2012).
During adolescence, the interaction between genes, hormones, nutrients, and environmental factors triggers a series of physical and functional alterations in the body (Borges et al., 2018). The capacities mentioned above show their greatest improvement during the adolescent growth spurt (Pearson et al., 2006). This period does not occur at the same time in all players, favoring the early maturing players. Teixeira et al. (2014) showed that early maturing players are taller and heavier and attained higher absolute values in ventilatory thresholds and maximal peak of oxygen consumption compared to average maturing players, even after normalizing for interindividual variability in anthropometric traits. Because of their advanced anthropometric and physical profile (Figueiredo et al., 2010; Meylan et al., 2010), early maturing footballers play regularly and are more often selected for regional and national teams (Meylan et al., 2010). In fact, the literature has confirmed that players that are born in the first months of the year, normally have an advantage as their maturation is more advanced compared to athletes who were born in the third and fourth quarters of the year (Deprez et al., 2012). In a study that analyzed age and maturity in soccer, Rommers et al. (2019), showed significant differences among several successive age categories, highlighting that the pubertal period is a critical time for skill acquisition and development of performance in youth elite soccer players.
Following the Long-Term Athlete Development model, players aged 16–18 are in the “training to compete” phase of development with highly structured training (Ford et al., 2011). Until that phase, players are still developing their metabolic conditioning, endurance strength, power, and the cognitive skills specific to soccer. Therefore, their response to training load and recovery will be evidently different from adults, who train predominantly to maintain and improve physical traits and performance, as they already have more developed physical characteristics (Lloyd and Oliver, 2012). Thus, to ascertain appropriate loads for each player also regarding their age, it is important to monitor training loads individually (Vahia et al., 2019). In fact, monitoring the training load in soccer is a key component of the training process as it helps set an adequate balance between training and recovery (Gaudino et al., 2015).
The study of youth soccer players’ fitness variations is recurrent in the literature (Vänttinen et al., 2011; Emmonds et al., 2020). However, few studies (Wrigley et al., 2014; Ramirez-Campillo et al., 2019) are found using maturation status or accumulated training load as covariables that interact with the changes in fitness across the season. This factor should be considered since maturation and accumulated training load seem to play an important role in players’ development (Malina, 2014). Additionally, little attention is given to those who improve or do not improve their fitness status across the season. In this respect, an analysis of responders and non-responders is relevant (Atkinson et al., 2019), namely, to identify how the player is responding or not to the process. Eventually, comparing both types of responses may provide additional information to understand the development of players across the season.
Based on the abovementioned reasons, the purposes of this study were (i) to analyze the variations in physiological variables (e.g., VO2max, maximal heart rate—HRmax, heart rate rest—HRrest), neuromuscular variables (e.g., ACC, maximal speed, COD ability), and running-based anaerobic sprint capacity (e.g., peak power—RPP, minimum power—RMP, average power (Schrapf and Tilp, 2013), and Fatigue Index—RFI) during a season using maturation status (MS) and accumulated training load (ATL) as covariables and (ii) to analyze the differences between responders and non-responders (players that improved and those that decreased performance) in relation to each time point with the previous step.
The participants were 23 male youth elite soccer players (Mean ± Standard deviation; age: 15.45 ± 0.24 years; height: 172.70 ± 4.24 cm; body mass: 61.30 ± 5.62 kg; body fat: 8.34 ± 2.89%; VO2max, 48.38 ± 2.55 ml kg–1 min–1) from the same team competing in the national under-16 competition. From the 23 players, 9 were defenders, 6 were midfielders, 4 were wingers, and 4 were forward. The inclusion criteria were as follows: (i) all players participated in at least 90% of the training season; (ii) no use of dietary supplements was allowed; (ii) no non-contact injuries in the 20-weeks period; (iii) players could not participate in any other training during the study; and (iv) players who did not participate in the match during the week had a separate training session without the ball or practice in a small-sided game. Exclusion criteria are as follows: (i) goalkeepers were excluded based on the high variations in physical demands compared to outfield players. Before starting the study, participants were given explanations about the various stages of the research. All players, along with their parents, were notified of the potential risks and benefits of participating in the study. Players, as well as their parents, signed their informed consent to participate in the project. Prior to the start of the study, the Ethics Committee of the University of Isfahan and the University of Mohaghegh Ardabili approved the study, and the recommendations of Human Ethics in Research were followed according to the Helsinki Declaration.
