- 1Faculty of Medicine, Centre for Functional Genomics and Bio-Chips, Institute of Biochemistry, University of Ljubljana, Ljubljana, Slovenia
- 2Faculty of Computer and Information Science, University of Ljubljana, Ljubljana, Slovenia
The liver is to date the best example of a sexually dimorphic non-reproductive organ. Over 1,000 genes are differentially expressed between sexes indicating that female and male livers are two metabolically distinct organs. The spectrum of liver diseases is broad and is usually prevalent in one or the other sex, with different contributing genetic and environmental factors. It is thus difficult to predict individual's disease outcomes and treatment options. Systems approaches including mathematical modeling can aid importantly in understanding the multifactorial liver disease etiology leading toward tailored diagnostics, prognostics and therapy. The currently established computational models of hepatic metabolism that have proven to be essential for understanding of non-alcoholic fatty liver disease (NAFLD) and hepatocellular carcinoma (HCC) are limited to the description of gender-independent response or reflect solely the response of the males. Herein we present LiverSex, the first sex-based multi-tissue and multi-level liver metabolic computational model. The model was constructed based on in silico liver model SteatoNet and the object-oriented modeling. The crucial factor in adaptation of liver metabolism to the sex is the inclusion of estrogen and androgen receptor responses to respective hormones and the link to sex-differences in growth hormone release. The model was extensively validated on literature data and experimental data obtained from wild type C57BL/6 mice fed with regular chow and western diet. These experimental results show extensive sex-dependent changes and could not be reproduced in silico with the uniform model SteatoNet. LiverSex represents the first large-scale liver metabolic model, which allows a detailed insight into the sex-dependent complex liver pathologies, and how the genetic and environmental factors interact with the sex in disease appearance and progression. We used the model to identify the most important sex-dependent metabolic pathways, which are involved in accumulation of triglycerides representing initial steps of NAFLD. We identified PGC1A, PPARα, FXR, and LXR as regulatory factors that could become important in sex-dependent personalized treatment of NAFLD.
Introduction
The pharmacological and clinical discussions about the influence of gender on drug metabolism and disease susceptibility are raising, while on the other hand studies that would reveal the molecular basis of sex-based differences in humans are limited (Flórez-Vargas et al., 2016). Sexual dimorphism in animal kingdom has been known for centuries. Despite this, the majority of studies still focus on one sex and the results are discussed in a generalized manner. The choice of males as the dominant research model was justified by studies that showed females having higher biological variability associated with fluctuation of sex hormones during the reproductive cycle (McGregor et al., 2016).
Sexual dimorphism is a widespread phenomenon of somatic, physiologic, and behavioral differences between females and males (Söder, 2007; Urlep et al., 2017). Genes regulated by sex hormones differ in their tissue expression, which is particularly true for liver metabolism (Gustafsson et al., 1983). Transcriptome and proteome studies report that scope of described sexually dimorphic gene expression is significantly larger than previously recognized. Thousands of genes differ in expression between females and males not only in the liver (Laz et al., 2004; Yang et al., 2006; Waxman and Holloway, 2009) but also in adipose tissue and muscle, while brain expression seems to be less sexually dimorphic (Yang et al., 2006). In the context of the liver pathologies dissimilarities of sex hormones are listed among the main reasons for the differences in the prevalence of liver diseases. Hepatocellular carcinoma (HCC) is more frequent in males (Zheng et al., 2017), while females have increased risk of autoimmune liver diseases and exacerbated liver damage in alcoholic liver disease (Guy and Peters, 2013). In non-alcoholic fatty liver disease (NAFLD) the distinction is less clear with inconsistent reports of increased incidence in males and post-menopausal women, possibly due to increased tendency for visceral fat accumulation (Suzuki and Abdelmalek, 2009; Pan and Fallon, 2014).
A recent comprehensive review of NAFLD studies (Ballestri et al., 2017) identified age, sex, body construction, susceptibility to gaining weight, existence of metabolic syndrome and genetically determined characteristics as critical factors influencing NAFLD onset and/or progression. Studies show that the progression of NAFLD in males is independent of age (Kojima et al., 2003; Xu et al., 2013). This is in contrast to the correlation between age and NAFLD incidence in females, where NAFLD occurrence is decreased in premenopausal, but not in postmenopausal women (Hamaguchi et al., 2012; Florentino et al., 2013). Another study reported that women with NAFLD are approximately 10 years older than men (Carulli et al., 2006). Based on these studies, premenopausal women might be better protected from developing NAFLD compared to men and postmenopausal women. Estrogens might provide part of the explanation, as it has been reported that women with NAFLD have lower concentrations of serum estradiol than woman without NAFLD (Gutierrez-Grobe et al., 2010).
To describe the complex nature of liver metabolism and predict all possible consequences of genetic and metabolic insults computational approaches are applied (Petta et al., 2016; Hoehme et al., 2017; Lorente et al., 2017). Several large-scale metabolic models have been established to investigate liver metabolism (Holzhütter et al., 2012; Drasdo et al., 2014) and liver related diseases, such as NAFLD (Mardinoglu et al., 2014; Naik et al., 2014) and HCC (Agren et al., 2014). Large-scale metabolic models of liver metabolism and their clinical applications have recently been reviewed (Cvitanović et al., 2017). Among the most popular state-of-the-art computational approaches are genome-scale metabolic networks, where omics data are integrated to better understand the genotype-phenotype relationships (Lewis et al., 2012). Large-scale metabolic models, however, do not differentiate between genders and are mostly established and validated on the unified or male data. Gender-based differentiation has been performed only in smaller models, which do not account for the whole liver metabolism. Matthews et al. (2007) constructed a database and a model to predict reproductive toxicity in both genders, fetal dysmorphogenesis, functional toxicity, mortality, growth, and new-born behavioral toxicity of untested chemicals. A computational model of oxygenation and transport of solutes in the kidneys of spontaneously hypertensive female rats was used to investigate the sex differences in nitric oxide levels (Chen et al., 2017). Agren et al. (2014) established a liver metabolic model that indirectly accounted for gender-related differences. They reconstructed a personalized genome-scale metabolic model for each of the 27 patients with hepatocellular carcinoma. Ten of the 27 patients were females, which shows that personalized approaches can and should take gender into account. The major reason for the lack of human gender-based large-scale metabolic models might be an insufficient number of liver transcriptome-based studies that would account for both sexes (Zhang et al., 2012; Oshida et al., 2016).
Herein we present LiverSex, the first gender based multi-tissue and multi-level liver metabolic model. The construction of the model was performed with the extension and adaptation of the SteatoNet model (Naik et al., 2014), which was generated to investigate hepatic metabolism and liver related deregulations. SteatoNet as well as LiverSex feature two crucial characteristics: they account for (1) interactions between hepatic metabolic pathways and extra-hepatic tissues, and (2) regulations on transcriptional and post-translational levels. The experimentally observed sexual differences in liver gene expression were successfully reproduced in silico with LiverSex. Finally, the sensitivity analysis was applied to identify sex-dependent liver metabolic network deregulations that transform healthy liver to NAFLD.
Materials and Methods
SteatoNet and Object Oriented Modeling
SteatoNet (Steatosis Network) (Naik et al., 2014) represents a dynamic semi-quantitative model based on a steady-state analysis of differential algebraic equations (DAEs). SteatoNet was established in object-oriented modeling language Modelica. It is based on the Systems Biology library SysBio (Belič et al., 2013), which was constructed to describe biological pathway entities. The SysBio library includes objects corresponding to the biological behavior of enzymes, metabolites, non-enzymatic regulatory proteins, mRNAs, flux sources, gene expression regulations, etc. Using object-oriented modeling approach models are easy to construct by linking the basic objects of SysBio library into a meaningful and hierarchical composition. Due to the steady-state normalization of the observed quantities most of the parameters describing the dynamics of the observed system are lumped when using SysBio library. This simplifies the model establishment, since only a small set of parameters needs to be evaluated. The main parameters governing the dynamical properties of the established models describe the metabolic flux distributions in each of the pathway branches. SteatoNet includes all major pathways of mammalian liver metabolism. Pathways that are included in the model were manually acquired from KEGG (Du et al., 2014) and REACTOME (Croft et al., 2011) databases and from the literature. SteatoNet additionally describes the transport of metabolites between liver, adipose tissue, pancreas, other extra-hepatic tissues, and macrophages via blood. The external sources of nutrients [influx of fatty acids and triglycerides (TG), glucose, cholesterol, and essential amino acids] have also been included into the model. Details of its structure have been extensively described before (Naik et al., 2014).
