- 1Department of Bioengineering, Faculty of Engineering, Ege University, Bornova-Izmir, Turkey
- 2Department of Bioengineering, Rice University, Houston, TX, United States
- 3Biomaterials Innovation Research Center, Division of Biomedical Engineering, Department of Medicine, Brigham and Women's Hospital, Harvard Medical School, Boston, MA, United States
- 4Harvard-MIT Division of Health Sciences and Technology, Cambridge, MA, United States
The most lethal form of brain cancer, glioblastoma multiforme, is characterized by rapid growth and invasion facilitated by cell migration and degradation of the extracellular matrix. Despite technological advances in surgery and radio-chemotherapy, glioblastoma remains largely resistant to treatment. New approaches to study glioblastoma and to design optimized therapies are greatly needed. One such approach harnesses computational modeling to support the design and delivery of glioblastoma treatment. In this paper, we critically summarize current glioblastoma therapy, with a focus on emerging nanomedicine and therapies that capitalize on cell-specific signaling in glioblastoma. We follow this summary by discussing computational modeling approaches focused on optimizing these emerging nanotherapeutics for brain cancer. We conclude by illustrating how mathematical analysis can be used to compare the delivery of a high potential anticancer molecule, delphinidin, in both free and nanoparticle loaded forms across the blood-brain barrier for glioblastoma.
Introduction
Glioblastoma is the most common primary malignant form of brain cancer, with a median survival of 7–15 months from the time of diagnosis. Hallmarks of the aggressive cancer include extensive infiltration and strong vascular proliferation into the surrounding brain parenchyma (Wei et al., 2014; Kim et al., 2015; Van Tellingen et al., 2015). Conventional therapy for glioblastoma, tumor resection followed by radiotherapy and chemotherapy [typically temozolomide (TMZ)], is limited in efficacy due to high rates of recurrence, overall resistance to therapy, and devastating neurological deterioration (Kim et al., 2015; Lin et al., 2015).
Over the past decade, there has been an explosion of research on glioblastoma, with thousands of published reports related to prognosis, treatment response and treatment targets. However, few studies have led to changes in patient outcome (Huang et al., 2013a; Hanada et al., 2014; Thuy et al., 2015). A new approach to brain cancer translational studies is greatly needed to address the overwhelmingly poor treatment results for patients currently diagnosed with glioblastoma. One emerging and promising avenue is nanotechnology based drug delivery (Dilnawaz and Sahoo, 2013; Thuy et al., 2015).
Nanotechnology helps address a major hurdle of glioma therapy: delivery of active compounds to brain tissue. Therapeutics delivery to the brain is limited and controlled by the presence of the blood-brain barrier (BBB), which blocks toxins as well as many essential drugs from reaching brain tissue (Bicker et al., 2014; Kim et al., 2015). The BBB represents a physical interface in the central nervous system (CNS) between the blood and neural tissue (Figure 1). The BBB is comprised of endothelial cells, astrocytes' end-feet, pericytes, and adjacent neurons. The unique interactions of tightly connected endothelial cells and astrocytes maintains the integrity of the BBB, the expression of tight junction proteins, and the prevention of paracellular diffusion (Grover et al., 2014). The BBB also actively functions to exclude substrates from cells through efflux proteins, including ATP-binding cassette transporters and p-glycoprotein (p-gp) (Demeule et al., 2002). The 170 kDa p-gp protein, located at the luminal surface of the brain microvascular endothelium, acts as a “brain gatekeeper” by actively transporting proteins out of the brain capillaries (Figure 1). Those compounds that escape p-gp, must also be lipid soluble small molecules, electro-neutral molecules, or nutrients under 400–600 Da in order to diffuse passively across the BBB's endothelial cell membrane. As such, an estimated 99% of drugs in development fail to cross the BBB, and this severely limits the number of neurological therapies. FDA-approved drugs that effectively cross the barrier are only available for a subset of neurological diseases such as depression, affective disorders, chronic pain, and epilepsy (Aryal et al., 2014; Cheng et al., 2014; Timbie et al., 2015). Advanced delivery methods and disruption of the BBB with nanomedicine techniques are approaches that can help address the bottleneck of brain drug delivery for glioblastoma. However, to capitalize on nanomedicine's benefits, the size, shape, and other nanoparticle delivery properties need to be optimized. To that end, computational models can provide a better understanding of the complex processes involved in delivering effective glioblastoma therapies, and to examine molecular-level interactions in a systemic way to increase therapeutic efficiency (Kim et al., 2013; Matsson and Bergström, 2015).
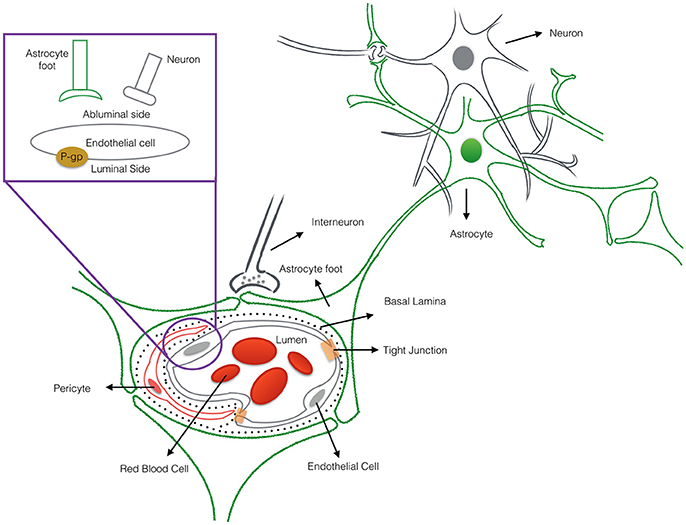
Figure 1. The cross-sectional view of the blood-brain barrier. The blood-brain barrier (BBB) is a physical interface formed by cerebral endothelial cells, separated from pericytes and astrocytic end-feet by the basal lamina. The interactions of the endothelial cells and astrocytes maintaining the integrity of the BBB. Routes for molecular transport across the BBB are not depicted except energy-dependent transport protein (p-glycprotein) which acts as an efflux transporter.
In this paper, we will briefly summarize milestones that led to the existing therapies in glioblastoma treatment (Figure 2), before reviewing novel experimental and computational approaches to optimizing targeted delivery of active nanoparticles to glioblastoma through the BBB.
Current Therapies for Glioblastoma Therapy
Surgery, Radiotherapy, Anti-angiogenic Therapy and Precision Chemotherapy
The standard treatment for glioblastoma is surgery. This approach includes consideration of maximum surgical resection of tumor tissue even if the entire tumor cannot be removed (Salcman, 1988; Quigley and Maroon, 1991). Even when possible, surgical resection in the case of glioblastoma is limited by the aggressiveness of the glioblastoma which is characterized by infiltration into surrounding tissue and extensive tumor vascularization (Alifieris and Trafalis, 2015; Séhédic et al., 2015). Hence resection surgery is coupled to a course of drug and/or radiation therapy. Chemotherapy for glioblastoma has evolved since the 1970s when researchers first reported data on the efficacy of carmustine (BCNU), a compound which is able to cross the BBB and attack glioma cells directly (Wilson, 1976; Walker et al., 1980). In 1979, Salazar and colleagues proved that radiation therapy is effective against brain cancers (Salazar et al., 1979). Shortly after radiation therapy was developed for treatment in glioblastoma, researchers showed that combining chemotherapy with radiation therapy helps patients live longer (Fine et al., 1993). Today, standard therapy for patients with glioblastoma is a combination treatment, including radiotherapy alone or with chemotherapy, both before and after surgery. Chemotherapy may also be used to delay the need for radiation in younger children.
The choice of drug therapy for glioblastoma is still limited to a handful of compounds. In 1999, Temozolomide, the oral chemotherapy drug was granted accelerated approval by the FDA to treat anaplastic astrocytoma (a form of high-grade glioma) (Yung et al., 1999; Brada et al., 2001). Currently, Temzolomide is the preferred FDA-approved chemotherapeutic agent for glioblastoma (Alifieris and Trafalis, 2015; Frosina, 2015). One feature enabling its success is that Temozolomide treatment can be tailored based on patient characteristics. Researchers discovered that patients with promoter MGMT gene methylation have increased median survival times when given Temozolomide with radiotherapy therapy vs. radiotherapy alone. The MGMT gene enables DNA repair, even where damage was caused by chemotherapy (Hegi et al., 2005; Alifieris and Trafalis, 2015).
