- 1Anhui Province Key Laboratory of Local Livestock and Poultry Genetic Resource Conservation and Bio-breeding, College of Animal Science and Technology, Anhui Agricultural University, Hefei, China
- 2Key Laboratory of Animal Reproduction and Germplasm Enhancement in Universities of Shandong, College of Life Sciences, Qingdao Agricultural University, Qingdao, China
- 3State Key Laboratory of Reproductive Regulation and Breeding of Grassland Livestock, College of Life Sciences, Inner Mongolia University, Hohhot, China
Long non-coding RNA (lncRNA) is involved in many biological processes, and it has been closely investigated. However, research into the role of lncRNA in ovine ovarian development is scant and poorly understood, particularly in relation to the molecular mechanisms of ovine oocyte maturation. In the current study, RNA sequencing was performed with germinal vesicle (GV) and in vitro matured metaphase II (MII) stage oocytes, isolated from ewes. Through the use of bioinformatic analysis, abundant candidate lncRNAs in stage-specific ovine oocytes were identified, and their trans- and cis-regulatory effects were deeply dissected using computational prediction software. Functional enrichment analysis of these lncRNAs revealed that they were involved in the regulation of many key signaling pathways during ovine oocyte development, which was reflected by their targeted genes. From this study, multiple lncRNA–mRNA networks were presumed to be involved in key signaling pathways regarding ovine oocyte maturation and meiotic resumption. In particular, one novel lncRNA (MSTRG.17927) appeared to mediate the regulation of phosphatidylinositol 3-kinase signaling (PI3K) signaling during ovine oocyte maturation. Therefore, this research offers novel insights into the molecular mechanisms underlying ovine oocyte meiotic maturation regulated by lncRNA–mRNA networks from a genome-wide perspective.
Introduction
In mammals, fully grown follicles release mature oocytes that are ready for fertilization. Each oocyte is enclosed in a follicle and undergoes an extended period of meiotic arrest that may last 40 years or more in humans and some other animals (Woodruff et al., 2010; Conti et al., 2012), during which time the oocytes remain at the first meiotic prophase. Only when luteinizing hormones (LH) triggers ovulation in the adolescent do the germinal vesicle (GV) oocytes resume meiosis, complete the first meiosis, extrude a polar body, and then reach the metaphase of the second meiosis (MII) ready for fertilization.
A long period of meiotic prophase or GV arrest is suggested to be of great importance for the production of a fertilization-competent oocyte (Conti et al., 2012; Coticchio et al., 2013). The maintenance of this arrest involves a regulatory network between oocytes and somatic cells, thought to be dependent on high levels of cyclic AMP (cAMP) (Cho et al., 1974; Bornslaeger et al., 1986; Conti et al., 2002, 2012) that can activate protein kinase A (PKA) and contribute to the inactivation of the meiosis-promoting factor (MPF), as cAMP decreases PKA is inactivated leading to the activation of MPF, which is responsible for the resumption of oocyte development and GV break down (GVBD) (Jones, 2004; Coticchio et al., 2013). During arrest, cAMP levels within oocytes usually remain relatively high. cAMP can be specifically degraded by phosphodiesterase 3A (PDE3A); however, PDE3A activity is inhibited by cyclic GMP (cGMP) (Norris et al., 2009; Vaccari et al., 2009) that is produced by cumulus cells and diffuses through gap junctions into the oocyte to prevent initiation of meiotic resumption. Following the LH surge, the secretion of natriuretic peptide precursor type C in mural granulosa cells is prevented, which impairs cGMP synthesis (Zhang M. et al., 2010; Wang et al., 2019); as a result, PDE3A is activated, and cAMP is reduced by phosphodiesterase, which in turn permits the resumption of meiosis.
Non-coding RNAs (ncRNAs) are generally divided into two classes according to length: short/small ncRNAs [such as micro-RNAs (miRNAs)] and long non-coding RNAs (lncRNAs) with a length generally exceeding 200 bp (Nagano and Fraser, 2011; Sun and Kraus, 2013). Following the prevalence of genome-wide transcriptome research, a substantial set of roles for lncRNAs are gradually being exposed. Growing evidence suggests that lncRNAs participate in many biological processes, such as the regulation of gene expression (Kim and Sung, 2012; Rinn and Chang, 2012), cellular differentiation and development (Fatica and Bozzoni, 2014; Liu et al., 2017), apoptosis, and disease (Wapinski and Chang, 2011; Rossi and Antonangeli, 2014; Zhang et al., 2019). Moreover, lncRNAs are known to target chromatin modification complexes that mediate posttranscriptional modifications and translational control of messenger RNA (Fatica and Bozzoni, 2014); they also play a part in genomic reprogramming (Nagano et al., 2008; Loewer et al., 2010). Despite the fact that lncRNAs, such as the well-known H19 and Xist (X inactive-specific transcript), were identified as early as the 1990s (Brockdorff et al., 1991; Carninci et al., 2005), their roles are relatively poorly investigated and understood, particularly in the ovary. Presently, most studies have focused on the association of lncRNAs with ovarian cancer (Norris et al., 2009; Mitra et al., 2017), and only a few reports have investigated their roles in ovarian development and follicle growth. Battaglia et al. (2017) suggest that 41 lncRNAs interact with oocyte miRNAs and are potentially involved in the regulation of folliculogenesis in humans. Meanwhile, Liu et al. (2018) discovered that lncRNAs/transcripts of uncertain coding potential (TU) are highly expressed in goat ovaries in the luteal phase and that this might regulate the synthesis of progesterone.
RNA transcriptome sequencing is now one of the most powerful methods available for the detection and expression analysis of lncRNAs (Mortazavi et al., 2008; Atkinson et al., 2012). In the present study, we explored the lncRNA and coding gene regulatory networks of ovine oocytes from GV to MII stages. Briefly, GV oocytes that were isolated from ewes and MII oocytes matured in vitro were sequenced for the expression of lncRNA. Through RNA data analysis, the stage-specific lncRNAs and coding genes were identified. Furthermore, the co-expression (trans) and co-location (cis) analyses were implemented with differentially expressed lncRNAs (DELs) and genes (DEGs) to explore the regulatory networks involved in oocyte transition from GV to MII stages.
Materials and Methods
Ethics Statement
All the experiments in this research followed national guidelines and were in accordance with the Ethical Committee of Anhui Agricultural University. All surgery on ewes had the approval of the Institution of Animal Care and Use Committee, Anhui Agricultural University (no. 2016017), China.
Ovine Oocyte Collection and Maturation in vitro
Ovine ovaries were collected from a slaughterhouse in Ürümqi in Xinjiang, China. They were then transported in saline solution supplemented with penicillin–streptomycin to the laboratory within 4 h. There, follicles of 2–8 mm in diameter were extracted from the washed ovaries. After settlement for a short time, the supernatant was removed, and the cell pellet was resuspended with washing buffer for subsequent dissection and selection under a stereoscopic microscope. The oocytes were divided into two classes: (i) denuded oocytes (GV stage) and (ii) oocytes surrounded by cumulus cells; the latter were treated with hyaluronidase (Sigma, H-3506) to remove the cumulus cells. Oocytes were then cultured in vitro with maturation medium in a humidified incubator at 38.5°C with 5% CO2 for 23–25 h. MII stage oocytes with an extruded first polar body were harvested. The sequence samples were prepared with 30–35 oocytes as one biological replicate.
As in previous reports (Peng et al., 2008; Su et al., 2014), the maturation medium (per 100 ml) for oocytes was composed of double-distilled water, 10 ml fetal bovine serum (FBS; Gibco, 00642), 0.95 g TCM199 (Gibco, 31100-03510), 0.22 g NaHCO3, 0.2384 g HEPES, 0.022 g Na-Py, 0.0075 g penicillin, 0.005 g streptomycin, and 0.003 g glutamine supplemented with 100 IU FSH, 1 ml 17β-E2 (working concentration is 1 μg/ml), and 200 IU LH. Phenol red was added to adjust the pH value, and the medium was filtered through a 0.22 μm filter and preserved at 4°C.
RNA Extraction, Library Construction, and Sample Sequence
RNA samples from the collected oocytes were extracted with TRIzol reagent, and the following pipeline was performed to ensure RNA quantity: 1% agarose gel electrophoresis for RNA degradation and contamination test, NanoPhotometer® spectrophotometry (IMPLEN, Westlake Village, CA, United States) for detecting RNA purity, Qubit® 2.0 Fluorometer (Life Technologies, Camarillo, CA, United States) for quantifying RNA concentration, and RNA integrity assessment with an RNA Nano 6000 Assay Kit and a Bioanalyzer 2100 system (Agilent Technologies, Santa Clara, CA, United States). An aliquot of 3 μg RNA from each sample was used as the input to prepare the sequence. After removal of ribosomal RNA, the sequence libraries were generated with a NEBNext® UltraTM Directional RNA Library Prep Kit (NEB, Ipswich, MA, United States) according to the manufacturer’s recommendations, the quality of which was assessed with an Agilent Bioanalyzer 2100 system. After sample clustering with a TruSeq PE Cluster Kit v3-cBot-HS (Illumina, San Diego, CA, United States) according to the manufacturer’s instructions; sequencing was conducted on an Illumina HiSeq 2000 platform using a 100 bp paired-end strategy.