The present study consists of two parts: (i) The first one was a quasi-experimental design with pre-, mid-, and post-tests. In this study, the assessments were performed three times: period 1 (P1 = before league), period 2 (P2 = after league), and middle period (Mid = mid league), in the eleventh week of the study. (ii) The second study was a cohort study with daily monitoring over 20 consecutive weeks in the competition season. Details can be seen in Table 1. This study started in August 2019 and ended in February 2020. The players performed a total of four training sessions, one match with two rest days per week. In each period, the players were assessed on four consecutive days. On the first day, assessments were made of anthropometric and body composition (e.g., height, sitting height, body mass, body fat), MS for calculating peak height velocity (PHV), and maturity offset performed only in P1. On the second day, the maximal speed, ACC, and COD ability with device Newtest Powertimer 300-series testing system made in Finland were measured. On the third day, the anaerobic power was assessed. Finally, on the fourth day, the aerobic power test with a heart rate sensor (Mi-Band 3; Xiaomi Company, China) for maximum heart rate (HRmax) was conducted. Players were divided into two groups according to the average scores in the P1 assessments: (i) responders, who were above average, and (ii) non-responders, who were below average in each variable (i.e., physiological, neuromuscular, and anaerobic), then comparisons of P1 to the Mid and P2 were performed. Testing sessions were carried out for each participant under similar environmental conditions (21–23°C temperature and 50% humidity) and at the same time on the 3 days of physical fitness tests (Pescatello et al., 2014). In all sessions, the tests were performed between at 3:30 and 6:30 pm. All players reported the training load 30 min after each training session, then each training load was calculated with the training time. Finally, in this study, to evaluate the factors affecting the bio-motor ability of the youth players, ATL and MS (age at PHV) were considered as covariates.
Height (standing and sitting) and weight measurements, respectively, were made with a SECA Model 213 stadiometer, made in Germany with a typical error of 0.5 mm and a SECA scale, model 813, made in England, with a typical error of ± 0.1 kg. Measurements were performed according to the International Society for the Advancement of Kinanthropometry Standards. The Mirwald formula was used to determine maturity offset and age at PHV (Mirwald et al., 2002).
All measurements were performed in the morning by an exercise physiologist with 5 years’ experience (Arazi et al., 2015). The Jackson and Pollock seven-point method (Jackson and Pollock, 1978) was used to determine the body fat percentage with a calibrated Lafayette Skinfold Calliper (Lafayette, IN, United States) with an accuracy of 0.1 mm. All measurements were performed on the right side of the body (chest, abdominal, thigh, triceps, subscapular, suprailiac, and midaxillary). At this point, considerations made in the previous study were used to reduce the measurement error (Nobari et al., 2020). Measurements were taken three times and the average of the three was used for the formula (Nobari et al., 2020).
Players recorded their rating of perceived exertion (RPE) using the CR-10 Borg’s scale (Borg, 1998) that is a valid and reliable scale to estimate the intensity of a session. Thirty minutes after the end of the training session, the RPE of each player was noted between 0 (minimum effort) and 10 (maximal effort) after answering this question “What was the intensity of your session?” As a measure of internal load, the session-RPE was calculated multiplying the score on the CR-10 scale by the duration of the session in minutes (Foster et al., 2001). Players were familiar with the scale and had used it for 2 years in the club. Total ATL for training and competition was calculated as follows: the first weekly TL+ the second weekly TL+…+ up to the twentieth week. Figure 1 presents information about the general characteristics of the weekly training loads carried out during the 20 consecutive weeks in the competitive season.
Figure 1. Demonstrated weekly training loads carried out during the 20 consecutive weeks. wTL, weekly training load; AU, arbitrary unit.
The Intermittent Fitness Test 30-15 (30-15IFT) was taken to calculate the VO2max and the readiness level of the subjects (Buchheit, 2008, 2010). This test consists of 30 s shuttle runs interspaced with 15 s passive recovery periods. The initial velocity was set at 8 km h–1 with 0.5 km h–1 increments for each stage. After 10 min of a standard warm-up, subjects were divided into groups of four and stood on the start line (A); when they heard the first beep, they were required to run back and forth between 2 lines set 40 m apart at a pace marked by a prerecorded beep. During the 15 s recovery time, subjects walked forward to the closest line depending on where they had stopped the previous run and began the next run from this line. This test went on until subjects could no longer continue the test, or three consecutive times could not reach the two end lines. The 30-15IFT was used to determine VO2max with the following formula (Buchheit, 2010): VO2max (ml kg–1 min–1) = 28.3 – (2.15 × 1) – (0.741 × 16 years) – (0.0357 × weight) + (0.0586 × 16 years × VIFT) + (1.03 × VIFT). VIFT was the final running speed attained. The test–retest reliability of the test was calculated with an intra-class correlation coefficient (ICC). The ICC was 0.86 for this test.
The HRmax calculation test was performed with the Mi-Band 3 in the 30-15IFT test. Each subject has been monitoring with the watch on the wrist. HRmax was recorded with the Mi-Band 3 in the 30-15IFT test, and each subject was fitted with the monitor on his wrist before each test. HRmax recorded during the test was considered as a reporting criterion of HRmax. To measure resting heart rate (HRrest), the recommendation was to measure their HRrest in the morning after a rest day. The measurement had to be performed in a supine position in the morning immediately after waking up. Subjects put an HR sensor (Mi-Band 3; Xiaomi Company, made in China) on their wrist, lay down on their backs, and stayed in a relaxed position. After about 10 min, they could start the HR monitor. They continued to lie still and breathe calmly for 3–5 min without looking at the monitor. Then they stopped it. This process was repeated for 3 days, then the researcher checked the summary for their average HR for the 3 days, which was taken as HRrest for all players.