Data Availability
Freely accessible version of the SteatoNet and LiverSex along with the SysBio library can be downloaded from http://lrss.fri.uni-lj.si/bio/sysbio. The simulations can be executed with the open source Modelica simulation environment OpenModelica, which can be downloaded from https://openmodelica.org.
Construction of LiverSex
The hormonal regulation is simplified to a level that still ensures normal function. All included hormones are arranged into three groups: growth hormone, androgen, and estrogens. Androgen or estrogen groups represent any steroid hormones that regulate the development and maintenance of sex characteristics in vertebrates by binding to corresponding steroid hormone receptor (Sharma et al., 2017). Each group of hormones has its own source of flux. These sources display differences in hormonal regulation of liver between females and males. The growth hormone source acts as a daily oscillator in males or has a constant concentration in females (Norstedt and Palmiter, 1984; Waxman and O'connor, 2006). The female estradiol source mimics the monthly estrous cycle that cannot be found in males (Ciana et al., 2003; Shanle and Xu, 2010; Villa et al., 2012). In males, the androgen source is 10-fold higher than the estrogens source (Domonkos et al., 2017), while in females the androgen source is three-fold lower than the estrogen source (Simpson, 2003). Every hormone source is connected to its respective receptor. Each hormone receptor has an active and inactive form, which allows us to simulate different diseases connected with the perturbations of receptor functions. Active form of the receptors is in addition linked to SteatoNet according to the literature evidence (Heine et al., 2000; Barclay et al., 2011; Gårevik et al., 2012) and these connections than finally lead to the female and male LiverSex model. A more detailed description of sex-dependent hormonal regulation of liver metabolism is included in the Supplementary Data.
Validation of LiverSex
Expression profiling data (GEO database GSE78892) obtained from the hepatocyte specific Cyp51 knockout mice (Cyp51flox/flox; Alb-Cre) (Lorbek et al., 2013, 2015) are used for direct LiverSex validation. Female and male mice of mixed genetic background (129/Pas × C57BL/6J, close to 90% C57BL/6J) were included in the experiment and after the weaning period (age 3 weeks) put on a standard laboratory chow (Altromin) or isocaloric high-fat diet with 1.25% (w/w) of cholesterol (western diet) for another 16 weeks. For validation of LiverSex three objects defining the diet have been included in the model: sources of glucose, cholesterol, and triglycerides. Experimental conditions were simulated in the model by altering the nutrient (triglyceride and cholesterol) influx into the network, thus mimicking the experimental diets. Inconsistencies between biological observations and model simulations mainly occurred due to the missing components and regulatory connections in the model. A series of simulations was executed to identify the network components that caused erroneous behavior. Further in-depth literature searches were performed in the context of these components to identify the regulations that were absent in the network or were incorrectly depicted. The western diet was replicated in silico by increasing the influx of triglycerides (10-fold) and cholesterol (five-fold) (fold-changes were estimated from the strict composition of western diet and standard laboratory chow).
Sensitivity Analysis
Sensitivity analysis can provide valuable insight in the robustness of the computational model in dependence on the perturbations of model inputs, i.e., parameters values (Bentele et al., 2004). Moreover, sensitivity analysis can identify parameters with the greatest impact on the observed outputs. These parameters present potential targets for further experimental analysis (Zi, 2011). Sensitivity analysis has an important function in the analysis of computational models in systems biology and medicine (Ingalls, 2008). Metabolic Control Analysis (MCA) presents a sensitivity analysis method (Fell and Sauro, 1985) that was initially focused to the analysis of metabolic networks. The method was later adapted to the models of other biological networks such as cell signaling models, models of genetic networks, and models of other biological processes (Westerhoff et al., 1984; Heinrich and Schuster, 1996). MCA assesses the sensitivity of the model output with respect to the selected input with evaluation of control coefficients. Here, metabolic fluxes through the observed metabolic reactions or concentration of metabolites can be used as model outputs while model parameters can be used as model inputs. The MCA of the distribution of the metabolic fluxes in each of the pathway branches can be performed with the following equation:
where represents the concentration control coefficient of parameter f with regard to X. X* and X describe the concentration of the observed metabolite, which presents a variable under study at nominal (f) and perturbed flux distribution value (f*). The ratio between f and X is normalized to achieve the relative value of sensitivity coefficient.
According to our previous experimental data and the literature data the NAFLD pathogenesis can be associated with de novo lipogenesis (Lorbek et al., 2013, 2015; Green et al., 2015; Sanders and Griffin, 2016; Softic et al., 2016). Our analyses of NAFLD progression were thus based on the observation of triglyceride accumulation. The variations in their accumulation were observed in dependence on the distribution of metabolic fluxes at pathway branch points, which can be described as model inputs. The concentration control coefficient from the Equation (1) was thus defined as a partial derivate of the alterations in triglyceride concentration with respect to the small changes in the distribution of fluxes at pathway branch points:
where TG* and TG are triglyceride concentrations with flux distribution parameter values of f* and f, respectively. The described method was used to identify the branch points with the largest sensitivities in each of the models. Moreover, the obtained values were used to identify branch points and their corresponding metabolic pathways, which have the largest gender-dependent influence on NAFLD progression.
To evaluate the sensitivity of hepatic triglyceride concentration in dependence on the metabolic flux distribution of LiverSex, the triglyceride influx into the model was raised by 10-fold to imitate high fat diet. The distribution parameter for each branch point in the pathway model was varied incrementally by 5% up to a maximum of 30%. Further investigation was focused on the analysis of gender-based influences of regulatory factors on the previously identified metabolic pathways. We calculated the concentration control coefficient of regulatory factors, i.e., , using Equation (1), where X is replaced with regulator R from the list of molecular regulators (see Supplementary Table 2). Analyses were performed for each of the molecular regulators.
Results
Construction of LiverSex by Modeling Hormonal Regulation With SysBio Library
LiverSex is composed of mathematical expressions of relationships between sex hormones and growth hormone, their corresponding receptors in hepatocytes and is based on literature evidence. An object named hormonal regulation is inserted into the blood section of the model. This object is connected with corresponding pathways in the hepatocyte. As explained in detail in the Materials and Methods section, it includes androgens, estrogens, and growth hormone (Figure 1). Each group of hormones has an effect on its corresponding receptor. The behavior of hormones is determined by gender, which results in two gender-specific models.
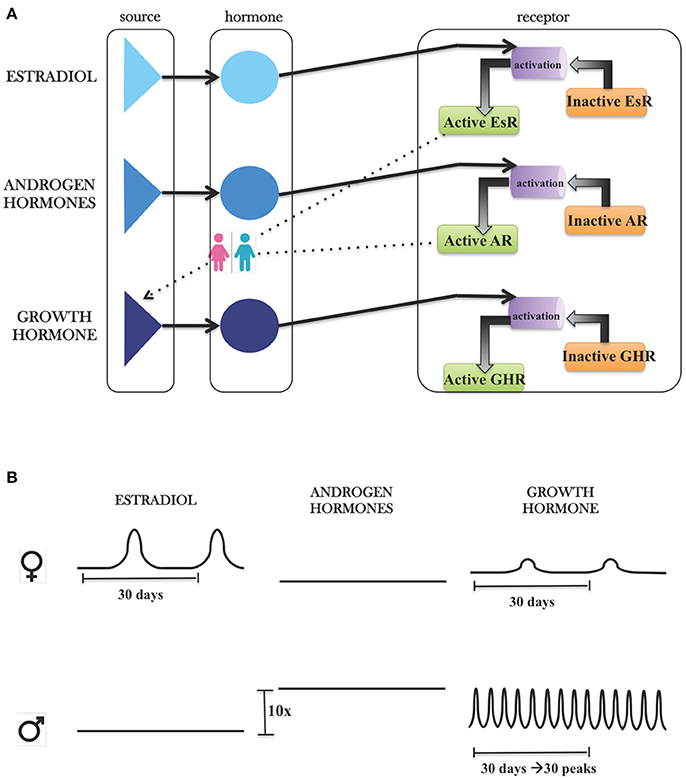
Figure 1. Modeling hormonal regulation with SysBio library. (A) Graphical presentation of hormonal object in SysBio library. Each hormone group contains its own source, the hormone and corresponding receptor, which is further connected with hepatocyte based on literature evidence. (B) Differences between hormone sources based on the literature evidence. FEMALES: Estrogen concentrations are three-fold higher than androgens, and estrogens have one peak, which is consistent with the monthly oestrous cycle. Because of the estrogen receptor feedback regulation on growth hormone, we can observe the influence of estrous cycle on growth hormone. MALES: Androgen concentrations are 10-fold higher than concentrations of estrogens. Growth hormone concentrations show daily oscillations with ~24-h period.