The use of Temozolomide therapy for patients with MGMT gene methylation highlights the importance of another growth area in glioblastoma clinical research: increasingly precise classification of tumors and patients. While drugs have been in development, so too have methods to classify and grade brain tumors. The World Health Organization (WHO) developed international standards for classifying brain and nervous system tumors in the 1990s (The new WHO Classification of Tumors affecting the Central Nervous System, 1993). Experts continue to update tumor classifications according to the cell type and tumor malignancy grade as scientific knowledge grows and new glioma subtypes are identified. These classification standards allow doctors and researchers to have a common language for describing and sharing knowledge about tumor stage, characterization, genetics, and treatment.
To help improve predictions of response to therapy, many researchers have searched for genetic cues in glioblastoma patients. The National Cancer Institute and The National Genome Research Institute launched The Cancer Genome Atlas Project in 2005, with the aim of mapping the genetic changes in glioblastoma and other cancers. In 2008, research studies reported the identification of several key mutations that are involved in inducing the development and invasiveness of glioblastoma (TCGA, 2008). In 2010, a set of nine genes were identified to predict the likelihood that a glioblastoma tumor would respond to therapy. The multivariate diagnostic test called DecisionDx-GBM, was created to determine the molecular signature of glioblastoma tumors and help identify the most effective existing therapy and/or suggest new treatments targeting tumors that do not respond to the standard therapies (Colman et al., 2010).
Building on important progress in understanding the molecular pathogenesis of malignant glioblastoma (McLendon et al., 2008; Wen and Kesari, 2008), we summarize known gene interactions and protein expression patterns in glioblastoma through a signaling network diagram representation (Figures 3, 4). Specifically, we analyzed aberrations in signaling pathways for glioblastoma cells compared to four different types of healthy cerebral cortex cells. Glioblastoma signaling pathway map served as our default template (“The Cancer Genomics at cBio—Glioblastoma (TCGA)”1 n.d.) and networks of related differentially expressed genes were rendered using Cytoscape. We extended and supplemented the original TCGA pathway map using protein expression levels of tumor cell and healthy cerebral cortex cells obtained from The Human Protein Atlas (Table 1). Each protein symbol is divided into quarters to represent, in a counterclockwise order: (1) endothelial cells, (2) glial cells, (3) neuronal cells, and (4) neuropil of the healthy cerebral cortex; the corresponding protein expression levels are shown in different colors (Figure 3). The analogous protein expression levels for glioma cancer cells are depicted as well (Figure 4). Proteins that are differentially expressed in glioma compared to the healthy cortex are highlighted in the context of known molecular signaling pathways. Recent studies have shown promising results of targeting one of the displayed over-expressed receptors using a nanodelivery system to the glioblastoma tumor (Krakstad and Chekenya, 2010; Qin et al., 2014; Weber and Ryan, 2015; Whittle et al., 2015). Future drug development can capitalize on these and similar analyses of known signaling mechanisms in glioma in order to help develop cell- and patient-specific targeted therapies.
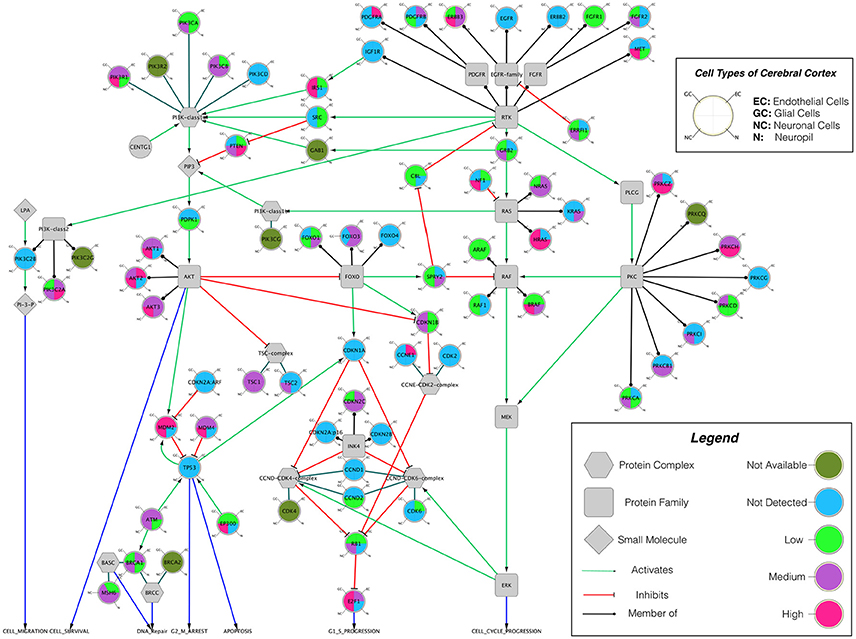
Figure 3. The protein expression levels of healthy cerebral cortex cells mapped onto the GBM pathway map from the TCGA data set. In this diagram, The Human Protein Atlas database was used to obtain protein expression levels of healthy cerebral cortex cells. Special shapes used in the map represent different types of molecules which are given in the legend of the figure as protein complex, protein family, protein or small molecule. Lines and arrows show the relationship of the molecules. Each protein symbol (circle) is divided into quarters to represent, in a clockwise order: endothelial cells, neuropil, neuronal cells, and glial cells. The corresponding protein expression levels are shown in different colors. Olive for not available, deep sky blue for not detected, green for low expression, medium orchid for medium expression, and deep pink for high expression. The original GBM pathway map in the Cytoscape format was downloaded from (“The Cancer Genomics at cBio—Glioblastoma (TCGA)” n.d.).
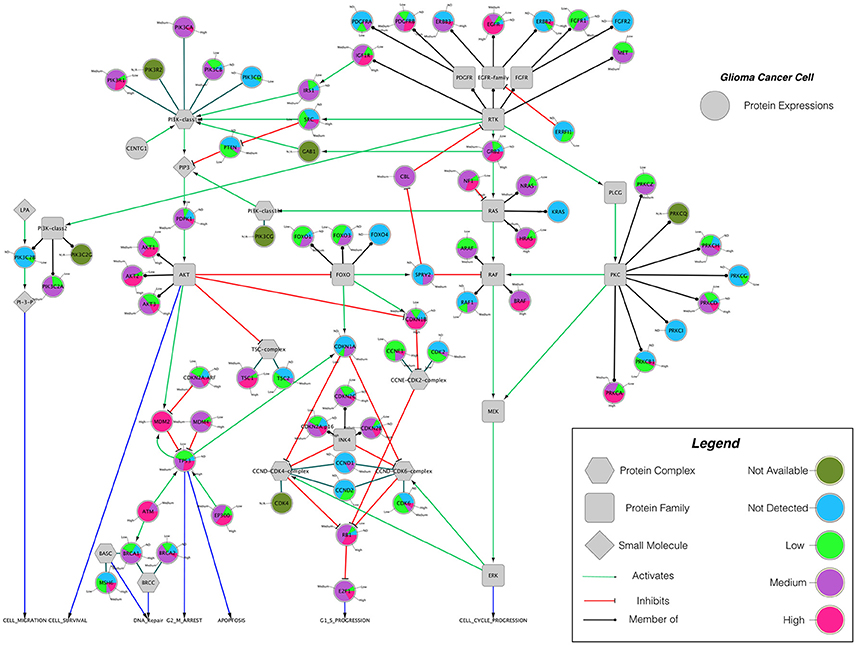
Figure 4. The protein expression levels of glioma cancer cells mapped onto the GBM pathway map from the TCGA data set. In this diagram, The Human Protein Atlas database were used to obtain protein expression levels of glioma cancer cells. Special shapes used in the map represent different types of molecules which are given in the legend of the figure as protein complex, protein family, protein and small molecule, respectively. Lines and arrows show the relationship of the molecules. Each protein symbol (circle) is shown as pie chart to represent different expression levels of glioma cancer cell which are depicted in different colors. Olive for not available, deep sky blue for not detected, green for low expression, medium orchid for medium expression, and deep pink for high expression. The original GBM pathway map in the Cytoscape format was downloaded from (“The Cancer Genomics at cBio—Glioblastoma (TCGA)” n.d.).
