- 1 CAP Center for Cognition, Action and Perception, Department of Psychology, University of Cincinnati, Cincinnati, OH, USA
- 2 Department of Psychology, Grant MacEwan University, Edmonton, AB, Canada
- 3 MINDLab, Aarhus University, Aarhus, Denmark
Much effort has gone into elucidating control of the body by the brain, less so the role of the body in controlling the brain. This essay develops the idea that the brain does a great deal of work in the service of behavior that is controlled by the body, a blue-collar role compared to the white-collar control exercised by the body. The argument that supports a blue-collar role for the brain is also consistent with recent discoveries clarifying the white-collar role of synergies across the body’s tensegrity structure, and the evidence of critical phenomena in brain and behavior.
Introduction
Lloyd Olsen shared fame in the 1940s with Mike the celebrity headless chicken. Mike’s head was lost when he was five and a half months old while being prepared by Lloyd to become chicken dinner. Mike lived on without a head for 18 months, fed through an eyedropper and growing from two pounds, at his beheading, to eight pounds at his death. In the mean time he traveled widely performing in New York City, Los Angeles, Atlantic City, and elsewhere. Our interest in Mike is the demonstrated coordination among the processes of his body, despite lacking a head. What was left of Mike’s brain – he probably still had a brainstem – would have marked a handkerchief somewhat less than a healthy sneeze. But he nonetheless retained the coordination among peripheral nervous system, organ systems, facia, muscles, and tendons, producing locomotion apparently indistinguishable from intact locomotion, even walking around and “pecking” right after losing his head.
To us Mike demonstrates that high-level control of the body has sources in addition to the central nervous system. An environment of constant red light, in a different demonstration, created feckless chickens. The steady-state environment obviated the chickens’ connection to the daily cycles of sunrise and sunset, and the pace markers or zeitgebers of the body’s circadian rhythm. Consequently the chickens suffered a breakdown of healthy coordination among the rhythms of physiology, including heart rate and cycles of deep body temperature, and the coupling of physiology with locomotor activities (Winget et al., 1968). Apparently, chicken physiology and behavior include necessary sources of control in the daily cycles of a circadian environment.
A sea squirt starts life as a rather simple tadpole-like creature, possessing a simple nervous system, and capable of locomotion and light detection. However, finding a surface upon which it can affix itself, the sea squirt will do so, and promptly ingest its nervous system (Birkeland et al., 1981). This sea squirt example, like the chicken examples, speaks again to sources of control of the body and behavior in addition to and distinct from the central nervous system. Deprivation of sleep cycles or nutrients illustrates this idea in human behavior. These deprivations destabilize human emotional control, increasing emotional lability. The effect is sufficiently reliable to have become a mainstay of the weekend initiation rituals of cults and extreme self-help programs. Initiates are kept awake in a common room without food for 24 h, which opens them up emotionally, becoming more receptive to the program being sold.
These examples all bear relationships to general theories of control, whether that of cybernetics or non-linear dynamics of self-organization (cf. Simon, 1973; Haken, 1977; Newell, 1990; Schiepek and Haken, 2006, respectively). The feckless chickens probably illustrate this relationship best. The faster changing processes of physiology are constrained in their coordination by the more slowly changing circadian rhythm – generally speaking, more slowly changing dynamics constrain faster dynamics, not vice versa. In self-organization, a key distinction between control and order parameters versus state dynamics is based on how fast one changes with respect to the other. Order parameters are defined to be particular configurations of state dynamics, which means they must change more slowly than state dynamics.
Thus the pacing of the phenomena of the body and brain, with respect to behavior, can tell us which processes constrain which in enacting behavior. Nonetheless, the idea that the body or behavior might control the brain, when first heard, may sound outrageous, depending on what you already believe about control, the brain, and behavior. The most widely held conventional belief is that the brain controls behavior, not the other way around. Yet, when compared with the lightening fast changes in the brain, the typically more slowly changing body suggests the exact opposite broad-stroke outline of control. The brain appears to take direction from the body, just as old school blue-collar workers took direction from white-collar counterparts in the front office.
This issue of Frontiers of Fractal Physiology is about critical phenomena of the brain. A close look at the critical phenomenon of fractal time suggests that the brain serves the blue-collar role in broad circumstances of on-going behavior. To understand this claim, we must first make explicit the links among related concepts of fractal physiology, criticality, non-linear dynamics, tensegrity, synergy, and control. The integrated ideas are that control of behavior originates in constraints on behavior changing on different timescales, and that constraints simultaneously sustain and are sustained by the emergent phenomena in which they participate.
Human Performance Data
To begin we require an understanding of fractal time. Fractal time is a performance phenomenon, so in this section we re-examine the basic idea of measurement of human performance together with the idea of critical states separating qualitatively different modes of behavior. Following that we describe how fractal time appears in brains and behaviors and how the body has been proposed to be an excitable medium of self-organizing synergies. It is the synergies of the body that also constrain the brain during behavior. Finally, we summarize conclusions that appear to us to be the logical consequences of a blue-collar perspective on the brain.
Cognitive scientists may tell you that they study human performance of specific cognitive functions such as memory, language, or motor control. In actual practice we study the measurements of a person performing a “memory” task, a “language” task, or a “motor” task. Yet all task performances are motor performances and language is ubiquitous in the instructions to participants, which must tax memory to be remembered when performing the somewhat arbitrary laboratory task. So most of the time, and maybe all of the time, the scientists who study cognitive activities study the coordination over time among memory, language, and motor activities.
In particular, scientists are concerned with the reliable changes that they observe in the measurements that they take, which is true of cognitive scientists as well. The measurements that we take in cognitive science range from millisecond-precise durations of event times in human activities to nominal measurements that tally which category an observed behavior is assumed to represent. In all cases it is patterns of change or variation in the measured values that are scrutinized and interpreted to motivate interesting conclusions and to test the hypotheses that stem from scientific theories.
Early in the twentieth century scientists derived powerful statistical tools with which to carve out the patterns in data, based on idealized assumptions about the central tendencies of data and uniform dispersion of data values around a central tendency – as though an average behavior of a system could be found reliably at the center of the noisily dispersed measured values, falling equally on all sides, though less densely, outward from the center. With hindsight as a crystal ball, the twentieth century the picture of data was neatly generalized to become chaos theory or non-linear dynamical systems theory. Linear patterns of change in data, in which related changes were also proportional changes, one to another, were neatly absorbed as special cases of broader categories of non-linear disproportional change and discontinuous change.