LiverSex Validation With Experimental Data
Three objects describing the diet have been included in LiverSex: glucose source, cholesterol source, and triglyceride source. Experimental conditions were mimicked in the model by altering the substrate (triglyceride and cholesterol) influx into the network in dependence on the experimental diets investigated (standard laboratory diet and western diet).
The set of 45 genes that were differentially expressed between females and males in mice fed with altromin and western diets (Lorbek et al., 2013, 2015) was screened for genes that are regulated inversely in females and males: either upregulated in males and downregulated in females or vice versa. Three inversely expressed genes were obtained (Figure 2A). The 1-AcylGlycerol-3-Phosphate O-AcylTransferase gene Agpat which is involved in lipid and glucose metabolism, and Protein Kinase AMP-Activated catalytic subunit Alpha gene Prkaa were downregulated in males and upregulated in females, while the Insulin Receptor Substrate gene Irs, which is involved in insulin signaling pathway, was upregulated in males and downregulated in females. While the simulation results obtained by the SteatoNet could only be attributed to males (Figure 2B), LiverSex correctly described the data from both genders (Figures 2C,D).
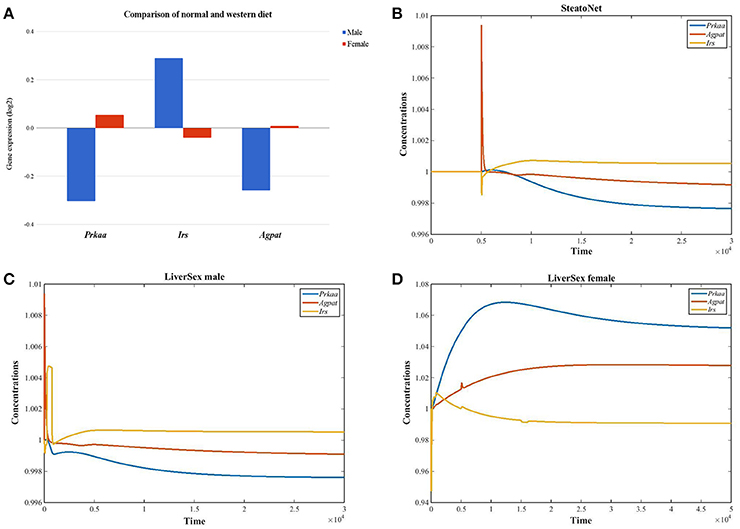
Figure 2. LiverSex validation with experimental data. (A) Graph represents gender based gene expression data obtained with the experiment (mice fed with standard laboratory diet and western diet). For these genes, we looked up the log2 fold change values and p-values for the comparison of both diets in wild-type female and male mice. (B) SteatoNet simulation results represent only the male gender. (C) LiverSex simulation results represent the male gene expression response on the high fat diet. (D) The female LiverSex response to the high fat diet.
LiverSex Prediction of Signaling Pathways That Trigger NAFLD
With sensitivity analysis we were able to identify metabolic reactions with the largest gender dependent influence on hepatic triglyceride accumulation, which is considered as the initial stage of NAFLD. Table 1 shows concentration control coefficients with respect to hepatic triglyceride accumulation listed in direction from the maximal to the minimal differences in sensitivity values for males and females. Only the first 20 parameters are listed in Table 1 (see Supplementary Material for the full list of parameters—Supplementary Table 1). If we classify the metabolic reaction with 1 as highly sensitive with respect to hepatic triglyceride accumulation, only 3 reactions can be regarded as highly sensitive: transformation of monoacylglycerol to glycerol (k159), transport of triglycerides from liver to adipose triglyceride lipid droplets (k177), and transformation of acetoacetate to β-hydroxybutyrate (k152). Transformation of monoacylglycerol to glycerol and transport of hepatic triglycerides to adipose triglyceride lipid droplets are highly sensitive metabolic pathways in both genders, but metabolic reaction from acetoacetate to β-hydroxybutyrate, which is a part of the body ketone metabolism, shows a higher tendency for female hepatic triglyceride accumulation only.
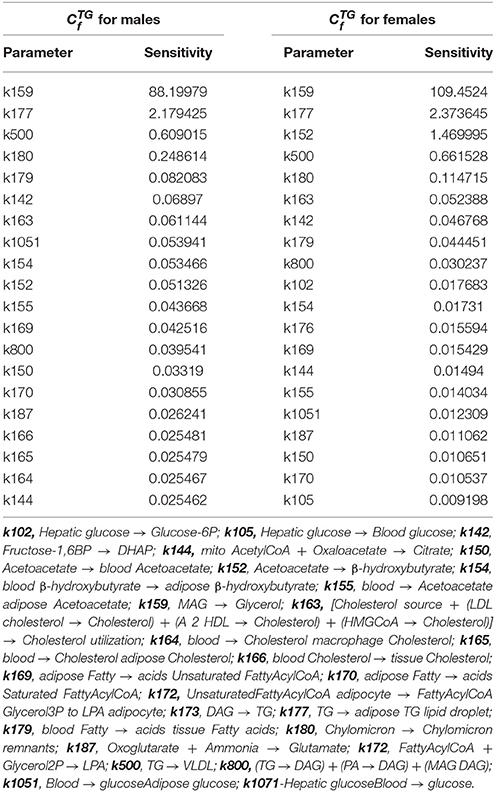
Table 1. Concentration control coefficients with respect to hepatic triglyceride accumulation in LiverSex for males in females.
The concentration control coefficients reflect the impact of perturbations on metabolite concentration. In our case, these coefficients measure the relative steady-state change in triglyceride accumulation in response to the relative change in fluxes at metabolic branch points. Data obtained with sensitivity analysis are sorted by descending absolute difference of sensitivity values between male and female. It is interesting that the first few pathways show a predominant effect in females (Figure 3). The terminal degradation of triglycerides by conversion of monoacylglycerol to glycerol (k159) seems to be the most powerful flux in the network in both sexes. However, upon perturbation with the diet, triglyceride accumulation is affected more in females. Hepatic glycerol utilization is a metabolic pathway, which is preferentially connected with carbohydrate metabolism in men and lipid metabolism in women (Rodríguez et al., 2015). Females display a higher sensitivity to ketone body metabolism (k152), transport of triglycerides (k177), and VLDL transport (k500), which carries triglycerides from the liver, possibly to avoid the development of fatty liver, taking them to the peripheral tissues for storage in adipose or for use in skeletal muscle.
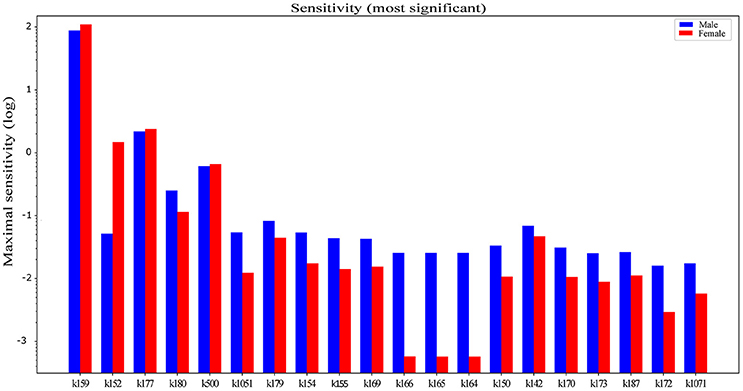
Figure 3. Difference between gender dependent concentration control coefficients with respect to hepatic triglyceride accumulation in LiverSex. Data are obtained with sensitivity analysis and sorted by descending absolute difference of sensitivity values in males and females. Data are presented in the logarithmic scale. k159, MAG → Glycerol; k152, Acetoacetate → β-hydroxybutyrate; k177, TG → adipose TG lipid droplet; k180, Chylomicron → Chylomicron remnants; k500, TG → VLDL; k1051, Blood glucose → Adipose glucose; k179, blood Fatty acids → tissue Fatty acids; k154, blood β-hydroxybutyrate → adipose β-hydroxybutyrate; k155, blood Acetoacetate adipose Acetoacetate; k169, adipose Fatty acids → Unsaturated FattyAcylCoA; k166, blood Cholesterol → tissue Cholesterol; k165, blood Cholesterol → adipose Cholesterol; k164, blood Cholesterol → macrophage Cholesterol; k150, Acetoacetate → blood Acetoacetate; k142, Fructose-1,6BP → DHAP; k170, adipose Fatty → acids Saturated FattyAcylCoA; k173, DAG → TG; k187, Oxoglutarate + Ammonia → Glutamate; k172, FattyAcylCoA + Glycerol2P LPA; k1071, Hepatic glucose → Blood glucose.
