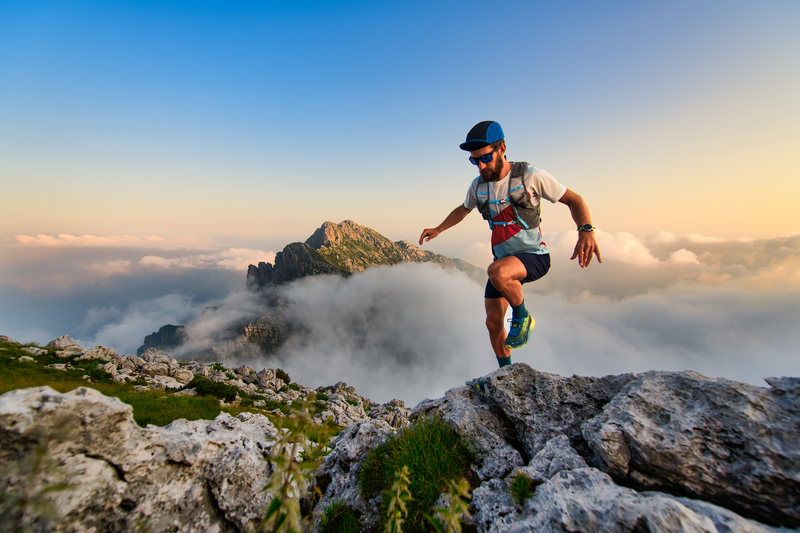
94% of researchers rate our articles as excellent or good
Learn more about the work of our research integrity team to safeguard the quality of each article we publish.
Find out more
REVIEW article
Front. Physiol. , 04 January 2018
Sec. Exercise Physiology
Volume 8 - 2017 | https://doi.org/10.3389/fphys.2017.00985
Surface electromyography (sEMG) is a popular research tool in sport and rehabilitation sciences. Common study designs include the comparison of sEMG amplitudes collected from different muscles as participants perform various exercises and techniques under different loads. Based on such comparisons, researchers attempt to draw conclusions concerning the neuro- and electrophysiological underpinning of force production and hypothesize about possible longitudinal adaptations, such as strength and hypertrophy. However, such conclusions are frequently unsubstantiated and unwarranted. Hence, the goal of this review is to discuss what can and cannot be inferred from comparative research designs as it pertains to both the acute and longitudinal outcomes. General methodological recommendations are made, gaps in the literature are identified, and lines for future research to help improve the applicability of sEMG are suggested.
Surface electromyography (electromyography, EMG; surface EMG, sEMG) is a common research tool used to investigate a wide range of research questions across various disciplines. sEMG is perhaps most useful for providing insight into how the neuromuscular system behaves. In its simplest sense, sEMG is a highly-sensitive voltmeter that detects depolarizations and hyperpolarizations (increases and decreases in voltage, respectively) that occur on the sarcolemma (muscle fiber membrane). These depolarizations are necessary for, and precede, the contraction of a muscle. Among other disciplines, the utilization of sEMG is popular in sport and rehabilitation sciences, with the number of studies including the terms “EMG” and “exercise” increasing exponentially since 1950 (R2 = 0.98) (Corlan, 2004). In those studies, sEMG amplitudes collected from different muscles are commonly compared between exercises, techniques, and/or loads. Based on such comparisons, researchers often attempt to draw conclusions that can be separated into two primary categories: (1) inferring mechanisms and (2) predicting longitudinal outcomes. Included in acute, mechanistic variables are activation, force production and sharing characteristics, and recruitment strategies. Knowledge of such mechanistic variables is expected to inform researchers and practitioners as to the underpinning of why unique adaptions occur and through which pathways. Conversely, inferred longitudinal outcomes primarily consist of muscle hypertrophy and increases in strength, in that it is assumed that greater sEMG amplitudes are predictive of greater adaptation (Andersen et al., 2006; Escamilla et al., 2010; Reiman et al., 2012; Tsaklis et al., 2015; Halperin et al., 2017). Oftentimes, however, such conclusions are unsubstantiated and unwarranted. These misinterpretations mainly stem from (1) the complicated nature of sEMG and (2) lack of longitudinal work. This leaves both researchers and practitioners with the important question: If we cannot make such conclusions, what does sEMG tell us in the context of exercise?
Numerous pieces have been written on the shortcomings of sEMG in the exercise fields (Cavanagh, 1974; De Luca, 1997; Farina, 2006; Enoka and Duchateau, 2015). Indeed, influential biomechanists have been writing about similar issues since the 1970s (Cavanagh, 1974). However, in our view, few have attempted to simplify the neuro- and electrophysiological concepts in their discussion, making it difficult for those who lack a strong relevant background to fully understand the content. Thus, our goal with this review is to allow the applied exercise scientists and educated coaches and practitioners to develop a better understanding of this important topic. Further, rather than a general overview of sEMG that pertains to many research designs and fields, we aimed to narrow our discussion to a number of common research designs in the exercise domain. With this in mind, the purpose of this review is manifold. First, we discuss what conclusions cannot be drawn from sEMG studies in sport and rehabilitation sciences. This subsection will cover both acute and longitudinal inferences. Next, we will discuss what conclusions can be drawn from sEMG studies and what experimental considerations need to be made before such conclusions can be drawn. Lastly, we will identify gaps in the literature and suggest lines for future research to help improve the applicability of sEMG.
Despite the apparent simplicity of sEMG, many terms relating to EMG and neuromuscular physiology are often misused and conflated with one another. Therefore, before delving into the primary topics of this review, it is necessary to operationally define a number of technical terms relating to EMG and neuromuscular physiology. A schematic of how many of these definitions relate to one another can be found in Figure 1.
• Neural Excitation–Electrochemical input from an α-motoneuron that depolarizes all of the muscle fibers that it innervates (Gottlieb et al., 1990; Winters, 1990; Zatsiorsky and Prilutsky, 2012).
• Muscle Excitation–Depolarization of the sarcolemma following neural excitation, delivered to the muscle via the neuromuscular junction (Farina et al., 2016). This is also referred to as the muscle fiber action potential.
• Activation Dynamics–Events following excitation that cause a muscle to produce active force via actin-myosin cross-bridging, also known as excitation-contraction coupling (Gottlieb et al., 1990; Winters, 1990; Zatsiorsky and Prilutsky, 2012). These events are affected by the state of the muscle (i.e., previous activation), and thus, so are the events that follow (Figure 1).
• Muscle Activation–The active state of a muscle, ranging from 0% (all fibers inactive) to 100% (all fibers active) (Gottlieb et al., 1990; Winters, 1990; Zatsiorsky and Prilutsky, 2012).
• Muscle Contraction Dynamics–Takes into account the length and velocity of the muscle (Zajac, 1989). Many phenomenological models exist to represent these dynamics, such as the Hill muscle model (Hill, 1938), the Huxley cross-bridge model (Huxley, 1957), and the three-filament model (includes titin) (Herzog et al., 2012, 2015).
• Muscle Force–Force that is produced by the muscle.
• Surface EMG–Electrophysiological recording technology used for the non-invasive detection of the electric potential resulting from the transmembrane current of muscle fibers (muscle excitation). With appropriate processing and based on sufficiently reasonable approximations, sEMG may provide information on the timing and degree of muscles' excitation (Zajac, 1989)1.
Figure 1. Production of muscle force from neural input. Excitation is the electrochemical input from the central nervous system into the muscle. This signal triggers excitation-contract coupling, which leads to an active muscle state (activation). Finally, muscle force is produced after cross-bridges are formed and force is transmitted through the muscle. Adapted from Zajac (1989).
In this section, we cover a number of acute and mechanistic variables that are commonly collected, analyzed, and interpreted with the goal of developing a better understanding of force production and the pathways leading to force generation. Specifically, motor unit recruitment, rate coding, activation, force generation, and force sharing are discussed in view of the common interpretation they receive in the sport and rehabilitation sciences.
A motor unit is the fundamental unit that converts efferent action potentials to force. It consists of a motor neuron and all of the muscle fibers that said motor neuron innervates. There are two primary mechanisms by which the nervous system enable a muscle to produce more force2: (1) motor unit recruitment, in which more motor units are utilized, and (2) rate coding, in which the motor units that are already recruited “fire” at a faster rate (Kukulka and Clamann, 1981; van Bolhuis et al., 1997; Farina et al., 2016). Importantly, the relative contribution of motor unit recruitment and rate coding depends on the muscle group (Kukulka and Clamann, 1981; De Luca et al., 1982; De Luca and Kline, 2012). To complicate things further, these recruitment characteristics are task-dependent, in that they change with different rates of force development, like during ballistic contractions, and thus are not specific to force levels per se (Desmedt and Godaux, 1977). Unless the specific recruitment characteristics of a muscle are known, one cannot discern motor unit recruitment from rate coding using sEMG amplitude. Moreover, how a muscle recruits its motor units will greatly affect sEMG amplitude; for example, a muscle that recruits motor units from deep to superficial will display a different sEMG amplitude-force relationship than would a muscle that recruits motor units from superficial to deep, assuming rate coding characteristics are equal (Figure 2; Farina et al., 2016). This is exemplified by Mesin et al. (2010), who found that the tibialis anterior likely has a superficial-to-deep recruitment pattern, and thus has an sEMG amplitude-force relationship similar to that depicted in Case 1 in Figure 2. Whether there is a preferential recruitment direction for different muscles or circumstances has yet to be determined. It is clear that heterogeneity in motor unit recruitment and rate coding patterns precludes one from making conclusions about either characteristic from sEMG amplitude alone.
Figure 2. Recruitment methods and their effects on sEMG amplitude. Case 1: If a muscle recruits motor units from superficial to deep, then this will result in sEMG amplitude rising at a faster rate than force; that is, sEMG amplitude (% MVIC) ≥ Force (% MVIC). Case 2: If a muscle recruits motor units from deep to superficial, then this will result in force levels rising at a greater rate than sEMG amplitude; that is, Force (% MVIC) ≥ sEMG amplitude (% MVIC).