In bifurcation theory, a tiny external change can break a balanced symmetry of possible outcomes, resulting in a qualitative change called a bifurcation. Bifurcation theory concerns the relation between locally continuous or incremental changes in control parameters and the abrupt fast qualitative restructuring that they may provoke. The tipping point of a bifurcation is a critical point and the behavior of systems near critical points is called criticality. The empirical foci of this essay are the observed scale-free behaviors of body and brain, predicted to occur near the critical bifurcation points of complex systems.
Scale-Free Behavior of the Brain and the Body
Multicellular living things comprise nested structures. The toes and fingers at the small-scale periphery of the human skeleton are composed of small toe and finger bones coupled by small articulating joints. Toes and fingers are nested within the next scale of rigid bones of arms and legs that are coupled by larger articulating joints. Arms and legs in turn sprout from the trunk of the human body and are connected to the trunk by rotating joints at the hips and shoulders. Similarly, viewing a tree we can see that leaves are nested within the structure of small branches that are nested, in turn, within the structure of larger and yet larger branches that culminate in its largest branches, sprouting from the tree trunk.
The anatomy of blood vessels throughout the body, the detailed anatomy of a kidney, and the airways of a lung all comprise nested tree-structures across multiple scales – an arrangement called fractal structure that is studied using the mathematical tools of fractal geometry. The scaling relations that define the spatial organization of living things indicate their fractal composition. In a scaling relation, the size of a structure is inversely proportional to how often structures of that same size recur. For example, within limits, the diameter of each blood vessel is inversely proportional to the total number of blood vessels of that same diameter that will be found in the body (West, 2006).
The structure of the body provides specific physical limits on possible behavior. Scaling relations seen in the body are accompanied by scaling relations in the temporal unfolding of behavior. However, these constraints are not unidirectional. Physical structure and temporal behavior are mutually dependent. Typical physiological and neural development in young children (e.g., Hausdorff et al., 1999; Thelen et al., 2001) and change due to neurodegenerative disorders (e.g., Schmit et al., 2006), as well as musculature change in adults (e.g., Schmit et al., 2005), all shape the temporal structure of behavior. Likewise, behavior shapes both small-scale neural structure (e.g., Maguire et al., 2000) and larger scale muscular and cardiovascular structure, with exercise for instance.
Event times of both human physiology and human behavior compose temporal scaling relations. In the scaling relations of event times, the magnitude of changes in the duration of event times is inversely proportional to how often a change of that magnitude recurs. Figure 1 portrays a physiological data series of brain activity to illustrate a scaling relation of fractal time. Across the top of Figure 1 we present raw electroencephalogram (EEG) data from a volunteer, collected from an electrode on his scalp while he performed the task of repeatedly estimating a 1 s time interval.
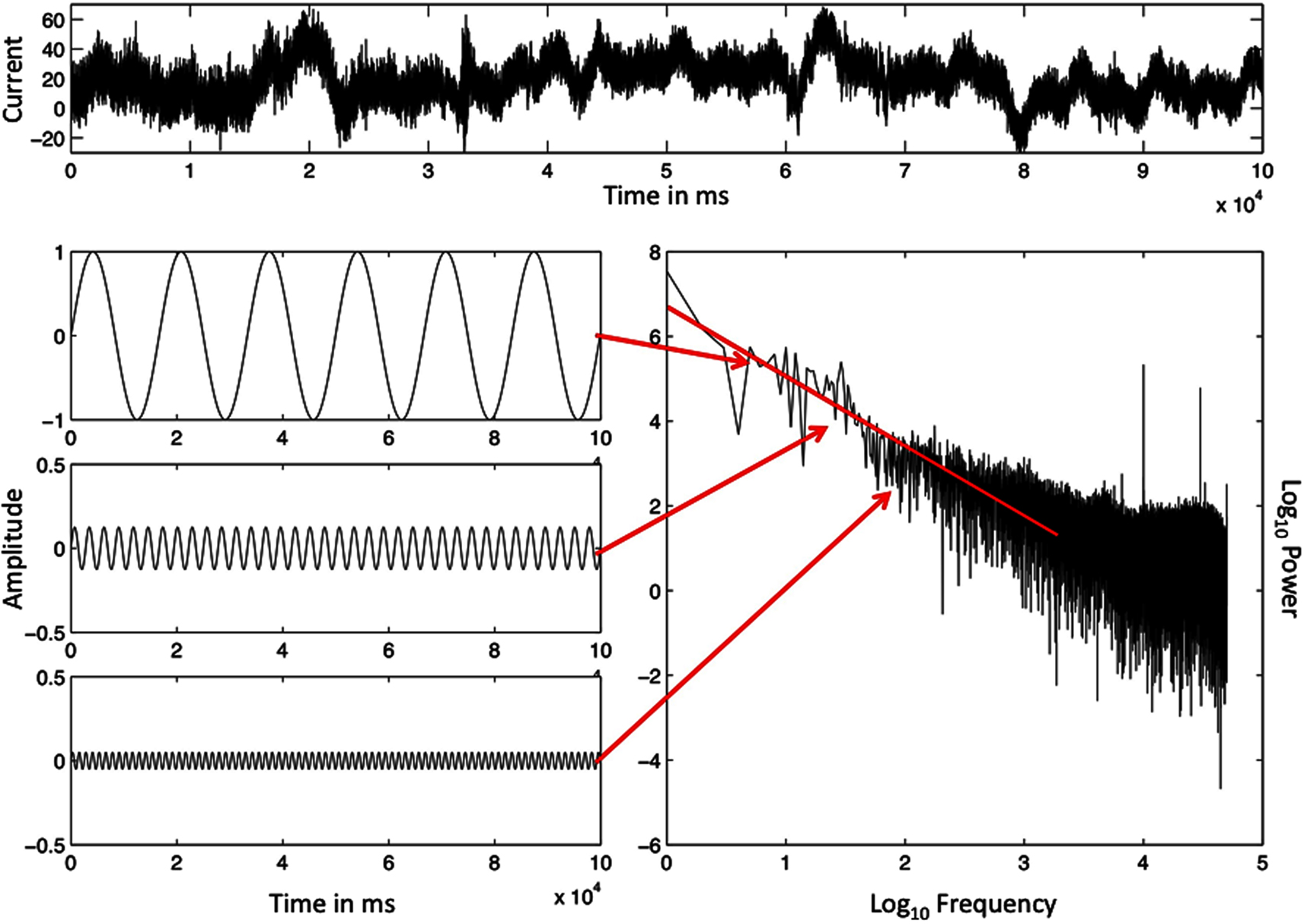
Figure 1. The ordered series of a single EEG-electrode record, sampled at 500 Hz (top) and the illustration of a spectral analysis of this record (bottom, right). Specific frequencies and magnitudes of change (bottom, left) are used to approximate the rough graph of the EEG data (top), and the outcome is the spectral portrait (lower right) on log–log axes. The spectral slope −α = −1.08 is close to idealized 1/f noise (−α = −1.00). The Y-axes in the illustrated sine waves have been adjusted to make smaller amplitude sine waves visible.