For the acceleration and maximal speed test, a digital timer connected to two photocells was placed at hip height, and after a 10 min specific warm-up subjects stood 70 cm behind the start line (line “A”). To calculate the ACC, when the voice from the speakers said: Ready, Go! subjects started to run from the start line, where the first photocell gate was placed, to the 10 m as fast as possible. When subjects passed through the first photocell gate, the digital timer started, and when they reached the second gate the timer stopped, recording the ACC for each subject.
To calculate maximal speed (Mirkov et al., 2008), the test was performed over a distance of 30 m. The best value obtained from 3 trials was used for statistical analysis. They had at least 3 min rest between each trial, and all phases of testing were monitored by the coach. The ICC was 0.91 for ACC and 0.94 for maximal speed.
The soccer players did a “modified 505” (Sheppard and Young, 2006) after the warm-up, they rested for 3–5 min to recover. Then, subjects stood 70 cm behind the start line “A.” Line “B” and line “C” were placed 5 and 10 m, respectively, from “A.” The tests were timed by photocells placed on line “B.” All the participants did the test twice with a 3 min recovery in between. They had to touch line C with their hands and then change direction as quickly as possible and return to the start line “A.” The tests were supervised by the coach or researcher, and the Photo-finish system recorded the time taken to complete the 5 m (out and return) (2 × 5 m). The best time was used for the statistical analysis. The ICC for the COD was equal to 0.94.
A RAST was used to measure anaerobic power. To perform this test, two photocells were placed at 35 m from each other. Each participant had to run at maximal speed for 6 repetitions and take a 10 s rest between each repetition. After performing a warm-up following the same instructions as for the other tests, the participants began running at maximal speed. After crossing the 35 m line, they rested for 10 s (seconds were counted aloud by the investigator) and then immediately started again at the end of 10 s; this process continued for six repetitions. The records of each participant were calculated with the following formulas and considered for anaerobic power variables; RAST of peak power (RPP) = the highest value; RAST of minimum power (RMP) = the lowest value; RAST of average power (Schrapf and Tilp, 2013) = sum of all six values ÷ 6; and RAST of Fatigue Index (RFI) = (Maximum power − Minimum power) ÷ Total time for the 6 sprints. Test–retest reliability calculated with the ICC was 0.91 for this test. All maximal speed, COD, and anaerobic power tests were performed using the Newtest Powertimer 300-series testing system made in Finland.
Homogeneity of the data was verified with the Levene test and the normality of the data with the Shapiro–Wilk test. Data (i.e., aerobic and anaerobic power, HR measurements, and speed variables) were analyzed using repeated-measure ANOVA with covariates (accumulated training load and PHV). In the model, they show the following values: accumulated training load = 32082.44 arbitrary units (AU) and age at PHV = 13.60 years. Then, all variables were analyzed with the LSD post hoc test. Hedge’s g effect size model was calculated for different time points regarding pairwise comparisons. The interpretation of magnitude of changes was as follows (Batterham and Hopkins, 2006; Hopkins et al., 2009): trivial < 0.2; small = 0.01; moderate = 0.6–1.2; large = 1.2–2.0; very large = 2.0–4.0; and extremely large≥4.0. Finally, the independent t-test divided responders and non-responders by the cutoff values considering the average scores in P1 assessments for each variable and then comparing P1 to the Mid and P2 (comparison of groups from the P1 is done, and this way the groups are considered the same in all comparisons.) We calculated the ICC for reliability (including neuromuscular variables) and test–retest reliability (including aerobic and anaerobic variables). Data analysis was performed using SPSS software (version 23.0), and the significance level was set at P < 0.05. We performed to calculate an a posteriori estimation of statistical power; the statistical software (G-Power; University of Dusseldorf, Dusseldorf, Germany) was used. Given the study design (the difference between two independent means for two groups), accordingly, an alpha risk of 0.05 was used in a two-sided test.
The individual characteristics of the subjects are presented in Table 2.
The repeated-measure ANOVA of the VO2max demonstrated main effects of time [F(2, 5.20), P = 0.009], whereas after the entry of the ATL [F(1, 0.95), P = 0.343] and MS [F(1, 1.39), P = 0.253] as covariates, there was not a statistical significance between time points (Table 3). Post hoc tests showed that there was a significant difference between P1-mid (P = 0.001) as well as with a covariate ATL and MS (P = 0.001). The overall effect size was small (g = 0.34) (Table 4).
Table 3. Accumulated training load and maturation status data for fitness tests and relationship with different time points.
Table 4. Accumulated training load and maturation status data for fitness tests in relation to different time points and pairwise comparisons.
The RPP demonstrated main effects of time [F(1.02, 30.96), P ≤ 0.001] while, after the entry of the ATL [F(1.02, 0.99) = 0.34] and MS [F(1.03, 0.57), P = 0.46] as a covariate, no significant difference between time points was noticed (Table 3). Post hoc tests showed there was a significant difference between P1-mid (LSD; P = 0.011 and with a covariate ATL and MS; P = 0.014; g = 0.04, trivial), mid-P2, and P1-P2 (P ≤ 0.001) with and without considering the covariate. In both these time periods, effect size was moderate (Table 4).