Pipeline of Data Processing and Analysis
Firstly, quantity control was performed to remove low-quality and adapter reads from the generated raw data using Trimmomatic software (v 0.38) (Bolger et al., 2014). FastQC (v0.11.8) reports were produced to check the obtained data (Andrews, 2010), which would be used for subsequent analyses. With the STAR software (v2.7.1a)1, clean data were aligned to the sheep reference genome (version Oar_v3.1 from ensemble) to produce Bam files. After Bam files were sorted with SAMtools (v1.9) (Li et al., 2009), transcript assembly was performed with StringTie software (v2.0) (Pertea et al., 2015). Subsequently, all samples were merged to generate one GTF file with a setting of a “-m 200” parameter, by which only transcripts with a length >200 bp were retained for the following lncRNA analyses.
Screening for Candidate lncRNAs
We identified candidate lncRNAs to carry out downstream analyses following previously reported procedures (Gao et al., 2017; Sun et al., 2018; Zhang et al., 2019). According to the assembled transcript, preliminary filtration was executed to select non-genetic transcripts with a “classcode” label annotated by GffCompare software2 (v0.11.2). The produced transcript file may then contain the lncRNA transcripts with protein coding potential that should be removed. Here, we predicted the protein coding ability of lncRNA transcripts through three methods: Coding–Non-coding Index (CNCI) (Sun et al., 2013), Coding Potential Calculator (CPC) (Kang et al., 2017), and PfamScan (Finn et al., 2016). The combined analyses guaranteed the accuracy of the final candidate lncRNAs (Figure 1D, marked with black arrow) in our study.
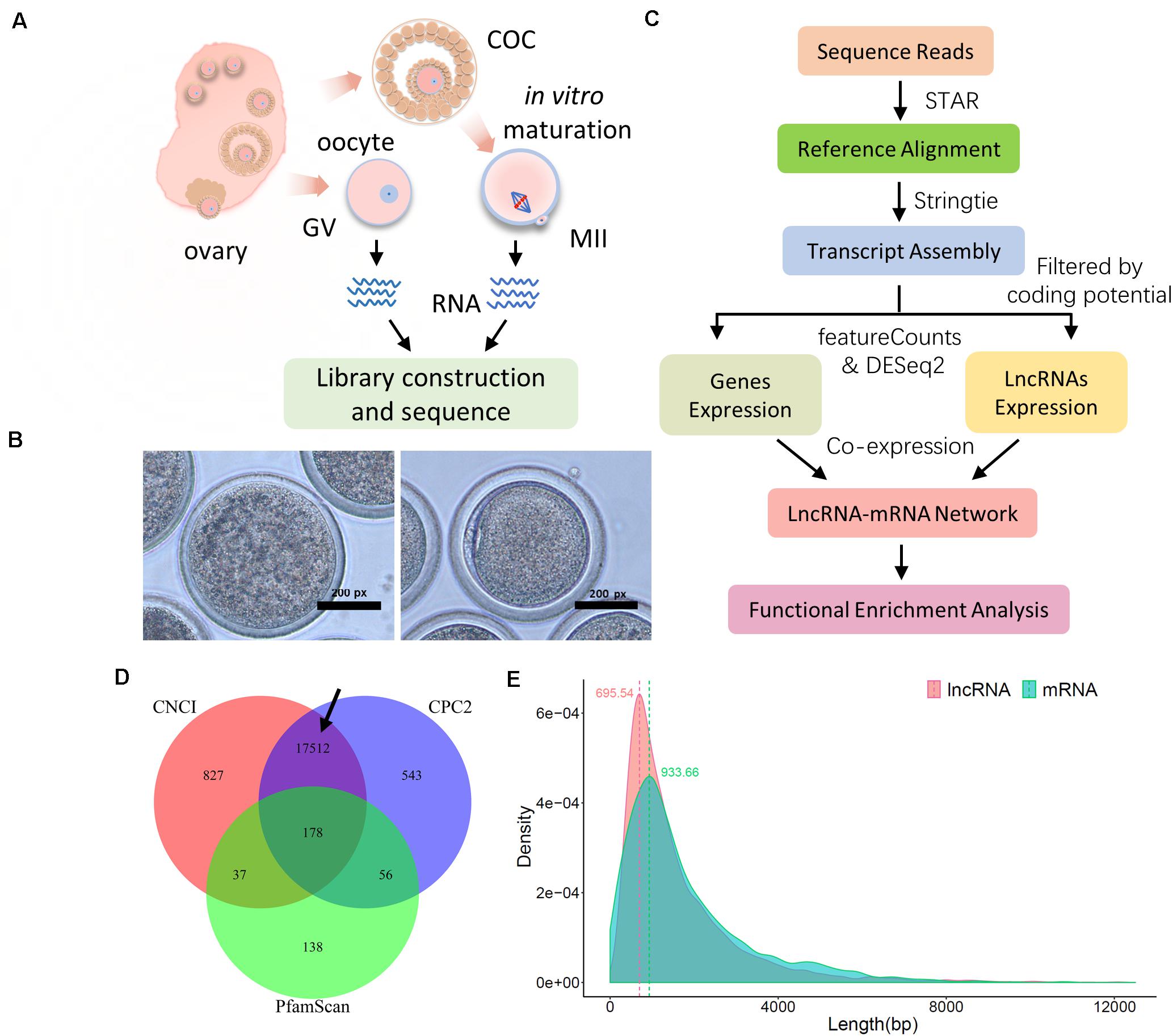
Figure 1. Sequence and data preprocessing of ovine oocytes at the germinal vesicle (GV) and meiosis II (MII) stages. (A) Schematic diagram of sample collection and RNA sequencing procedure. (B) Representative bright-field images of ovine GV (left) and MII (right) oocytes. (C) Data preprocessing pipeline for mRNA and lncRNA. (D) Candidate lncRNA identification with coding potential assessment of CNCI, CPC2, and PfamScan; the black arrow refers to the final candidate lncRNA. (E) Distribution of transcript length of lncRNA and mRNA; the transcript length of lncRNA and mRNA was generated using the assembly process of StringTie software, and density distribution was assembled using the ggplot2 package in the R environment.
Expression Analysis of mRNA and lncRNA
The expression levels of mRNA and lncRNA were calculated using featureCounts (v1.6.4) (Liao et al., 2013) based on assembled transcript files. Then, the DESeq2 package (Love et al., 2014) was used to normalize expression levels and perform differentially expressed analyses for mRNAs and lncRNAs using R (v 3.6.0). In our research, p-values of the transcripts were adjusted using Benjamini and Hochberg’s approach for controlling the false discovery rate (FDR). Significant DEGs and DELs were determined with padj (adjusted p-value) <0.05.
Co-expression and Co-location Analysis
It is thought that lncRNAs play a trans-role through their related co-expression genes (Yan et al., 2017). In our study, the pairwise significant DEGs and DELs were estimated with Pearson’s correlation coefficient (r) using the R package Hmisc (v4.3)3. Those mRNAs with a p-value < 0.01 and |r-value| > 0.9 were considered as co-expressed genes of their lncRNAs. Further, for cis-regulation, based on the assembled GTF file of candidate lncRNAs, we employed FEELnc (v 0.1.1) (Wucher et al., 2017) to screen the coding genes that were located within 100 kb upstream and downstream of lncRNAs (that is, co-location), and the cis-regulated genes of their lncRNAs were decided with the parameter of “isBest = 1.” According to the illustration of FEELnc software, the lncRNA subtypes were divided into two classes: “Genic” and “Intergenic.” “Genic” included subtypes of “overlapping,” “containing,” and “nested”; “Intergenic” consisted of “same strand,” “convergent,” and “divergent” subtypes. Meanwhile, the core regulatory network was determined with common paired mRNA–lncRNA for co-expression and co-location modules.
Functional Enrichment Analysis
Gene Ontology (GO) and Kyoto Encyclopedia of Genes and Genomes (KEGG) enrichment analyses were performed predominantly based on Metascape4 (Zhou et al., 2019), a web-based portal that operates using gene symbols as inputs and integrates a board of current biological databases. In the output results, it highlights the key and non-redundant functional terms, also the enrichment network displays intra- and inter-cluster connection of terms. Due to the limitations of Metascape, large data sets (>3.000 genes) were processed using the clusterProfiler package (Yu et al., 2012) for enrichment analysis; the top 20 most enriched terms are displayed in order according to “GeneRatio,” and the “p.adjust” value was produced using the “BH” method in the parameter of “pAdjustMethod” (Figure 2). Given the inadequate database of functional analysis in sheep, the ovine gene symbols were converted into murine homologous gene symbol IDs through the g:profile website5 (Raudvere et al., 2019).