The bottom, left side of Figure 1 portrays a subset of the periodic sine waves used to simulate the aperiodic EEG signal. Arrows extend from each sine wave to its paired coordinate point in a power spectral graph, appearing below the raw EEG data. The amplitude and frequency of each sine wave become the two coordinates of a single point in the power spectral graph. The amplitude of the sine wave (squared) corresponds to the power or magnitude of changes in the data values that the sine wave simulates. The frequency of the sine wave estimates how often the changes of that magnitude recur.
Frequency of change and magnitude of change are the coordinate X- and Y-axes of the power spectral graph (after logarithmic transformations). Thus the power spectral graph presents a relation between the magnitude, or power, of the changes and the corresponding frequency of changes of that magnitude. The regression line, also portrayed in Figure 1, summarizes this relationship. The slope of the line in Figure 1 indicates scale-free behavior because power is proportional to frequency. Data like these are called scale-free because the data pattern will look similar whether the vantage point of the analysis zooms in, to a finer scale, or zooms out, to a coarser scale.
The scale-free pattern of the data in Figure 1 is further illustrated in Figure 2 by repeatedly zooming in to examine the middle half of the time-series of the EEG data. Each tighter frame on the EEG data reveals another self-similar pattern in the variation. This self-similar pattern is the predominant pattern of variation in EEG data and begs to be explained with a high priority. An explanation may begin with the apparent fact of the fractal pattern, that the same pattern is observed whether the focus is one half of the original data, one fourth of the original data, one eighth of the original data, and so on.
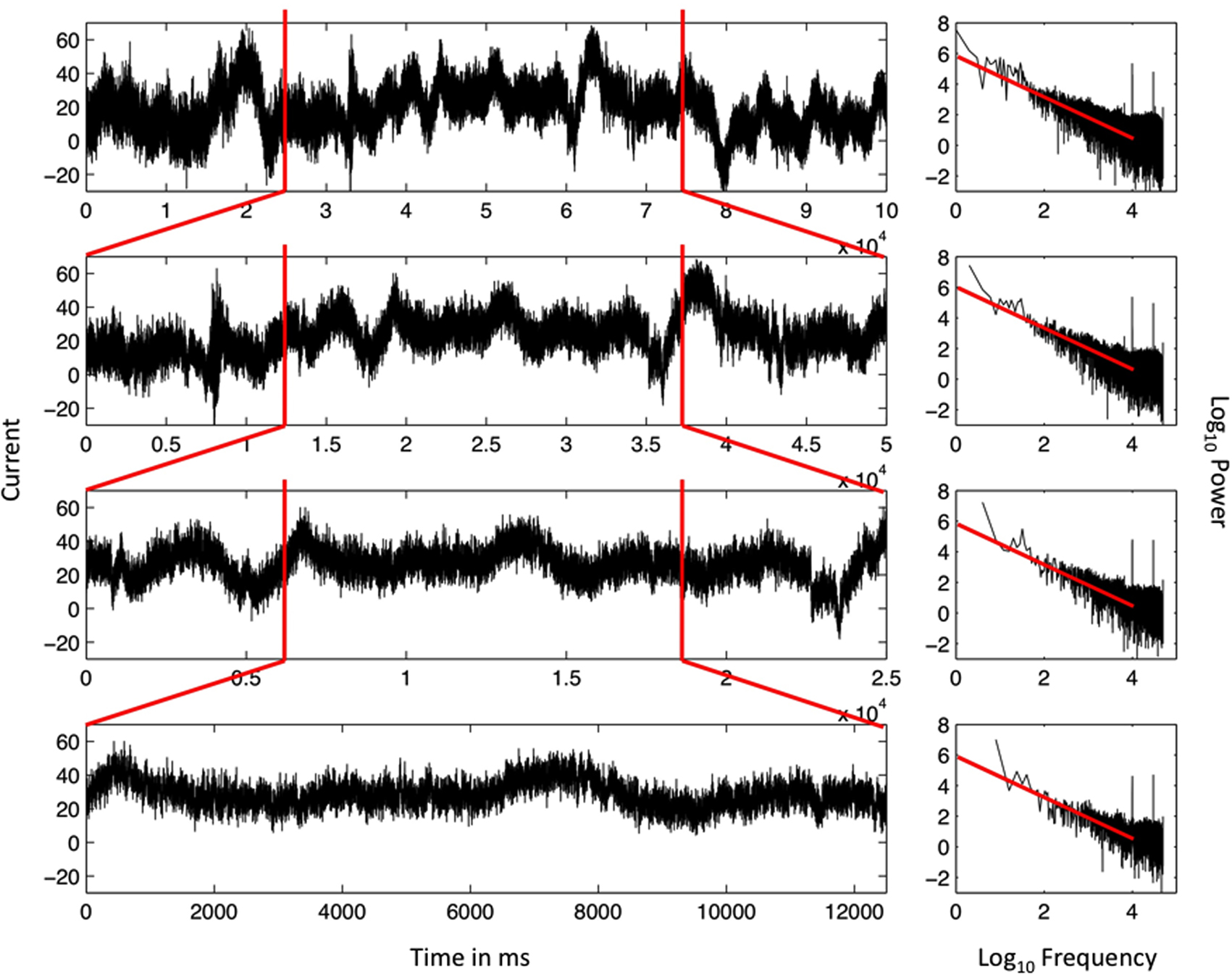
Figure 2. Ordered series of the single EEG-electrode recording from Figure 1 (top, left) and the resulting spectral plot (right) on log–log axes. The top panel includes 16 min of continuous EEG recordings. All other panels are subsets of the original data series. The first and last quarters of each data series are both deleted in each iteration, yielding eventually a data series that ran for 2 min (bottom). The scaling relation remains very similar for each nested series, close to idealized 1/f noise, and demonstrating the statistical self-similarity of the data series.