The RFI demonstrated main effects of time [F(1.28, 34.46), P ≤ 0.001] while, after the entry of the ATL [F(1.30, 0.28), P = 0.666] and MS [F(1.30, 2.56), P = 0.114] as a covariate, there was not a significant result between time points (Table 3). Post hoc tests showed that there was a significant difference between mid-P2 (P ≤ 0.001; g = 0.82 moderate) and P1–P2 (P ≤ 0.001; g = 0.86 moderate) with and without considering the covariate.
Post hoc power analysis was performed to identify the power obtained for t-test analysis, based on an alpha risk of 0.05 in a two-sided test. Statistical power acceptance was for VO2max (97%), HRrest (99%), Max speed (99%), AG (94%), RPP (99%), RAP (98%), and RFI (96%). Therefore, a statistically significant difference can be identified.
Table 5 presents the differences in performance between responders and non-responders. Between P1 to mid and P1 to P2, all variables showed significant results (all with P < 0.001) between responders and non-responders. Only HRmax showed no differences between responders and non-responders at P1 to P2.
Table 5. Between-group differences of physiological variables (mean ± standard deviation) between responders and non-responders considering the baseline levels.
This study aimed to analyze the variations in physiological, neuromuscular, and running-based anaerobic sprint variables during a season using maturation status and accumulated training load as covariables. Additionally, it was also the purpose of this study analyze the differences between responders and non-responders (players that improved and those that decreased performance) in relation to each time point with the previous step. The main evidence of the current study revealed that VO2max, maximal speed, RPP, RAP, and RFI significantly changed across the assessment periods. Interestingly, using accumulated training load and maturation status as covariates revealed no statistical significance. When analyzing responders and non-responders, only HRmax (between periods 1 and 2) showed no differences between the groups.
In the first period of evaluation, increases in VO2max, RPP, and RAP were noticed with and without the inclusion of the ATL and MS covariates. In fact, soccer requires a high aerobic capacity, as the duration of a match is 90 min, and although less abundant compared with adults, studies on youth soccer report distances of 6.5 km (Under-13), 7.4 km (Under-14), and 8.1 km (Under-15) covered during competitions (Stolen et al., 2005). The focus of its increment seems to be in the preseason (about 27.2% in endurance performance) (Dragijsky et al., 2017), which could explain only a small effect in the results of the present study, as the analysis was initiated already at the beginning of the league competitions, excluding the preseason. Nevertheless, in other studies (Bangsbo, 1994; Haritonidis et al., 2004), an increased aerobic endurance level was reported at the beginning to the middle of the season. Likewise, repeated high-speed actions are considered a crucial physical component in soccer matches (Buchheit et al., 2010), as during a match some 150–250 are performed (Bangsbo et al., 2007). However, studies in young soccer players seem to suggest that its stimulation does not occur during the preseason (Dragijsky et al., 2017), as opposed to adults (Dianzenza et al., 2009). However, this study does not cover the pre-season period. However, in the first period of competition, a seemingly good progress was observed in the RAP and RPP variables with trivial and moderate effect size, respectively.
From the mid to the P2 periods, maximal speed, RPP, RAP, and RFI increased. In fact, considering the anaerobic variables related to sprinting, only RMP did not record significant results. During a soccer game, sprinting bouts generally occur every 90 s and each of these sprints last approximately 2–4 s (Bangsbo et al., 2006; Burgess et al., 2006), totaling 1,000–1,400 high-intensity short-duration activities (Stolen et al., 2005). Thus, preserving a high level of these capacities throughout the season is necessary for achieving consistent high-quality performance (Reilly et al., 2000a), while the basis for these individual players’ components are built during youth (Dragijsky et al., 2017). The literature has shown that elite players are faster than sub-elite players in maximal speed tests, and sprint time over 15 m was the strongest discriminator no matter the player’s position. Hence, elite players were found to better reproduce their top speed in the repetitive sprint tests and were more tolerant to fatigue (Reilly et al., 2000b). The results of the present study seem to follow this assumption, as a moderate effect size was recorded for RPP and RFI between the mid and P2 periods, confirming the focus of their coach to develop sprint capacity. Likewise, although with a lower effect (small), maximal speed and RAP also showed significant improvements in this period.
When analyzing the evolution of soccer matches, a greater focus on sprint abilities rather than aerobic capacity has been observed in different studies, underlying that elite or professional players have become faster over time while aerobic capacity has plateaued or slightly decreased (Haugen et al., 2013). On the one hand, these concerns seem to be confirmed in the present study, since in the second observation period (mid to P2 period), almost all speed components showed significant increases over the 20 weeks of analysis. The effect was also moderate for RPP and RFI, and small for maximal speed and RAP. On the other hand, the player’s age and stage of development, i.e., biological maturation, should be considered as they have a great influence on maximal speed, power, and strength improvements (Dragijsky et al., 2017). This capacity, which is considered a complex fitness trait, is apparently related to neuromuscular (Buchheit, 2012) and metabolic factors (Girard et al., 2011) and is strongly based on the myelination of motor nerves and neural maturation.
This process is not complete until sexual maturity is reached (De Ste Croix et al., 2003). In fact, muscle strength in the lower limbs has been considered to increase up to 50% between 11 and 15 years in boys (Degache et al., 2010), influencing sprint ability that improves progressively from ages 11 to 17 years (Valente-dos-Santos et al., 2012).