Regulatory Factors Involved in Sex-Dependent Differences in NAFLD Progression
Pathway branch points with the most significant absolute difference of between sexes were further investigated to identify regulatory factors, which are sensitive to alterations in flux distribution at these branches. By calculating with respect to various regulatory factors in the LiverSex, we obtained results presented in Table 2 and Supplementary Table 2. Figure 4 illustrates the regulatory factors with high sensitivity to alterations in flux distributions within the respective metabolic pathways. PGC1A (Peroxisome proliferator-activated receptor gamma coactivator 1-alpha, known also as PPARGC1A), which induces mitochondrial biogenesis, PPARα (Peroxisome Proliferator Activated Receptor alpha), the major regulator of lipid metabolism, FXR (Farnesoid X Receptor), a regulator of bile acid synthesis and excretion, LXR (Liver X Receptor), involved in lipid and cholesterol metabolism, and ADIPO (adiponectin), which is involved in glucose regulation and fatty acid oxidation, display global sensitivity to alterations in metabolic flux distribution at the majority of the high sensitivity pathway branches, indicating the broad role of these transcription factors.
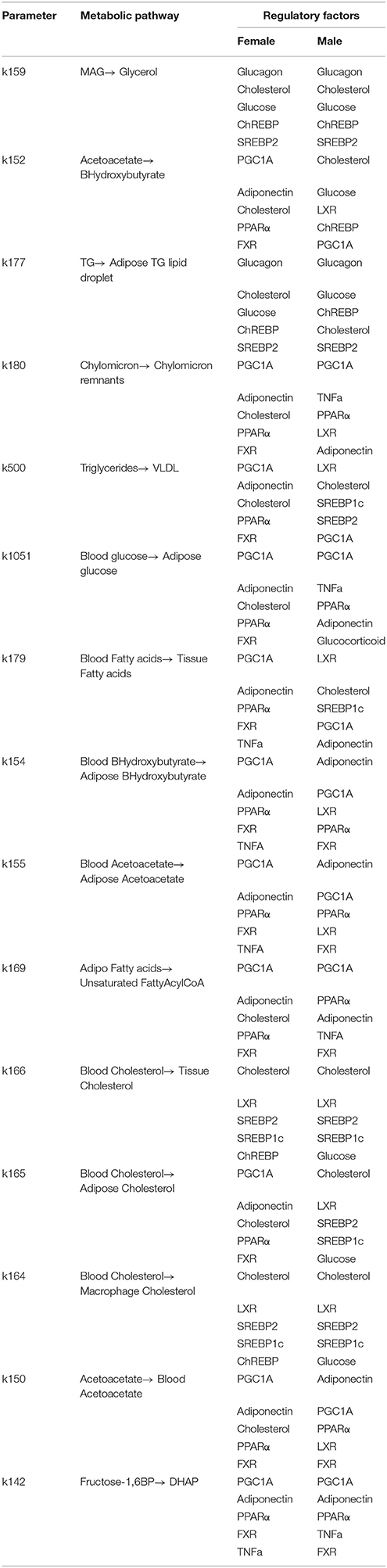
Table 2. Regulatory factors with high concentration control coefficients to alterations in flux distribution at presented branch points.
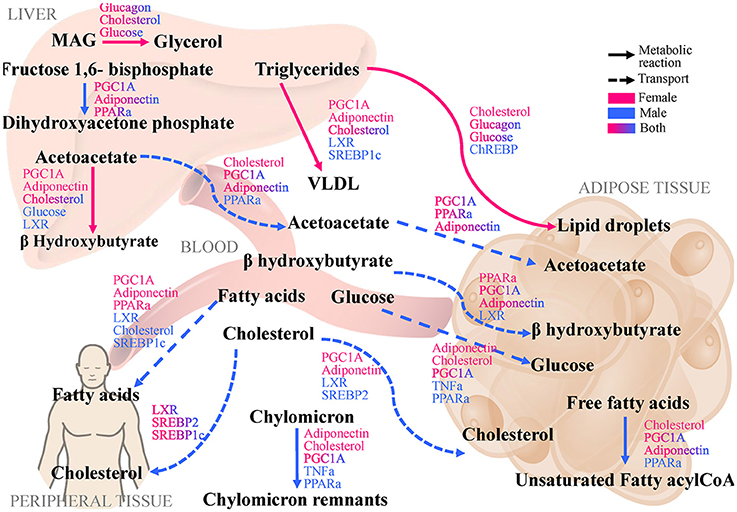
Figure 4. LiverSex identified pathways and regulatory factors with largest difference between genders and with respect to hepatic triglyceride accumulation as the first step of NAFLD. The displayed pathways that show the largest differences between genders and determine the change in hepatic triglyceride accumulation highlight branch-points with high dynamic sensitivity. Regulatory factors that are affected by modifications in flux distribution are categorized at the corresponding reaction to which they are sensitive. MAG, Monoacylglycerol; PGC1A, Peroxisome Proliferator-Activated Receptor Gamma Coactivator 1 alpha; PPARa, Peroxisome Proliferator-Activated Receptor alpha; FXR, Farnesoid X Receptor; LXR, Liver X Receptor; ChREBP, Carbohydrate Response Element-Binding Protein; SREBP-1c, Sterol Regulatory Element-Binding Protein-1c; SREBP-2, Sterol Regulatory Element-Binding Protein-2; VLDL, Very-Low Density Lipoprotein; TNFa, Tumor Necrosis Factor alpha.
Discussion
Object oriented modeling can be successfully employed to support hierarchical structuring, reuse and evolution of more complex models, independently of the application domain. This also holds true for the LiverSex, where SteatoNet is reused for adaptation to gender specific data. An object with hormonal regulation is added to the SysBio library and after that positioned into SteatoNet and connected to the liver based on literature data. These models are constructed manually, i.e., by connecting the SysBio objects that correspond to the biological entities within the observed network. We are, however, currently working on the automation of this construction process. The LiverSex model by itself has certain limitations, which mainly originate from the derivation of the SysBio library, which is used for model construction. In order to reduce the space of unknown parameters, the SysBio library objects presume the normalized steady-state of the system. Obtained results thus do not correspond to the actual concentrations of observed metabolites due to the normalization of their concentrations (Naik et al., 2014). The LiverSex was, however, successful in replicating the results that correspond to different mice strains as well as human data found in the literature.
The mechanism responsible for NAFLD progression in humans is not yet fully understood. Furthermore, sex differences have a big impact on the prevalence of NAFLD. Based on the current data, premenopausal women are better protected from developing NAFLD compared to men and postmenopausal women (Ballestri et al., 2017). In this study, we tried to expose the sexual differences in NAFLD based on hepatic triglyceride accumulation. After performing sensitivity analyses, metabolic differences between sexes in NAFLD were identified (see Figure 4). Sensitivity analyses pinpointed to metabolic pathways, in which the same perturbations cause the largest differences in hepatic triglyceride accumulation. According to the literature data all metabolic pathways with a high sensitivity for hepatic triglyceride accumulation as reported by LiverSex are involved in the earliest stage of NAFLD pathogenesis (Cohen et al., 2011). One of the initial steps of NAFLD is hepatic steatosis, which is characterized by the deposition of triglycerides as lipid droplets (Reingold et al., 2005) and according to the results of the sensitivity analyses of LiverSex, this metabolic step is more sensitive in females.