Another fact begging for explanation is that, similar to the brain data, human performance data reveal a scale-free pattern (cf. Gilden, 2001), although it is possible to manipulate both patterns, to become more like white noise or brown noise (Van Orden et al., 2011; van Rooij and Van Orden, 2011). The performance data of the same volunteer, whose brain data appear in Figures 1 and 2, are portrayed in Figure 3. Each Y-value of a data point in the raw data series of Figure 3 is the estimate produced by the volunteer of the duration of 1 s – the volunteer pressed a key to mark the time of each second’s passing. The raw data are portrayed across the top of Figure 3. Each datum is portrayed in the order in which it was collected; the data value from the first estimated event time is leftmost on the X-axis of the raw data and the data value of the last estimated event time is rightmost on the X-axis.
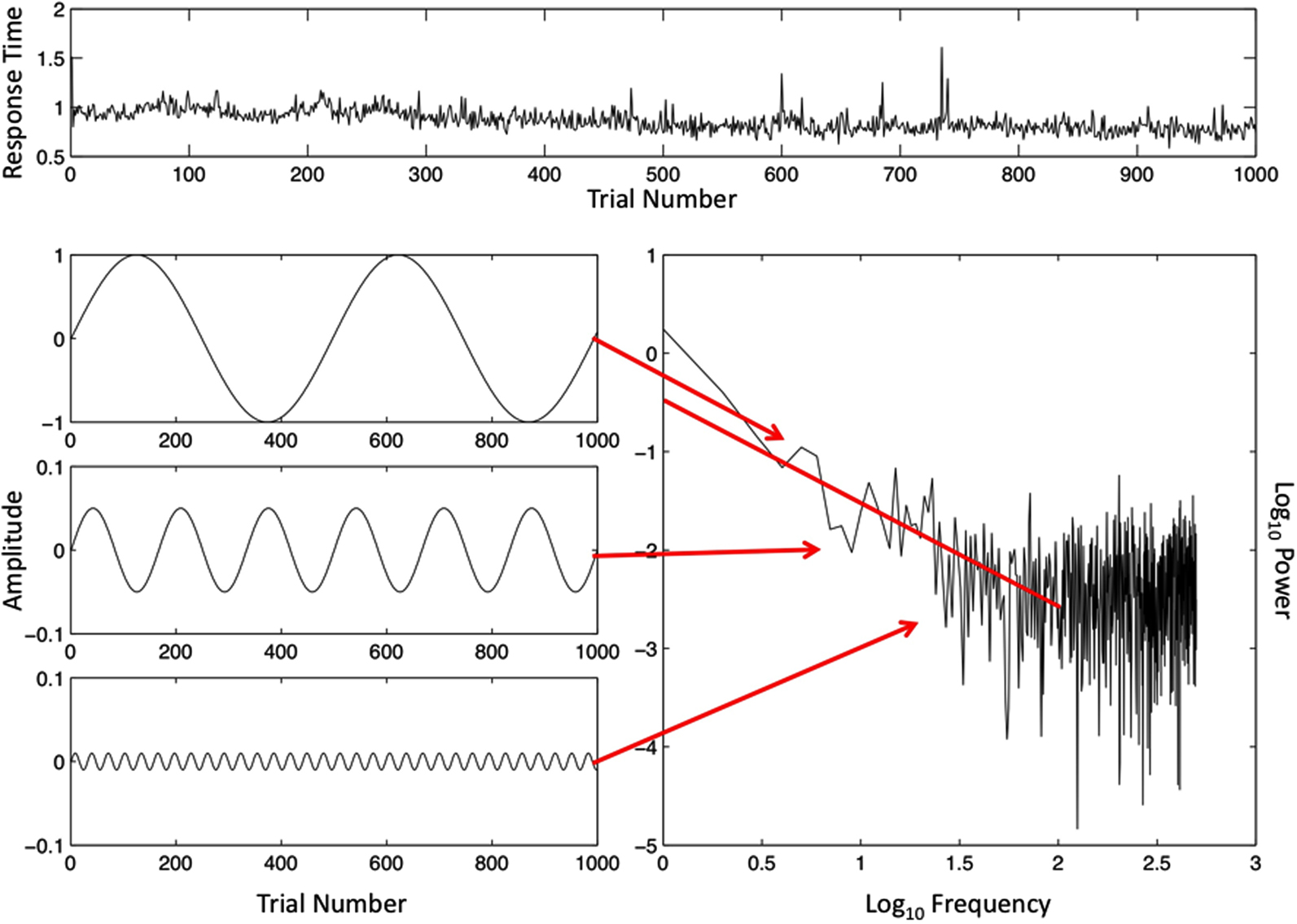
Figure 3. The ordered trial-series of 1000 intervals between the button presses defining time estimates of 1 s (top) and an illustration of a spectral analysis of this time-series (bottom, right). Specific frequencies and magnitudes of change (lower left) are used to approximate the rough graph of the behavioral button-press data (top), and the outcome of the spectral analysis (lower right) on log–log axes. The spectral slope −α = −0.99 is close to idealized 1/f noise (−α = −1.00). The Y-axes in the illustrated sine waves have been adjusted to make smaller amplitude sine waves visible.
A subset of the sine waves that were used to simulate the raw behavioral data series is portrayed on the left side of Figure 3. Each sine wave yielded two coordinates defining a point on the power spectral graph, again below the data series graph. Arrows connect each sine wave to its point coordinates. The amplitude of each sine wave (squared) estimates the size of changes in data values, and the frequency of the sine wave estimates how often changes of that size recur. The logarithms of frequency and size of change (power) are again the respective coordinate X- and Y-axes of the power spectral plot, and the summary regression line again has a slope near minus 1, which translates into a scaling exponent a close to positive 1.