During adolescence, the interaction between genes, hormones, nutrients, and environmental factors triggers a series of physical and functional alterations in the body (Borges et al., 2018). The literature has shown that physical performance is highly influenced by maturation (Vandendriessche et al., 2012), it being the optimal period (window of opportunity) to improve physical, technical, and physiological capacities between the ages of 12 and 16 years (Balyi and Hamilton, 2004). In fact, studies have highlighted that early maturating players are at an advantage, as they have shown to have greater height, body mass, strength, and aerobic endurance (Teixeira et al., 2018). Therefore, the pubertal period is a critical time frame for skill acquisition and development of performance in youth elite soccer players (Rommers et al., 2019). All of the above could explain the impact observed with the inclusion of MS and ATL covariates, since the players that participated in the present study are within these ages.
The analysis between responders and non-responders made it possible to confirm that the variation in response around the mean alludes to an interindividual variation in exercise and training responses, since only HRmax (between P1 and P2) showed no differences between these groups. Indeed, it is known that HRmax is relatively unaltered regardless of training status in a given group (Zavorsky, 2000). The non-responder term has been used to describe individuals who showed a worsened or unchanged response after training (Rampinini et al., 2007) or, more accurately, individuals whose training response did not exceed the variation of a particular measurement (Vigne et al., 2010). In the present study, this comparison strengthened the impact of covariates, i.e., the ATL perception and the MS could really influence the performance improvement, although the average values did not always indicate this. In fact, in all variables where improvements were recorded in the whole group (mean values), both MS and ATL showed their impact.
The current study presents some limitations. One of them is the small sample tested. Eventually, future research should include a larger sample to reinforce the power of the evidence. Additionally, monitoring both internal and external training load during the periods may be interesting to add more information about the discriminating factor of different types of stimuli. Future research should consider also monitoring additional activities such as weight room training or regular physical activity performed extra to field-based training. A final limitation is the fact that responders and non-responders were not specifically analyzed using the smallest worthwhile change. Future studies should use such an approach to better determine if a player can be a bad responder to the training.
Despite these limitations, and as a conclusion, maximal speed and its derived variables seem to be the focus in this age group of soccer players, as significant improvements were noticed in the whole evaluated period, especially between mid and P2. In the first period of evaluation, the main concern of their coach would be the improvement of aerobic capacity, as VO2max showed significant improvements in the whole group. All these variables seemed to be influenced by ATL and MS, since when included as covariates, the differences vanished. Additionally, almost all variables presented differences between responders and non-responders, highlighting the individual responses to training.
As a practical application, the relevance of accumulated training load and maturation status on the development of the fitness level of the players should be emphasized. Thus, coaches should consider adapting the training stimuli to the specific characteristics of the players (namely, maturation status) as well as not interpreting changes in fitness exclusively focusing on the training, but also weighing the maturation process. Possibly, more individualized training is needed, as well as a better relationship between player’s assessment and monitoring and the training plan.
The original contributions presented in the study are included in the article/supplementary material, further inquiries can be directed to the corresponding authors.
The studies involving human participants were reviewed and approved by the Ethics Committee of the University of Isfahan and the University of Mohaghegh Ardabili. Written informed consent to participate in this study was provided by the participants’ legal guardian/next of kin.
HN, FC, and JP-G designed the study and drafted the manuscript. HN performed the experiments. HN, AS, MS, MG-G, and JA participated in the data analysis and drafted the manuscript. JP-G, FC, and HN revised the critical manuscript. All authors read and approved the final version of the manuscript.
The authors declare that the research was conducted in the absence of any commercial or financial relationships that could be construed as a potential conflict of interest.
We thank Sepahan F. C. for helping to increase their football knowledge.
Alfredson, H., Nordström, P., and Lorentzon, R. (1996). Total and regional bone mass in female soccer players. Calcif. Tissue Int. 59, 438–442. doi: 10.1007/BF00369207
Arazi, H., Mirzaei, B., and Nobari, H. (2015). Anthropometric profile, body composition and somatotyping of national Iranian cross-country runners. Turkish J. Sport Exerc. 17:35. doi: 10.15314/tjse.49873
Atkinson, G., Williamson, P., and Batterham, A. M. (2019). Issues in the determination of ‘responders’ and ‘non-responders’ in physiological research. Exp. Physiol. 104, 1215–1225. doi: 10.1113/EP087712
Balyi, I., and Hamilton, A. (2004). Long-Term Athlete Development: Trainability in Childhood and Adolescence. Windows of Opportunity. Optimal Trainability. Victoria: National Coaching Institute British Columbia & Advanced Training and Performance Ltd.
Bangsbo, J. (1994). The physiology of soccer - with special reference to intense intermittent exercise. Acta Physiol. Scand. 619(Suppl. 02), 1–155.