Microsomal triglyceride transfers protein (MTTP) facilitates triglyceride, cholesteryl ester, and phospholipid transport between phospholipid surfaces (k500). A defect in lipid export from the liver may also contribute to the pathogenesis of steatosis (Fabbrini et al., 2008). MTTP is necessary for the assembly and secretion of VLDL from hepatocytes (Jamil et al., 1995). It is responsible for lipoprotein assembly by transferring triglycerides, to nascent apolipoproteins B. The LiverSex demonstrates that transferring triglycerides by MTTP, which is involved in NAFLD progression, is more sensitive in females. It was previously reported that MTTP expression is sex-dependent and that female GH secretory pattern has a significant influence on its expression (Améen and Oscarsson, 2003).
Hormone sensitive lipase (HSL) converts monoacylglycerides to free fatty acids and glycerol (MGLL or MAGL) (k159) and this step regulates the quantity of fatty acids, which are used as signaling molecules and have been shown to promote cancer cell migration, invasion and tumor growth (Nomura et al., 2010). MAGL is a crucial lipolytic enzyme and an important regulator of tumor progression. It can promote hepatocellular carcinoma progression and was recently suggested as a potential therapeutic target and as a biomarker for prognosis in patients with HCC (Zhu et al., 2016). Expression of HSL is decreased in NAFLD compared with normal liver (Kohjima et al., 2007), which was also observed in our simulation results. There is currently no literature data describing the sex-specific differences in the expression of liver HSL. However, differences were reported previously in skeletal muscles, where women were found to have a higher intramuscular triacylglycerol during exercise than men, and also higher mRNA levels of HSL in the muscle. Yet, as HSL activity during prolonged exercise is higher in men it is likely that the enzyme-substrate interactions differ between the sexes (Roepstorff et al., 2006). In addition, our results show that women have a higher susceptibility to changes in lipolysis. show that women have a higher susceptibility to changes in lipolysis.
Removal of FA from the liver occurs by secretion as VLDL (k500) and by FA oxidation (k152) (Hodson and Frayn, 2011). Some studies suggest women have enhanced production and clearance of VLDL compared to men (Wang et al., 2011). Few studies have investigated sex-specific differences in FA oxidation and found out that after a prolonged overnight fasting, women metabolize FA toward 3-hydroxybutyrate (3OHB) to a greater extent than men (Halkes et al., 2003; Marinou et al., 2011). Other studies (Koutsari et al., 2011; Marinou et al., 2011) indicate that women exhibit a higher non-oxidative FFA disposal (i.e., esterification and storage as triglycerides) and, after an overnight fast, they are prone to partition fatty acids toward ketone body production rather than VLDL. These differences were also reflected by our LiverSex model.
Partition of fatty acids to ketone body production, VLDL synthesis and fatty acids oxidation, together with deposition of triglycerides as lipid droplets are considered as parts of NAFLD pathology, which were all found to be more sensitive in females in response to a high-fat diet challenge. The ability to partition fatty acids into different pathways might be one of the possible protective mechanisms in females leading to delayed NAFLD progression compared to males. However, further research is needed to confirm this hypothesis.
There are few metabolic pathways for which regulators do not show sexual dimorphism, such as transformation of monoacylglycerides to glycerol, fructose-1,6 bisphosphate to dihidroxyacetone phosphate and transport of cholesterol from blood to peripheral tissues. However, transport of fatty acids from blood to peripheral tissues and cholesterol from blood to the adipose tissue are pathways, in which regulatory factors differ substantially between genders. The activity of regulatory factors such as PGC1A, PPARα, FXR, and LXR is highly related to gender (see Figure 4).
Several studies have focused on the analysis of mice with liver-specific knockouts crucial for growth hormone signaling proteins indicating an important role of growth hormone in hepatic triglyceride secretion (Cui et al., 2007; Fan et al., 2009; Barclay et al., 2011; Sos et al., 2011). Pgc1a has been proposed as one of the transcriptional targets responsible for steatosis (Cui et al., 2007; Fan et al., 2009; Barclay et al., 2011; Sos et al., 2011) and based on our observations women are more susceptible for this. Skeletal muscle-specific PGC-1a overexpression increased glucose uptake, glycogen and lipid droplets quantity (Mormeneo et al., 2012), raising the possibility that PGC1A could also promote triglyceride accumulation into adipose lipid droplets as predicted by our model.
PPARα is known to be highly expressed in the liver and exhibits a sex-dimorphic nature. In the liver, PPARα promotes fatty acid oxidation, which makes it a possible drug target for treating hypertriglyceridemia (Rando and Wahli, 2011). In PPARα-null mice, gross hepatic abnormalities, disclosed to the steatotic liver and hepatomegaly, were found in males, but not females (Costet et al., 1998). LiverSex revealed a higher capacity of females to secrete triglycerides via VLDL compared to males, which was also the presumed cause for the increased steatosis resistance in female PPARα-null mice (Lindén et al., 2001).
Literature search did not reveal any sex-dependent FXR and LXR correlation to liver. FXR modulates hepatic inflammation, thus, FXR sex-dimorphic function in that context is less clear (Rando and Wahli, 2011). Indication that many of the FXR, PPARα, LXRα overlapping binding sites are functional (Boergesen et al., 2012) might present the reason for sex-dimorphic behavior of these nuclear receptors. Higher FXR sensitivity to the triglycerides accumulation in females could be correlated with higher binding of Retinoic X Receptor (RXRα), which is obligate heterodimerization partner of FXR to female hepatic chromatin for the essential lipid processing genes (Kosters et al., 2013). In addition, these sex-specific binding patterns and differences in ligand responsiveness may be the reasons for sex-specific distinctive effects of drugs. LXRs act as liver lipid sensors and regulate the metabolism of cholesterol and fatty acids (Schultz et al., 2000). LXR indirectly regulates Srebp1c, which is directly acting on Pnpla3 (Huang et al., 2010). It has been previously reported that LXR is not the subject of regulation by either dietary cholesterol or sex (Lorbek et al., 2013; Feillet et al., 2016), but LiverSex reported higher sensitivity of LXR in males in the initial steps of NAFLD.
Several nuclear receptors together with their molecular cascades are promising pharmacological targets for NAFLD treatment (Serviddio et al., 2013). Based on the results obtained by LiverSex, PGC1A, PPARα, FXR, and LXR could provide novel pharmacological targets for sex-based therapy in the future. Although the various studies emphasize the importance of regulators in modulating hepatic lipid homeostasis, data regarding the sex-dependent effects on the development of steatosis is still missing, emphasizing the need for further studies.
Our results suggest that one of the major hepatic characteristics is its sexually dimorphic nature. Sex steroids and growth hormone play a crucial role in fine-tuning the sex-dependent metabolic pathways in the liver. Further studies of sexually dimorphic genes and pathways as well as differences in their expression, are required for better insights into the complex functions of the liver and the relation to disease progression in both sexes. LiverSex and its future extensions in the context of personalized models may help with finding preventive approaches for NAFLD as well as other liver related sex-specific diseases.
Author Contributions
TC: Wrote the manuscript and conducted the computational part of the study; TC and ŽU: Conducted the experimental work and analyzed the data; MMo: Devised and supervised the computational part of the study; DR, MMo, and MMr: Provided critical feedback and helped shape the research, analysis, and manuscript.
Funding
Supported by the resources of FP7 CASyM (Coordinating Action Systems Medicine Europe, grant no. 305033) and by Slovenian Research Agency grants P1-0390, P2-0359, and the infrastructure grant ELIXIR.
Conflict of Interest Statement
The authors declare that the research was conducted in the absence of any commercial or financial relationships that could be construed as a potential conflict of interest.
The handling Editor declared a past co-authorship with one of the authors DR.