Although there are methods to investigate motor unit recruitment and rate coding using sEMG—such as spike-triggered averaging and spectral analyses—these techniques are complex and their validity, especially during dynamic, high-intensity, or fatiguing exercise, is questionable (Farina et al., 2010, 2014). Moreover, sEMG amplitude alone, which is likely the variable most-often reported from sEMG experiments, cannot be used to infer motor unit recruitment or rate coding. This is because both motor unit recruitment and rate coding have significant, and nearly equal, contributions to time-averaged sEMG amplitude [root mean square (RMS) or average rectified value (ARV)] under non-fatiguing conditions (Farina et al., 2016). Hence, since the relative contribution of each of the two pathways cannot be separated using sEMG amplitude, the conclusions that can be drawn for each mechanism are limited. Indeed, a recent model suggests that similar recruitment can occur under different loads with different levels of excitation, simply due to differences in rate coding (Potvin and Fuglevand, 2017). In addition, under fatiguing conditions and following training periods, sEMG amplitudes may be altered by intra- and extracellular ion concentrations and motor unit synchronization in unintuitive ways (Dimitrova and Dimitrov, 2003; Arabadzhiev et al., 2010a,b). These alterations affect sEMG amplitudes and confound the neural drive collected with sEMG with other measures (Arabadzhiev et al., 2010a,b; Dideriksen et al., 2011). From these inherent characteristics, it is clear that the array of contributors to sEMG amplitude precludes one from drawing conclusions that pertain to motor unit recruitment and rate coding from sEMG amplitude. Hopefully, future work implementing new, more sophisticated technologies, like high-density (HD) EMG and decomposition techniques, may allow for greater insight into these mechanisms (Merletti et al., 2008). However, until such technologies are available and validated, researchers are limited as to the mechanistic conclusions that can be drawn from sEMG signals (Farina et al., 2010, 2014; Del Vecchio et al., 2017).
Muscle activation refers to the state of the muscle and is related to the magnitude of force that a muscle actively produces (i.e., not including passive contributions) relative to its maximum ability to produce force actively (Figure 3A). Although force is related to activation, force and activation differ in a number of ways. First, activation only deals with active contributions to muscle force and thus ignores passive components (Figure 3A). Second, activation is essentially a scaling factor, which relates active force and maximum potential active force at a given fiber length and velocity (Zajac, 1989; Figure 3B). Lastly, activation is unaffected by length and velocity, whereas force production is highly influenced by fiber length and velocity. This is because activation does not take into account muscle contraction dynamics (force-length, force-velocity, history dependence, etc.). In other words, activation is related to the number of fibers that are active and not the force-generating capacity of those fibers.
Figure 3. The isometric relationships between muscle force, activation, excitation, and fiber length. (A) Only the active curve is affected by activation, and any activation can occur regardless of the muscle's normalized force output. That is, activation is independent of fiber length; normalized force is a function of fiber length and activation, in addition to contraction velocity (not shown). The passive length-tension curve is unaffected by activation; this has important implications for force production and force sharing. Both force and length are represented relative to force and length, respectively, at optimal lengtha. (B) Excitation is curvilinearly related to activation and force, whereas force and activation are directly related to one another (one-to-one). Different muscles have different excitation-activation relationships, and thus, excitation for a given muscle can be one of the numerous lines that are plotted. Graph derived from Potvin et al. (1996) and Lloyd and Besier (2003). (C) Raw EMG is influenced primarily by motor unit recruitment and rate coding. The envelope of this signal, rectified EMG, may be considered the sum of neural drive to the area of muscle over which the electrode is placed, which, somehow [e.g., Σui(t)], is related to excitation, u(t). By filtering this signal, one can obtain data that are related to activation, a(t). Adapted from Zajac (1989). aThe optimal length shifts to the right with decreasing levels of activation (Lloyd and Besier, 2003; de Brito Fontana and Herzog, 2016), but a thorough description of these changes is outside the scope of this review.
Rather than measuring activation, sEMG measures changes in the polarity of the muscle fibers' membrane resulting from neural excitation; in other words, sEMG is a measure of muscle excitation. Activation and excitation differ in that excitation is a precursor to activation (Figure 1). Specifically, activation takes into account excitation-contraction dynamics (Zajac, 1989), including electromechanical delays, ion kinetics, etc., whereas excitation does not (Figure 1). Activation can be estimated from muscle excitation and sEMG, but the relationship is not straightforward (Figures 3B,C; Lloyd and Besier, 2003; Staudenmann et al., 2010). Because sEMG is not a direct measure of muscle activation, we suggest that authors avoid using the term “activation” when referring to sEMG amplitudes. Instead, authors should simply utilize the term “sEMG amplitude,” “muscle excitation,” or “myoelectric activity.” Moreover, we also propose that the term “muscle activity” is ambiguous and should be avoided, as muscles can provide large contributions to a movement without being excited.
sEMG is particularly attractive because its primary constituents, motor unit recruitment and rate coding, are also the precursors to active force generation (Staudenmann et al., 2010). As such, one may be eager to associate sEMG amplitudes, especially those that are normalized to maximum voluntary isometric contractions (MVIC), with muscle force. This attraction has been eloquently and satirically described by Dr. Peter Cavanagh in 1974, “The day that most electromyographic kinesiologists are collectively awaiting, with the enthusiasm of Doomsday watchers, is that when they can use some measurement derived from the electromyogram to indicate the force being produced by a muscle group during unrestricted movement of the body” (Cavanagh, 1974). Just like in 1974, doing so today is erroneous for a number of reasons. First, the passive force-length curve is ignored (i.e., force contributions from non-contractile structures, such as collagen/extracellular matrix, tendons, fascia, titin, etc.). A muscle can produce force with an sEMG amplitude of zero due to its passive properties (Figure 3A–black line). Second, the active length-tension curve and contraction history of a given muscle must be taken into account (Herzog et al., 2015). The often-cited curvilinear relationship between sEMG amplitude and force pertains to isometric contractions at one joint angle. However, when muscles change length, or even have velocities, then this relationship becomes much more complicated (Farina, 2006). When considering the active length-tension relationship, an activation of 70% at a muscle length of 0.83 will produce similar force to an activation of 60% at a relative fiber length of 1.0 (Figure 3A). Contraction history, on the other hand, tells us that a muscle can produce more force following an eccentric contraction than a concentric one (Herzog, 2004; Herzog et al., 2015; Seiberl et al., 2015). Lastly, the amplitude of sEMG detected locally from the muscle does not take muscle properties into account—that is, physiological cross-sectional area and normalized muscle force—which will affect the force-generating capabilities of muscle (Zajac, 1989). These cautionary points have been elucidated in vivo, in animal work that has shown that EMG cannot reliably predict muscle force during dynamic tasks (Roberts and Gabaldón, 2008). The inherent heterogeneity and dynamic nature of the neuromuscular system preclude the deduction of muscle force from normalized sEMG signals.
Force sharing is highly related to force production, as it aims to understand how different muscles contribute to a net joint moment. It is quite logical to think that sEMG would provide valuable insight for force sharing, but not only do the aforementioned points on force production apply, but differences in muscle architecture must also be considered (e.g., physiological cross-sectional area, muscle moment arms, etc.). To estimate force sharing, one must utilize musculoskeletal modeling rather than just sEMG; however, sEMG can be quite useful for “informing” musculoskeletal models (Sartori et al., 2016). Such models are enormously complex, but they are necessary to better describe force sharing characteristics. Without such models, sEMG amplitude alone does not provide great insight into force sharing characteristics of mechanically redundant muscles.
Collectively, sEMG studies that aim to draw conclusions about muscle force production, muscle activation, or mechanisms of force production are problematic when based solely on sEMG amplitude. Oftentimes, in order to draw mechanistic conclusions, more advanced sEMG processing and modeling techniques are needed. At face value, at best, sEMG amplitude is strictly indicative of muscle excitation.
Longitudinal outcomes are undoubtedly the most applicable, clinically relevant measures that a sport or rehabilitation scientist can provide to a practitioner. In this section, we discuss two commonly-measured and sought after outcomes: muscular strength and hypertrophy. Measuring these outcomes, however, can be quite difficult and time-consuming (Halperin et al., 2017). Before their discussion, it is important to introduce the term “surrogate endpoint,” borrowed from the medical literature. Surrogate endpoints are often utilized to assess a difficult-to-measure variable—such as one that may take months or years to occur (like strength or hypertrophy)—with greater ease (Halperin et al., 2017). For example, cholesterol and blood pressure have been used as surrogate endpoints for cardiovascular health (Fleming and DeMets, 1996). Implied by the idea of a surrogate endpoint is that, not only is the surrogate endpoint correlated with the clinical endpoint or real variable of interest, but net effects of interventions on clinical outcomes are also reflected (Prentice, 1989; Fleming and DeMets, 1996). A myriad of studies has attempted to utilize sEMG as a surrogate endpoint of both strength and hypertrophy (Andersen et al., 2006; Escamilla et al., 2010; Reiman et al., 2012; Tsaklis et al., 2015; Halperin et al., 2017). While such studies may provide insight into the neuromuscular system and how changes in exercise variables, ranging from the exercise itself to load, may affect muscle excitation, at present, it is unknown if greater muscle excitation measured with sEMG is predictive of long-term adaptation, as studies investigating the predictive validity of sEMG are lacking (Halperin et al., 2017).