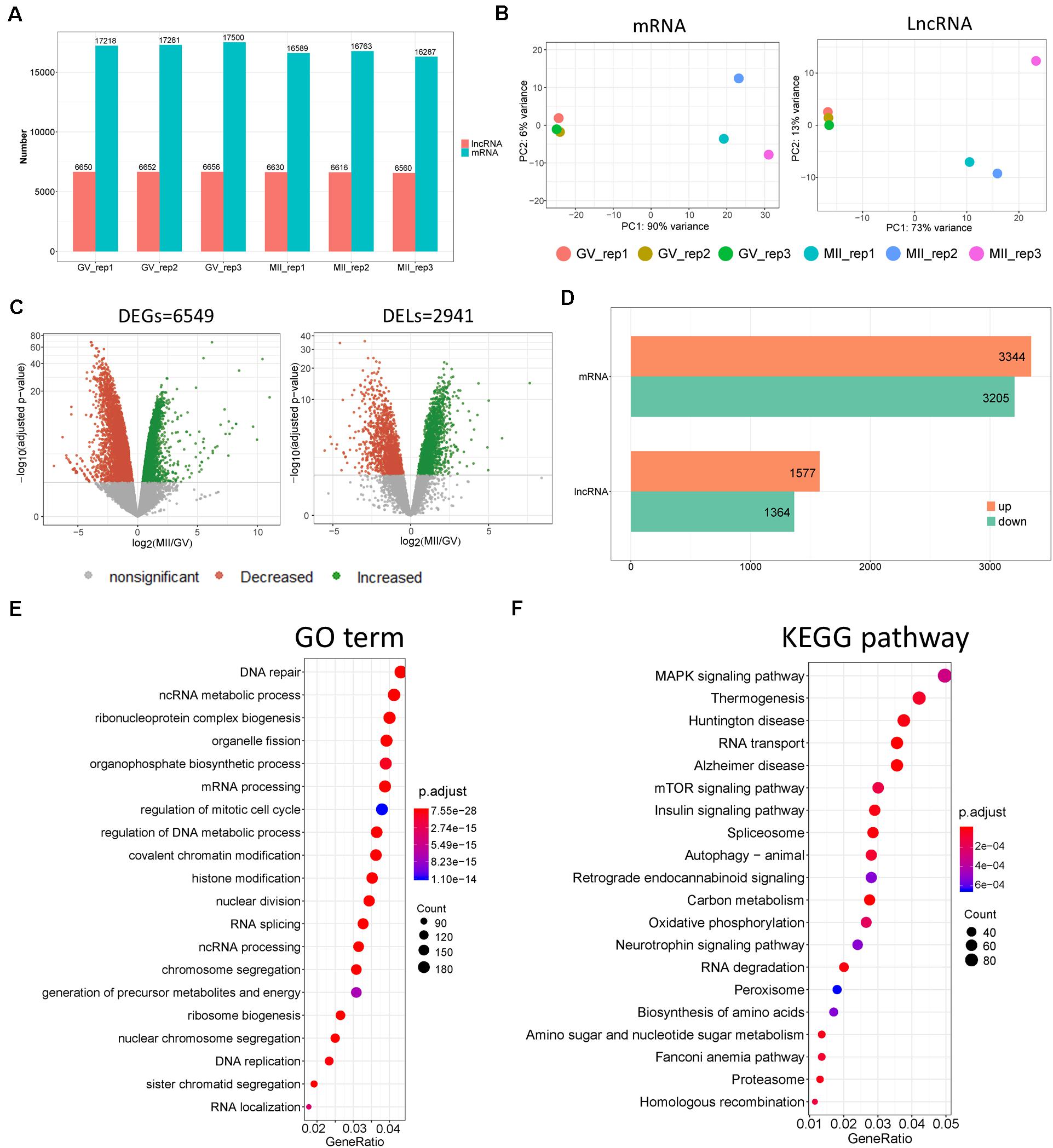
Figure 2. Divergent expression patterns of lncRNA and mRNA in ovine oocytes. (A) Number of lncRNA and mRNA transcripts with a non-zero count in each sample. (B) Principal component analysis (PCA) based on lncRNA and mRNA. (C) Volcano plots of differentially expressed genes (DEGs) and lncRNAs (DELs) of MII vs. GV stages. (D) Number of upregulated and downregulated DEGs and DELs of MII vs. GV stages. (E) GO and (F) KEGG enrichment of DEGs with top 20 terms; “Count” is the number of DEGs that were enriched in the term; “GeneRatio” refers to the ratio of DEG numbers that were enriched in the term vs. all DEG number.
Results
Sequence and Data Preprocessing of Ovine Oocytes at the GV and MII Stages
In the present study, denuded oocytes (GV stage) and COCs were isolated from ovine ovaries. Cumulus cells were removed from COCs and the isolated oocytes developed to the mature MII phase during a 23–25 h incubation in vitro. These harvested oocytes were gathered in two groups, “GV” and “MII,” for RNA sequencing (Figure 1A). Representative images of GV and MII ovine oocytes are shown in Figure 1B. To determine the molecular mechanism of lncRNA and related target genes, with quality control and high mapping rates (around 90%; Supplementary Figure S1A), the clean data were processed to identify candidate lncRNAs and coding gene transcripts according to standard pipelines (Figure 1C). In particular, candidate lncRNAs were generated after protein coding potential assessment with CNCI, CPC2, and PfamScan (Figure 1D with a black arrow). Ultimately, we obtained transcript results of lncRNAs and mRNAs with density peaks of 695.54 and 933.66 bp in length, respectively (Figure 1E), which were used for downstream analyses.
Divergent Expression Patterns of lncRNA and mRNA
According to data processing, more transcripts of coding genes were generated than lncRNAs (Figure 2A). To understand the temporal expression patterns of lncRNA and mRNA in ovine oocyte samples, principal component analysis (PCA) was conducted (Figure 2B); it showed differing expression patterns of lncRNAs and mRNAs in GV and MII oocytes, which suggested that the mRNAs and lncRNAs displayed a temporal expression pattern in ovine oocytes dependent on the stage of development. Furthermore, volcano plots demonstrated there were 2.941 DELs (Supplementary Table S1) and 6.549 DEGs (Supplementary Table S2 and Figure 2C) of MII vs. GV stage. Moreover, the number of upregulated lncRNAs and mRNAs were both slightly more than that of downregulated ones (Figure 2D). In addition, the top 40 DELs (Supplementary Figure S1B) and DEGs (Supplementary Figure S1C) were made into a heatmap. In order to explore the biological processes of DEGs, functional enrichment was performed (Figure 2E). GO results showed that the top enriched terms were DNA repair, ncRNA metabolic processes, and ribonucleoprotein complex biogenesis; others were also closely related to cellular transcriptional states, such as mRNA processing, mitotic cell cycle, and chromatin and histone modification. Moreover, KEGG revealed that mitogen-activated protein kinase (MAPK), mammalian target of rapamycin (mTOR), and the insulin signaling pathways were likely involved in the regulation of oocyte fate during transition from GV to MII (Figure 2F).
Co-expression Analysis of DELs and DEGs in Ovine Oocytes
To precisely identify the regulatory mechanisms of lncRNAs and mRNAs, co-expression analysis was executed based on DEGs and DELs as previously reported (Liu et al., 2017; Zhang et al., 2019). After filtration based on p-value (p < 0.01, 16.52%) and Pearson’s correlation coefficient (|r-value| > 0.9), 173.268 pairwise lncRNA–mRNAs were produced (Supplementary Table S3), which uniquely included 357 DEGs and 2.935 DELs (Figure 3A); among these DEGs, more genes were downregulated at the MII stage (Supplementary Figure S2A). Further, GO enrichment analysis was applied using the co-expressed DEGs, and several significant GO terms were mostly connected with reproductive processes including calcium ion transport, stress-activated MAPK cascade, cellular response to cAMP, insulin secretion, and phosphatidylinositol 3-kinase signaling (PI3K; Figure 3B), despite the top terms appearing to be unrelated (Supplementary Figure S2B). In addition, the expression levels of genes enriched in the five reproductive terms are shown in the heatmap in Figure 3C, which were under the control of numerous lncRNAs (Supplementary Table S4).