Repeatedly measured data values, whether from brain activity or behavior, are generally scale-free with exponents a ∼ 1, consistent with our examples (for brain see Buzsáki, 2006; for behavior see Newell and Slifkin, 1998; Gilden, 2001, 2009; Riley and Turvey, 2002). This fact, plus the idea of intuitive brain-to-body control, has led to speculation that the scale-free behavior of the brain causes the scale-free variation in behavior, in whole or in part (Raichle and Gusnard, 2005). The speculation is likely false however because the priority of control, as we mentioned already rests on relatively slowly changing constraints and the scale-free behavior of the body includes several orders-of-magnitude slower changes than the co-occurring brain activity. However, it is precisely these fast time scales of brain activity that have been emphasized in the control of behavior. For control to flow this way, from faster to slower time scales, control would require an extra source of influence, in addition to brain dynamics, to amplify the activity of the brain in such a way that it could affect the dynamics of behavior.
Brain activity in the EEG record displays scale-free properties. This means at least two things: first, the magnitude of fluctuations of fast time scales in nervous activity is not sufficient to singlehandedly account for behavioral control. Second, the faster time scales in the brain are constrained by its slower time scales (i.e., long-range traveling waves and neuroplasticity) as well as by the slower time scales on which behavior unfolds. Since the dynamics of brain and behavior both display scaling over a certain temporal range, this might indicate they are measures of the same process at different granularities. Although there is a relevant distinction between behavior and brain activity insofar as our measurement tools allow us to sample their changes at different rates, the issue of a fundamental distinction between “behavior” and “brain activity” is less important than the point that slower changes constrain faster changes.
We created an idealized illustration of how the range of sale-free behavior observed across the time scales of behavior and brain might look together on the same graph, using the duration of the sine wave periods that would suffice to simulate the time scales of variation in repeated measurements of behavior and brain. The idealization appears in Figure 4. The behavioral data fill out the slower region of low-frequency high-power change on the logarithmic X- and Y-axes; the longest data set, to our knowledge, coming from a study lasting over a year (Delignieres et al., 2004). The powerful amplitudes of change in the behavioral data are several orders-of-magnitude larger than those of the brain data. The low amplitude changes of the brain are thus too weak and change too fast to be the causes of the much slower and more strongly varying changes of the body in behavior. Perhaps then the activities of the body somehow “cause” those of the brain.
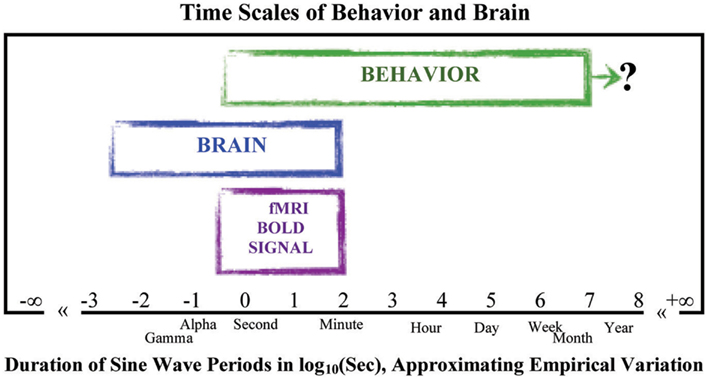
Figure 4. Time scales of behavior and brain were estimated from the time scales implicated in sine wave simulations of variation across repeated measurements (as in Figure 4). Landmarks of durations (day, week, etc.) or brain activity (Alpha, Gamma) are placed near their values in log10(Sec). This figure also includes the span of brain activity observed in the BOLD signal of brain metabolism used in fMRI studies, all to give context to the contrast between the span of time scales observed of behavior and the span of time scales observed of the brain (for reviews see Gilden, 2001; Buzsáki, 2006; Van Orden et al., 2011; van Rooij and Van Orden, 2011). The question mark to the right of the behavioral span symbolizes the fact that no upper bound short of death has yet been discovered in longitudinal studies estimating the presence of scaling relations in the variation across measurements of behavior.
Presence of Mind
Low amplitude changes of the brain are too weak and change too fast to be causes of the much slower and much more strongly varying changes in the body. This claim might sound odd when adopting an overly exclusive “brain controls the body” way of thinking. But it is not odd at all from an engineering perspective. Some engineered systems produce scaling relations in their behavior and the scaling relation characterizes a kind of marriage among different functions of “memory” and “context.” The consequences concern how engineered processes on very different timescales constrain each other in their interaction.
Very slowly changing constraints could appear to be static if seen from the perspective of a very rapidly changing process. But the slow and fast changes are of course concurrent. On the one hand, concurrence allows very slowly changing constraints to serve a kind of memory function for more rapidly changing constraints. Slowly changing constraints remind a rapidly changing process of the constraints coming from the slow timescale, which may change only slightly, or not at all, from the constraints on previous cycles. Slower changes are in this way a means for faster changes to “remember” what they need to know about the status of all the more slowly changing constraints in the system (Keshner, 1982).
On the other hand, very slowly changing constraints also function as a relatively stable context, a slowly changing platform on which rapidly changing dynamics are staged. In this emphasis, the very slowly changing constraints limit the degrees of freedom available to a faster changing process, thus restricting the degrees of freedom for what can happen on faster time scales. The faster changing dynamics must evolve within the limited degrees of freedom that the context leaves available.
The crucial importance of memory and context is reflected in how the brain consumes energy. The brain alone accounts for 20% of the body’s energy consumption (Clarke and Sokoloff, 1999). Yet in a task performance the range of changes in energy consumption in the brain’s activity spans less than 1% of total bodily energy consumption (Raichle, 2010). In other words, our present state of knowledge about energy consumption implies that a complex brainy task requires little- or no-more energy than simply relaxing with eyes closed. This pattern of energy use is consistent with a brain that is primarily about updating and maintaining predictive aspects of history and current events from the lived perspective of the actor.