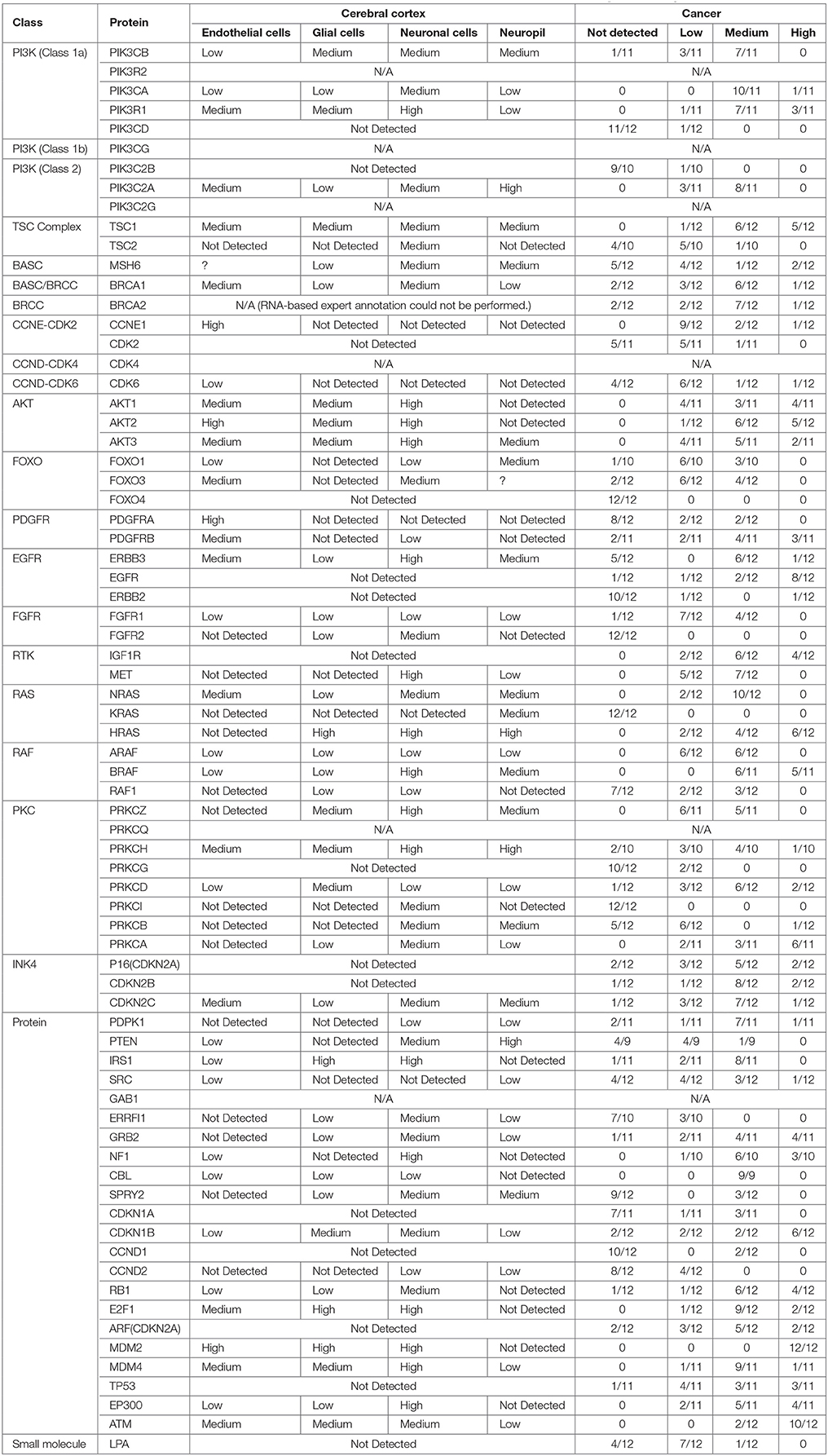
Table 1. Protein classes, names and expression levels in the cerebral cortex and brain tumor which is obtained from www.proteinatlas.org.
Ongoing research into angiogenesis also has offered hopeful glioblastoma targets based on the hypothesis that cutting off a tumor's blood supply could starve growing cancer cells. Bevacizumab (Avastin), a drug targeting vascular endothelial growth factor (VEGF-A), was suggested as an early-stage trial of targeted therapy for brain cancer. Avastin is an anti-angiogenic drug that interferes with the development of blood vessels essential to tumor growth and invasiveness (Friedman et al., 2009). The FDA granted accelerated approval for Bevacizumab, based on its efficacy in treating recurrent glioblastoma (Whittle et al., 2015). However, Bevacizumab's use has been rife with controversy. Anti-angiogenic therapy has failed to show improvement for patient overall survival, while still showing efficacy in shrinking or halting tumor growth (Francescone et al., 2012). As a result of lackluster responses by glioblastoma patients to diverse chemotherapies and anti-angiogenic compounds, radiation-combined therapies are considered essential and unavoidable. However, a drawback of radiation is its severe side effects, includes DNA lesions, cognitive impairment, and other systemic effects (Séhédic et al., 2015).
Given the limitation of all current therapeutics (whether surgery, chemotherapy and/or radiation), development of novel approaches to treating glioblastoma remains a great need. To be effective, any new therapy should be specific and controllable and should be able to cross the BBB; moreover it needs to show efficacy. A suite of new nanomedicines have emerged to fill this niche. To that end, we focus the remainder of this review on emerging nanomedicines for glioblastoma and the methods used to study them.
Nanomedicine
First a definition of what constitutes nanomedicine: Living organisms hold innate nanoscale functional components including proteins which have an average size of about 5 nm, and DNA molecules, which are on the order of 2.5 nm in diameter (Salata, 2004; Kawadkar et al., 2011). Nanotechnology methods have emerged to help understand the biological processes that occur at this nanoscale level. These tools form the basis of the nano-biotechnology field, which integrates biology, physics, and chemistry (Kawadkar et al., 2011) Application of nano-biotechnology to medicine is called nanomedicine, a subfield which has contributed to new directions in drug development, discovery, and delivery for treating malignant brain tumors (Nduom et al., 2012; Zhou et al., 2012; Aryal et al., 2014; Fakhoury, 2015; Jo et al., 2015; Lauzon et al., 2015; Fernandez-Piñeiro et al., 2017). Drug administration to or within tissues of the CNS faces significant challenges including toxicity and BBB crossing. These challenges are compounded in brain cancer by the complex tumor microenvironment, invasive tumor cells, and cancer-associated changes in metabolism (Wei et al., 2014; Séhédic et al., 2015). Nanomedicine offers a potential means to optimize delivery of drugs to brain tumors, by enabling better permeability through the BBB and specific targeting of tumor cell subtypes and of processes in the tumor microenvironment (e.g., tumor stem cells, acidosis) (Jain and Stylianopoulos, 2010; Huang et al., 2013b; Hanada et al., 2014; Wei et al., 2014). Nanoparticle-mediated delivery systems can extend the life-span of active drug compounds and provide for their controlled, continued and local release within brain tissue. To enable their efficacy, it is critical to regulate the physiochemical properties of the delivery carriers, such as their size, overall surface charge, and chemical composition. Careful analysis to determine a nanoparticle's cytotoxicity, biocompatibility, and biodegradability are all needed prior to clinical application to the brain (Jo et al., 2015; Lauzon et al., 2015). A detailed, quantitative understanding of how physicochemical properties of nanoparticles affect therapeutic delivery and efficacy is necessary to optimize the design of nanoparticles for the treatment of brain diseases. Computational modeling can address this need.
Computational Models
Many computational models have been developed to represent some aspects of glioblastoma, and the developed simulation tools can be utilized to predict tumor expansion and understand the unique tumor microenvironment (Gevertz et al., 2008). Overall, models can be classified into different categories varying from minimalistic models simulating just the growth of the tumor volume to molecular-detailed models including many genetic or proteomic processes involved in the development and progression of glioblastoma (Watanabe et al., 2016). As researchers become more aware of the complexity of the biology, modeling approaches have evolved to provide insights into glioblastoma across multiple length scales (tissue, cellular, and molecular) (Gevertz, 2011; Watanabe et al., 2016). While there is an extreme amount of diversity in the glioblastoma models' details and scope, the common aim in all models is to reliably predict certain features of tumor progression to regulate, prevent, or reverse the invasive glioma growth pattern (Gevertz et al., 2008). The more accurately this tumor growth is predicted, the more reliably therapy can be optimized for each cancer patient. Overall models have focused on three main glioma behaviors: vascularization, diffusion, and invasion capacity (Eikenberry et al., 2009; Tektonidis et al., 2011; Alfonso et al., 2016). The models consider key parameters like hypoxia which correlates strongly with glioma invasiveness and malignancy; immune response dynamics which can result in tumor regression and provide therapeutic benefits; and diffusion of therapeutic nano-particles in a three-dimensional space representing the brain's geometry and heterogeneity (Eikenberry et al., 2009; Böttger et al., 2012; Schlüter et al., 2012; Alfonso et al., 2016; Reppas et al., 2016; Watanabe et al., 2016; Rutter et al., 2017).