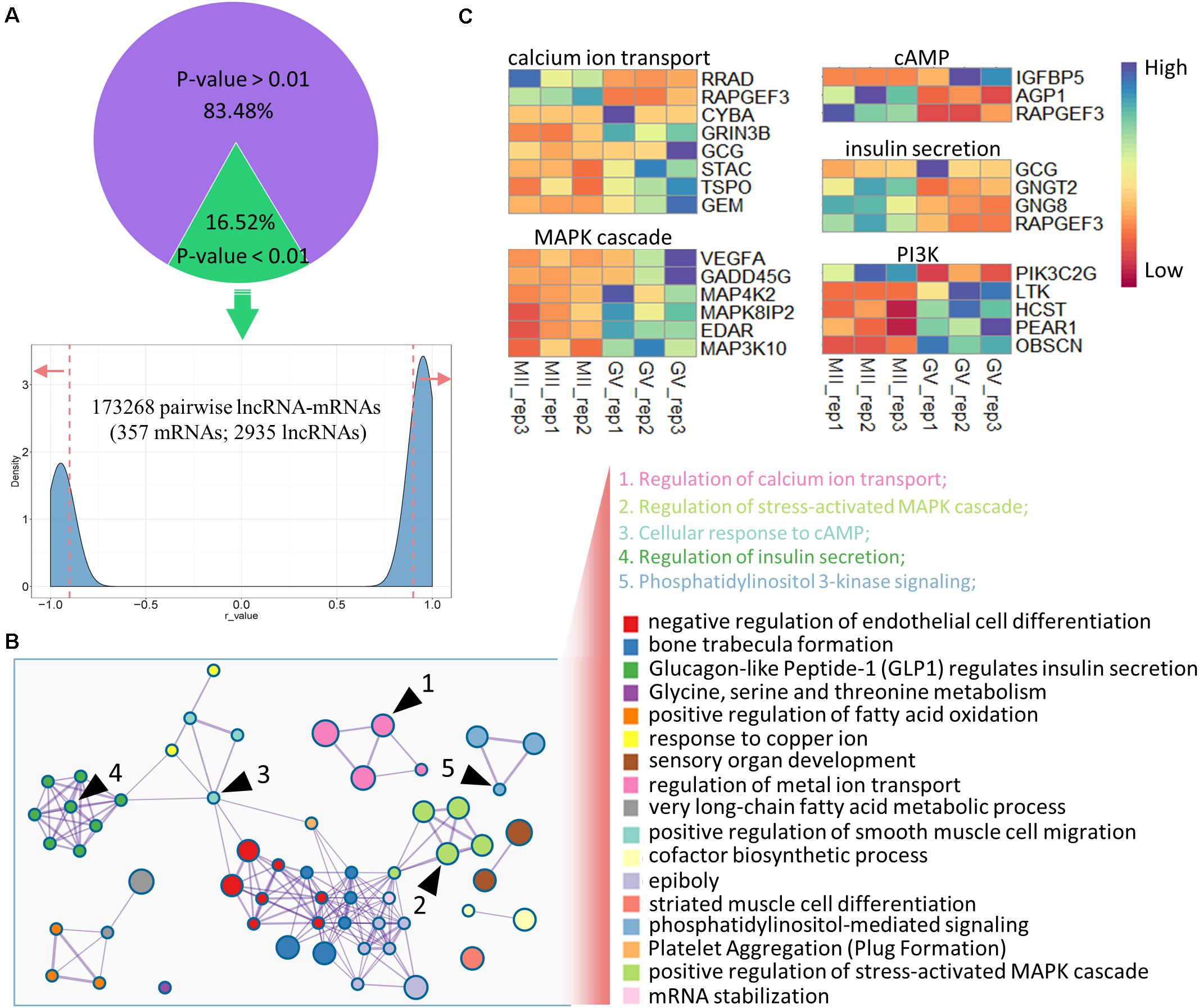
Figure 3. Co-expression analysis of DELs and DEGs in ovine oocytes. (A) Identification of pairwise lncRNA–mRNA in co-expression analysis; the dashed lines mark the r-value (0.9 and –0.9), and red arrows refer to the selected regions with r-values > 0.9 and <–0.9. (B) Functional enrichment analysis of co-expressed genes; similar clusters are annotated with the same color; node size represents gene numbers in term; a line of inter-clusters indicates a common gene; the five reproductive terms are marked with black arrows and labeled with numbers. (C) Heatmap of the expressions of genes enriched in five reproductive terms.
Cis-Regulation of lncRNAs With Target Genes
lncRNAs also export a potential cis-regulatory capacity to their adjacent coding genes (Wang and Chang, 2011; Yan et al., 2017); therefore, FEELnc software was used to detect coding genes adjacent to candidate lncRNAs. We obtained 14.821 pairs of cis-regulatory lncRNA genes with the best match (Supplementary Table S5), which included 5.908 unique lncRNAs. In particular, 2.591 lncRNAs (key cis-lncRNAs) were common with co-expressed lncRNAs (Figure 4A), and almost half of them were increased (Supplementary Figure S3A). According to statistical analysis of the subtype, most key cis-lncRNAs occupied the same strand as coding genes (Figure 4B), and results of genome location showed that over one third of key cis-lncRNAs were located either downstream or upstream of the target gene (Figure 4C). The analysis also showed that there were 2.039 cis-genes under the control of these cis-lncRNAs. Functional enrichment suggested they gathered in cell projection organization, developmental growth, and anatomical structure size (Figure 4D and Supplementary Figure S3B). Moreover, the most enriched pathways were insulin secretion, estrogen, and cAMP signaling (Figure 5A), which are all involved in reproductive processes. Among them, the cis-regulatory lncRNA–mRNA networks of estrogen (Supplementary Table S6) and cAMP signaling (Supplementary Table S7) were highlighted (Figure 5B).
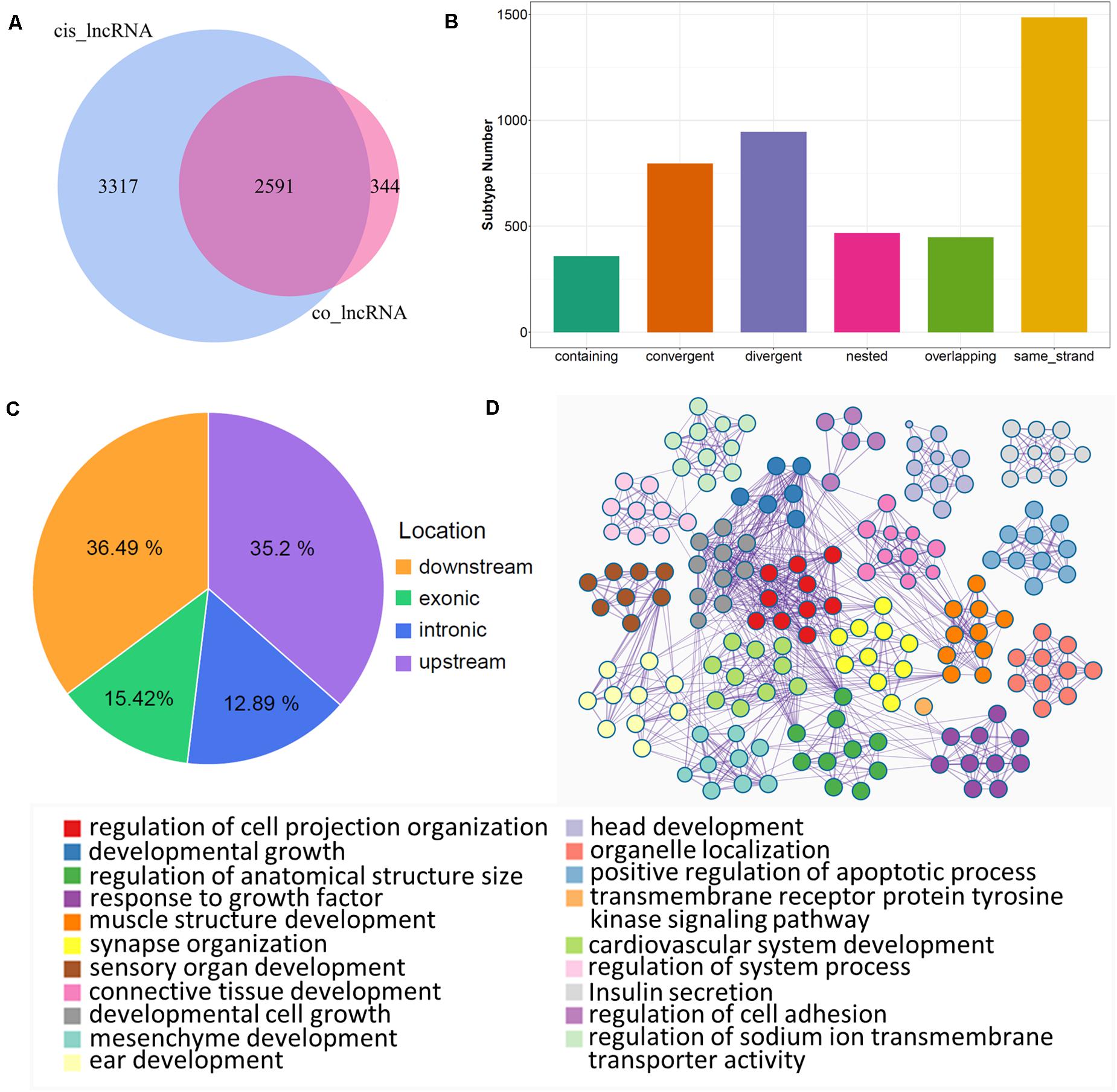
Figure 4. Cis-regulation of lncRNAs with target genes. (A) Venn plot of unique lncRNAs in cis-regulation with co-expressed lncRNAs; common region references to key cis-lncRNAs. (B) Subtype statistics of key cis-regulatory lncRNAs. According to the illustration, “Genic” includes the subtypes of “overlapping,” “containing,” and “nested”; “Intergenic” consists of “same strand,” “convergent,” and “divergent” subtypes. (C) Genome location statistics of key cis-regulatory lncRNAs. (D) Functional enrichment analysis of cis-genes under control of key cis-lncRNAs; similar clusters are annotated with the same color, node size represents gene number in term, and a line of inter-clusters indicates a common gene.