Muscle hypertrophy, or the growth of muscle fibers, is a highly sought-after exercise outcome for individuals ranging from patients with sarcopenia to physique competitors. Due to its longitudinal nature, it can be quite difficult and time-consuming to measure experimentally. As such, sport and rehabilitation scientists have commonly utilized sEMG to acutely compare exercises and loading schemes to help drive exercise programming. However, recommendations from these studies are often unjustified and ill-advised as, at present, there are no longitudinal studies to suggest that sEMG is predictive of hypertrophic outcomes (Halperin et al., 2017).
The premise upon which the assumption that sEMG amplitude may be useful for predicting hypertrophy could be challenged on a theoretical level; that is, muscle excitation is predictive of hypertrophic outcomes. A number of avenues have been explored that may challenge this premise, including the relationship between muscle protein synthesis (MPS) and hypertrophy, the presence of hypertrophy without muscle excitation, and differential hypertrophy with similar excitation, which are explained herein.
Acutely, it has been postulated that maximum motor unit recruitment, a primary constituent of the sEMG signal, is important for stimulating hypertrophy (Wernbom et al., 2007; Marcotte et al., 2015; Dankel et al., 2017). From a more mechanistic standpoint, the presumed implicit (NB this has not been explicitly stated) rationale for sEMG amplitude being able to predict hypertrophy would be that greater sEMG amplitude implies greater muscle excitation, which implies greater MPS (Kumar et al., 2009; Holm et al., 2010), which implies a greater hypertrophic response. This argument breaks down when considering that acute fractional protein synthesis, a measure of MPS, is not correlated with hypertrophy (Mitchell et al., 2014). Thus, even if sEMG amplitude was a valid surrogate for MPS, a study that to our knowledge has yet to take place, it will not necessarily be predictive of hypertrophic outcomes.
It has been known since the 1970s that a muscle does not need to be excited for growth to occur (Goldberg et al., 1975). In fact, a hypertrophic response can be elicited in denervated muscle by applying mechanical tension (stretch) (Sola et al., 1973). Such findings, that stretch itself is a stimulus for hypertrophy, were recently replicated in humans, in a study that demonstrated an increase in gastrocnemii muscle thickness following 6 weeks of static stretching (Simpson et al., 2017). Taken together, it is clear that a muscle does not need to be excited to grow; however, this evidence does not suggest that hypertrophy can be maximized without a muscle being excited.
More recent data further support the idea that muscle excitation alone cannot predict hypertrophy: Eftestøl et al. (2016) divided 20 rats into two groups: 10 high-load (100% of maximum isometric strength) and 10 low-load (50–60% of maximum isometric strength, thus resulting in a fast, concentric contraction). Experimenters stimulated tibialis anterior (TA) and extensor digitorum longus (EDL) with identical electrical stimulation every other day for 6 weeks (Eftestøl et al., 2016). It was found that the magnitude of hypertrophy of both TA and EDL were dependent upon and proportional to the load (Eftestøl et al., 2016). Therefore, hypertrophic responses were dependent on more than just excitation. Of relevance, activation-independent (not excitation-independent) differences in hypertrophy have also been shown to occur in humans. Noorkoiv et al. (2014) trained young adult males using isometric knee extensions at short or long muscle lengths (ascending and descending limbs of the length-tension curve, respectively), with loads corresponding to 80% of each participant's MVIC strength, measured at each participant's optimal joint angle, over a period of 6 weeks. Only participants who trained at long muscle lengths experienced increases in quadriceps muscle volume (Noorkoiv et al., 2014). From these data, it can be argued that hypertrophic responses were either independent of activation or, alternatively, greater with what may have been slightly lower activation, as passive-elastic forces may have contributed to the long-length condition (Noorkoiv et al., 2014). One may argue that the relationship between force output and sEMG amplitude is suggestive that sEMG may be able to serve as proxy for the force “felt” by a given muscle, and thus hypertrophy, but this relationship is muscle-length specific and can become quite complex, as discussed in the preceding section (section Acute and Mechanistic Variables).
In addition to the above, substantial evidence suggests that there is a disconnect between sEMG amplitudes and hypertrophy during highly-fatiguing conditions. Mitchell et al. (2012) measured the effects of 3 sets of 30% vs. 3 sets of 80% vs. 1 set of 80% of one-repetition maximum (1 RM) knee extensions on quadriceps muscle hypertrophy. Investigators found that 3 sets of 30% and 3 sets of 80% elicited similar growth (Mitchell et al., 2012). In an attempt to elucidate the mechanisms underlying these outcomes, Jenkins et al. (2015) carried out a sEMG study and found that, when taken to momentary muscular failure, 3 sets of 80% 1 RM elicited greater sEMG amplitude than did 3 sets of 30% 1 RM. These results indicate that sEMG amplitude alone cannot be used to predict hypertrophic outcomes in highly-fatiguing conditions (Vigotsky et al., 2016) and are consistent with several other studies, which have investigated either hypertrophy or sEMG (Schoenfeld et al., 2014, 2016, 2017; Looney et al., 2016).
Both basic science and applied research challenge the notion that excitation—the construct measured by sEMG—is necessarily predictive of hypertrophy, as other variables influence both sEMG amplitude and the strength and hypertrophy responses to resistance training. Notwithstanding these data, complementary data are necessary to paint a broader and more complete picture of the potential role of excitation in hypertrophy. Specifically, results from experiments in which excitation is the independent variable and load is a control variable are needed. For example, a study in which the load utilized is equal to 50% MVIC and excitations are 50% (isometric) and 90% (concentric) of maximum. A related study could explore the influence of sEMG amplitude on hypertrophy while keeping constant muscle length and contraction velocity. Moreover, it is important to note that absence of evidence is not evidence of absence; although sEMG has not been established to be predictive of hypertrophy, it does not mean that no relationship exists. Some indirect evidence loosely suggests that sEMG amplitude may have a potential role in predicting hypertrophy: When comparing rectus femoris sEMG amplitude in single-joint exercises (knee extension) to that elicited by multi-joint exercises (simultaneous hip and knee extension), it is clear that the former elicits much greater sEMG amplitudes (Yamashita, 1988; Ema et al., 2016). Separate, longitudinal evidence suggests that single-joint exercises are effective for rectus femoris hypertrophy (Ema et al., 2013), while multi-joint exercises (barbell back squats) are not (Fonseca et al., 2014; Earp et al., 2015). These data alone are not enough to suggest that a strong, predictive relationship exists, especially because the data are from different cohorts, but taken together may be used as a rationale to investigate whether a relationship does indeed exist. In other words, using these data to justify a relationship is tautological and lacks pragmatism; put much more eloquently, “… one must not verify an idea using the same data that suggested the idea in the first place” (Feynman et al., 2011).
Strength, like hypertrophy, is an outcome of resistance training that is highly desirable. Because there are many ways to test strength (Buckner et al., 2016), the working definition of strength within the context of this piece is the ability of a muscle to produce force in any objective, measurable context. Throughout the physical therapy and rehabilitation literature, sEMG amplitude thresholds for adaptation have been suggested; for example, thresholds of 40–60% of MVIC have been suggested to be necessary for strength gains to be elicited from a given muscle (Andersen et al., 2006; Ayotte et al., 2007; Escamilla et al., 2010; Reiman et al., 2012). It seems that these recommendations for sEMG interpretation stem from training load recommendations (Andersen et al., 2006). The rationale behind this is simply that sEMG amplitudes increase, or are correlated, with force, and greater loads are needed for greater strength gains. Implicated by such a rationale are a couple of assumptions that will be addressed: (1) normalized sEMG amplitude is equal to the relative load of an exercise, and (2) the relative loads provided are actually what is needed to improve strength.
The supposition that sEMG amplitude is equal to the relative load of an exercise may be true for some isometric muscle actions when performed in the same position to which sEMG has been normalized (Alkner et al., 2000), although not always (see section Acute and Mechanistic Variables) (Potvin et al., 1996). However, in the context of dynamic exercises, or when positions differ from the normalization position, this relationship does not hold (Aspe and Swinton, 2014; Calatayud et al., 2015; Vigotsky et al., 2015). Accordingly, there is no basis to assume that loading recommendations can be extrapolated to sEMG amplitude recommendations.
The second assumption of this rationale is that heavier loads are needed to increase strength. Indeed, while this is in line with the principle of specificity, in some contexts, lighter loads (<40%) have also been shown to have the ability to increase strength (Mitchell et al., 2012; Morton et al., 2016), albeit not as well as heavier loads (Schoenfeld et al., 2015). Moreover, there are data to suggest that strength gains may occur from unloaded activities, such as downhill walking (Maeo et al., 2015, 2016), which only elicits EMG amplitudes of ~30% MVIC for the vastus medialis and rectus femoris (Maeo et al., 2012). The construct of strength gain is not straightforward, as results will depend on how strength is tested (Buckner et al., 2016; Gentil et al., 2017) and that strength gains are not a binary outcome, but rather, they occur on a continuum. As such, we feel that this premise is not supported by more recent literature. Because strength is born from a variety of exercise programming variables (load, frequency, volume, etc.) and characteristics (kinetics and kinematics), more work is needed to understand exactly how sEMG amplitudes play an interactive role in exercise prescription. Finally, because strength is often tested as an emergent property of one or multiple joints, adaptations of surrounding musculature (agonists, synergists, and antagonists) must also be considered; these adaptations may decrease the relative importance of single-muscle adaptation for single- or multi-joint strength outcomes (de Boer et al., 2007).
An interesting and relevant longitudinal study was carried out by Calatayud et al. (2015), who had participants perform either a 6 RM elastic band-resisted push-up or 6 RM bench press over a 5-week period. Acutely, these exercises elicited similar sEMG amplitudes of the pectoralis major and anterior deltoid, and longitudinally, resulted in similar increases in bench press 6 RM and 1 RM (Calatayud et al., 2015). While this is a step in the right direction, the similarities in sEMG amplitude may very well have been an epiphenomenon rather than a cause for the strength outcomes. That is, both sEMG amplitudes and strength gains may have been a function of training load. More studies are needed that manipulate the relationship between load and sEMG amplitude to filter out their relative importance in rendering strength gains.