Computational modeling has the ability to provide predictive and explanatory frameworks for glioblastoma nanoparticle design and delivery, that in vitro and in vivo glioblastoma models lack (Bicker et al., 2014). Specifically, computational modeling can be used to study the characteristic high heterogeneity of the tumor microenvironment, and predict effects of disrupting molecular pathways in specific brain regions (Escribá et al., 2015). Molecular signaling pathway analysis like that described in the previous section can help quantitatively highlight potential cancer targets (Figures 3, 4), while mathematical models of mass transport phenomena enable predictions of drug delivery to the brain, and they can help in the design of experiments (Lauzon et al., 2015). A variety of exciting mathematical models have been developed to study and predict the progression of glioblastoma (Frieboes et al., 2013; Branco et al., 2014; Martirosyan et al., 2015), including patient-specific ones (Rockne et al., 2004; Swanson et al., 2011; Neal et al., 2013; Colombo et al., 2015).
In parallel with the increase in models of glioblastoma progression, there have been a growing number of studies focused on quantitative approaches to study and improve nanoparticle delivery to the CNS across the BBB (Huang et al., 2009). Effect of nanoparticle formulation, shape, and binding properties on delivery across the BBB has been studied previously both theoretically and in biological studies (Gosk et al., 2004; Takae et al., 2005; Chithrani et al., 2006; Decuzzi and Ferrari, 2008; Fakhari et al., 2011). There are also a few studies which show alternative access into the CNS across the epithelial blood-cerebrospinal fluid barrier (Langlet et al., 2013; Langlet, 2014). However, while diffusion-kinetic models for drug release into brain tissue have been developed for years (Saltzman and Radomsky, 1991; Bandara et al., 2007; Groh et al., 2014), no models have yet explored the optimization of novel anthocyanidin-based compounds and their CNS delivery for treatment of glioblastoma.
To address this knowledge gap, we focus the following sections on the delivery of delphinidin, an anthocyanidin and antioxidant, as a means to both (1) highlight an emerging new nanomedicine in development for glioblastoma, and (2) illustrate how mathematical analysis can be used to improve the design of delphinidin and related new compounds. A summary of presented modeling studies has been show in Table 2.
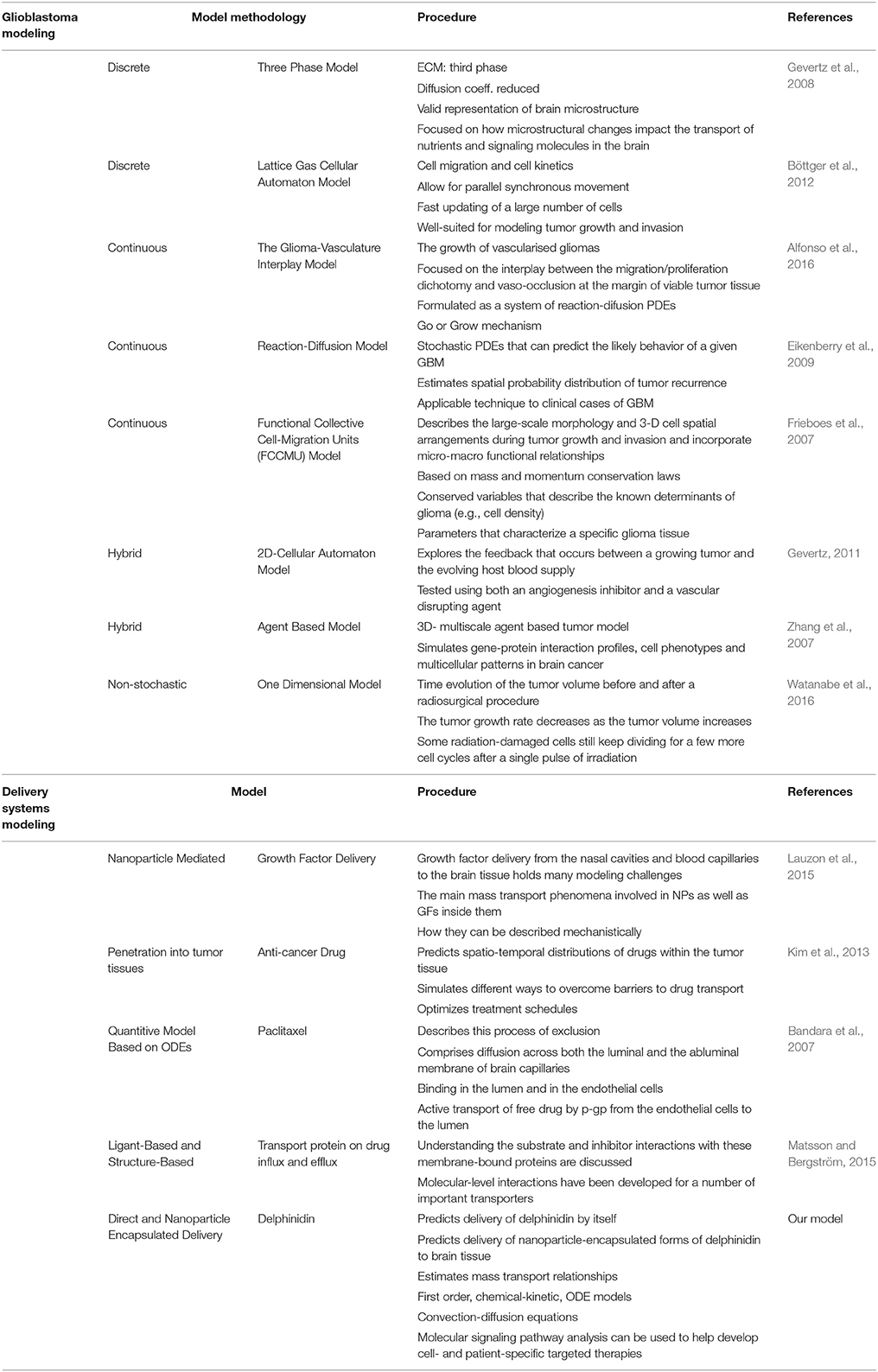
Table 2. The summary of glioblastoma modeling and delivery systems modeling (ECM, Extra cellular matrix; Coeff., Coefficient; PDE, Partial differential equations; GBM, Glioblastoma; ODE, Ordinary differential equations).
Optimizing Nanomedicine: Transport of Delphinidin Across the Blood Brain Barrier
Derivatives of the anthocyanin family are nanoparticles of great recent interest for treating multiple diseases (Amin et al., 2017; Kim et al., 2017). Antioxidant properties of anthocyanin active molecules, such as delphinidin synthesized from berries, provide potency across diseases: cited health benefits include reduced risk of coronary heart disease, reduced risk of stroke, anti-tumor properties, anti-inflammatory effects, and improved cognitive behavior (Martins et al., 2011; Chakrabarti and Ray, 2015). Notable for applications to glioma, studies have shown anthocyanins selectively inhibit the growth of human tumor cells while enabling normal growth of healthy cells (Galvano et al., 2004). Furthermore, delphinidin can cross the BBB and is taken up by brain tissue, in a process mediated by p-gp efflux (Andres-Lacueva et al., 2005; Chakrabarti and Ray, 2015).
To systematically test the effects of different anthocyanin derivatives on glioblastoma progression, we developed a computational model for the delivery of direct and encapsulated forms of delphinidin to glioblastoma through the BBB. Specifically, we developed two models: (1) to predict delivery of delphinidin by itself and (2) to predict delivery of nanoparticle-encapsulated forms of delphinidin to brain tissue. In both cases, we can estimate mass transport relationships. In the first model, we need four aggregated kinetic terms (diffusivity, binding, dissociation, and active transport) that enable us to define the following processes: (1) passive diffusion across the luminal and the abluminal membrane of brain capillaries, (2) active transport of delphinidin from the endothelial cells to the vascular lumen by p-gp, and (3) release of the compound by control of p-gp activity. In the nanoparticle delivery model, we instead include (1) transport of the nanoparticles from the blood stream through the BBB into the brain parenchyma; and (2) release of the delphinidin from the nanoparticles.