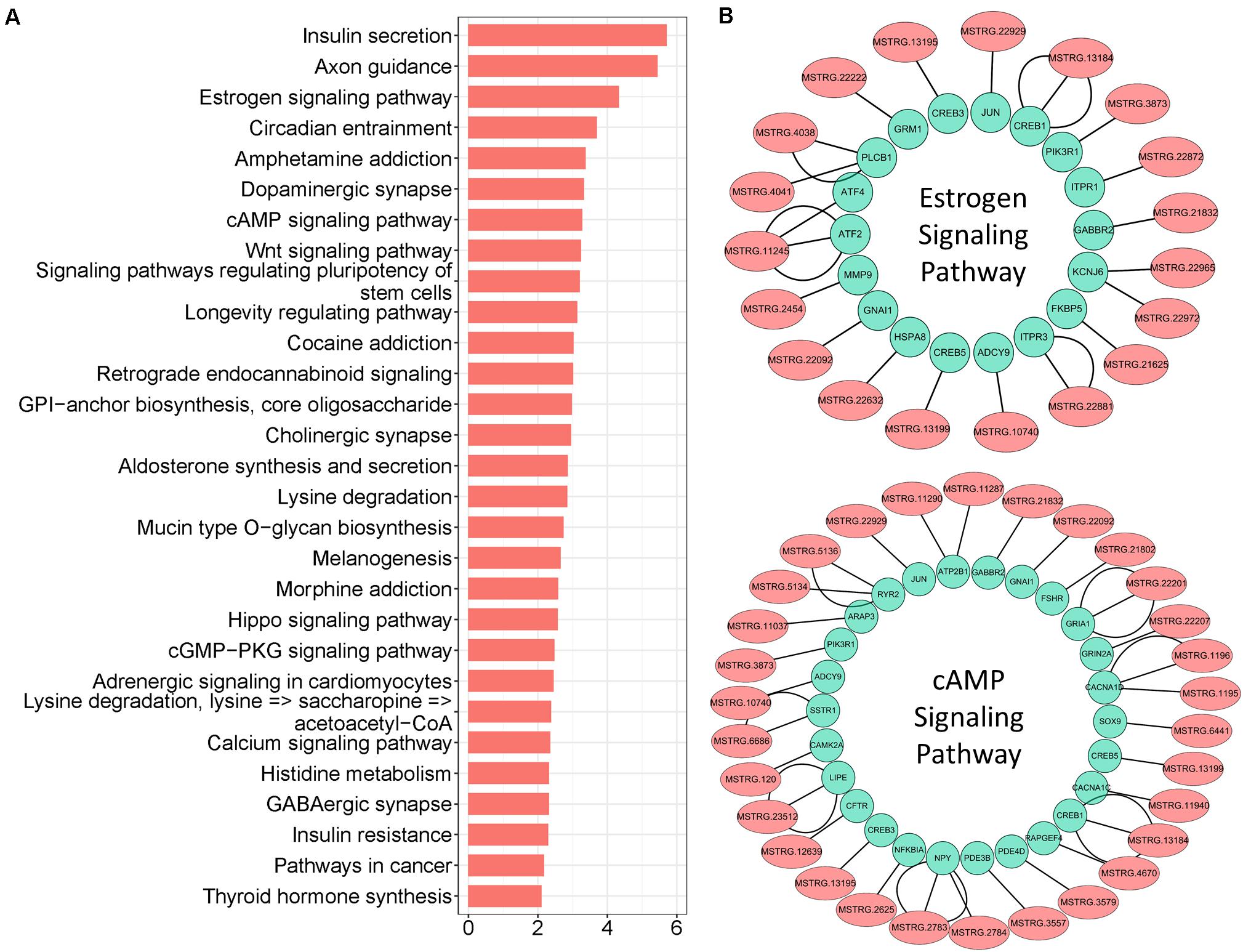
Figure 5. Pathway enrichment analysis of key cis-lncRNA targeted genes. (A) Pathway enrichment of key cis-lncRNA targeted genes. The x-axis text represent the value of “-Log(p-value)”. (B) The cis-regulatory networks of lncRNA–mRNA for estrogen and cAMP signaling; lncRNAs are marked with light coral nodes and targeted genes with light green; the inter-line represents targeted relationships.
Identification of Core lncRNA–mRNA Network
In addition, core networks were further identified, derived from common terms of trans- and cis-regulation. In our results, 23 cis-genes overlapped with co-expressed genes (Figure 6A), among which six paired lncRNA–mRNA were both detected by the two methods (Figure 6B). Of note, only one lncRNA was upregulated (MSTRG.1318); others were all significantly downregulated during the GV-to-MII transition (Figure 6C). The target genes controlled by these core lncRNAs (Supplementary Table S8) mostly were enriched in epiboly and PI3K signaling, while more genes were associated with monovalent inorganic cation transport and transport of small molecules (Figure 6D). Among them, MSTRG.17927 was a candidate for tracking; there were 69 genes (which had annotated gene symbols) under its control (Figure 6E and Supplementary Table S9), which were significantly gathered in PI3K signaling (Figure 6F and Supplementary Figure S3C); among them, 12 genes increased (Figure 6G), and 57 were downregulated (Supplementary Figure S3D) during the GV-to-MII transition.
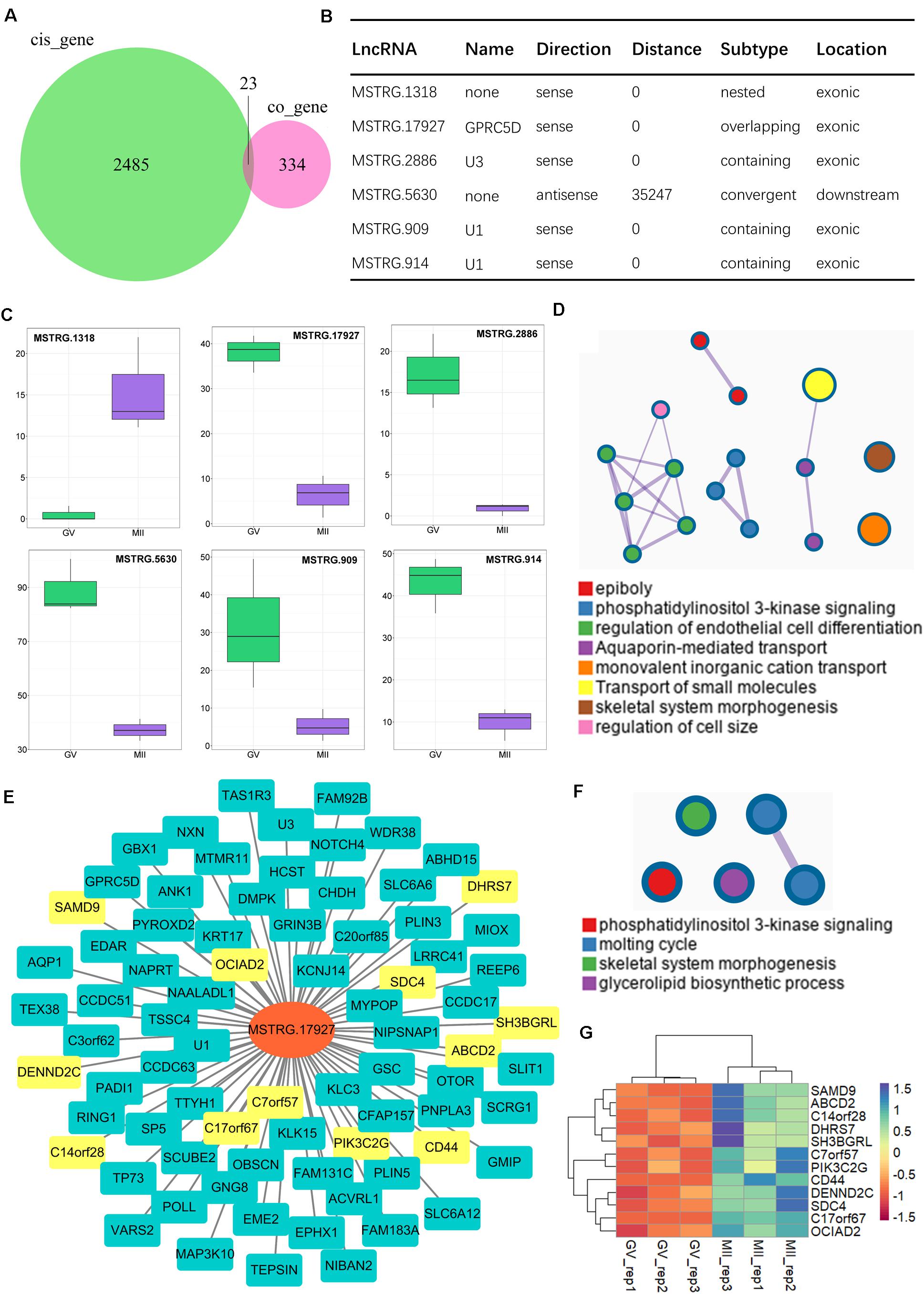
Figure 6. Identification of core lncRNA–mRNA networks for the maturation of ovine oocytes. (A) Venn plot of cis-regulation with co-expressed genes; common regions refer to candidate core genes. (B) Candidate core lncRNA–mRNA network. (C) Expression of core lncRNAs from GV to MII stages. (D) Enrichment analysis of genes targeted by core lncRNAs. (E) Core lncRNA–mRNA network of targeted genes and MSTEG 17927; the yellow symbol marks negative regulatory genes, and the cyan marks positive regulatory genes. (F) Functional enrichment analysis of targeted genes of MSTEG 17927. (G) Expression of negative regulatory genes targeted by MSTEG 17927.