Bangsbo, J., Iaia, F. M., and Krustrup, P. (2007). Metabolic response and fatigue in soccer. Int. J. Sports Physiol. Perform. 2, 111–127. doi: 10.1123/ijspp.2.2.111
Bangsbo, J., Mohr, M., and Krustrup, P. (2006). Physical and metabolic demands of training and match-play in the elite football player. Nutr. Footb. 0414, 1–18. doi: 10.4324/9780203967430
Batterham, A. M., and Hopkins, W. G. (2006). Making meaningful inferences about magnitudes. Int. J. Sports Physiol. Perform. 1, 50–57. doi: 10.1123/ijspp.1.1.50
Boraczyński, M., Boraczyński, T., Podstawski, R., and Wójcik, Z. (2015). Relationships between anthropometric traits, body composition and aerobic capacity in male soccer players aged 13–15 years. J. Kinesiol. Exer Sci. 69, 33–40.
Borges, P. H., Cumming, S., Ronque, E. R. V., Cardoso, F., Avelar, A., Rechenchosky, L., et al. (2018). Relationship between tactical performance, somatic maturity and functional capabilities in young soccer players. J. Hum. Kinet. 64, 160–169. doi: 10.1515/hukin-2017-0190
Buchheit, M. (2008). The 30-15 intermittent fitness test: accuracy for individualizing interval training of young intermittent sport players. J. Strength Cond. Res. 22, 365–374. doi: 10.1519/JSC.0b013e3181635b2e
Buchheit, M. (2012). Repeated-Sprint performance in team sport players: associations with measures of aerobic fitness, metabolic control and locomotor function. Int. J. Sports Med. 33, 230–239. doi: 10.1055/s-0031-1291364
Buchheit, M., Mendez-Villanueva, A., Simpson, B. M., and Bourdon, P. C. (2010). Match running performance and fitness in youth soccer. Int. J. Sports Med. 31, 818–825. doi: 10.1055/s-0030-1262838
Burgess, D. J., Naughton, G., and Norton, K. I. (2006). Profile of movement demands of national football players in Australia. J. Sci. Med. Sport 9, 334–341. doi: 10.1016/j.jsams.2006.01.005
Carling, C. (2013). Interpreting physical performance in professional soccer match-play: should we be more pragmatic in our approach? Sports Med. 43, 655–663. doi: 10.1007/s40279-013-0055-8
Coratella, G., Beato, M., and Schena, F. (2016). The specificity of the Loughborough Intermittent Shuttle Test for recreational soccer players is independent of their intermittent running ability. Res. Sports Med. 24, 363–374. doi: 10.1080/15438627.2016.1222279
De Ste Croix, M. B. A., Deighan, M. A., and Armstrong, N. (2003). Assessment and interpretation of isokinetic muscle strength during growth and maturation. Sports Med. 33, 727–743. doi: 10.2165/00007256-200333100-00002
Degache, F., Richard, R., Edouard, P., Oullion, R., and Calmels, P. (2010). The relationship between muscle strength and physiological age: a cross-sectional study in boys aged from 11 to 15. Ann. Phys. Rehabil. Med. 53, 180–188. doi: 10.1016/j.rehab.2010.02.001
Deprez, D., Vaeyens, R., Coutts, A., Lenoir, M., and Philippaerts, R. (2012). Relative age effect and Yo-Yo IR1 in youth soccer. Int. J. Sports Med. 33, 987–993. doi: 10.1055/s-0032-1311654
Dianzenza, E., rusin, M., and stupnicki, R. (2009). Anaerobic resistance of soccer players. Fit. Perform. J. 8, 199–203. doi: 10.3900/fpj.8.3.199.e
Dragijsky, M., Maly, T., Zahalka, F., Kunzmann, E., and Hank, M. (2017). Seasonal variation of agility, speed and endurance performance in young elite soccer players. Sports 5:12. doi: 10.3390/sports5010012
Emmonds, S., Sawczuk, T., Scantlebury, S., Till, K., and Jones, B. (2020). Seasonal changes in the physical performance of elite youth female soccer players. J. Strength Cond. Res. 34, 2636–2643. doi: 10.1519/JSC.0000000000002943
Figueiredo, A. J., Silva, M. J. C., Cumming, S. P., and Malina, R. M. (2010). Size and maturity mismatch in youth soccer players 11- to 14-years-old. Pediatr. Exerc. Sci. 22, 596–612. doi: 10.1123/pes.22.4.596
Ford, P., De Ste Croix, M., Lloyd, R., Meyers, R., Moosavi, M., Oliver, J., et al. (2011). The long-term athlete development model: physiological evidence and application. J. Sports Sci. 29, 389–402. doi: 10.1080/02640414.2010.536849
Foster, C., Florhaug, J., Franklin, J., Gottschall, L., Hrovation, L., Parker, S., et al. (2001). A new approach to monitoring exercise training. J. Strength Cond. Res. 15, 109–115.
Gaudino, P., Iaia, F. M., Strudwick, A. J., Hawkins, R. D., Alberti, G., Atkinson, G., et al. (2015). Factors influencing perception of effort (Session Rating of Perceived Exertion) during elite soccer training. Int. J. Sports Physiol. Perform. 10, 860–864. doi: 10.1123/ijspp.2014-0518
Gil, S. M., Badiola, A., Bidaurrazaga-Letona, I., Zabala-Lili, J., Gravina, L., Santos-Concejero, J., et al. (2014). Relationship between the relative age effect and anthropometry, maturity and performance in young soccer players. J. Sports Sci. 32, 479–486. doi: 10.1080/02640414.2013.832355
Girard, O., Mendez-Villanueva, A., and Bishop, D. (2011). Repeated-Sprint Ability – Part I: factors contributing to fatigue. Sports Med. 41, 673–694. doi: 10.2165/11590550-000000000-00000
Haritonidis, K., Koutlianos, N., Kouidi, E., Haritonidou, M., and Deligiannis, A. (2004). Seasonal variation of aerobic capacity in elite soccer, basketball and volleyball players. J. Hum. Mov, Stud. 46, 289–302.