Briefly, these models take the form of first order, chemical-kinetic, ordinary differential equation models. Convection-diffusion equations define the change in concentration of the delphinidin (1) and delphinidin-encapsulated nanoparticle (2) in the blood stream, and they are quantified in two compartments: in the lumen and in the endothelial cells of the brain capillaries. The right-hand side of the 1st equation includes three terms as diffusion, convection and creation or destruction of the quantity. Where ∇ is spatial gradient operator, ϑ is the velocity field that represents the convection or advection and R is the sources or sinks of the quantity C. The concentration in the lumen (L) and endothelial cell cytoplasm (E) of both free delphinidin (CL,1, CE,1) and delphinidin-encapsulated nanoparticles (CL,2, CE,2), respectively, were all considered. The concentration of the compound in the whole brain, CM, was considered constant. In the human brain, the total length of all capillaries is estimated to l = 600 km (Bandara et al., 2007). In our model, the geometry of the single-tube like capillary is shown in Figure 5, and its dimensions are defined by the luminal radius rL and the endothelial outer radius rE, the lumenal volume VL, the endothelial volume VE, the surface area between lumen and endothelia ALE, and the outer surface area of the capillary AEM. It is assumed that the transport by diffusion occurs across membranes only, and this is modeled between the vascular lumen and the cytoplasm of endothelial cells, and between endothelial cells and the surrounding brain tissue by the following equations:
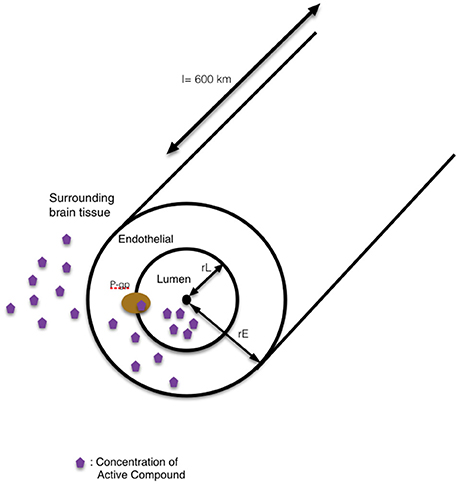
Figure 5. The schematic representation of our single-tube like capillary model. In this 1D model, the blood-brain barrier is simplified into a single blood vessel with a lumen inside and surrounding tissue outside. The wall of the vessel is represented as the endothelial side. The route for the transport of compound into the surrounding brain tissue by modulation of pgp-mediated efflux is shown in the schematic diagram.
Different diffusion coefficients for the transport across each membrane, DLE1, DLE2 and DEM, were defined as model parameters based on typical lipid composition in the membrane and speed of diffusion (Table 3).
Binding and release, as well as active transport was modeled by linear chemical-kinetic relationships with the following equation:
where Cu* = the concentration of delphinidin-encapsulated nanoparticle which are taken up by the efflux transporter p-gp, C* = the concentration of released delphinidin-encapsulated nanoparticle, k1 = the rate of release of delphinidin from endothelial cells, k2 = the rate of p-gp uptake of delphinidin from endothelial cells. The model was implemented in the MATLAB. The full set of equations and MATLAB code are provided in the supplementary material.
When free delphinidin is encapsulated into a nanoparticle, the diffusion coefficient changes, and Figure 6 shows the predicted tissue concentration distributions from Equations (3–6) for the delphinidin free and encapsulated forms, using the parameters summarized in Table 3. To represent how encapsulation might cause effective diffusion into the brain, the free delphinidin diffusion coefficient was estimated for this system. As seen from the graph, our model predicts the distribution of delphinidin in its free and encapsulated forms. The maximum concentration of delphinidin in the brain is reached ~50.1 min following injection, a value obtained from solving Equation (7). Validation of the model is currently infeasible for humans, however future in vivo assays are merited to test these predictions and build on the quantitative theory.
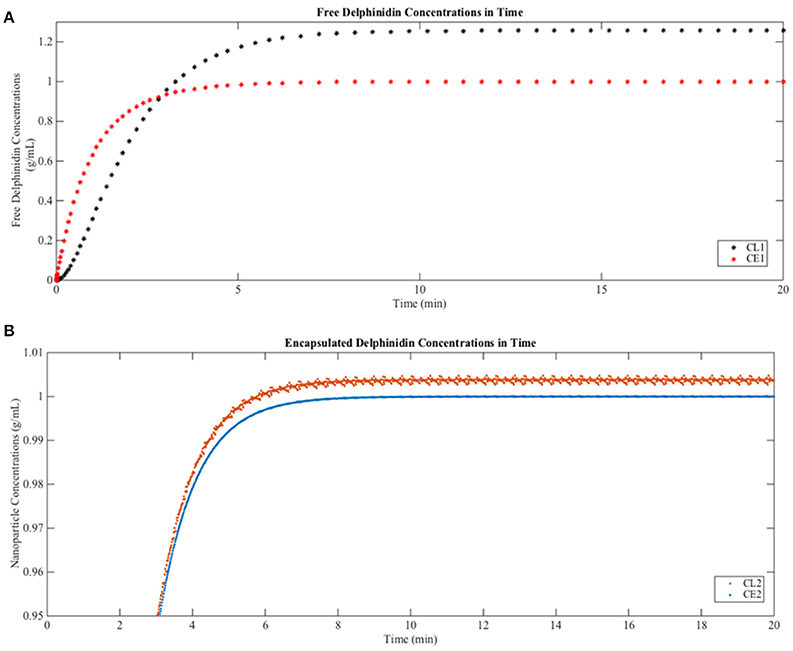
Figure 6. Predictions of free and encapsulated delphinidin delivery to brain tissue (A) Free delphinidin concentration vs. time graph where y-axis 0–1.3. (B) Encapsulated delphinidin concentration vs. time graph where y-axis 0.95–1.01. CL, concentration in the lumen; CE, concentration in the endothelial cells of the barrier.
We introduced this model to understand the transport of delphinidin in comparison to delphinidin-encapsulated nanoparticles across the BBB quantitatively, which is controlled by diffusion, active transport, and p-gp binding. It is important to emphasize that all models are idealized representations of reality, and the models described here are significant simplifications of complex biology and geometry. Hereby, adjusting nanoparticle shape, size, and other properties could result in avoiding p-gp blocking at the BBB and successfully passing through the membrane for the therapy of glioblastoma with delphinidin. While building from a similar foundation, future models of nanomedicine delivery may consider many of the other microenvironmental and cellular factors involved in effective brain tumor-targeted drug delivery (Liu and Lu, 2012). Along with the BBB, other major membranes which impose obstacles to brain tumor treatment include the blood-cerebrospinal fluid barrier (Béduneau et al., 2007) and blood-tumor barrier (Van Tellingen et al., 2015). Additionally, an enhanced permeability and retention (EPR) effect (Torchilin, 2011) could be incorporated in subsequent models to optimize predictions for the delivery of new therapeutic agents. In vitro experiments to estimate kinetic rates can be used to validate and/or improve parameters of the models and include more complex terms like possible interactions of the nanoparticles with local tissue (adsorption/ desorption), nanoparticle swelling and erosion.
Conclusion
This review summarizes examples of current advances in the glioblastoma therapy to increase our understanding of molecular mechanisms underlying glioma progression and explore the potential of new nanomedicines. We have highlighted the use of two systems biology tools, network signaling diagrams, and mathematical models, in distinguishing differential glioma cell signaling and in predicting delivery of delphinidin to brain tissue. In this regard, quantitative tools open up a new avenue for glioblastoma research, and provide an essential method to explore the potential of nanomedicine in brain cancer treatment.
Author Contributions
EO-K and AQ: Drafted the manuscript; EO-K: Design and made the figures and tables. All authors read and revised the manuscript and approved its content.
Funding
This research did not receive any specific grant from funding agencies in the public, commercial, or not-for-profit sectors.
Conflict of Interest Statement
The authors declare that the research was conducted in the absence of any commercial or financial relationships that could be construed as a potential conflict of interest.
Acknowledgments
The authors are grateful for access to the Qutub Lab of the Department of Bioengineering at Rice University.
Supplementary Material
The Supplementary Material for this article can be found online at: https://www.frontiersin.org/articles/10.3389/fphys.2018.00170/full#supplementary-material
Footnotes
1. ^CancerGenomics at cBio - Glioblastoma (TCGA) [WWW Document], n.d. URL Available online at: http://cbio.mskcc.org/research/cancergenomics/gbm/pathways/index.html (Accessed February 28, 2018).