Discussion
Long non-coding RNAs are associated with many reproductive processes in animals (Golicz et al., 2018), including sex determination, spermatogenesis, and genomic imprinting (Gabory et al., 2009; Zhang L. et al., 2010; Arun et al., 2012). However, their roles in ovarian development are poorly understood. In the present study, genome-wide candidate lncRNAs in GV and MII stage oocytes were identified; and trans- and cis-regulation of lncRNAs were inferred with their targeted coding genes based on DELs and DEGs. Moreover, lncRNA–mRNA networks were assumed to regulate key signaling pathways regarding oocyte meiotic maturation. It is important to note that the mechanisms involved in the in vitro maturation of oocytes are potentially different from those occurring in vivo. Nevertheless, the current study provides a guide to the mechanisms involved in oocyte maturation that are regulated by lncRNA.
As reported, the potential coding capability is an important index, which can be used to distinguish protein-coding genes from non-coding transcripts (Sun et al., 2013; Zhao et al., 2018). In our study, 17.512 candidate lncRNAs were identified using strict screening conditions, and only around 6.600 lncRNAs were expressed in at least one sample. It was found that the length of lncRNA transcripts was shorter than that of coding genes, consistent with many previous reports (Ran et al., 2016; Liu et al., 2018). Furthermore, the expression of lncRNA usually exerts tissue- and stage-specific patterns in animals (Liu et al., 2017; Yan et al., 2017). Thus, the abundance profiles identified here were assumed to be candidate oocyte-specific lncRNAs. Among these, we detected 2.941 lncRNAs that were differently expressed between GV and MII stage (Figure 2C); we suggest that these lncRNAs were possibly related to the specificity of the developmental stage of the ovine oocytes.
Currently, the potential functions of lncRNAs are investigated through their target genes by using trans- and cis- regulatory methods as previously described (Gao et al., 2017; Zhang et al., 2019). In this study, we demonstrated that the co-expressed genes (trans-regulation) might be involved in the functional regulation of calcium ion transport and cAMP response. It has been well demonstrated that calcium in many species is involved in oocyte meiotic maturation (Tosti, 2006; Miao and Williams, 2012). In addition, cAMP is responsible for oocyte meiotic arrest and resumption (Norris et al., 2009; Cao et al., 2018). In this study, RAPGEF3, also termed EPAC1, was identified as an important targeted gene of lncRNAs (Supplementary Table S4). Interestingly, RAPGEF3 expression distinctly decreased from GV to MII stage, and the gene has been shown to serve as a cAMP-stimulated GDP exchange factor, whose mutation is known to increase the affinity for cAMP by around 2.5-fold (Dao et al., 2006). Moreover, GO enrichment analysis revealed that several co-expressed genes were enriched in MAPK and PI3K signaling, both of which are known to participate in oocyte meiotic resumption and maturation (Schmitt and Nebreda, 2002; Liang et al., 2007). In addition, lncRNA has previously been reported to be involved in cAMP and MAPK signaling pathways (Sulayman et al., 2019; Wang, 2019). Thus, we suggested that many lncRNAs might participate in oocyte maturation via trans-regulation.
For cis-regulation, the coding genes within 100 kb upstream and downstream of lncRNAs were predicted by FEELnc software. Our results indicated that key cis-lncRNAs mostly were enriched at the subtypes of “same strand” and “divergents”. The majority of cis-lncRNAs are “intergenic” lncRNAs in our study; accordingly, it suggests the intergenic lncRNAs are likely related to modified chromatin as seen in previous reports (Khalil et al., 2009; Ransohoff et al., 2018), which implies that the cis-lncRNA is potentially related to meiotic maturation via chromatin modification. The cis-regulatory effects of lncRNAs on neighboring genes have been well examined (Sun and Kraus, 2013; Yan et al., 2017). Here, with the enrichment of cis-regulated genes, we uncovered the cis-regulatory pathway of lncRNAs; more specifically, two lncRNA–mRNA networks were highlighted (estrogen and cAMP signaling). Furthermore, several cis-regulatory pathways of key lncRNAs were identified, which included insulin secretion, Wnt, Hippo, cGMP-PKG, and calcium signaling (Figure 5A). These results indicated that cis-regulatory lncRNAs likely account for a broad range of biological processes in oocytes during the GV-to-MII stage transition. Importantly, the core lncRNA–mRNA networks were identified by overlapping of the trans- and cis-methods. It is a remarkable point that the lncRNA MSTRG.17927, which declined significantly in MII stage oocytes, is thought to be involved in oocyte maturation via PI3K signaling. Matten et al. (1996) reported that the activation of a MAPK cascade is related to MPF activation, and PI3K has been suggested to be essential for insulin-induced oocyte meiotic resumption (Bagowski et al., 2001; Liang et al., 2007). Interestingly, the co-expressed genes in this study were mostly enriched in insulin secretion. It is also known that preovulatory follicles have an abundance of insulin compared with subordinate follicles in cattle (Landau et al., 2000). Insulin exposure affects meiotic chromatin during oocyte maturation and meiotic progression in mice (Acevedo et al., 2007; Das and Arur, 2017), indicating that insulin plays a role in oocyte maturation. However, lncRNA-mediated insulin secretion in oocyte maturation needs further investigation.
In summary, RNA sequencing was performed with isolated GV and in vitro matured MII stage oocytes. Through prediction analysis, genome-wide candidate lncRNAs that were stage-specific were identified, and their trans- and cis-regulatory functions were investigated. As a result, we suggested that multiple lncRNA–mRNA networks may be involved in key signaling pathways throughout oocyte differentiation during the GV-to-MII stage transition. In particular, MSTRG.17927 was shown to mediate the regulation of PI3K signaling. This research provides novel bioinformatic insights into the role of lncRNA in the molecular mechanisms underlying oocyte development.
Data Availability Statement
The datasets presented in this study can be found in online repositories. The names of the repository/repositories and accession number(s) can be found below: https://www.ncbi.nlm.nih.gov/, GSE148022.
Ethics Statement
The animal study was reviewed and approved by the Institution of Animal Care and Use Committee, Anhui Agricultural University, China.
Author Contributions
J-JW, M-HN, and TZ conducted the experiments. J-JW analyzed the data. J-JW, H-GC, and WS wrote the manuscript. H-GC and WS designed the experiments. All authors reviewed the manuscript.
Funding
This work was supported by the National Natural Science Foundation of China (31372309), the National Key Research and Development Program of China (2018YFD0502001), the Opening Project of the State Key Laboratory of Reproductive Regulation and Breeding of Grassland Livestock (30500-518390204), and the Taishan Scholar Construction Foundation of Shandong Province of China (ts20190946).
Conflict of Interest
The authors declare that the research was conducted in the absence of any commercial or financial relationships that could be construed as a potential conflict of interest.
Acknowledgments
The authors would like to thank Dr. Paul Dyce at Auburn University for his careful review of the manuscript.
Supplementary Material
The Supplementary Material for this article can be found online at: https://www.frontiersin.org/articles/10.3389/fphys.2020.01019/full#supplementary-material
FIGURE S1 | Statistical data of RNA sequence and expression of differentially expressed genes (DEGs) and differentially expressed lncRNAs (DELs). (A) Table of quality control and mapping rate statistics of RNA sequences; Heatmap of top 40 DELs (B) and DEGs (C).
FIGURE S2 | Co-expression analysis of DELs and DEGs. (A) Heatmap of unique co-expressed DEGs; (B) GO enrichment analysis of unique co-expressed DEGs.
FIGURE S3 | Cis-regulatory analysis of DELs and DEGs. (A) Heatmap of genes targeted by core cis-lncRNAs; (B) Functional enrichment of genes targeted by core cis-lncRNA; (C) Functional enrichment of targeted genes of MSTEG 17927; (D) Expression of positive regulatory genes targeted by MSTEG 17927.
TABLE S1 | Results of differential expression analysis of lncRNAs.
TABLE S2 | Results of differential expression analysis of mRNAs.
TABLE S3 | Results of co-expression analysis.
TABLE S4 | Co-expressed gene pairs of reproduction related GO terms.
TABLE S5 | Classes of lncRNAs for co-location.
TABLE S6 | Estrogen cis-gene pathway.
TABLE S7 | cAMP cis-gene pathway.
TABLE S8 | Candidate core lncRNA-mRNA networks.
TABLE S9 | Target genes of MSTRG.17927.