Muscular strength and hypertrophy are adaptations of interest for practitioners of all types, ranging from physical therapists to trainers and coaches. Due to the ease-of-use of sEMG and the seemingly logical basis for inferring strength and hypertrophy adaptations from sEMG amplitude, numerous authors have attempted to utilize sEMG amplitude as a surrogate endpoint for these longitudinal measures. Doing so, however, is not supported by the current body of literature. We strongly urge practitioners and researchers to view acute data through a critical lens; implying and inferring longitudinal outcomes from acute data should be condemned until acute measures have been validated as surrogate outcomes (Halperin et al., 2017). Lastly, from some of the data presented hitherto, it is likely that sEMG amplitudes will be interactive with other exercise programming variables in the determination of longitudinal adaptation. This is not a limitation of sEMG, but rather a limitation of working with complex biological systems.
Up to this point, the limitations of sEMG have been covered in great detail. However, it is prudent to discuss how sEMG can be properly applied and interpreted, in addition to how gray areas can affect interpretation. Therefore, this section focuses on what conclusions can “cleanly” be drawn from sEMG and under what conditions one must approach sEMG interpretations with more caution.
Knowing the state of a muscle is incredibly important for experiments that aim to measure variables that require no active contributions; for example, passive stiffness. This is often thought to be one of the most valid applications of sEMG, provided that the electrode is representative of the entire muscle (Cavanagh, 1974; Farina, 2006). This, however, is not always the case, as electrodes only cover a small, select portion of muscle (Figure 4A). Indeed, recent evidence suggests that some parts of a muscle may be electromyographically “silent” while other parts are not (dos Anjos et al., 2017; Figure 4B). These uncertainties likely also apply to greater contraction intensities, as negative electromechanical delay was recently observed in up to 23% of isometric elbow flexion trials with net joint moments ranging from 20 to 70% MVIC (Dieterich et al., 2017). These data suggest that an electrode, for a short period of time, is not representative of the entire muscle over which it is placed (Dieterich et al., 2017). It has been proposed that such findings are at least partially a function of interelectrode distance; smaller interelectrode distances result in smaller pick-up volumes, which decreases how representative a sEMG signal is of the muscle over which the electrodes are placed (Vieira et al., 2017). Fortunately, the periods during which electrodes are poorly representative of a muscle's binary state (on/off) are often transient (Figure 4B), and thus, it is likely that an electrode will provide insight as to the muscle's current state eventually. In other words, although an electrode may not accurately represent the state of a muscle at any instant (a single time point), it will provide the state of the muscle over longer periods of time. When dealing with short bursts of excitation, the implications are less clear and more caution should be used when interpreting results (Cavanagh, 1974). It follows that one may reasonably conclude whether or not a muscle is “on” or “off” if the time of interest is >~200 ms (Dieterich et al., 2017). Finally, one can increase their confidence in knowing the state of a muscle by using multiple electrodes or HD-sEMG, which accounts for intramuscular heterogeneity (Dieterich et al., 2017; dos Anjos et al., 2017; Le Mansec et al., 2017).
Figure 4. Under-representative sampling of motor units with sEMG. (A) Unlike observed for skin parallel-fibered muscles, the same action potentials propagating along the fibers of pennated muscles are not sampled by a single pair of surface electrodes positioned anywhere on the muscle. As exemplified above, action potentials of motor units A and D are detected mainly by proximal and distal electrodes (red and green ellipses), respectively. sEMG amplitudes detected locally likely provide an unrepresentative view of the actual degree of muscle excitation. Biased inferences may be drawn from unrepresentative sEMG. Consider, for example, the amplitude of sEMG detected by any pair of distal electrodes in the figure (from i to n). If, during a given submaximal contraction, only the distal muscle region is excited, normalized sEMG amplitude will likely indicate a nearly 100% degree of excitation. Conversely, normalized sEMG detected proximally would overly underestimate the degree of excitation. These considerations presume excitation of the whole muscle volume during the reference, normalization condition. Inferences on the degree and timing of excitation are not supported by EMGs detected locally from large, pennated muscles. Reproduced from Merletti et al. (2016), with permission. (B) Raw sEMG detected with pairs of electrodes from different locations along medial gastrocnemius. Gray, shaded areas indicate periods when the root mean square amplitude (30 ms epochs) was greater than the background, rest level during a standing task. Nota bene the false conclusions that can be drawn regarding the state of a muscle by looking at only one pair of electrodes (e.g., electrode pair #1). Reproduced from dos Anjos et al. (2017) (under CC-BY 4.0).
Much like knowing if a muscle is “on” or “off,” it can be incredibly insightful to know when a muscle changes its state; for example, determining when a muscle turns “on” during the gait cycle. Such insight has applications for musculoskeletal modeling, understanding pathology, and basic motor control. Due to the variability in electromechanical delays (Dieterich et al., 2017), gathering the timing of activation can be more complicated (Cavanagh, 1974). Thus, depending on the application and speed of movement (e.g., isometric vs. ballistic), electromechanical delays—although on the order of a few tenths of a second—may need to be considered (Cavanagh, 1974), as should the possibility that an electrode may not readily represent the state of a muscle (dos Anjos et al., 2017; Figure 4). Notwithstanding these points, if small errors are acceptable for the application in question (e.g., <200 ms; Dieterich et al., 2017), then sEMG can be very useful for this purpose. Finally, one can increase their confidence in knowing the state of the muscle by using multiple electrodes or HD-sEMG (Figure 4; Dieterich et al., 2017; dos Anjos et al., 2017; Le Mansec et al., 2017).
Muscle excitation is the process by which a motor neuron action potential depolarizes the sarcolemma of muscle fibers. This process leads to muscle activation and then the production of muscle force (Figure 1). Because muscle excitation involves the depolarization of a muscle fiber (muscle fiber action potential), the sum of muscle excitations can be recorded using sEMG (Figure 3C). Thus, rectified sEMG amplitude scales with the level of global muscle excitation (Figure 3C). However, when attempting to compare excitation between timepoints, individuals, or muscles, a number of factors must be considered. The following subsections describe considerations when attempting to draw conclusions on relative muscle excitation, and what information can be obtained under “perfect” experimental conditions, with controls for important confounding variables (e.g., electrode movement and changes in tissue conductivity) (Farina, 2006). It should be noted that such factors are quite difficult to control during dynamic contractions; in many cases, these factors are inherent and thus are impossible to control for (Farina, 2006). A summary of this section is shown in Table 1, and all of the examples/applications provided assume the consideration criteria provided in Table 1.
Table 1. Examples of practical research questions and possible conclusions that can be drawn based on the relative excitation of muscle from sEMG amplitudes.
When performed acutely, within a single session, relative muscle excitation can be measured, assuming that fatigue, electrode position/movement (relative to both the skin and underlying motor units), tissue conductivity, and signal nonstationarity (rapid changes in signal properties) are taken into account (Farina, 2006). Longitudinally, especially following a training program, such results should be more strongly scrutinized (Arabadzhiev et al., 2014). In such cases, changes in sEMG amplitude may arise from not only central factors4, but also peripheral factors5. Because these changes may arise from both neural and peripheral sources, it can be difficult to draw meaningful conclusions. In many cases, central adaptations may not be able to be discerned from peripheral adaptations using just sEMG.
Perhaps one of the most common types of comparisons made in sport and rehabilitation sciences are within-subject, within-muscle comparisons. For example, researchers will compare sEMG amplitudes elicited during different exercises (Andersen et al., 2006; Escamilla et al., 2010). Notwithstanding the noted considerations, acute within-subject, within-muscle (i.e., pre- and post-conditions within the same session) studies performed under controlled conditions (i.e., kinematically-matched; preferably isometric) may provide useful information. For example, Trajano et al. (2013) used sEMG normalized to maximum M-wave amplitude to help tease out peripheral factors from central factors that affect force loss following an acute bout of static stretching (Table 1). Longitudinally, sEMG may inform mechanisms of changes in function, so long as the aforementioned potential confounders are controlled for or, at the very least, taken into consideration (Table 1).
For measures to be indicative of muscle excitation, the previously-discussed confounding variables (fatigue, electrode movement, tissue conductivity, and nonstationarity) must be taken into account; tissue conductivity will necessarily be different between muscles, due to differences in geometry and dynamics (Farina, 2006). It should also be considered that an individual may be better able to voluntarily excite one muscle more than another during an MVIC task, or alternatively, electrodes may be more or less representative of a respective muscle (Figure 4). Both of these scenarios may result in altered (inflated or deflated) normalized sEMG amplitudes6. Moreover, the kinematics of the muscle over which the electrode is placed must be considered: Are the relative lengths and velocities of the muscles in question comparable, and how is electrode position relative to each muscle's innervation zone changing? One should be wary when attempting to compare excitation patterns of different muscles, especially during dynamic efforts (Farina, 2006).
If signals are properly normalized and appropriate considerations are made (Table 1), one may infer relative muscle excitation from within-subject, between-muscle sEMG studies. For example, such studies may provide insight as to how differences in strength arise following verbal cueing (Lohse et al., 2011) or how co-contraction following a resistance training program may help explain changes in strength (Erskine et al., 2010, 2014; Table 1). Other studies have utilized sEMG to compare which muscles experience the greatest excitation during different exercises (Santana et al., 2007; Table 1), but interpreting what these differences mean is ambiguous (see section What Conclusions Cannot Be Drawn from sEMG Studies?).