References
Alfonso, J. C., Köhn-Luque, A., Stylianopoulos, T., Feuerhake, F., Deutsch, A., and Hatzikirou, H. (2016). Why one-size-fits-all vaso-modulatory interventions fail to control glioma invasion: in silico insights. Sci. Rep. 6:37283. doi: 10.1038/srep37283
Alifieris, C., and Trafalis, D. T. (2015). Glioblastoma multiforme: pathogenesis and treatment. Pharmacol. Ther. 152, 63–82. doi: 10.1016/j.pharmthera.2015.05.005
Amin, F. U., Shah, S. A., Badshah, H., Khan, M., and Kim, M. O. (2017). Anthocyanins encapsulated by PLGA@PEG nanoparticles potentially improved its free radical scavenging capabilities via p38/JNK pathway against Aβ1–42-induced oxidative stress. J. Nanobiotechnol. 15, 12. doi: 10.1186/s12951-016-0227-4
Andres-Lacueva, C., Shukitt-Hale, B., Galli, R. L., Jauregui, O., Lamuale-Raventos, R. M., and Joseph, J. A. (2005). Anthocyanins in aged blueberry-fed rats are found centrally and may enhance memory. Nutr. Neurosci. 8, 111–120. doi: 10.1080/10284150500078117
Aryal, M., Arvanitis, C. D., Alexander, P. M., and McDannold, N. (2014). Ultrasound-mediated blood-brain barrier disruption for targeted drug delivery in the central nervous system. Adv. Drug Deliv. Rev. 72, 94–109. doi: 10.1016/j.addr.2014.01.008
Bandara, S., Diehl, M., and Fricker, G. (2007). Mathematical model for the transport of Paclitaxel (Taxol) across the blood-brain barrier. Chem. Eng. Res. Des. 85, 1065–1071. doi: 10.1205/cherd06238
Béduneau, A., Saulnier, P., and Benoit, J. P. (2007). Active targeting of brain tumors using nanocarriers. Biomaterials 28, 4947–4967. doi: 10.1016/j.biomaterials.2007.06.011
Bicker, J., Alves, G., Fortuna, A., and Falcão, A. (2014). Blood-brain barrier models and their relevance for a successful development of CNS drug delivery systems: a review. Eur. J. Pharm. Biopharm. 87, 409–432. doi: 10.1016/j.ejpb.2014.03.012
Böttger, K., Hatzikirou, H., Chauviere, A., and Deutsch, A. (2012). Investigation of the migration/proliferation dichotomy and its impact on avascular glioma invasion. Math. Model. Nat. Phenom. 7, 105–135. doi: 10.1051/mmnp/20127106
Brada, M., Hoang-Xuan, K., Rampling, R., Dietrich, P. Y., Dirix, L. Y., Macdonald, D., et al. (2001). Multicenter phase II trial of temozolomide in patients with glioblastoma multiforme at first relapse. Ann. Oncol. 12, 259–266. doi: 10.1023/A:1008382516636
Branco, J. R., Ferreira, J. A., and de Oliveira, P. (2014). Mathematical modeling of efficient protocols to control glioma growth. Math. Biosci. 255, 83–90. doi: 10.1016/j.mbs.2014.07.002
Chakrabarti, M., and Ray, S. K. (2015). Direct transfection of miR-137 mimics is more effective than DNA demethylation of miR-137 promoter to augment anti-tumor mechanisms of delphinidin in human glioblastoma U87MG and LN18 cells. Gene 573, 141–152. doi: 10.1016/j.gene.2015.07.034
Cheng, Y., Morshed, R. A., Auffinger, B., Tobias, A. L., and Lesniak, M. S. (2014). Multifunctional nanoparticles for brain tumor imaging and therapy. Adv. Drug Deliv. Rev. 66, 42–57. doi: 10.1016/j.addr.2013.09.006
Chithrani, B. D., Ghazani, A. A., and Chan, W. C. (2006). Determining the size and shape dependence of gold nanoparticle uptake into mammalian cells. Nano Lett. 6, 662–668. doi: 10.1021/nl052396o
Colman, H., Zhang, L., Sulman, E. P., McDonald, J. M., Shooshtari, N. L., Rivera, A., et al. (2010). A multigene predictor of outcome in glioblastoma. Neuro. Oncol. 12, 49–57. doi: 10.1093/neuonc/nop007
Colombo, M. C., Giverso, C., Faggiano, E., Boffano, C., Acerbi, F., and Ciarletta, P. (2015). Towards the personalized treatment of glioblastoma: integrating patient-specific clinical data in a continuous mechanical model. PLoS ONE 10:e0132887. doi: 10.1371/journal.pone.0132887
Decuzzi, P., and Ferrari, M. (2008). The receptor-mediated endocytosis of nonspherical particles. Biophys. J. 94, 3790–3797. doi: 10.1529/biophysj.107.120238
Demeule, M., Régina, A., Jodoin, J., Laplante, A., Dagenais, C., Berthelet, F., et al. (2002). Drug transport to the brain: key roles for the efflux pump P-glycoprotein in the blood-brain barrier. Vascul. Pharmacol. 38, 339–348. doi: 10.1016/S1537-1891(02)00201-X
Dilnawaz, F., and Sahoo, S. K. (2013). Enhanced accumulation of curcumin and temozolomide loaded magnetic nanoparticles executes profound cytotoxic effect in glioblastoma spheroid model. Eur. J. Pharm. Biopharm. 85, 452–462. doi: 10.1016/j.ejpb.2013.07.013
Dunn, S., Constantinides, A., and Moghe, P. V. (2006). Numerical Methods in Biomedical Engineering. New York, NY: Academic Press; Elsevier.
Eikenberry, S. E., Sankar, T., Preul, M. C., Kostelich, E. J., Thalhauser, C. J., and Kuang, Y. (2009). Virtual glioblastoma: growth, migration and treatment in a three-dimensional mathematical model. Cell Prolif. 42, 511–528. doi: 10.1111/j.1365-2184.2009.00613.x
Escribá, P. V., Busquets, X., Inokuchi, J. I., Balogh, G., Török, Z., Horváth, I., et al. (2015). Membrane lipid therapy: Modulation of the cell membrane composition and structure as a molecular base for drug discovery and new disease treatment. Prog. Lipid Res. 59, 38–53. doi: 10.1016/j.plipres.2015.04.003
Evans, C. J., Phillips, R. M., Jones, P. F., Loadman, P. M., Sleeman, B. D., Twelves, C. J., et al. (2009). A mathematical model of doxorubicin penetration through multicellular layers. J. Theor. Biol. 257, 598. doi: 10.1016/j.jtbi.2008.11.031
Fakhari, A., Baoum, A., Siahaan, T. J., Le, K. B., and Berkland, C. (2011). Controlling ligand surface density optimizes nanoparticle binding to ICAM-1. J. Pharm. Sci. 100, 1045–1056. doi: 10.1002/jps.22342
Fakhoury, M. (2015). Drug delivery approaches for the treatment of glioblastoma multiforme. Artif. Cells Nanomed. Biotechnol. 1401, 1–9. doi: 10.3109/21691401.2015.1052467
Fernandez-Piñeiro, I., Badiola, I., and Sanchez, A. (2017). Nanocarriers for microRNA delivery in cancer medicine. Biotechnol. Adv. 35, 350–360. doi: 10.1016/j.biotechadv.2017.03.002
Fine, H. A., Dear, K. B., Loeffler, J. S., Black, P. M., and Canellos, G. P. (1993). Meta-analysis of radiation therapy with and without adjuvant chemotherapy for malignant gliomas in adults. Cancer 71, 2585–2597. doi: 10.1002/1097-0142(19930415)71:8<2585::AID-CNCR2820710825>3.0.CO;2-S
Francescone, R., Scully, S., Bentley, B., Yan, W., Taylor, S. L., Oh, D., et al. (2012). Glioblastoma-derived tumor cells induce vasculogenic mimicry through Flk-1 protein activation. J. Biol. Chem. 287, 24821–24831. doi: 10.1074/jbc.M111.334540
Frieboes, H. B., Lowengrub, J. S., Wise, S., Zheng, X., Macklin, P., Bearer, E., et al. (2007). Computer simulation of glioma growth and morphology. Neuroimage 37, 59–70. doi: 10.1016/j.neuroimage.2007.03.008