The facts about energy consumption make clear the importance of the brain “knowing” its place in the world, at any given time. This knowledge could be sustained in positive feedback loops of glutamate cycling (Davia, 2005), and it is estimated that between 60 and 80% of the overall energy consumption of the brain occurs in glutamate cycling (Raichle, 2010). If an actor’s history and context – presence of mind – are sustained in the energy patterns of feedback loops, then the amount of energy dedicated to this blue-collar task is consistent with the importance of support for on-going perception and action. Whether viewed as history or context, the slower the change, the more constant, or stably constraining is the influence of the past.
Slower dynamics thus constrain faster dynamics, which allows the flow of visible or audible, or otherwise available, context to constrain the dynamics the brain. The flow of invariants across perception occurs on the slower time scales of change in brain activity (see Figure 4), supplying constraints that reduce the degrees of freedom for what may happen next. The residual degrees of freedom allowed by a visible checkerboard, for example, slowly changing its position across the visual field on which flickering rings create expanding or contracting traveling waves (1/32 or 1/48 Hz), gives structure to the activity in visual cortex. These slowly changing constraints reveal a more spatially precise picture of retinotopic organization, compared to previous attempts (Engel et al., 1993, 1994, 1997).
We suggest that the brain is primarily about maintaining presence of mind. In our meaning, “presence of mind” includes the present configuration of the body as it is currently entwined in meaningful relations with the present configuration of the world. Relations among configurations are all themselves changing relatively slowly (compared to the brain). Slower changes provide constraints to the brain in the shape of the pattern of energy flow in the brain. Constraints provide knowledge about possible futures, and they are had for free in the immediate status of relations between the body and the world (Stepp and Turvey, 2009).
A Third Category of Phenomena
Behavior and brain share the same scaling relation, which they also share with other measured signals of physiology such as heart rate, colon contraction, transduction at the retina, neural firing, and many others (Glass, 2001). We believe there are fundamental consequences of this shared scaling relation. There are practical advantages for a system to maintain scaling relations in its patterns of change, these advantages place practical constraints on the development of species and organisms, and have staggering consequences for cognitive science.
An empirical scaling relation with scaling exponent a ∼ 1 is approximately the mathematical scaling relation called fractal time, 1/f scaling, or pink noise. It is called pink noise due to a resemblance to the empirical spectral portrait of pink light, which concentrates power in the lower frequencies of red light relative to the higher frequencies of blue light. Pink noise is observed of complex systems near the critical points of bifurcations. By staying near to its critical points, a system sustains a poised attitude, ready at any moment to change the organization of its behavior.
Subtle changes in the relation between the task and the performer are often met by qualitative changes in the organization of performance. The relation between task and performer even shapes the expression of learning disabilities (Hendriks and Kolk, 1997). Encouraged to read aloud very quickly, developmental dyslexics make errors consistent with a deficit in the “lexical” process in reading, producing symptoms of a type of dyslexia that is defined by visual/phonological errors and semantic errors (e.g., POND → /pool/, BUSH → /tree/). When encouraged to read aloud accurately, the same dyslexics produce symptoms of a different type of dyslexia, exhibiting the ponderous letter-by-letter, or syllable-by-syllable reading associated with a “non-lexical” process of reading.
Human performance may undergo a bifurcation between speed versus accuracy conditions, self-organizing a different dynamical system suited for speed than for accuracy (cf. Dutilh et al., 2011; Wijnants et al., in press). This hypothesis is consistent with the two types of dyslexic performance, one under speed conditions and the other under accuracy conditions. These speed-versus-accuracy types also closely parallel the two types of acquired dyslexia that were featured conspicuously in a double dissociation of reading processes that kicked off modern cognitive neuropsychology (Marshall and Newcombe, 1973, 1977). And extreme speed conditions also induce errors by intact readers that resemble the errors defining acquired dyslexias (Kello and Plaut, 2000).
Different task demands elicit the symptoms of different types of aphasia from the same brain-damaged individual(s) (Kolk et al., 1985; Kolk and Heeschen, 1992; Hofstede and Kolk, 1994; Kolk and Hofstede, 1994). This would seem to require brain-to-body control, if only to guarantee performance will satisfy the task requirements described in instructions to a brain-damaged individual. Brain-to-body control could occur if the weaker and faster changes of the brain could be susceptible locally, as when a weak external perturbation can change the next stronger source of constraint. Local susceptibility of this sort could be passed up the hierarchy of constraints, each pace of change in turn, to usurp the stronger and slower dynamics of the body and change the course of behavior.
This is a reasonable way to imagine the white-collar control of behavior by the brain, capitalizing on the relatively unstable dynamics near critical points. But brain-to-body control is not the focus of this essay. Our goal is to shine more light on the blue-collar work of the brain. Blue-collar work exploits the relatively stable dynamics near critical points, which may at first seem to contradict what we just supposed to be the basis for white-collar control – that is, susceptibility stemming from relatively unstable dynamics near critical points.
Yet a critical state has the unique feature of being simultaneously the locus of stability and instability, regular and random variation, universal and singular structures – both together or neither alone – a third kind of behavior (Keshner, 1982; Ulanowicz, 2006; Nicolis and Rouvas-Nicolis, 2007; Sporns, 2007; Tsonis, 2008; Van Orden et al., 2011). Before complexity science the variation in measured values was divided exclusively between the regular changes of explainable variance and the random changes of measurement error, signal versus noise. But pink noise is neither signal nor noise, or it is both, as already noted, and so it cannot be classified within the conventional dichotomy. Pink noise is a third category of behavior, a widely acknowledged game-changing phenomenon of complexity science. It is the simultaneous presence of instability together with stability that defines a critical state.
Thus our thesis: if white-collar control can be said to exploit the instability of a critical state then blue-collar work depends upon stability. Brain-to-body control by the faster changing dynamics of the brain exploits the instability near a critical state to change the course of the slower dynamics of the body. Blue-collar work exploits constraints supplied by the more slowly changing “ghost” parameter dynamics of criticality that lend stability to the faster changing dynamics of the brain.
Additional sources of constraints for brain dynamics include the repetitively similar behavioral trajectories of organ systems, the expressed modes of physiological processes, the repetitive movements of human gait, as well as cognitive problems that persist over time or constraints due to intentions that remain unsatisfied. These few examples illustrate the reservoir of constraints present in the generally more slowly changing dynamics of behavior compared to brain. We next describe the structural composition of the body that self-organizes as movement trajectories of the body in behavior.