Supplementary Material
The Supplementary Material for this article can be found online at: https://www.frontiersin.org/articles/10.3389/fphys.2018.00360/full#supplementary-material
References
Agren, R., Mardinoglu, A., Asplund, A., Kampf, C., Uhlen, M., and Nielsen, J. (2014). Identification of anticancer drugs for hepatocellular carcinoma through personalized genome-scale metabolic modeling. Mol. Syst. Biol. 10:721. doi: 10.1002/msb.145122
Améen, C., and Oscarsson, J. (2003). Sex difference in hepatic microsomal triglyceride transfer protein expression is determined by the growth hormone secretory pattern in the rat. Endocrinology 144, 3914–3921. doi: 10.1210/en.2003-0518
Ballestri, S., Nascimbeni, F., Baldelli, E., Marrazzo, A., Romagnoli, D., and Lonardo, A. (2017). NAFLD as a sexual dimorphic disease: role of gender and reproductive status in the development and progression of nonalcoholic fatty liver disease and inherent cardiovascular risk. Adv. Ther. 34, 1291–1326. doi: 10.1007/s12325-017-0556-1
Barclay, J. L., Nelson, C. N., Ishikawa, M., Murray, L. A., Kerr, L. M., McPhee, T. R., et al. (2011). GH-dependent STAT5 signaling plays an important role in hepatic lipid metabolism. Endocrinology 152, 181–192. doi: 10.1210/en.2010-0537
Belič, A., Ačimovič, J., Naik, A., and Goličnik, M. (2013). Analysis of the steady-state relations and control-algorithm characterisation in a mathematical model of cholesterol biosynthesis. Simul. Model. Pract. Theor. 33, 18–27. doi: 10.1016/j.simpat.2011.12.010
Bentele, M., Lavrik, I., Ulrich, M., Stösser, S., Heermann, D., Kalthoff, H., et al. (2004). Mathematical modeling reveals threshold mechanism in CD95-induced apoptosis. J. Cell. Biol. 166, 839–851. doi: 10.1083/jcb.200404158
Boergesen, M., Pedersen, T. Å., Gross, B., van Heeringen, S. J., Hagenbeek, D., Bindesbøll, C., et al. (2012). Genome-wide profiling of liver X receptor, retinoid X receptor, and peroxisome proliferator-activated receptor α in mouse liver reveals extensive sharing of binding sites. Mol. Cell. Biol. 32, 852-867. doi: 10.1128/MCB.06175-11
Carulli, L., Lonardo, A., Lombardini, S., Marchesini, G., and Loria, P. (2006). Gender, fatty liver and GGT. Hepatology 44, 278–279. doi: 10.1002/hep.21218
Chen, Y., Sullivan, J. C., Edwards, A., and Layton, A. T. (2017). Sex-specific computational models of the spontaneously hypertensive rat kidneys: factors affecting nitric oxide bioavailability. Am. J. Physiol. Renal. 313, 174–183. doi: 10.1152/ajprenal.00482.2016
Ciana, P., Raviscioni, M., Mussi, P., Vegeto, E., Que, I., Parker, M. G., et al. (2003). In vivo imaging of transcriptionally active estrogen receptors. Nat. Med. 9, 82–86. doi: 10.1038/nm809
Cohen, J. C., Horton, J. D., and Hobbs, H. H. (2011). Human fatty liver disease: old questions and new insights. Science 332, 1519–1523. doi: 10.1126/science.1204265
Costet, P., Legendre, C., Moré, J., Edgar, A., Galtier, P., and Pineau, T. (1998). Peroxisome proliferator-activated receptor α-isoform deficiency leads to progressive dyslipidemia with sexually dimorphic obesity and steatosis. J. Biol. Chem. 273, 29577–29585. doi: 10.1074/jbc.273.45.29577
Croft, D., O'Kelly, G., Wu, G., Haw, R., Gillespie, M., Matthews, L., et al. (2011). Reactome: a database of reactions, pathways and biological processes. Nucleic Acids Res. 39(Database issue), D691–D697. doi: 10.1093/nar/gkq1018
Cui, Y., Hosui, A., Sun, R., Shen, K., Gavrilova, O., Chen, W., et al. (2007). Loss of signal transducer and activator of transcription 5 leads to hepatosteatosis and impaired liver regeneration. Hepatology 46, 504–513. doi: 10.1002/hep.21713
Cvitanović, T., Reichert, M. C., Moškon, M., Mraz, M., Lammert, F., and Rozman, D. (2017). Large-scale computational models of liver metabolism: how far from the clinics? Hepatology 66, 1323–1334. doi: 10.1002/hep.29268
Domonkos, E., Borbelyova, V., Csongova, M., Bosy, M., Kacmarova, M., Ostatnikova, D., et al. (2017). Sex differences and sex hormones in anxiety-like behavior of aging rats. Horm. Behav. 93, 159–165. doi: 10.1016/j.yhbeh.2017.05.019
Drasdo, D., Bode, J., Dahmen, U., Dirsch, O., Dooley, S., Gebhardt, R., et al. (2014). The virtual liver: state of the art and future perspectives. Arch. Toxicol. 88, 2071–2075. doi: 10.1007/s00204-014-1384-6
Du, J., Yuan, Z., Ma, Z., Song, J., Xie, X., and Chen, Y. (2014). KEGG-PATH: kyoto encyclopedia of genes and genomes-based pathway analysis using a path analysis model. Mol. Biosyst. 10, 2441–2447. doi: 10.1039/C4MB00287C
Fabbrini, E., Mohammed, B. S., Magkos, F., Korenblat, K. M., Patterson, B. W., and Klein, S. (2008). Alterations in adipose tissue and hepatic lipid kinetics in obese men and women with nonalcoholic fatty liver disease. Gastroenterology 134, 424–431. doi: 10.1053/j.gastro.2007.11.038
Fan, Y., Menon, R. K., Cohen, P., Hwang, D., Clemens, T., DiGirolamo, D. J., et al. (2009). Liver-specific deletion of the growth hormone receptor reveals essential role of growth hormone signaling in hepatic lipid metabolism. J. Biol. Chem. 284, 19937–19944. doi: 10.1074/jbc.M109.014308
Feillet, C., Guérin, S., Lonchampt, M., Dacquet, C., Gustafsson, J. Å., Delaunay, F., et al. (2016). Sexual dimorphism in circadian physiology is altered in LXRα deficient mice. PLoS ONE 11:e0150665. doi: 10.1371/journal.pone.0150665
Florentino, G. S., Cotrim, H. P., Vilar, C. P., Florentino, A. V., Guimarães, G. M., and Barreto, V. S. (2013). Nonalcoholic fatty liver disease in menopausal women. Arq. Gastroenterol. 50, 180–185. doi: 10.1590/S0004-28032013000200032
Flórez-Vargas, O., Brass, A., Karystianis, G., Bramhall, M., Stevens, R., Cruickshank, S., et al. (2016). Bias in the reporting of sex and age in biomedical research on mouse models. Elife 5:e13615. doi: 10.7554/eLife.13615
Gårevik, N., Skogastierna, C., Rane, A., and Ekström, L. (2012). Single dose testosterone increases total cholesterol levels and induces the expression of HMG CoA Reductase. Subst. Abuse Treat. Prev. Policy 7:12. doi: 10.1186/1747-597X-7-12
Green, C. J., Pramfalk, C., Morten, K. J., and Hodson, L. (2015). From whole body to cellular models of hepatic triglyceride metabolism: man has got to know his limitations. Am. J. Physiol. Endocrinol. Metab. 308, E1–E20. doi: 10.1152/ajpendo.00192.2014
Gustafsson, J. A., Mode, A., Norstedt, G., and Skett, P. (1983). Sex steroid induced changes in hepatic enzymes. Annu. Rev. Physiol. 45, 51–60. doi: 10.1146/annurev.ph.45.030183.000411
Gutierrez-Grobe, Y., Ponciano-Rodríguez, G., Ramos, M. H., Uribe, M., and Méndez-Sánchez, N. (2010). Prevalence of non alcoholic fatty liver disease in premenopausal, postmenopausal and polycystic ovary syndrome women. The role of estrogens. Ann. Hepatol. 9, 402–409.
Guy, J., and Peters, M. G. (2013). Liver disease in women: the influence of gender on epidemiology, natural history, and patient outcomes. Gastroenterol. Hepatol. 9, 633–639.