Much like comparing between-muscles, there are a number of concerns pertaining to whether or not individuals in a group, especially symptomatic ones, have the ability to maximally excite a muscle during a normalization trial7. Furthermore, different participants respond differently to different normalization positions and techniques (Vera-Garcia et al., 2010; Contreras et al., 2015), so one can never be sure whether they are comparing apples-to-apples, so to speak, unless more robust normalization techniques are used, such as maximum M-wave amplitude. In such cases, training (exercise) experience may still confound maximum M-wave normalized sEMG signals (Arabadzhiev et al., 2014). Therefore, comparisons between subjects of starkly different populations (trained vs. untrained or those in pain vs. not in pain) may be confounded (Farina, 2006).
In more homogeneous populations, one can use sEMG to understand mechanisms for differences in function. For example, authors have used maximum M-wave normalized sEMG amplitudes to help us understand differences in strength between individuals (Trezise et al., 2016) and to explain how changes in strength may arise following different interventions (Jenkins et al., 2017; Table 1).
The considerations for between-subject and between-muscle comparisons above both apply to this category, but are amplified due to the larger potential for differences to exist.
sEMG can be useful for comparing co-contraction between populations—such young and old individuals—to understand how differences in function—such as strength—may arise (Macaluso et al., 2002; Table 1). Moreover, sEMG from several muscles drive muscle synergy analyses, which are showing to be informative for understanding neural control differences in those with cerebral palsy or changes following a stroke (Steele et al., 2015; Table 1). Muscle synergy analyses can also be applied to experimental questions in sport, which could provide insight into motor control strategy differences between populations while carrying out a task (e.g., expert vs. non-expert) or under different biomechanical constraints (e.g., limiting degrees of freedom or adding external load). Longitudinally, one may wish to understand how between-muscle neural control strategies explain differential adaptations to an intervention (Erskine et al., 2010, 2014; Table 1).
Challenging the applicability of sEMG can be viewed as bittersweet. While it is quite humbling that we do not truly understand what we are measuring or its implications, this also opens the door for high quality, impactful research. Ultimately, longitudinal outcomes are likely of greatest interest to practitioners (Halperin et al., 2017). Therefore, there is a tremendous need to evaluate the ability of sEMG to be used as a surrogate endpoint for muscle strengthening and hypertrophy (Halperin et al., 2017). We, the authors, have pondered potential study designs to investigate this question but have been unable to ideate something that we believe is sufficient to answer the question. While previous investigations may be thinking along the right lines, they do not provide robust evidence (Calatayud et al., 2015). However, we encourage readers and fellow scientists to brainstorm and carry out such research, with the end goal of determining (1) if sEMG amplitude can be predictive of strength improvements or hypertrophy, (2) what the minimum difference in sEMG amplitude is for predicting greater strength or hypertrophy adaptations, (3) how generalizable the results are, and (4) how new technologies and more advanced signal processing techniques can be utilized. Indeed, hypertrophy and strength are likely highly multifactorial and nonlinear, which will make such research tremendously difficult, if not impossible. Similar work has already been carried out as it pertains to muscle protein synthesis and hormone responses to exercise bouts (Mitchell et al., 2012, 2014; West and Phillips, 2012; Damas et al., 2016; Morton et al., 2016), and we believe it is time that sEMG be scrutinized in a similar fashion. Such research will validate or invalidate hundreds, if not thousands, of sEMG studies that were intended to be extrapolated for these longitudinal outcomes.
1. Factors other than muscular effort influence the myoelectric signal, including muscle length, contraction mode, contraction speed, etc. Comparing sEMG signals between different exercises that do not control for these variables should be avoided.
2. Even when the sEMG signal adequately represents the force of the muscle, caution should be exercised when concluding that a certain exercise will be better for increasing strength or hypertrophy due to other factors that influence these adaptations.
3. Comparing normalized sEMG values between individuals with and without pain should be viewed cautiously. Changes in the normalized sEMG value over time cannot indicate changes in excitation because the normalized value can be influenced by excitation during the normalization contraction or during the measured exercise. Normalizing to maximum M-wave amplitude can, to an extent, help diminish such effects.
4. Within-subject, within-muscle comparisons of the sEMG signal across different exercises may be able to provide insight into muscular force production, provided the previously mentioned controls are made.
sEMG is a useful tool for gaining insight into the neuromuscular system, musculoskeletal modeling, and basic science work, but its practical applicability is limited at present. Researchers wishing to produce applicable research pertaining to longitudinal adaptation should prioritize longitudinal studies rather than acute, cross-sectional sEMG work (Halperin et al., 2017). Because sEMG has not been validated as a surrogate endpoint for longitudinal measures, readers should be wary of bold conclusions. Important mechanistic details of sEMG, such as signals being confounded by peripheral factors and data not being representative of a muscle, must be considered when attempting to draw conclusions—even acute, mechanistic ones. For these points, we wish to stress that the burden of proof is on researchers to show that cross-sectional sEMG findings are practically meaningful for longitudinal outcomes, and until this is shown, discussions and conclusions should not imply that they are. Finally, although this review was expansive, depth was sacrificed for breadth and communicabilty. For readers interested in learning more about some of the topics discussed in this review, recommended texts, chapters, papers, and reviews are provided in Table 2.
AV, IH, GT, and TV conceived the manuscript. AV, IH, GL, GT, and TV wrote, reviewed, and approved the final version of the manuscript. AV and TV created the figures. AV and IH constructed the tables.
The authors declare that the research was conducted in the absence of any commercial or financial relationships that could be construed as a potential conflict of interest.
1. ^This is not representative of the number of active cross-bridges in the muscle, given the number of binding sites in an active muscle changes with its length. For example, the number of cross-bridges established in an active muscle reduces as the muscle shortens from its optimal length. Therefore, such measurements are not a measure of muscle force, namely due to contraction dynamics.
2. ^It should be noted that both of these mechanisms only pertain to active force production, due to cross-bridging, and are independent of passive contributors to force production (e.g., parallel elastic elements).
3. ^Units are arbitrary, relative to the number of active fibers and the muscle fibers' optimal length, respectively.
4. ^Central factors include, but are not limited to, corticomotor (Fisher et al., 2016) and motoneuron (Heckman et al., 2009) excitability.
5. ^Peripheral factors include, but are not limited to, increased intracellular action potentials due to changes in the biochemical environment (e.g., increased resting calcium levels, Arabadzhiev et al., 2014), changes in tissue properties (e.g., subcutaneous fat, Kuiken et al., 2003), and changes in muscle architecture (Lieber and Fridén, 2000).
6. ^Normalizing to a submaximum level of excitation when maximum is assumed will inflate the normalized sEMG amplitude. This effect may be amplified with differential recruitment patterns (Figure 2). Moreover, in the case of submaximal normalizations, the linear assumption of normalizations may be violated (Hug and Tucker, 2017).
7. ^Pain centrally inhibits muscle excitation (Farina et al., 2005) and changes EMG-to-force ratios (Graven-Nielsen et al., 1997) without affecting muscle function (Arvidsson et al., 1986; Graven-Nielsen et al., 1997). Thus, someone in pain may produce lower excitation during a normalization trial (e.g., MVIC), which would inflate normalized sEMG amplitudes.
Alkner, B. A., Tesch, P. A., and Berg, H. E. (2000). Quadriceps EMG/force relationship in knee extension and leg press. Med. Sci. Sports Exerc. 32, 459–463. doi: 10.1097/00005768-200002000-00030
Andersen, L. L., Magnusson, S. P., Nielsen, M., Haleem, J., Poulsen, K., and Aagaard, P. (2006). Neuromuscular activation in conventional therapeutic exercises and heavy resistance exercises: implications for rehabilitation. Phys. Ther. 86, 683–697. doi: 10.1093/ptj/86.5.683
Arabadzhiev, T. I., Dimitrov, V. G., Dimitrova, N. A., and Dimitrov, G. V. (2010a). Influence of motor unit synchronization on amplitude characteristics of surface and intramuscularly recorded EMG signals. Eur. J. Appl. Physiol. 108, 227–237. doi: 10.1007/s00421-009-1206-3
Arabadzhiev, T. I., Dimitrov, V. G., Dimitrova, N. A., and Dimitrov, G. V. (2010b). Interpretation of EMG integral or RMS and estimates of “neuromuscular efficiency” can be misleading in fatiguing contraction. J. Electromyogr. Kinesiol. 20, 223–232. doi: 10.1016/j.jelekin.2009.01.008
Arabadzhiev, T. I., Dimitrov, V. G., and Dimitrov, G. V. (2014). The increase in surface EMG could be a misleading measure of neural adaptation during the early gains in strength. Eur. J. Appl. Physiol. 114, 1645–1655. doi: 10.1007/s00421-014-2893-y
Arvidsson, I., Eriksson, E., Knutsson, E., and Arnér, S. (1986). Reduction of pain inhibition on voluntary muscle activation by epidural analgesia. Orthopedics 9, 1415–1419.
Aspe, R. R., and Swinton, P. A. (2014). Electromyographic and kinetic comparison of the back squat and overhead squat. J. Strength Cond. Res. 28, 2827–2836. doi: 10.1519/JSC.0000000000000462
Ayotte, N. W., Stetts, D. M., Keenan, G., and Greenway, E. H. (2007). Electromyographical analysis of selected lower extremity muscles during 5 unilateral weight-bearing exercises. J. Orthop. Sports Phys. Ther. 37, 48–55. doi: 10.2519/jospt.2007.2354
Buckner, S. L., Jessee, M. B., Mattocks, K. T., Mouser, J. G., Counts, B. R., Dankel, S. J., et al. (2016). Determining strength: a case for multiple methods of measurement. Sports Med. 47, 193–195. doi: 10.1007/s40279-016-0580-3
Calatayud, J., Borreani, S., Colado, J. C., Martin, F., Tella, V., and Andersen, L. L. (2015). Bench press and push-up at comparable levels of muscle activity results in similar strength gains. J. Strength Cond. Res. 29, 246–253. doi: 10.1519/JSC.0000000000000589
Cavanagh, P. R. (1974). Electromyography: its use and misuse in physical education. J. Health Phys. Educ. Recreation 45, 61–64.