Haugen, T. A., Tønnessen, E., and Seiler, S. (2013). Anaerobic performance testing of professional soccer players 1995–2010. Int. J. Sports Physiol. Perform. 8, 148–156. doi: 10.1123/ijspp.8.2.148
Hopkins, W. G., Marshall, S. W., Batterham, A. M., and Hanin, J. (2009). Progressive statistics for studies in sports medicine and exercise science. Med. Sci. Sports Exerc. 41, 3–13. doi: 10.1249/MSS.0b013e31818cb278
Hulse, M., Morris, J., Hawkins, R., Hodson, A., Nevill, A., and Nevill, M. (2012). A field-test battery for elite, young soccer players. Int. J. Sports Med. 34, 302–311. doi: 10.1055/s-0032-1312603
Jackson, A. S., and Pollock, M. L. (1978). Generalized equations for predicting body density of men. Br. J. Nutr. 40, 497–504. doi: 10.1079/BJN19780152
Lloyd, R. S., and Oliver, J. L. (2012). The Youth physical development model. Strength Cond. J. 34, 61–72. doi: 10.1519/SSC.0b013e31825760ea
Malina, R. M. (2014). Top 10 research questions related to growth and maturation of relevance to physical activity, performance, and fitness. Res. Q. Exerc. Sport 85, 157–173. doi: 10.1080/02701367.2014.897592
Malina, R. M., Bouchard, C., and Bar-Or, O. (2004). Growth, Maturation, and Physical Activity. Champaign, IL: Human kinetics.
Meylan, C., Cronin, J., Oliver, J., and Hughes, M. (2010). Talent identification in soccer: the role of maturity status on physical, physiological and technical characteristics. Int. J. Sports Sci. Coach. 5, 571–592. doi: 10.1260/1747-9541.5.4.571
Mirkov, D., Nedeljkovic, A., Kukolj, M., Ugarkovic, D., and Jaric, S. (2008). Evaluation of the reliability of soccer-specific field tests. J. Strength Cond. Res. 22, 1046–1050. doi: 10.1519/JSC.0b013e31816eb4af
Mirwald, R. L., Baxter-Jones, A. D., Bailey, D. A., and Beunen, G. P. (2002). An assessment of maturity from anthropometric measurements. Med. Sci. Sports Exerc. 34, 689–694. doi: 10.1097/00005768-200204000-00020
Nobari, H., Aquino, R., Clemente, F. M., Khalafi, M., Adsuar, J. C., and Pérez-Gómez, J. (2020). Description of acute and chronic load, training monotony and strain over a season and its relationships with well-being status: a study in elite under-16 soccer players. Physiol. Behav. 225:113117. doi: 10.1016/j.physbeh.2020.113117
Pearson, D. T., Naughton, G. A., and Torode, M. (2006). Predictability of physiological testing and the role of maturation in talent identification for adolescent team sports. J. Sci. Med. Sport 9, 277–287. doi: 10.1016/j.jsams.2006.05.020
Pescatello, L. S., Arena, R., Riebe, D., and Kluwer, P. D. T. W. (2014). ACSM’s Guidelines for Exercise Testing and Prescription. Philadelphia, PA: Lippincott Williams & Wilkins.