Footnotes
- ^ https://github.com/alexdobin/STAR
- ^ https://github.com/gpertea/gffcompare
- ^ https://github.com/harrelfe/Hmisc
- ^ http://metascape.org/gp/index.html
- ^ https://biit.cs.ut.ee/gprofiler/convert
References
Acevedo, N., Ding, J., and Smith, G. D. (2007). Insulin signaling in mouse oocytes. Biol. Reproduct. 77, 872–879. doi: 10.1095/biolreprod.107.060152
Andrews, S. (2010). FastQC: A Quality Control Tool for High Throughput Sequence Data. Cambridge, UK: Babraham Institute. Available online at: http://www.bioinformatics.babraham.ac.uk/projects/fastqc
Arun, G., Akhade, V. S., Donakonda, S., and Rao, M. R. S. (2012). mrhl RNA, a long noncoding RNA, negatively regulates Wnt signaling through its protein partner Ddx5/p68 in mouse spermatogonial cells. Mol. Cell. Biol. 32, 3140–3152. doi: 10.1128/mcb.00006-12
Atkinson, S. R., Marguerat, S., and Bähler, J. (2012). “Exploring long non-(coding)RNAs through sequencing,” in Seminars in Cell & Developmental Biology, Ed. J. Davey (Amsterdam: Elsevier), 200–205. doi: 10.1016/j.semcdb.2011.12.003
Bagowski, C. P., Myers, J. W., and Ferrell, J. E. (2001). The classical progesterone receptor associates with p42 MAPK and is involved in phosphatidylinositol 3-kinase signaling inXenopus oocytes. J. Biol. Chem. 276, 37708–37714. doi: 10.1074/jbc.m104582200
Battaglia, R., Vento, M. E., Borzì, P., Ragusa, M., Barbagallo, D., Arena, D., et al. (2017). Non-coding RNAs in the ovarian follicle. Front. Genet. 8:57. doi: 10.3389/fgene.2017.00057
Bolger, A. M., Lohse, M., and Usadel, B. (2014). Trimmomatic: a flexible trimmer for Illumina sequence data. Bioinformatics 30, 2114–2120. doi: 10.1093/bioinformatics/btu170
Bornslaeger, E. A., Mattei, P., and Schultz, R. M. (1986). Involvement of cAMP-dependent protein kinase and protein phosphorylation in regulation of mouse oocyte maturation. Dev. Biol. 114, 453–462. doi: 10.1016/0012-1606(86)90209-5
Brockdorff, N., Ashworth, A., Kay, G. F., Cooper, P., Smith, S., Mccabe, V. M., et al. (1991). Conservation of position and exclusive expression of mouse Xist from the inactive X chromosome. Nature 351, 329. doi: 10.1038/351329a0
Cao, H., Bian, Y., Zhang, F., Tang, Y., Li, C., Chen, J., et al. (2018). Functional role of Forskolin and PD166285 in the development of denuded mouse oocytes. Asian Aust. J. Anim. Sci. 31, 344–353. doi: 10.5713/ajas.17.0441
Carninci, P., Kasukawa, T., Katayama, S., Gough, J., Frith, M., Maeda, N., et al. (2005). The transcriptional landscape of the mammalian genome. Science 309, 1559–1563. doi: 10.1126/science.1112014
Cho, W. K., Stern, S., and Biggers, J. D. (1974). Inhibitory effect of dibutyryl cAMP on mouse oocyte maturation in vitro. J. Exp. Zool. 187, 383–386. doi: 10.1002/jez.1401870307
Conti, M., Andersen, C. B., Richard, F., Mehats, C., Chun, S.-Y., Horner, K., et al. (2002). Role of cyclic nucleotide signaling in oocyte maturation. Mol. Cell. Endocrinol. 187, 153–159. doi: 10.1016/s0303-7207(01)00686-4
Conti, M., Hsieh, M., Zamah, A. M., and Oh, J. S. (2012). Novel signaling mechanisms in the ovary during oocyte maturation and ovulation. Mol. Cell. Endocrinol. 356, 65–73. doi: 10.1016/j.mce.2011.11.002
Dao, K. K., Teigen, K., Kopperud, R., Hodneland, E., Schwede, F., Christensen, A. E., et al. (2006). Epac1 and cAMP-dependent protein kinase holoenzyme have similar cAMP affinity, but their cAMP domains have distinct structural features and cyclic nucleotide recognition. J. Biol. Chem. 281, 21500–21511. doi: 10.1074/jbc.m603116200
Das, D., and Arur, S. (2017). Conserved insulin signaling in the regulation of oocyte growth, development, and maturation. Mol. Reproduct. Dev. 84, 444–459. doi: 10.1002/mrd.22806
Fatica, A., and Bozzoni, I. (2014). Long non-coding RNAs: new players in cell differentiation and development. Nat. Rev. Genet. 15, 7–21. doi: 10.1038/nrg3606
Finn, R. D., Coggill, P., Eberhardt, R. Y., Eddy, S. R., Mistry, J., Mitchell, A. L., et al. (2016). The Pfam protein families database: towards a more sustainable future. Nucleic Acids Res. 44, D279–D285.
Gabory, A., Ripoche, M.-A., Le Digarcher, A., Watrin, F., Ziyyat, A., Forné, T., et al. (2009). H19 acts as a trans regulator of the imprinted gene network controlling growth in mice. Development 136, 3413–3421. doi: 10.1242/dev.036061
Gao, X., Ye, J., Yang, C., Zhang, K., Li, X., Luo, L., et al. (2017). Screening and evaluating of long noncoding RNAs in the puberty of goats. BMC Genom. 18:164. doi: 10.1186/s12864-017-3578-9
Golicz, A. A., Bhalla, P. L., and Singh, M. B. (2018). lncRNAs in plant and animal sexual reproduction. Trends Plant Sci. 23, 195–205. doi: 10.1016/j.tplants.2017.12.009
Jones, K. T. (2004). Turning it on and off: M-phase promoting factor during meiotic maturation and fertilization. MHR Basic Sci. Reproduct. Med. 10, 1–5. doi: 10.1093/molehr/gah009
Kang, Y.-J., Yang, D.-C., Kong, L., Hou, M., Meng, Y.-Q., Wei, L., et al. (2017). CPC2: a fast and accurate coding potential calculator based on sequence intrinsic features. Nucleic Acids Res. 45, W12–W16.
Khalil, A. M., Guttman, M., Huarte, M., Garber, M., Raj, A., Morales, D. R., et al. (2009). Many human large intergenic noncoding RNAs associate with chromatin-modifying complexes and affect gene expression. Proc. Natl. Acad. Sci. 106, 11667–11672. doi: 10.1073/pnas.0904715106
Kim, E.-D., and Sung, S. (2012). Long noncoding RNA: unveiling hidden layer of gene regulatory networks. Trends Plant Sci. 17, 16–21. doi: 10.1016/j.tplants.2011.10.008
Landau, S., Braw-Tal, R., Kaim, M., Bor, A., and Bruckental, I. (2000). Preovulatory follicular status and diet affect the insulin and glucose content of follicles in high-yielding dairy cows. Anim. Reproduct. Sci. 64, 181–197. doi: 10.1016/s0378-4320(00)00212-8
Li, H., Handsaker, B., Wysoker, A., Fennell, T., Ruan, J., Homer, N., et al. (2009). The sequence alignment/map format and SAMtools. Bioinformatics 25, 2078–2079. doi: 10.1093/bioinformatics/btp352
Liang, C.-G., Su, Y.-Q., Fan, H.-Y., Schatten, H., and Sun, Q.-Y. (2007). Mechanisms regulating oocyte meiotic resumption: roles of mitogen-activated protein kinase. Mol. Endocrinol. 21, 2037–2055. doi: 10.1210/me.2006-0408
Liao, Y., Smyth, G. K., and Shi, W. (2013). featureCounts: an efficient general purpose program for assigning sequence reads to genomic features. Bioinformatics 30, 923–930. doi: 10.1093/bioinformatics/btt656
Liu, S., Wang, Z., Chen, D., Zhang, B., Tian, R.-R., Wu, J., et al. (2017). Annotation and cluster analysis of spatiotemporal-and sex-related lncRNA expression in rhesus macaque brain. Genome Res. 27, 1608–1620. doi: 10.1101/gr.217463.116
Liu, Y., Qi, B., Xie, J., Wu, X., Ling, Y., Cao, X., et al. (2018). Filtered reproductive long non-coding RNAs by genome-wide analyses of goat ovary at different estrus periods. BMC Genom. 19:866. doi: 10.1186/s12864-018-5268-7
Loewer, S., Cabili, M. N., Guttman, M., Loh, Y.-H., Thomas, K., Park, I. H., et al. (2010). Large intergenic non-coding RNA-RoR modulates reprogramming of human induced pluripotent stem cells. Nat. Genet. 42:1113. doi: 10.1038/ng.710
Love, M. I., Huber, W., and Anders, S. (2014). Moderated estimation of fold change and dispersion for RNA-seq data with DESeq2. Genome Biol. 15:550.
Matten, W., Copeland, T., Ahn, N., and Woude, G. V. (1996). Positive Feedback between MAP Kinase and Mos duringXenopusOocyte Maturation. Dev. Biol. 179, 485–492. doi: 10.1006/dbio.1996.0277
Miao, Y. L., and Williams, C. J. (2012). Calcium signaling in mammalian egg activation and embryo development: the influence of subcellular localization. Mol. Reproduct. Dev. 79, 742–756. doi: 10.1002/mrd.22078
Mitra, R., Chen, X., Greenawalt, E. J., Maulik, U., Jiang, W., Zhao, Z., et al. (2017). Decoding critical long non-coding RNA in ovarian cancer epithelial-to-mesenchymal transition. Nat. Commun. 8:1604.