Tensegrity Structure of the Body
A mollusk’s body naturally self-organizes survivable relationships with its environment. While slowly treading water, for instance, the mollusk abruptly recruits interneurons within a self-ordering central pattern generator, allowing a rapid escape from a predator (Nishikawa et al., 2007). In doing so, the central pattern generator illustrates the soft-assembly of a synergy.
Synergies are “softly” (temporarily) assembled dynamical processes. Temporary soft-assembly allows changes in control to stay apace with the changing demands for reorganization of behavior. Perpetually changing demands exist at the perpetually changing interface of an organism with its environment. Central pattern generators stay apace by re-organizing their network connectivity (Harris-Warrick and Marder, 1991; Morton and Chiel, 1994; Hooper, 2001). The changing relationship with an environment is sometimes served by previously inhibitory connections that now become excitatory connections, by neurons that are recruited into networks in which they did not participate before, or by the fusion of previously separate networks.
The spontaneous dynamics of the brain’s so-called default network will change depending on what the participant just heard. The volunteer’s investment of attention to a task and other task demands can also change the soft-assembled organization of brain activity among the regions of the default network (Hasson et al., 2009). Almost any change pertaining to ordinary standing around will yield uniquely soft-assembled postural dynamics (Riley et al., 2012). The body and brain thus create of themselves unlimited solutions, apace with the idiosyncratic local contexts in which they find themselves.
The organism at its changing interface with the environment requires this flexible self-control, and context-sensitive soft-assembly appears to be the vital organizing principle of brain architecture (Nikolić, 2010). Organism-wide synergies emerge across a tensegrity structure. The tensegrity structure is formed by a taught web of muscles and fascia to fully connect the parts of the skeleton, appearing to wrap it like a mummy. Similar to tensegrity structures in architecture (e.g., Tomassian, 1997) or robotics and biology (e.g., Tur and Juan, 2009), the skeleton supplies the struts while the muscles, ligaments, and fascia form the tension lines eliminating slack from the tensegrity structure (Levin, 2002). The taught web of tension lines ensures that movement at any one place in the tensegrity structure has consequences throughout the structure, creating a robust mechanical holism that even survives damage that has left the body paralyzed (Carello et al., 2008). The neuromusculoskeletal structure of the body, in the guise of this tensegrity structure, is an excitable medium of self-organizing constraints to sustain the coordinated movements of the body.
Synergies allow the tensegrity structure to behave in some ways but not others, and control works as a process of elimination. Synergies are webs of constraints that limit how the body can change in coordination. Respiratory and cardiovascular processes change together with a change of locomotor gait, for example, ensuring the right amount of oxygen to the cells at the right time (e.g., Gonzales et al., in press). Behavioral processes in a skilled tennis player are constrained to run for the ball and make forehand shots, backhand shots, and to serve and return serves. A swimmer is constrained by synergies to breath out through the nose and breath in through the mouth apace with the strokes of swimming. A web of constraints in each case delimits the possibilities for coordination among the processes of the body, in the actions at hand.
Synergies and tensegrity structure also harvest energy from the temporary contexts of the body (Kugler and Turvey, 1987). Some good configurations of the body with its environment exploit potential energy from inertial forces or from gravity in on-going movement (Bernstein, 1967; Kugler and Turvey, 1987; Dickinson et al., 2000; Turvey, 2007; Wijnants et al., in press). Other good configurations knit the body together, head to foot, in the endlessly novel solutions of postural control (Riccio and Stoffregen, 1988). We are two legged creatures who must balance a large heavy head on a thin neck and, to maintain balance, our center of mass should not exceed its base of support, approximately circumscribed within a perimeter around the feet. Lest we tip over, remote preflexes must anticipate all overt movements (Belen’kii et al., 1967). And yet walking is also falling because the body moves outside of its center of mass in each step, utilizing the potential energy from gravity in the process.
Evidence of Synergy
The taught tension-line coupling across the tensegrity structure allows the body to perform as a single functional unit. To do so, synergies tailor the available degrees of freedom for coordinated changes among the processes of the body. In a classic example, the lips must be in contact to say the/b/in/bob/ (Kelso et al., 1984). Synergy ensures this contact by coupling neuromuscular processes to exclude all non-contact relations between the lips at the time that contact is required. As we already noted, the taught web of tension lines ensures that change at any one place in the tensegrity structure has consequences throughout the structure, allowing synergies to contribute to presence of mind, supplying a way of knowing about the body and brain penultimate to an action itself.
Prior to saying the/b/in/bob/, the exclusion of unlikely configurations retains sufficient degrees of freedom prior to action, to allow the lips to compensate for each other, if something goes wrong (e.g., Scholz and Schöner, 1999; Latash et al., 2002; Riley et al., 2012). Thus, to test for control by synergy, simply perturb on-going speech and look whether compensation occurs in the coupled articulators. In the classic study, a speaker’s attempt to say the/b/in/bob/was perturbed by a sudden, unexpected, downward tug on the speaker’s jaw. Ultrafast compensation began within 5–10 ms – faster than the brain can compute and return a new plan of articulation (Wallot and Van Orden, in press) – and the lower lip, not the jaw, stretched upward to form a new configuration of contact, producing a fully intelligible pronunciation of/bob/with no audible distortion (Kelso et al., 1984; see also Folkins and Zimmermann, 1982; Abbs and Gracco, 1984).
Ultrafast compensation reconfigured the bilabial and laryngeal gestures (at least), producing compensatory lip gestures to respect abstract phonology as well as compensations in the kinematics of the larynx (Saltzman et al., 1998; see also Bauer et al., 1995). In the theoretical language of cognitive psychology, bi-level coupling of kinematic micro-dynamics and linguistic macro-dynamics is a coupling between body and mind. Synergies in speech generally include coupling across different levels of organization (van Lieshout, 2004) and coupling across multiple levels of organization solves the essential problem of speech production – the on-line coordination of about 70 muscles to stay within narrow trajectories of legible meaningful speech (e.g., van Lieshout et al., 2007).