Frieboes, H. B., Wu, M., Lowengrub, J., Decuzzi, P., and Cristini, V. (2013). A computational model for predicting nanoparticle accumulation in tumor vasculature. PLoS ONE 8:e56876. doi: 10.1371/journal.pone.0056876
Friedman, H. S., Prados, M. D., Wen, P. Y., Mikkelsen, T., Schiff, D., Abrey, L. E., et al. (2009). Bevacizumab alone and in combination with Irinotecan in recurrent glioblastoma. J. Clin. Oncol. 27, 4733–4740. doi: 10.1200/JCO.2008.19.8721
Frosina, G. (2015). Limited advances in therapy of glioblastoma trigger re-consideration of research policy. Crit. Rev. Oncol. Hematol. 96, 257–261. doi: 10.1016/j.critrevonc.2015.05.013
Galvano, F., La Fauci, L., Lazzarino, G., Fogliano, V., Ritieni, A., Ciappellano, S., et al. (2004). Cyanidins: metabolism and biological properties. J. Nutr. Biochem. 15, 2–11. doi: 10.1016/j.jnutbio.2003.07.004
Gevertz, J. L. (2011). Computational modeling of tumor response to vascular-targeting therapies- Part I: validation. Comput. Math. Methods Med. 2011:830515. doi: 10.1155/2011/830515
Gevertz, J. L., Gillies, G. T., and Torquato, S. (2008). Simulating tumor growth in confined heterogeneous environments. Phys. Biol. 5:036010. doi: 10.1088/1478-3975/5/3/036010
Gosk, S., Vermehren, C., Storm, G., and Moos, T. (2004). Targeting anti-transferrin receptor antibody (OX26) and OX26-conjugated liposomes to brain capillary endothelial cells using in situ perfusion. J. Cereb. Blood Flow. Metab. 24, 1193–1204. doi: 10.1097/01.WCB.0000135592.28823.47
Groh, C. M., Hubbard, M. E., Jones, P. F., Loadman, P. M., Periasamy, N., Sleeman, B. D., et al. (2014). Mathematical and computational models of drug transport in tumours. J. R. Soc. Interface 11:20131173. doi: 10.1098/rsif.2013.1173
Grover, A., Hirani, A., and Sutariya, V. (2014). Blood-brain barrier permeation of glutathione-coated nanoparticle. SOJ Pharm. Pharm. Sci. 4, 2–5. doi: 10.15226/2374-6866/1/1/00103
Hanada, S., Fujioka, K., Inoue, Y., Kanaya, F., Manome, Y., and Yamamoto, K. (2014). Cell-based in vitro blood–brain barrier model can rapidly evaluate nanoparticles' brain permeability in association with particle size and surface modification. Int. J. Mol. Sci. 15, 1812–1825. doi: 10.3390/ijms15021812
Hegi, M. E., Diserens, A. C., Gorlia, T., Hamou, M. F., de Tribolet, N., Weller, M., et al. (2005). MGMT gene silencing and benefit from temozolomide in glioblastoma. N. Engl. J. Med. 352, 997–1003. doi: 10.1056/NEJMoa043331
Huang, S., Shao, K., Kuang, Y., Liu, Y., Li, J., An, S., et al. (2013a). Tumor targeting and microenvironment-responsive nanoparticles for gene delivery. Biomaterials 34, 5294–5302. doi: 10.1016/j.biomaterials.2013.03.043
Huang, S., Shao, K., Liu, Y., Kuang, Y., Li, J., An, S., et al. (2013b). Tumor-targeting and microenvironment-responsive smart nanoparticles for combination therapy of antiangiogenesis and apoptosis. ACS Nano 7, 2860–2871. doi: 10.1021/nn400548g
Huang, Y., Arifin, D. Y., and Wang, C. H. (2009). Three-dimensional simulation of paclitaxel delivery to a brain tumor. Brain 1, 2. Available online at: http://pdfs.semanticscholar.org/ceca/6a347a1342e8a94919c5682519b0bf4e366b.pdf
Jain, R. K., and Stylianopoulos, T. (2010). Delivering nanomedicine to solid tumors. Nat. Rev. Clin. Oncol. 7, 653–664. doi: 10.1038/nrclinonc.2010.139
Jo, D. H., Kim, J. H., Lee, T. G., and Kim, J. H. (2015). Size, surface charge, and shape determine therapeutic effects of nanoparticles on brain and retinal diseases. Nanomedicine 11, 1603–1611. doi: 10.1016/j.nano.2015.04.015
Kawadkar, J. I., Chauhan, M. K., and Maharana, M. A. (2011). Nanobiotechnology: application of nanotechnology in diagnosis, drug discovery and drug development. Asian J. Pharm. Clin. Res. 4, 23–28. Available online at: http://www.ajpcr.com/Vol4Issue1/218.pdf
Kim, M., Gillies, R. J., and Rejniak, K. A. (2013). Current advances in mathematical modeling of anti-cancer drug penetration into tumor tissues. Front. Oncol. 3:278. doi: 10.3389/fonc.2013.00278
Kim, M. J., Rehman, S. U., Amin, F. U., and Kim, M. O. (2017). Enhanced neuroprotection of anthocyanin-loaded PEG-gold nanoparticles against Aβ1–42-induced neuroinflammation and neurodegeneration via the NF-KB/ JNK/ GSK3β signaling pathway. Nanomedicine 13, 2533–2544. doi: 10.1016/j.nano.2017.06.022
Kim, S. S., Harford, J. B., Pirollo, K. F., and Chang, E. H. (2015). Effective treatment of glioblastoma requires crossing the blood-brain barrier and targeting tumors including cancer stem cells: The promise of nanomedicine. Biochem. Biophys. Res. Commun. 468, 485–489. doi: 10.1016/j.bbrc.2015.06.137
Krakstad, C., and Chekenya, M. (2010). Survival signalling and apoptosis resistance in glioblastomas: opportunities for targeted therapeutics. Mol. Cancer 9:135. doi: 10.1186/1476-4598-9-135
Langlet, F. (2014). Tanycytes: a gateway to the metabolic hypothalamus. J. Neuroendocrinol. 26, 753–760. doi: 10.1111/jne.12191
Langlet, F., Mullier, A., Bouret, S. G., Prevot, V., and Dehouck, B. (2013). Tanycyte-like cells form a blood–cerebrospinal fluid barrier in the circumventricular organs of the mouse brain. J. Comp. Neurol. 521, 3389–3405. doi: 10.1002/cne.23355
Lauzon, M. A., Daviau, A., Marcos, B., and Faucheux, N. (2015). Nanoparticle-mediated growth factor delivery systems: a new way to treat Alzheimer's disease. J. Control. Release 206, 187–205. doi: 10.1016/j.jconrel.2015.03.024
Lin, K. W., Liao, A., and Qutub, A. A. (2015). Simulation predicts IGFBP2-HIF1α interaction drives glioblastoma growth. PLOS Comput. Biol. 11:e1004169. doi: 10.1371/journal.pcbi.1004169
Liu, Y., and Lu, W. (2012). Recent advances in brain tumor-targeted nano-drug delivery systems. Expert Opin. Drug Deliv. 9, 671–686. doi: 10.1517/17425247.2012.682726
Martins, S., Mussatto, S. I., Martínez-Avila, G., Monta-ez-Saenz, J., Aguilar, C. N., and Teixeira, J. A. (2011). Bioactive phenolic compounds: production and extraction by solid-state fermentation. A review. Biotechnol. Adv. 29, 365–373. doi: 10.1016/j.biotechadv.2011.01.008
Martirosyan, N. L., Rutter, E. M., Ramey, W. L., Kostelich, E. J., Kuang, Y., and Preul, M. C. (2015). Mathematically modeling the biological properties of gliomas: a review. Math. Biosci. Eng. 12, 879–905. doi: 10.3934/mbe.2015.12.879
Matsson, P., and Bergström, C. A. (2015). Computational modeling to predict the functions and impact of drug transporters. Silico Pharmacol. 3, 8. doi: 10.1186/s40203-015-0012-3
McLendon, R., Friedman, A., Bigner, D., Van Meir, E. G., Brat, D. J., Mastrogianakis, G. M., et al. (2008). Comprehensive genomic characterization defines human glioblastoma genes and core pathways. Nature 455, 1061–1068.