Clarys, J. P. (2000). Electromyography in sports and occupational settings: an update of its limits and possibilities. Ergonomics 43, 1750–1762. doi: 10.1080/001401300750004159
Clarys, J. P., and Cabri, J. (1993). Electromyography and the study of sports movements: a review. J. Sports Sci. 11, 379–448. doi: 10.1080/02640419308730010
Contreras, B., Vigotsky, A. D., Schoenfeld, B. J., Beardsley, C., and Cronin, J. (2015). A comparison of two gluteus maximus EMG maximum voluntary isometric contraction positions. PeerJ 3:e1261. doi: 10.7717/peerj.1261
Corlan, A. D. (2004). Medline Trend: Automated Yearly Statistics of Pubmed Results for any Query [Online]. Available online at: http://dan.corlan.net/medline-trend.html (Accessed January 1, 2017).
Damas, F., Phillips, S. M., Libardi, C. A., Vechin, F. C., Lixandrão, M. E., Jannig, P. R., et al. (2016). Resistance training-induced changes in integrated myofibrillar protein synthesis are related to hypertrophy only after attenuation of muscle damage. J. Physiol. 594, 5209–5222. doi: 10.1113/JP272472
Dankel, S. J., Mattocks, K. T., Jessee, M. B., Buckner, S. L., Mouser, J. G., and Loenneke, J. P. (2017). Do metabolites that are produced during resistance exercise enhance muscle hypertrophy? Eur. J. Appl. Physiol. 17, 2125–2135. doi: 10.1007/s00421-017-3690-1
de Boer, M. D., Morse, C. I., Thom, J. M., de Haan, A., and Narici, M. V. (2007). Changes in antagonist muscles' coactivation in response to strength training in older women. J. Gerontol. A Biol. Sci. Med. Sci. 62, 1022–1027. doi: 10.1093/gerona/62.9.1022
de Brito Fontana, H., and Herzog, W. (2016). Vastus lateralis maximum force-generating potential occurs at optimal fascicle length regardless of activation level. Eur. J. Appl. Physiol. 116, 1267–1277. doi: 10.1007/s00421-016-3381-3
De Luca, C. J. (1997). The use of surface electromyography in biomechanics. J. Appl. Biomech. 13, 135–163. doi: 10.1123/jab.13.2.135
De Luca, C. J., and Kline, J. C. (2012). Influence of proprioceptive feedback on the firing rate and recruitment of motoneurons. J. Neural Eng. 9:016007. doi: 10.1088/1741-2560/9/1/016007
De Luca, C. J., LeFever, R. S., McCue, M. P., and Xenakis, A. P. (1982). Behaviour of human motor units in different muscles during linearly varying contractions. J. Physiol. 329, 113–128. doi: 10.1113/jphysiol.1982.sp014293
Del Vecchio, A., Negro, F., Felici, F., and Farina, D. (2017). Associations between motor unit action potential parameters and surface EMG features. J. Appl. Physiol. (1985) 123, 835–843. doi: 10.1152/japplphysiol.00482.2017
Desmedt, J. E., and Godaux, E. (1977). Ballistic contractions in man: characteristic recruitment pattern of single motor units of the tibialis anterior muscle. J. Physiol. 264, 673–693. doi: 10.1113/jphysiol.1977.sp011689
Dideriksen, J. L., Enoka, R. M., and Farina, D. (2011). Neuromuscular adjustments that constrain submaximal EMG amplitude at task failure of sustained isometric contractions. J. Appl. Physiol. (1985) 111, 485–494. doi: 10.1152/japplphysiol.00186.2011
Dieterich, A. V., Botter, A., Vieira, T. M., Peolsson, A., Petzke, F., Davey, P., et al. (2017). Spatial variation and inconsistency between estimates of onset of muscle activation from EMG and ultrasound. Sci. Rep. 7:42011. doi: 10.1038/srep42011
Dimitrova, N. A., and Dimitrov, G. V. (2003). Interpretation of EMG changes with fatigue: facts, pitfalls, and fallacies. J. Electromyogr. Kinesiol. 13, 13–36. doi: 10.1016/S1050-6411(02)00083-4
dos Anjos, F. V., Pinto, T. P., Gazzoni, M., and Vieira, T. M. (2017). The spatial distribution of ankle muscles activity discriminates aged from young subjects during standing. Front. Hum. Neurosci. 11:190. doi: 10.3389/fnhum.2017.00190
Earp, J. E., Newton, R. U., Cormie, P., and Blazevich, A. J. (2015). Inhomogeneous quadriceps femoris hypertrophy in response to strength and power training. Med. Sci. Sports Exerc. 47, 2389–2397. doi: 10.1249/MSS.0000000000000669
Eftestøl, E., Egner, I. M., Lunde, I. G., Ellefsen, S., Andersen, T., Sjåland, C., et al. (2016). Increased hypertrophic response with increased mechanical load in skeletal muscles receiving identical activity patterns. Am. J. Physiol. Cell Physiol. 311, C616–C629. doi: 10.1152/ajpcell.00016.2016
Ema, R., Sakaguchi, M., Akagi, R., and Kawakami, Y. (2016). Unique activation of the quadriceps femoris during single- and multi-joint exercises. Eur. J. Appl. Physiol. 116, 1031–1041. doi: 10.1007/s00421-016-3363-5
Ema, R., Wakahara, T., Miyamoto, N., Kanehisa, H., and Kawakami, Y. (2013). Inhomogeneous architectural changes of the quadriceps femoris induced by resistance training. Eur. J. Appl. Physiol. 113, 2691–2703. doi: 10.1007/s00421-013-2700-1
Enoka, R. M., and Duchateau, J. (2015). Inappropriate interpretation of surface EMG signals and muscle fiber characteristics impedes understanding of the control of neuromuscular function. J. Appl. Physiol. (1985) 119, 1516–1518. doi: 10.1152/japplphysiol.00280.2015
Erskine, R. M., Fletcher, G., and Folland, J. P. (2014). The contribution of muscle hypertrophy to strength changes following resistance training. Eur. J. Appl. Physiol. 114, 1239–1249. doi: 10.1007/s00421-014-2855-4
Erskine, R. M., Jones, D. A., Williams, A. G., Stewart, C. E., and Degens, H. (2010). Inter-individual variability in the adaptation of human muscle specific tension to progressive resistance training. Eur. J. Appl. Physiol. 110, 1117–1125. doi: 10.1007/s00421-010-1601-9
Escamilla, R. F., Lewis, C., Bell, D., Bramblet, G., Daffron, J., Lambert, S., et al. (2010). Core muscle activation during Swiss ball and traditional abdominal exercises. J. Orthop. Sports Phys. Ther. 40, 265–276. doi: 10.2519/jospt.2010.3073
Farina, D. (2006). Interpretation of the surface electromyogram in dynamic contractions. Exerc. Sport Sci. Rev. 34, 121–127. doi: 10.1249/00003677-200607000-00006
Farina, D., Arendt-Nielsen, L., and Graven-Nielsen, T. (2005). Experimental muscle pain decreases voluntary EMG activity but does not affect the muscle potential evoked by transcutaneous electrical stimulation. Clin. Neurophysiol. 116, 1558–1565. doi: 10.1016/j.clinph.2005.03.009
Farina, D., Holobar, A., Merletti, R., and Enoka, R. M. (2010). Decoding the neural drive to muscles from the surface electromyogram. Clin. Neurophysiol. 121, 1616–1623. doi: 10.1016/j.clinph.2009.10.040
Farina, D., Merletti, R., and Enoka, R. M. (2014). The extraction of neural strategies from the surface emg: an update. J. Appl. Physiol. (1985) 117, 1215–1230. doi: 10.1152/japplphysiol.00162.2014
Farina, D., Stegeman, D. F., and Merletti, R. (2016). “Biophysics of the generation of EMG signals,” in Surface Electromyography: Physiology, Engineering, and Applications, eds R. Merletti and D. Farina (Hoboken, NJ: John Wiley & Sons, Inc.), 1–24.
Feynman, R. P., Leighton, R. B., and Sands, M. (2011). Six Easy Pieces: Essentials of Physics Explained by Its Most Brilliant Teacher. New York, NY: Basic Books.
Fisher, B. E., Southam, A. C., Kuo, Y. L., Lee, Y. Y., and Powers, C. M. (2016). Evidence of altered corticomotor excitability following targeted activation of gluteus maximus training in healthy individuals. Neuroreport 27, 415–421. doi: 10.1097/WNR.0000000000000556
Fleming, T. R., and DeMets, D. L. (1996). Surrogate end points in clinical trials: are we being misled? Ann. Intern. Med. 125, 605–613. doi: 10.7326/0003-4819-125-7-199610010-00011
Fonseca, R. M., Roschel, H., Tricoli, V., de Souza, E. O., Wilson, J. M., Laurentino, G. C., et al. (2014). Changes in exercises are more effective than in loading schemes to improve muscle strength. J. Strength Cond. Res. 28, 3085–3092. doi: 10.1519/JSC.0000000000000539
Gentil, P., Del Vecchio Fabricio, B., Paoli, A., Schoenfeld Brad, J., and Bottaro, M. (2017). Isokinetic dynamometry and 1RM tests produce conflicting results for assessing alterations in muscle strength, J. Hum. Kinet. 56, 19–27. doi: 10.1515/hukin-2017-0019
Goldberg, A. L., Etlinger, J. D., Goldspink, D. F., and Jablecki, C. (1975). Mechanism of work-induced hypertrophy of skeletal muscle. Med. Sci. Sports 7, 185–198.