Ramirez-Campillo, R., Alvarez, C., Sanchez-Sanchez, J., Slimani, M., Gentil, P., Chelly, M. S., et al. (2019). Effects of plyometric jump training on the physical fitness of young male soccer players: modulation of response by inter-set recovery interval and maturation status. J. Sports Sci. 37, 2645–2652. doi: 10.1080/02640414.2019.1626049
Rampinini, E., Coutts, A. J., Castagna, C., Sassi, R., and Impellizzeri, F. M. (2007). Variation in top level soccer match performance. Int. J. Sports Med. 28, 1018–1024. doi: 10.1055/s-2007-965158
Reilly, T., Bangsbo, J., and Franks, A. (2000a). Anthropometric and physiological predispositions for elite soccer. J. Sports Sci. 18, 669–683. doi: 10.1080/02640410050120050
Reilly, T., Williams, A. M., Nevill, A., and Franks, A. (2000b). A multidisciplinary approach to talent identification in soccer. J. Sports Sci. 18, 695–702. doi: 10.1080/02640410050120078
Rommers, N., Mostaert, M., Goossens, L., Vaeyens, R., Witvrouw, E., Lenoir, M., et al. (2019). Age and maturity related differences in motor coordination among male elite youth soccer players. J. Sports Sci. 37, 196–203. doi: 10.1080/02640414.2018.1488454
Schrapf, N., and Tilp, M. (2013). Action sequence analysis in team handball. J. Hum. Sport Exerc. 8, S615–S621. doi: 10.4100/jhse.2013.8.Proc3.07
Sheppard, J. M., and Young, W. B. (2006). Agility literature review: classifications, training and testing. J. Sports Sci. 24, 919–932. doi: 10.1080/02640410500457109
Stolen, T., Chamari, K., Castagna, C., and Wisloff, U. (2005). Physiology of soccer: an update. Sports Med. 35, 501–536. doi: 10.2165/00007256-200535060-00004
Svensson, M., and Drust, B. (2005). Testing soccer players. J. Sports Sci. 23, 601–618. doi: 10.1080/02640410400021294
Teixeira, A. S., da Silva, J. F., Carminatti, L. J., Dittrich, N., Castagna, C., and Guglielmo, L. G. A. (2014). Reliability and validity of the carminatti’s test for aerobic fitness in youth soccer players. J. Strength Cond. Res. 28, 3264–3273. doi: 10.1519/JSC.0000000000000534
Teixeira, A. S., Guglielmo, L. G. A., Fernandes-da-Silva, J., Konarski, J. M., Costa, D., Duarte, J. P., et al. (2018). Skeletal maturity and oxygen uptake in youth soccer controlling for concurrent size descriptors. PLoS One 13:e0205976. doi: 10.1371/journal.pone.0205976
Tomlin, D. L., and Wenger, H. A. (2001). The relationship between aerobic fitness and recovery from high intensity intermittent exercise. Sports Med. 31, 1–11. doi: 10.2165/00007256-200131010-00001
Vahia, D., Kelly, A., Knapman, H., and Williams, C. A. (2019). Variation in the correlation between heart rate and session rating of perceived exertion-based estimations of internal training load in youth soccer players. Pediatr. Exerc. Sci. 31, 91–98. doi: 10.1123/pes.2018-0033
Valente-dos-Santos, J., Coelho-e-Silva, M., Martins, R., Figueiredo, A., Cyrino, E., Sherar, L., et al. (2012). Modelling developmental changes in repeated-sprint ability by chronological and skeletal ages in young soccer players. Int. J. Sports Med. 33, 773–780. doi: 10.1055/s-0032-1308996
Vandendriessche, J. B., Vaeyens, R., Vandorpe, B., Lenoir, M., Lefevre, J., and Philippaerts, R. M. (2012). Biological maturation, morphology, fitness, and motor coordination as part of a selection strategy in the search for international youth soccer players (age 15–16 years). J. Sports Sci. 30, 1695–1703. doi: 10.1080/02640414.2011.652654
Vänttinen, T., Blomqvist, M., Nyman, K., and Häkkinen, K. (2011). Changes in body composition, hormonal status, and physical fitness in 11-, 13-, and 15-year-old finnish regional youth soccer players during a two-year follow-up. J. Strength Cond.Res. 25, 3342–3351. doi: 10.1519/JSC.0b013e318236d0c2
Vigne, G., Gaudino, C., Rogowski, I., Alloatti, G., and Hautier, C. (2010). Activity profile in elite italian soccer team. Int. J. Sports Med. 31, 304–310. doi: 10.1055/s-0030-1248320
Wrigley, R., Drust, B., Stratton, G., Atkinson, G., and Gregson, W. (2014). Long-term soccer-specific training enhances the rate of physical development of academy soccer players independent of maturation status. Int. J. Sports Med. 35, 1090–1094. doi: 10.1055/s-0034-1375616
Keywords: internal load, monitoring, performance, football, youth
Citation: Nobari H, Silva AF, Clemente FM, Siahkouhian M, García-Gordillo MÁ, Adsuar JC and Pérez-Gómez J (2021) Analysis of Fitness Status Variations of Under-16 Soccer Players Over a Season and Their Relationships With Maturational Status and Training Load. Front. Physiol. 11:597697. doi: 10.3389/fphys.2020.597697
Received: 21 August 2020; Accepted: 22 December 2020;
Published: 05 February 2021.
Edited by:
Beat Knechtle, University Hospital Zurich, SwitzerlandReviewed by:
Beata Pluta, Poznań University of Physical Education, PolandCopyright © 2021 Nobari, Silva, Clemente, Siahkouhian, García-Gordillo, Adsuar and Pérez-Gómez. This is an open-access article distributed under the terms of the Creative Commons Attribution License (CC BY). The use, distribution or reproduction in other forums is permitted, provided the original author(s) and the copyright owner(s) are credited and that the original publication in this journal is cited, in accordance with accepted academic practice. No use, distribution or reproduction is permitted which does not comply with these terms.
*Correspondence: Hadi Nobari, aGFkaS5ub2JhcmkxQGdtYWlsLmNvbQ==; Jorge Pérez-Gómez, am9yZ2VwZzEwMEBnbWFpbC5jb20=
Disclaimer: All claims expressed in this article are solely those of the authors and do not necessarily represent those of their affiliated organizations, or those of the publisher, the editors and the reviewers. Any product that may be evaluated in this article or claim that may be made by its manufacturer is not guaranteed or endorsed by the publisher.
Research integrity at Frontiers
Learn more about the work of our research integrity team to safeguard the quality of each article we publish.