Nduom, E. K., Bouras, A., Kaluzova, M., and Hadjipanayis, C. G. (2012). Nanotechnology applications for glioblastoma. Neurosurg. Clin. N. Am. 23, 439–449. doi: 10.1016/j.nec.2012.04.006
Neal, M. L., Trister, A. D., Ahn, S., Baldock, A., Bridge, C. A., Guyman, L., et al. (2013). Response classification based on a minimal model of glioblastoma growth is prognostic for clinical outcomes and distinguishes progression from pseudoprogression. Cancer Res. 73, 2976–2986. doi: 10.1158/0008-5472.CAN-12-3588
Qin, Y., Fu, M., Takahashi, M., Iwanami, A., Kuga, D., Rao, R. G., et al. (2014). Epithelial membrane protein-2 (EMP2) activates Src protein and is a novel therapeutic target for glioblastoma. J. Biol. Chem. 289, 13974–13985. doi: 10.1074/jbc.M113.543728
Quigley, M. R., and Maroon, J. C. (1991). The relationship between survival and the extent of the resection in patients with supratentorial malignant gliomas. Neurosurgery 29, 385–388. Discussion: 388–389. doi: 10.1227/00006123-199109000-00008
Reppas, A. I., Alfonso, J. C., and Hatzikirou, H. (2016). In silico tumor control induced via alternating immunostimulating and immunosuppressive phases. Virulence 7, 174–186. doi: 10.1080/21505594.2015.1076614
Rockne, R. C., Trister, A. D., Jacobs, J., Hawkins-Daarud, A. J., Neal, M. L., Hendrickson, K., et al. (2004). A patient-specific computational model of hypoxia-modulated radiation resistance in glioblastoma using 18F-FMISO-PET. J. R. Soc. Interface 12:20141174. doi: 10.1098/rsif.2014.1174
Rutter, E. M., Stepien, T. L., Anderies, B. J., Plasencia, J. D., Woolf, E. C., Scheck, A. C., et al. (2017). Mathematical analysis of glioma growth in a murine model. Sci. Rep. 7:2508. doi: 10.1038/s41598-017-02462-0
Salata, O. (2004). Applications of nanoparticles in biology and medicine. J. Nanobiotechnol. 2:3. doi: 10.1186/1477-3155-2-3
Salazar, O. M., Rubin, P., Feldstein, M. L., and Pizzutiello, R. (1979). High dose radiation therapy in the treatment of malignant gliomas: final report. Int. J. Radiat. Oncol. Biol. Phys. 5, 1733–1740. doi: 10.1016/0360-3016(79)90554-6
Salcman, M. (1988). Surgical resection of malignant brain tumors: who benefits? Oncology 2, 47–56, 59–60, 63.
Saltzman, W. M., and Radomsky, M. L. (1991). Drugs released from polymers: diffusion and elimination in brain tissue. Chem. Eng. Sci. 46, 2429–2444. doi: 10.1016/0009-2509(91)80036-X
Schlüter, D. K., Ramis-Conde, I., and Chaplain, M. A. J. (2012). Computational modeling of single-cell migration: the leading role of extracellular matrix fibers. Biophys. J. 6, 1141–1151. doi: 10.1016/j.bpj.2012.07.048
Séhédic, D., Cikankowitz, A., Hindré, F., Davodeau, F., and Garcion, E. (2015). Nanomedicine to overcome radioresistance in glioblastoma stem-like cells and surviving clones. Trends Pharmacol. Sci. 36, 236–252. doi: 10.1016/j.tips.2015.02.002
Swanson, K. R., Rockne, R. C., Claridge, J., Chaplain, M. A., Alvord, E. C., and Anderson, A. R. (2011). Quantifying the role of angiogenesis in malignant progression of gliomas: in silico modeling integrates imaging and histology. Cancer Res. 71, 7366–7375. doi: 10.1158/0008-5472.CAN-11-1399
Takae, S., Akiyama, Y., Otsuka, H., Nakamura, T., Nagasaki, Y., and Kataoka, K. (2005). Ligand density effect on biorecognition by PEGylated gold nanoparticles: regulated interaction of RCA120 lectin with lactose installed to the distal end of tethered PEG strands on gold surface. Biomacromolecules 6, 818–824. doi: 10.1021/bm049427e
TCGA (2008). Comprehensive genomic characterization defines human glioblastoma genes and core pathways. Nature 455, 1061–1068. doi: 10.1038/nature07385
Tektonidis, M., Hatzikirou, H., Chauvière, A., Simon, M., Schaller, K., and Deutsch, A. (2011). Identification of intrinsic in vitro cellular mechanisms for glioma invasion. J. Theor. Biol. 287, 131–147. doi: 10.1016/j.jtbi.2011.07.012
The New WHO Classification of Tumors Affecting the Central Nervous System [WWW Document] (1993). Available online at: http://neurosurgery.mgh.harvard.edu/newwhobt.htm (Accessed May 24, 2017).
Thuy, M. N., Kam, J. K., Lee, G. C., Tao, P. L., Ling, D. Q., Cheng, M., et al. (2015). A novel literature-based approach to identify genetic and molecular predictors of survival in glioblastoma multiforme: analysis of 14,678 patients using systematic review and meta-analytical tools. J. Clin. Neurosci. 22, 785–799. doi: 10.1016/j.jocn.2014.10.029
Timbie, K. F., Mead, B. P., and Price, R. J. (2015). Drug and gene delivery across the blood–brain barrier with focused ultrasound. J. Control. Release 219, 61–75. doi: 10.1016/j.jconrel.2015.08.059
Torchilin, V. (2011). Tumor delivery of macromolecular drugs based on the EPR effect. Adv. Drug Deliv. Rev. 63, 131–135. doi: 10.1016/j.addr.2010.03.011
Van Tellingen, O., Yetkin-Arik, B., de Gooijer, M. C., Wesseling, P., Wurdinger, T., and de Vries, H. E. (2015). Overcoming the blood-brain tumor barrier for effective glioblastoma treatment. Drug Resist. Updat. 19, 1–12. doi: 10.1016/j.drup.2015.02.002
Walker, M. D., Green, S. B., Byar, D. P., Alexander Jr, E., Batzdorf, U., Brooks, W. H., et al. (1980). Randomized comparisons of radiotherapy and nitrosoureas for the treatment of maligant glioma after surgery. N. Engl. J. Med. 303, 1323–1329. doi: 10.1056/NEJM198012043032303
Watanabe, Y., Dahlman, E. L., Leder, K. Z., and Hui, S. K. (2016). A mathematical model of tumor growth and its response to single irradiation. Theor. Biol. Med. Model. 13, 6. doi: 10.1186/s12976-016-0032-7
Weber, A. M., and Ryan, A. J. (2015). ATM and ATR as therapeutic targets in cancer. Pharmacol. Ther. 149, 124–138. doi: 10.1016/j.pharmthera.2014.12.001
Wei, X., Chen, X., Ying, M., and Lu, W. (2014). Brain tumor-targeted drug delivery strategies. Acta Pharm. Sin. B 4, 193–201. doi: 10.1016/j.apsb.2014.03.001
Wen, P. Y., and Kesari, S. (2008). Malignant gliomas in adults. New Engl. J. Med. 359, 492–507. doi: 10.1056/NEJMra0708126
Whittle, J. R., Lickliter, J. D., Gan, H. K., Scott, A. M., Simes, J., Solomon, B. J., et al. (2015). First in human nanotechnology doxorubicin delivery system to target epidermal growth factor receptors in recurrent glioblastoma. J. Clin. Neurosci. 22, 1889–1894. doi: 10.1016/j.jocn.2015.06.005
Yung, W. K., Prados, M. D., Yaya-Tur, R., Rosenfeld, S. S., Brada, M., Freidman, H. S., et al. (1999). Multicenter phase II trial of temozolomide in patients with anaplastic astrocytoma or anaplastic oligoastrocytoma at first relapse. Temodal. Brain Tumor. Group. J. Clin. Oncol. 17, 2762–2771. doi: 10.1200/JCO.1999.17.9.2762
Zhang, L., Athale, C. A., and Deisboeck, T. S. (2007). Development of three-dimensional multiscale agent-based tumor model: simulating gene protein interaction profiles, cell phenotypes and multicellular patterns in brain cancer. J. Theor. Biol. 244, 96–107. doi: 10.1016/j.jtbi.2006.06.034
Keywords: glioblastoma, delphinidin, nanoparticle, cytoscape, blood-brain barrier modeling
Citation: Ozdemir-Kaynak E, Qutub AA and Yesil-Celiktas O (2018) Advances in Glioblastoma Multiforme Treatment: New Models for Nanoparticle Therapy. Front. Physiol. 9:170. doi: 10.3389/fphys.2018.00170
Received: 28 June 2017; Accepted: 20 February 2018;
Published: 19 March 2018.
Edited by:
Radhakrishnan Nagarajan, University of Kentucky, United StatesReviewed by:
Preetam Ghosh, Virginia Commonwealth University, United StatesOksana Sorokina, University of Edinburgh, United Kingdom
Copyright © 2018 Ozdemir-Kaynak, Qutub and Yesil-Celiktas. This is an open-access article distributed under the terms of the Creative Commons Attribution License (CC BY). The use, distribution or reproduction in other forums is permitted, provided the original author(s) and the copyright owner are credited and that the original publication in this journal is cited, in accordance with accepted academic practice. No use, distribution or reproduction is permitted which does not comply with these terms.
*Correspondence: Elif Ozdemir-Kaynak, ZWxpZm96ZGVtaXI4OUBnbWFpbC5jb20=