Gottlieb, G. L., Corcos, D. M., Agarwal, G. C., and Latash, M. L. (1990). “Principles underlying single-joint movement strategies,” in Multiple Muscle Systems: Biomechanics and Movement Organization, eds J. M. Winters and S. L. Y. Woo (New York, NY: Springer New York), 236–250.
Graven-Nielsen, T., Svensson, P., and Arendt-Nielsen, L. (1997). Effects of experimental muscle pain on muscle activity and co-ordination during static and dynamic motor function. Electroencephalogr. Clin. Neurophysiol. 105, 156–164. doi: 10.1016/S0924-980X(96)96554-6
Halperin, I., Vigotsky, A. D., Foster, C., and Pyne, D. B. (2017). Strengthening the practice of exercise and sport science. Int. J. Sports Physiol. Perform 8, 1–26. doi: 10.1123/ijspp.2017-0322
Heckman, C. J., Mottram, C., Quinlan, K., Theiss, R., and Schuster, J. (2009). Motoneuron excitability: the importance of neuromodulatory inputs. Clin. Neurophysiol. 120, 2040–2054. doi: 10.1016/j.clinph.2009.08.009
Hermens, H. J., Freriks, B., Merletti, R., Stegeman, D., Blok, J., Rau, G., et al. (1999). European Recommendations for Surface Electromyography. Enschede: Roessingh Research and Development.
Herzog, W. (2004). History dependence of skeletal muscle force production: implications for movement control. Hum. Mov. Sci. 23, 591–604. doi: 10.1016/j.humov.2004.10.003
Herzog, W., Leonard, T., Joumaa, V., DuVall, M., and Panchangam, A. (2012). The three filament model of skeletal muscle stability and force production. Mol. Cell. Biomech. 9, 175–191. doi: 10.3970/mcb.2012.009.175
Herzog, W., Powers, K., Johnston, K., and Duvall, M. (2015). A new paradigm for muscle contraction. Front. Physiol. 6:174. doi: 10.3389/fphys.2015.00174
Hill, A. V. (1938). The heat of shortening and the dynamic constants of muscle. Proc. R. Soc. B Biol. Sci. 126, 136–195. doi: 10.1098/rspb.1938.0050
Holm, L., van Hall, G., Rose, A. J., Miller, B. F., Doessing, S., Richter, E. A., et al. (2010). Contraction intensity and feeding affect collagen and myofibrillar protein synthesis rates differently in human skeletal muscle. Am. J. Physiol. Endocrinol. Metab. 298, E257–269. doi: 10.1152/ajpendo.00609.2009
Hug, F., and Tucker, K. (2017). “Surface electromyography to study muscle coordination,” in Handbook of Human Motion, eds B. Müller, S. I. Wolf, G.-P. Brueggemann, Z. Deng, A. McIntosh, F. Miller, and W. S. Selbie (Cham: Springer International Publishing), 1–21.
Huxley, A. F. (1957). Muscle structure and theories of contraction. Prog. Biophys. Biophys. Chem. 7, 255–318.
ISEK (2017). Standards for reporting EMG data. J. Electromyogr. Kinesiol. 35, I–II. doi: 10.1016/S1050-6411(17)30219-5
Jenkins, N. D., Housh, T. J., Bergstrom, H. C., Cochrane, K. C., Hill, E. C., Smith, C. M., et al. (2015). Muscle activation during three sets to failure at 80 vs. 30 % 1RM resistance exercise. Eur. J. Appl. Physiol. 115, 2335–2547. doi: 10.1007/s00421-015-3214-9
Jenkins, N. D. M., Miramonti, A. A., Hill, E. C., Smith, C. M., Cochrane-Snyman, K. C., Housh, T. J., et al. (2017). Greater neural adaptations following high- vs. low-load resistance training. Front. Physiol. 8:331. doi: 10.3389/fphys.2017.00331
Kuiken, T. A., Lowery, M. M., and Stoykov, N. S. (2003). The effect of subcutaneous fat on myoelectric signal amplitude and cross-talk. Prosthet. Orthot. Int. 27, 48–54. doi: 10.3109/03093640309167976
Kukulka, C. G., and Clamann, H. P. (1981). Comparison of the recruitment and discharge properties of motor units in human brachial biceps and adductor pollicis during isometric contractions. Brain Res. 219, 45–55. doi: 10.1016/0006-8993(81)90266-3
Kumar, V., Selby, A., Rankin, D., Patel, R., Atherton, P., Hildebrandt, W., et al. (2009). Age-related differences in the dose-response relationship of muscle protein synthesis to resistance exercise in young and old men. J. Physiol. 587, 211–217. doi: 10.1113/jphysiol.2008.164483
Le Mansec, Y., Nordez, A., Dorel, S., and Jubeau, M. (2017). Reaction time can be measured during voluntary contractions with electrode array. Clin. Physiol. Funct. Imaging. doi: 10.1111/cpf.12455
Lieber, R. L., and Fridén, J. (2000). Functional and clinical significance of skeletal muscle architecture. Muscle Nerve 23, 1647–1666. doi: 10.1002/1097-4598(200011)23:11<1647::AID-MUS1>3.0.CO;2-M
Lloyd, D. G., and Besier, T. F. (2003). An EMG-driven musculoskeletal model to estimate muscle forces and knee joint moments in vivo. J. Biomech. 36, 765–776. doi: 10.1016/S0021-9290(03)00010-1
Lohse, K. R., Sherwood, D. E., and Healy, A. F. (2011). Neuromuscular effects of shifting the focus of attention in a simple force production task. J. Mot. Behav. 43, 173–184. doi: 10.1080/00222895.2011.555436
Looney, D. P., Kraemer, W. J., Joseph, M. F., Comstock, B. A., Denegar, C. R., Flanagan, S. D., et al. (2016). Electromyographical and perceptual responses to different resistance intensities in a squat protocol: does performing sets to failure with light loads produce the same activity? J. Strength Cond. Res. 30, 792–799. doi: 10.1519/JSC.0000000000001109
Macaluso, A., Nimmo, M. A., Foster, J. E., Cockburn, M., McMillan, N. C., and De Vito, G. (2002). Contractile muscle volume and agonist-antagonist coactivation account for differences in torque between young and older women. Muscle Nerve 25, 858–863. doi: 10.1002/mus.10113
Maeo, S., Kanemoto, W., Miyazaki, K., and Yamamoto, M. (2012). Muscular activities during uphill and downhill treadmill walking which stimulates mountaineering with respect to changes in slope, velocity, and backpack load. Jpn. J. Mountain Med. 32, 103–105.
Maeo, S., Yamamoto, M., and Kanehisa, H. (2015). Muscular adaptations to short-term low-frequency downhill walking training. Int. J. Sports Med. 36, 150–156. doi: 10.1055/s-0034-1385885
Maeo, S., Yamamoto, M., and Kanehisa, H. (2016). Downhill walking training with and without exercise-induced muscle damage similarly increase knee extensor strength. J. Sports Sci. 34, 2018–2026. doi: 10.1080/02640414.2016.1149607
Marcotte, G. R., West, D. W., and Baar, K. (2015). The molecular basis for load-induced skeletal muscle hypertrophy. Calcif. Tissue Int. 96, 196–210. doi: 10.1007/s00223-014-9925-9
Merletti, R., Holobar, A., and Farina, D. (2008). Analysis of motor units with high-density surface electromyography. J. Electromyogr. Kinesiol. 18, 879–890. doi: 10.1016/j.jelekin.2008.09.002
Merletti, R., Vieira, T. M., and Farina, D. (2016). “Techniques for information extraction from the surface EMG signalhigh-density surface EMG,” in Surface Electromyography: Physiology, Engineering, and Applications, eds R. Merletti and D. Farina (Hoboken, NJ: John Wiley & Sons, Inc.), 126–157.
Mesin, L., Merlo, E., Merletti, R., and Orizio, C. (2010). Investigation of motor unit recruitment during stimulated contractions of tibialis anterior muscle. J. Electromyogr. Kinesiol. 20, 580–589. doi: 10.1016/j.jelekin.2009.11.008
Mitchell, C. J., Churchward-Venne, T. A., Parise, G., Bellamy, L., Baker, S. K., Smith, K., et al. (2014). Acute post-exercise myofibrillar protein synthesis is not correlated with resistance training-induced muscle hypertrophy in young men. PLoS ONE 9:e89431. doi: 10.1371/journal.pone.0089431
Mitchell, C. J., Churchward-Venne, T. A., West, D. W., Burd, N. A., Breen, L., Baker, S. K., et al. (2012). Resistance exercise load does not determine training-mediated hypertrophic gains in young men. J. Appl. Physiol. (1985) 113, 71–77. doi: 10.1152/japplphysiol.00307.2012
Moritani, T., and deVries, H. A. (1979). Neural factors versus hypertrophy in the time course of muscle strength gain. Am. J. Phys. Med. 58, 115–130.