Halkes, C., Van Dijk, H., Verseyden, C., de Jaegere, P. T., Plokker, H., Meijssen, S., et al. (2003). Gender differences in postprandial ketone bodies in normolipidemic subjects and in untreated patients with familial combined hyperlipidemia. Arterioscl. Thromb. Vasc. 23, 1875–1880. doi: 10.1161/01.ATV.0000092326.00725.ED
Hamaguchi, M., Kojima, T., Ohbora, A., Takeda, N., Fukui, M., and Kato, T. (2012). Aging is a risk factor of nonalcoholic fatty liver disease in premenopausal women. World J. Gastroenterol. 18:237. doi: 10.3748/wjg.v18.i3.237
Heine, P., Taylor, J., Iwamoto, G., Lubahn, D., and Cooke, P. (2000). Increased adipose tissue in male and female estrogen receptor-α knockout mice. Proc. Natl. Acad. Sci. U.S.A. 97, 12729–12734. doi: 10.1073/pnas.97.23.12729
Heinrich, R., and Schuster, S. (1996). The Regulation of Cellular Systems. New York, NY: Springer Science & Business Media. doi: 10.1007/978-1-4613-1161-4
Hodson, L., and Frayn, K. N. (2011). Hepatic fatty acid partitioning. Curr. Opin. Lipidol. 22, 216–224. doi: 10.1097/MOL.0b013e3283462e16
Hoehme, S., Friebel, A., Hammad, S., Drasdo, D., and Hengstler, J. G. (2017). Creation of three-dimensional liver tissue models from experimental images for systems medicine. Methods Mol. Biol. 1506, 319–362. doi: 10.1007/978-1-4939-6506-922
Holzhütter, H. G., Drasdo, D., Preusser, T., Lippert, J., and Henney, A. M. (2012). The virtual liver: a multidisciplinary, multilevel challenge for systems biology. Wires Syst. Biol. Med. 4, 221–235. doi: 10.1002/wsbm.1158
Huang, Y., He, S., Li, J. Z., Seo, Y.-K., Osborne, T. F., Cohen, J. C., et al. (2010). A feed-forward loop amplifies nutritional regulation of PNPLA3. Proc. Natl. Acad. Sci. U.S.A. 107, 7892–7897. doi: 10.1073/pnas.1003585107
Ingalls, B. (2008). Sensitivity analysis: from model parameters to system behaviour. Essays Biochem. 45, 177–194. doi: 10.1042/bse0450177
Jamil, H., Dickson, J. K., Chu, C. H., Lago, M. W., Rinehart, J. K., Biller, S. A., et al. (1995). Microsomal triglyceride transfer protein specificity of lipid binding and transport. J. Biol. Chem. 270, 6549–6554. doi: 10.1074/jbc.270.12.6549
Kohjima, M., Enjoji, M., Higuchi, N., Kato, M., Kotoh, K., Yoshimoto, T., et al. (2007). Re-evaluation of fatty acid metabolism-related gene expression in nonalcoholic fatty liver disease. Int. J. Mol. Med. 20, 351–358. doi: 10.3892/ijmm.20.3.351
Kojima, S. I., Watanabe, N., Numata, M., Ogawa, T., and Matsuzaki, S. (2003). Increase in the prevalence of fatty liver in Japan over the past 12 years: analysis of clinical background. J. Gastroenterol. 38, 954–961. doi: 10.1007/s00535-003-1178-8
Kosters, A., Sun, D., Wu, H., Tian, F., Felix, J. C., Li, W., et al. (2013). Sexually dimorphic genome- wide binding of retinoid X receptor alpha (RXRα) determines male-female differences in the expression of hepatic lipid processing genes in mice. PLoS One 8:e71538. doi: 10.1371/journal.pone.0071538
Koutsari, C., Basu, R., Rizza, R. A., Nair, K. S., Khosla, S., and Jensen, M. D. (2011). Nonoxidative free fatty acid disposal is greater in young women than men. J. Clin. Endocrinol. Metab. 96, 541–547. doi: 10.1210/jc.2010-1651
Laz, E. V., Wiwi, C. A., and Waxman, D. J. (2004). Sexual dimorphism of rat liver nuclear proteins: regulatory role of growth hormone. Mol. Cell. Proteomics 3, 1170–1180. doi: 10.1074/mcp.M400102-MCP200
Lewis, N. E., Nagarajan, H., and Palsson, B. O. (2012). Constraining the metabolic genotype-phenotype relationship using a phylogeny of in silico methods. Nat. Rev. Microbiol. 10:291. doi: 10.1038/nrmicro2737
Lindén, D., Alsterholm, M., Wennbo, H., and Oscarsson, J. (2001). PPARα deficiency increases secretion and serum levels of apolipoprotein B-containing lipoproteins. J. Lipid Res. 42, 1831–1840.
Lorbek, G., Perše, M., Horvat, S., Björkhem, I., and Rozman, D. (2013). Sex differences in the hepatic cholesterol sensing mechanisms in mice. Molecules 18, 11067–11085. doi: 10.3390/molecules180911067
Lorbek, G., Perše, M., Jeruc, J., Juvan, P., Gutierrez-Mariscal, F. M., Lewinska, M., et al. (2015). Lessons from hepatocyte-specific Cyp51 knockout mice: impaired cholesterol synthesis leads to oval cell-driven liver injury. Sci. Rep. 5:8777. doi: 10.1038/srep08777
Lorente, D., Martínez-Martínez, F., Rupérez, M., Lago, M., Martínez-Sober, M., Escandell-Montero, P., et al. (2017). A framework for modelling the biomechanical behaviour of the human liver during breathing in real time using machine learning. Expert Syst. Appl. 71, 342–357. doi: 10.1016/j.eswa.2016.11.037
Mardinoglu, A., Agren, R., Kampf, C., Asplund, A., Uhlen, M., and Nielsen, J. (2014). Genome-scale metabolic modelling of hepatocytes reveals serine deficiency in patients with non-alcoholic fatty liver disease. Nat. Commun. 5:3083. doi: 10.1038/ncomms4083
Marinou, K., Adiels, M., Hodson, L., Frayn, K. N., Karpe, F., and Fielding, B. A. (2011). Young women partition fatty acids towards ketone body production rather than VLDL-TAG synthesis, compared with young men. Br. J. Nutr. 105, 857–865. doi: 10.1017/S0007114510004472
Matthews, E. J., Kruhlak, N. L., Daniel Benz, R., and Contrera, J. F. (2007). A comprehensive model for reproductive and developmental toxicity hazard identification: I development of a weight of evidence QSAR database. Regul. Toxicol. Pharmacol. 47, 115–135. doi: 10.1016/j.yrtph.2006.11.002
McGregor, A. J., Hasnain, M., Sandberg, K., Morrison, M. F., Berlin, M., and Trott, J. (2016). How to study the impact of sex and gender in medical research: a review of resources. Biol. Sex. Differ. 7:46. doi: 10.1186/s13293-016-0099-1
Mormeneo, E., Jimenez-Mallebrera, C., Palomer, X., De Nigris, V., Vázquez-Carrera, M., Orozco, A., et al. (2012). PGC-1α induces mitochondrial and myokine transcriptional programs and lipid droplet and glycogen accumulation in cultured human skeletal muscle cells. PLoS ONE 7:e29985. doi: 10.1371/journal.pone.0029985
Naik, A., Rozman, D., and Belic, A. (2014). SteatoNet: the first integrated human metabolic model with multi-layered regulation to investigate liver-associated pathologies. PLoS Comput. Biol. 10:e1003993. doi: 10.1371/journal.pcbi.1003993
Nomura, D. K., Long, J. Z., Niessen, S., Hoover, H. S., Ng, S. W., and Cravatt, B. F. (2010). Monoacylglycerol lipase regulates a fatty acid network that promotes cancer pathogenesis. Cell 140, 49–61. doi: 10.1016/j.cell.2009.11.027
Norstedt, G., and Palmiter, R. (1984). Secretory rhythm of growth hormone regulates sexual differentiation of mouse liver. Cell 36, 805–812. doi: 10.1016/0092-8674(84)90030-8
Oshida, K., Waxman, D. J., and Corton, J. C. (2016). Correction: chemical and hormonal effects on STAT5b- dependent sexual dimorphism of the liver transcriptome. PLoS ONE 11:e0161519. doi: 10.1371/journal.pone.0161519
Pan, J. J., and Fallon, M. B. (2014). Gender and racial differences in nonalcoholic fatty liver disease. World J. Hepatol. 6:274. doi: 10.4254/wjh.v6.i5.274
Petta, S., Valenti, L., Bugianesi, E., Targher, G., Bellentani, S., and Bonino, F. (2016). A “systems medicine” approach to the study of non-alcoholic fatty liver disease. Digest. Liver Dis. 48, 333–342. doi: 10.1016/j.dld.2015.10.027
Rando, G., and Wahli, W. (2011). Sex differences in nuclear receptor-regulated liver metabolic pathways. Biochim. Biophys. Acta 1812, 964–973. doi: 10.1016/j.bbadis.2010.12.023
Reingold, J. S., McGavock, J. M., Kaka, S., Tillery, T., Victor, R. G., and Szczepaniak, L. S. (2005). Determination of triglyceride in the human myocardium by magnetic resonance spectroscopy: reproducibility and sensitivity of the method. Am. J. Physiol. Endocrinol. Metab. 289, E935–E939. doi: 10.1152/ajpendo.00095.2005
Rodríguez, A., Marinelli, R. A., Tesse, A., Frühbeck, G., and Calamita, G. (2015). Sexual dimorphism of adipose and hepatic aquaglyceroporins in health and metabolic disorders. Front. Endocrinol. 6:171. doi: 10.3389/fendo.2015.00171
Roepstorff, C., Donsmark, M., Thiele, M., Vistisen, B., Stewart, G., Vissing, K., et al. (2006). Sex differences in hormone-sensitive lipase expression, activity, and phosphorylation in skeletal muscle at rest and during exercise. Am. J. Physiol. Endocrinol. Metab. 291, E1106–E1114. doi: 10.1152/ajpendo.00097.2006
Sanders, F. W., and Griffin, J. L. (2016). De novo lipogenesis in the liver in health and disease: more than just a shunting yard for glucose. Biol. Rev. 91, 452–468. doi: 10.1111/brv.12178
Schultz, J. R., Tu, H., Luk, A., Repa, J. J., Medina, J. C., Li, L., et al. (2000). Role of LXRs in control of lipogenesis. Gene. Dev. 14, 2831–2838.