Mortazavi, A., Williams, B. A., Mccue, K., Schaeffer, L., and Wold, B. (2008). Mapping and quantifying mammalian transcriptomes by RNA-Seq. Nat. Methods 5, 621–628. doi: 10.1038/nmeth.1226
Nagano, T., and Fraser, P. (2011). No-nonsense functions for long noncoding RNAs. Cell 145, 178–181. doi: 10.1016/j.cell.2011.03.014
Nagano, T., Mitchell, J. A., Sanz, L. A., Pauler, F. M., Ferguson-Smith, A. C., Feil, R., et al. (2008). The Air noncoding RNA epigenetically silences transcription by targeting G9a to chromatin. Science 322, 1717–1720. doi: 10.1126/science.1163802
Norris, R. P., Ratzan, W. J., Freudzon, M., Mehlmann, L. M., Krall, J., Movsesian, M. A., et al. (2009). Cyclic GMP from the surrounding somatic cells regulates cyclic AMP and meiosis in the mouse oocyte. Development 136, 1869–1878. doi: 10.1242/dev.035238
Peng, X.-R., Liu, T., and Zhang, Y. (2008). Addition of Alpha-Tocopherol to Culture Medium Improves the Quality and Cryosurvival of Nnuclear-Transferred Ovine Embryos. J. Reproduct. Dev. 54, 403–407. doi: 10.1262/jrd.19188
Pertea, M., Pertea, G. M., Antonescu, C. M., Chang, T.-C., Mendell, J. T., and Salzberg, S. L. (2015). StringTie enables improved reconstruction of a transcriptome from RNA-seq reads. Nat. Biotechnol. 33, 290–295. doi: 10.1038/nbt.3122
Ran, M., Chen, B., Li, Z., Wu, M., Liu, X., He, C., et al. (2016). Systematic identification of long noncoding RNAs in immature and mature porcine testes. Biol. Reproduct. 94, 71–79.
Ransohoff, J. D., Wei, Y., and Khavari, P. A. (2018). The functions and unique features of long intergenic non-coding RNA. Nat. Rev. Mol. Cell Biol. 19, 143–157. doi: 10.1038/nrm.2017.104
Raudvere, U., Kolberg, L., Kuzmin, I., Arak, T., Adler, P., Peterson, H., et al. (2019). g: Profiler: a web server for functional enrichment analysis and conversions of gene lists (2019 update). Nucleic Acids Res. 47, w191–w198. doi: 10.1093/nar/gkz369
Rinn, J. L., and Chang, H. Y. (2012). Genome regulation by long noncoding RNAs. Ann. Rev. Biochem. 81, 145–166.
Rossi, M. N., and Antonangeli, F. (2014). LncRNAs: new players in apoptosis control. Int. J. Cell Biol. 2014:473857.
Schmitt, A., and Nebreda, A. R. (2002). Signalling pathways in oocyte meiotic maturation. J. Cell Sci. 115, 2457–2459.
Su, J., Wang, Y., Zhang, L., Wang, B., Liu, J., Luo, Y., et al. (2014). Oocyte-secreted factors in oocyte maturation media enhance subsequent development of bovine cloned embryos. Mol. Reproduct. Dev. 81, 341–349. doi: 10.1002/mrd.22302
Sulayman, A., Tian, K., Huang, X., Tian, Y., Xu, X., Fu, X., et al. (2019). Genome-wide identification and characterization of long non-coding RNAs expressed during sheep fetal and postnatal hair follicle development. Sci. Rep. 9, 1–14.
Sun, L., Luo, H., Bu, D., Zhao, G., Yu, K., Zhang, C., et al. (2013). Utilizing sequence intrinsic composition to classify protein-coding and long non-coding transcripts. Nucleic Acids Res. 41, e166–e166. doi: 10.1093/nar/gkt646
Sun, M., and Kraus, W. L. (2013). Minireview: long noncoding RNAs: new “links” between gene expression and cellular outcomes in endocrinology. Mol. Endocrinol. 27, 1390–1402. doi: 10.1210/me.2013-1113
Sun, Y., Chen, X., Qin, J., Liu, S., Zhao, R., Yu, T., et al. (2018). Comparative analysis of long noncoding RNAs expressed during intramuscular adipocytes adipogenesis in fat-type and lean-type pigs. J. Agricult. Food Chem. 66, 12122–12130. doi: 10.1021/acs.jafc.8b04243
Tosti, E. (2006). Calcium ion currents mediating oocyte maturation events. Reproduct. Biol. Endocrinol. 4, 26.
Vaccari, S., Weeks, J. L., Hsieh, M., Menniti, F. S., and Conti, M. (2009). Cyclic GMP signaling is involved in the luteinizing hormone-dependent meiotic maturation of mouse oocytes. Biol. Reproduct. 81, 595–604. doi: 10.1095/biolreprod.109.077768
Wang, H., Cai, H., Wang, X., Zhang, M., Liu, B., Chen, Z., et al. (2019). HDAC3 maintains oocyte meiosis arrest by repressing amphiregulin expression before the LH surge. Nat. Commun. 10, 1–12.
Wang, K. C., and Chang, H. Y. (2011). Molecular mechanisms of long noncoding RNAs. Mol. Cell 43, 904–914. doi: 10.1016/j.molcel.2011.08.018
Wang, Y. (2019). Comprehensive long non-coding RNA expression profiling by RNA sequencing reveals potential biomarkers for acute myeloid leukemia risk. Cancer Biomark. 26, 93–108. doi: 10.3233/cbm-190215
Wapinski, O., and Chang, H. Y. (2011). Long noncoding RNAs and human disease. Trends Cell Biol. 21, 354–361. doi: 10.1016/j.tcb.2011.04.001
Woodruff, T. J., Janssen, S. J., Guillette, L. J. Jr., and Giudice, L. C. (2010). Environmental Impacts on Reproductive Health and Fertility. Cambridge: Cambridge University Press.
Wucher, V., Legeai, F., Hedan, B., Rizk, G., Lagoutte, L., Leeb, T., et al. (2017). FEELnc: a tool for long non-coding RNA annotation and its application to the dog transcriptome. Nucleic Acids Res. 45:e57.
Yan, P., Luo, S., Lu, J. Y., and Shen, X. (2017). Cis-and trans-acting lncRNAs in pluripotency and reprogramming. Curr. Opin. Genet. Dev. 46, 170–178. doi: 10.1016/j.gde.2017.07.009
Yu, G., Wang, L.-G., Han, Y., and He, Q.-Y. (2012). clusterProfiler: an R package for comparing biological themes among gene clusters. Omics 16, 284–287. doi: 10.1089/omi.2011.0118
Zhang, F.-L., Li, N., Wang, H., Ma, J.-M., Shen, W., and Li, L. (2019). Zearalenone Exposure Induces the Apoptosis of Porcine Granulosa Cells and Changes Long Noncoding RNA Expression To Promote Antiapoptosis by Activating the JAK2–STAT3 Pathway. J. Agricult. Food Chem. 67, 12117–12128. doi: 10.1021/acs.jafc.9b05189
Zhang, L., Lu, H., Xin, D., Cheng, H., and Zhou, R. (2010). A novel ncRNA gene from mouse chromosome 5 trans-splices with Dmrt1 on chromosome 19. Biochem. Biophys. Res. Commun. 400, 696–700. doi: 10.1016/j.bbrc.2010.08.130
Zhang, M., Su, Y.-Q., Sugiura, K., Xia, G., and Eppig, J. J. (2010). Granulosa cell ligand NPPC and its receptor NPR2 maintain meiotic arrest in mouse oocytes. Science 330, 366–369. doi: 10.1126/science.1193573
Zhao, J., Xu, J., Wang, W., Zhao, H., Liu, H., Liu, X., et al. (2018). Long non-coding RNA LINC-01572: 28 inhibits granulosa cell growth via a decrease in p27 (Kip1) degradation in patients with polycystic ovary syndrome. EBioMedicine 36, 526–538. doi: 10.1016/j.ebiom.2018.09.043
Keywords: lncRNA–mRNA network, ewe, oocytes, meiotic maturation, RNA-Seq
Citation: Wang J-J, Niu M-H, Zhang T, Shen W and Cao H-G (2020) Genome-Wide Network of lncRNA–mRNA During Ovine Oocyte Development From Germinal Vesicle to Metaphase II in vitro. Front. Physiol. 11:1019. doi: 10.3389/fphys.2020.01019
Received: 17 April 2020; Accepted: 24 July 2020;
Published: 18 August 2020.
Edited by:
Kui Liu, The University of Hong Kong, Hong KongReviewed by:
Yimin Zhu, Zhejiang University School of Medicine, ChinaHuan Shen, Peking University, China
Copyright © 2020 Wang, Niu, Zhang, Shen and Cao. This is an open-access article distributed under the terms of the Creative Commons Attribution License (CC BY). The use, distribution or reproduction in other forums is permitted, provided the original author(s) and the copyright owner(s) are credited and that the original publication in this journal is cited, in accordance with accepted academic practice. No use, distribution or reproduction is permitted which does not comply with these terms.
*Correspondence: Wei Shen, d3NoZW5AcWF1LmVkdS5jbg==; c2hlbndlaTQyN0AxNjMuY29t; Hong-Guo Cao, Y2FvaG9uZ2d1b0AxMjYuY29t; Y2FvaG9uZ2d1bzFAMTI2LmNvbQ==