Morton, R. W., Oikawa, S. Y., Wavell, C. G., Mazara, N., McGlory, C., Quadrilatero, J., et al. (2016). Neither load nor systemic hormones determine resistance training-mediated hypertrophy or strength gains in resistance-trained young men. J. Appl. Physiol. (1985) 121, 129–138. doi: 10.1152/japplphysiol.00154.2016
Noorkõiv, M., Nosaka, K., and Blazevich, A. J. (2014). Neuromuscular adaptations associated with knee joint angle-specific force change. Med. Sci. Sports Exerc. 46, 1525–1537. doi: 10.1249/MSS.0000000000000269
Pieter Clarys, J., Scafoglieri, A., Tresignie, J., Reilly, T., and Van Roy, P. (2010). Critical appraisal and hazards of surface electromyography data acquisition in sport and exercise. Asian J. Sports Med. 1, 69–80. doi: 10.5812/asjsm.34868
Potvin, J. R., and Fuglevand, A. J. (2017). A motor unit-based model of muscle fatigue. PLoS Comput. Biol. 13:e1005581. doi: 10.1371/journal.pcbi.1005581
Potvin, J. R., Norman, R. W., and McGill, S. M. (1996). Mechanically corrected EMG for the continuous estimation of erector spinae muscle loading during repetitive lifting. Eur. J. Appl. Physiol. Occup. Physiol. 74, 119–132. doi: 10.1007/BF00376504
Prentice, R. L. (1989). Surrogate endpoints in clinical trials: definition and operational criteria. Stat. Med. 8, 431–440. doi: 10.1002/sim.4780080407
Reiman, M. P., Bolgla, L. A., and Loudon, J. K. (2012). A literature review of studies evaluating gluteus maximus and gluteus medius activation during rehabilitation exercises. Physiother. Theory Pract. 28, 257–268. doi: 10.3109/09593985.2011.604981
Roberts, T. J., and Gabaldón, A. M. (2008). Interpreting muscle function from EMG: lessons learned from direct measurements of muscle force. Integr. Comp. Biol. 48, 312–320. doi: 10.1093/icb/icn056
Santana, J. C., Vera-Garcia, F. J., and McGill, S. M. (2007). A kinetic and electromyographic comparison of the standing cable press and bench press. J. Strength Cond. Res. 21, 1271–1277. doi: 10.1519/R-20476.1
Sartori, M., Lloyd, D. G., Besier, T. F., Fernandez, J. W., and Farina, D. (2016). “Electromyography-driven modeling for simulating subject-specific movement at the neuromusculoskeletal level,” in Surface Electromyography: Physiology, Engineering, and Applications, eds R. Merletti and D. Farina (Hoboken, NJ: John Wiley & Sons, Inc.), 247–272.
Schoenfeld, B. J., Contreras, B., Vigotsky, A. D., Ogborn, D., Fontana, F., and Tiryaki-Sonmez, G. (2016). Upper body muscle activation during low-versus high-load resistance exercise in the bench press. Isokinet. Exerc. Sci. 24, 217–224. doi: 10.3233/IES-160620
Schoenfeld, B. J., Contreras, B., Willardson, J. M., Fontana, F., and Tiryaki-Sonmez, G. (2014). Muscle activation during low- versus high-load resistance training in well-trained men. Eur. J. Appl. Physiol. 114, 2491–2497. doi: 10.1007/s00421-014-2976-9
Schoenfeld, B. J., Grgic, J., Ogborn, D., and Krieger, J. W. (2017). Strength and hypertrophy adaptations between low- vs. high-load resistance training: a systematic review and meta-analysis. J. Strength Cond. Res. 31, 3508–3523. doi: 10.1519/JSC.0000000000002200
Schoenfeld, B. J., Peterson, M. D., Ogborn, D., Contreras, B., and Sonmez, G. T. (2015). Effects of low- versus high-load resistance training on muscle strength and hypertrophy in well-trained men. J. Strength Cond. Res. 29, 2954–2963. doi: 10.1519/JSC.0000000000000958
Seiberl, W., Power, G. A., Herzog, W., and Hahn, D. (2015). The stretch-shortening cycle (SSC) revisited: residual force enhancement contributes to increased performance during fast SSCs of human m. adductor pollicis. Physiol Rep 3:e12401. doi: 10.14814/phy2.12401
Simpson, C. L., Kim, B. D. H., Bourcet, M. R., Jones, G. R., and Jakobi, J. M. (2017). Stretch training induces unequal adaptation in muscle fascicles and thickness in medial and lateral gastrocnemii. Scand. J. Med. Sci. Sports. doi: 10.1111/sms.12822. [Epub ahead of print].
Soderberg, G. L., and Knutson, L. M. (2000). A guide for use and interpretation of kinesiologic electromyographic data. Phys. Ther. 80, 485–498. doi: 10.1093/ptj/80.5.485
Sola, O. M., Christensen, D. L., and Martin, A. W. (1973). Hypertrophy and hyperplasia of adult chicken anterior latissimus dorsi muscles following stretch with and without denervation. Exp. Neurol. 41, 76–100. doi: 10.1016/0014-4886(73)90182-9
Staudenmann, D., Roeleveld, K., Stegeman, D. F., and van Dieën, J. H. (2010). Methodological aspects of SEMG recordings for force estimation–a tutorial and review. J. Electromyogr. Kinesiol. 20, 375–387. doi: 10.1016/j.jelekin.2009.08.005
Steele, K. M., Rozumalski, A., and Schwartz, M. H. (2015). Muscle synergies and complexity of neuromuscular control during gait in cerebral palsy. Dev. Med. Child Neurol. 57, 1176–1182. doi: 10.1111/dmcn.12826
Trajano, G. S., Seitz, L., Nosaka, K., and Blazevich, A. J. (2013). Contribution of central vs. peripheral factors to the force loss induced by passive stretch of the human plantar flexors. J. Appl. Physiol. (1985) 115, 212–218. doi: 10.1152/japplphysiol.00333.2013
Trezise, J., Collier, N., and Blazevich, A. J. (2016). Anatomical and neuromuscular variables strongly predict maximum knee extension torque in healthy men. Eur. J. Appl. Physiol. 116, 1159–1177. doi: 10.1007/s00421-016-3352-8
Tsaklis, P., Malliaropoulos, N., Mendiguchia, J., Korakakis, V., Tsapralis, K., Pyne, D., et al. (2015). Muscle and intensity based hamstring exercise classification in elite female track and field athletes: implications for exercise selection during rehabilitation. Open Access J. Sports Med. 6, 209–217. doi: 10.2147/OAJSM.S79189
van Bolhuis, B. M., Medendorp, W. P., and Gielen, C. C. (1997). Motor unit firing behavior in human arm flexor muscles during sinusoidal isometric contractions and movements. Exp. Brain Res. 117, 120–130. doi: 10.1007/s002210050205
Vera-Garcia, F. J., Moreside, J. M., and McGill, S. M. (2010). MVC techniques to normalize trunk muscle EMG in healthy women. J. Electromyogr. Kinesiol. 20, 10–16. doi: 10.1016/j.jelekin.2009.03.010
Vieira, T. M., Botter, A., Muceli, S., and Farina, D. (2017). Specificity of surface EMG recordings for gastrocnemius during upright standing. Sci. Rep. 7:13300. doi: 10.1038/s41598-017-13369-1
Vigotsky, A. D., Harper, E. N., Ryan, D. R., and Contreras, B. (2015). Effects of load on good morning kinematics and EMG activity. Peer J 3:e708. doi: 10.7717/peerj.708
Vigotsky, A. D., Ogborn, D., and Phillips, S. M. (2016). Motor unit recruitment cannot be inferred from surface EMG amplitude and basic reporting standards must be adhered to. Eur. J. Appl. Physiol. 116, 657–658. doi: 10.1007/s00421-015-3314-6
Wernbom, M., Augustsson, J., and Thomeé, R. (2007). The influence of frequency, intensity, volume and mode of strength training on whole muscle cross-sectional area in humans. Sports Med. 37, 225–264. doi: 10.2165/00007256-200737030-00004
West, D. W., and Phillips, S. M. (2012). Associations of exercise-induced hormone profiles and gains in strength and hypertrophy in a large cohort after weight training. Eur. J. Appl. Physiol. 112, 2693–2702. doi: 10.1007/s00421-011-2246-z
Winter, D. A., Rau, G., Kadefors, R., Broman, H., and de Luca, C. J. (1980). Units, Terms and Standards in the Reporting of EMG Research. A Report by the Ad Hoc Committee of the International Society of Electrophysiology and Kinesiology, Department of Medical Research, Rehabilitation Institute of Montreal, Montreal.
Winters, J. M. (1990). “Hill-based muscle models: a systems engineering perspective,” in Multiple Muscle Systems: Biomechanics and Movement Organization, eds J. M. Winters and S. L. Y. Woo (New York, NY: Springer New York), 69–93.
Yamashita, N. (1988). EMG activities in mono- and bi-articular thigh muscles in combined hip and knee extension. Eur. J. Appl. Physiol. Occup. Physiol. 58, 274–277. doi: 10.1007/BF00417262
Zajac, F. E. (1989). Muscle and tendon: properties, models, scaling, and application to biomechanics and motor control. Crit. Rev. Biomed. Eng. 17, 359–411.
Keywords: strength, hypertrophy, rate coding, motor unit recruitment, exercise, activation, excitation, muscle force
Citation: Vigotsky AD, Halperin I, Lehman GJ, Trajano GS and Vieira TM (2018) Interpreting Signal Amplitudes in Surface Electromyography Studies in Sport and Rehabilitation Sciences. Front. Physiol. 8:985. doi: 10.3389/fphys.2017.00985
Received: 17 September 2017; Accepted: 17 November 2017;
Published: 04 January 2018.
Edited by:
Billy Sperlich, Integrative & Experimentelle Trainingswissenschaft, Universität Würzburg, GermanyReviewed by:
Christian Baumgart, University of Wuppertal, GermanyCopyright © 2018 Vigotsky, Halperin, Lehman, Trajano and Vieira. This is an open-access article distributed under the terms of the Creative Commons Attribution License (CC BY). The use, distribution or reproduction in other forums is permitted, provided the original author(s) or licensor are credited and that the original publication in this journal is cited, in accordance with accepted academic practice. No use, distribution or reproduction is permitted which does not comply with these terms.
*Correspondence: Andrew D. Vigotsky, YXZpZ290c2t5QGdtYWlsLmNvbQ==
Disclaimer: All claims expressed in this article are solely those of the authors and do not necessarily represent those of their affiliated organizations, or those of the publisher, the editors and the reviewers. Any product that may be evaluated in this article or claim that may be made by its manufacturer is not guaranteed or endorsed by the publisher.
Research integrity at Frontiers
Learn more about the work of our research integrity team to safeguard the quality of each article we publish.