Serviddio, G., Bellanti, F., and Vendemiale, G. (2013). Free radical biology for medicine: learning from nonalcoholic fatty liver disease. Free Radic. Biol. Med. 65, 952–968. doi: 10.1016/j.freeradbiomed.2013.08.174
Shanle, E. K., and Xu, W. (2010). Endocrine disrupting chemicals targeting estrogen receptor signaling: identification and mechanisms of action. Chem. Res. Toxicol. 24, 6–19. doi: 10.1021/tx100231n
Sharma, G., Mauvais-Jarvis, F., and Prossnitz, E. R. (2017). Roles of G protein-coupled estrogen receptor GPER in metabolic regulation. J. Steroid Biochem. Mol. Biol. 176, 31–37. doi: 10.1016/j.jsbmb.2017.02.012
Simpson, E. (2003). Sources of estrogen and their importance. J. Steroid Biochem. Mol. Biol. 86, 225–230. doi: 10.1016/S0960-0760(03)00360-1
Söder, O. (2007). Sexual dimorphism of gonadal development. Best Pract. Res. Clin. Endocrinol. Metab. 21, 381–391. doi: 10.1016/j.beem.2007.05.002
Softic, S., Cohen, D. E., and Kahn, C. R. (2016). Role of dietary fructose and hepatic de novo lipogenesis in fatty liver disease. Dig. Dis. Sci. 61, 1282–1293. doi: 10.1007/s10620-016-4054-0
Sos, B. C., Harris, C., Nordstrom, S. M., Tran, J. L., Balázs, M., Caplazi, P., et al. (2011). Abrogation of growth hormone secretion rescues fatty liver in mice with hepatocyte-specific deletion of JAK2. J. Clin. Invest. 121, 1412–1423. doi: 10.1172/JCI42894
Suzuki, A., and Abdelmalek, M. F. (2009). Nonalcoholic fatty liver disease in women. Womens Health 5, 1–13. doi: 10.2217/17455057.5.2.191
Urlep, Ž., Lorbek, G., Perše, M., Jeruc, J., Juvan, P., Matz-Soja, M., et al. (2017). Disrupting hepatocyte Cyp51 from cholesterol synthesis leads to progressive liver Injury in the developing mouse and decreases RORC signalling. Sci. Rep. 7:40775. doi: 10.1038/srep40775
Villa, A., Della Torre, S., Stell, A., Cook, J., Brown, M., and Maggi, A. (2012). Tetradian oscillation of estrogen receptor α is necessary to prevent liver lipid deposition. Proc. Natl. Acad. Sci. U.S.A. 109, 11806–11811. doi: 10.1073/pnas.1205797109
Wang, X., Magkos, F., and Mittendorfer, B. (2011). Sex differences in lipid and lipoprotein metabolism: it's not just about sex hormones. J. Clin. Endocrinol. Metab. 96, 885–893. doi: 10.1210/jc.2010-2061
Waxman, D. J., and Holloway, M. G. (2009). Sex differences in the expression of hepatic drug metabolizing enzymes. Mol. Pharmacol. 76, 215–228. doi: 10.1124/mol.109.056705
Waxman, D. J., and O'connor, C. (2006). Growth hormone regulation of sex-dependent liver gene expression. Mol. Endocrinol. 20, 2613–2629. doi: 10.1210/me.2006-0007
Westerhoff, H. V., Groen, A. K., and Wanders, R. J. (1984). Modern theories of metabolic control and their applications. Biosci. Rep. 4, 1–22. doi: 10.1007/BF01120819
Xu, C., Yu, C., Ma, H., Xu, L., Miao, M., and Li, Y. (2013). Prevalence and risk factors for the development of nonalcoholic fatty liver disease in a nonobese Chinese population: the Zhejiang Zhenhai Study. Am. J. Gastroenterol. 108, 1299–1304. doi: 10.1038/ajg.2013.104
Yang, X., Schadt, E. E., Wang, S., Wang, H., Arnold, A. P., Ingram-Drake, L., et al. (2006). Tissue-specific expression and regulation of sexually dimorphic genes in mice. Genome Res. 16, 995–1004. doi: 10.1101/gr.5217506
Zhang, Y., Laz, E. V., and Waxman, D. J. (2012). Dynamic, sex-differential STAT5 and BCL6 binding to sex-biased, growth hormone-regulated genes in adult mouse liver. Mol. Cell Biol. 32, 880–896. doi: 10.1128/MCB.06312-11
Zheng, B., Zhu, Y. J., Wang, H. Y., and Chen, L. (2017). Gender disparity in hepatocellular carcinoma (HCC): multiple underlying mechanisms. Sci. China Life Sci. 60, 575–584. doi: 10.1007/s11427-016-9043-9
Zhu, W., Zhao, Y., Zhou, J., Wang, X., Pan, Q., Zhang, N., et al. (2016). Monoacylglycerol lipase promotes progression of hepatocellular carcinoma via NF-κB-mediated epithelial-mesenchymal transition. J. Hematol. Oncol. 9:127. doi: 10.1186/s13045-016-0361-3
Keywords: sexual dimorphism, hepatic metabolism, systems medicine, large-scale metabolic model, NAFLD, liver
Citation: Cvitanović Tomaš T, Urlep Ž, Moškon M, Mraz M and Rozman D (2018) LiverSex Computational Model: Sexual Aspects in Hepatic Metabolism and Abnormalities. Front. Physiol. 9:360. doi: 10.3389/fphys.2018.00360
Received: 15 December 2017; Accepted: 22 March 2018;
Published: 12 April 2018.
Edited by:
Rolf Gebhardt, Leipzig University, GermanyReviewed by:
Manlio Vinciguerra, International Clinical Research Center (FNUSA-ICRC), CzechiaShikha Prasad, Northwestern University, United States
Copyright © 2018 Cvitanović Tomaš, Urlep, Moškon, Mraz and Rozman. This is an open-access article distributed under the terms of the Creative Commons Attribution License (CC BY). The use, distribution or reproduction in other forums is permitted, provided the original author(s) and the copyright owner are credited and that the original publication in this journal is cited, in accordance with accepted academic practice. No use, distribution or reproduction is permitted which does not comply with these terms.
*Correspondence: Damjana Rozman, damjana.rozman@mf.uni-lj.si