In other evidence of synergetic control, the perturbation is again sufficient to prompt a change in degrees of freedom for reorganization of human performance. Dual-task paradigms can be interpreted according to whether performing one task perturbs the performance of another task (Riley et al., 2012). For instance, pressure to respond quickly in a cognitive task can perturb and decrease the stability of motor-task performance, compared to performing the motor task by itself (Temprado et al., 1999, 2001). Other times, a concurrent cognitive task is sufficient to change the organization of the motor performance (Pellecchia et al., 2005; Shockley and Turvey, 2005, 2006). In the latter case, dynamical models suggest that a higher-order synergy envelops cognitive and motor performance (Fuchs et al., 1996). However, the motor task of walking on a treadmill takes priority over a concurrent cognitive performance, lest the participant fall, yielding a reorganization of cognitive performance. The same dynamics of locomotion are present with or without the cognitive task, while cognitive dynamics are reorganized in the dual-task (Kiefer et al., 2009).
In concurrent observations of brain and behavior, changes that anticipate reorganization are seen in the repeated measurements of both brain and behavior, and the coincident changes strictly resemble those that precede known physical examples of bifurcations called phase transitions (Fuchs et al., 1992; Kelso and Fuchs, 1995). Reorganization across a bifurcation point is preceded by patterns of change called critical slowing, critical instability, and eventually the sudden-jump in a bifurcation. The time delay from the sudden-jump reorganization of the brain to the sudden-jump reorganization of behavior is also about right, given our speculation about brain-to-body control, occurring within the time required for a single jolt of activation running from brain to behavior (Fabre-Thorpe et al., 2001; Thorpe, 2002; Riley et al., 2012; Wallot and Van Orden, in press).
The coupling of processes in synergy is a refinement of the idea of coordinative structures in motor coordination, the previous solution to the notorious degrees of freedom problem of behavior (Turvey, 2007): there exist incalculably more possible configurations of the possible states of the body than there are smoothly and appropriately coordinated ways to make behavior (Bernstein, 1967). Tensegrity structure and synergies reduce the degrees of freedom of the body, limiting the possible configurations to task, and context appropriate “symphonies” of movement for coordinated change in behavior (Haken, 1977; Kugler et al., 1980, 1982; Kelso, 1995, 1998, 2009; Juarrero, 1999; Van Orden et al., 2011; Riley et al., 2012).
Another test for the presence of a synergy is to look for reduced degrees of freedom in the processes that are entailed in a behavior (e.g., Riley et al., 2011). For instance, the reduced degrees of freedom observed of one process may anticipate the reduced degrees of freedom of another process not yet enacted. Raising an arm requires anticipation by remote muscles on the opposite side of the body prior to any change in the arm’s position – else the body would tip over. If the arm movement were made to signal a cognitive choice then the preflex of the remote muscles would “signal” the same choice. If so then the fact of the reduced degrees of freedom in the anticipatory preflex corroborates the synergy of the soft-assembled choice response.
One widely used cognitive task includes a judgment of whether a visually presented letter string correctly spells a word in a reference language – that is, standing before a screen on which letter-strings will appear, raise one arm for each American English “word” and the other arm for “non-words.” Event times as “response times” by anticipatory preflexes can be measured in the onset of change in electromyographic activity in the right or left thigh, the right or left paraspinal muscles of the lower back, or the right or left shoulder muscles. If the preflexes reliably distinguish words from non-words instead of leaving the available degrees of freedom open, to accommodate either arm response, then the predicted, anticipatory, synergetic reduction in the degrees of freedom would be confirmed.
Moreno et al. (2011) conducted this experiment, and the side of the body of the preflex reliably distinguished the word from the non-word letter-strings. The observed reduced degrees of freedom in the corresponding preflexes corroborated synergetic control. Otherwise, they observed typical average “word” decision times of about 649 ms in the arm movement data and an identical advantage for “word” over “non-word” response times in each of the anticipatory preflexes. On average, the preflex “word” response times preceded the arm “word” response time by 120 ms at the shoulder, 189 ms at the trunk, and fully 225 ms at the thigh. Synergies appear to have soft-assembled a multilevel whole-body “American English word versus non-word judgment device” (cf. Fowler and Turvey, 1980; Turvey, 1990, 2007; Hollis et al., 2009; Kello and Van Orden, 2009; Kloos and Van Orden, 2009).
Synergies self-organize apace with the flow of context and behavior. This is sufficient to update on-going constraints that anticipate the requirements for oncoming behavior. Invariant or smoothly changing aspects of the world yield invariant or smoothly changing constraints at a pace that is slower than brain dynamics. These constraints inform behavior by limiting the degrees of freedom about what can happen next, leaving open the possible kinematic changes that the body may enact in behavior. A muscle contraction here or a postural adjustment there are nonetheless always constrained by, and constraining of, the total configuration of the behaving body – the organism as an integrated whole.
Summary Conclusions
We began this essay with several examples of control that did not require an intact central nervous system. Mike the celebrity chicken may now be seen to illustrate the importance of tensegrity as an organizing principle of behavior. Taught tension lines across skeletal struts imbue the body with the self-organizing properties of excitable media. Chickens who lose their circadian coordination among physiology and behavior illustrate a coupling to the environment that contributes to control and regulation of health and wellbeing. The sea squirt is perhaps the ultimate illustration of how a nervous system can be necessary (although not sufficient) for some aspects of being, and dispensable for other aspects.
The blue-collar contribution brings together the concepts of timescale, constraint, synergy, and criticality to understand how the brain supports on-going behavior, to anticipate forthcoming behavior. Constraints that reduce the degrees of freedom for behavior unfold on different timescales, and the more slowly changing constraints have priority over faster change constraints. Control in this sense in non-specific, a practically unlimited set of possible actions is reduced to a smaller subset, shaped by the contemporary states of physiological processes, environmental regularities, and the idiosyncratic history of the organism. The smaller subset is sustained in a state of criticality, lacking only a contingent discriminating circumstance to enact one of the possible actions (Järvilehto, 1998; Hollis et al., 2009; Van Orden et al., 2011; Riley et al., 2012; Wallot and Van Orden, in press).
Criticality is thus essential; it is no accident that the body and the brain stay near to critical states. Systems that stay near critical states are called metastable systems and the advantages of metastability are legion. A metastable system can commit to a region of the state space of possibilities for action, without otherwise narrowing its options. This allows a healthy codetermination of action by the actor’s history and context together with the momentary contingencies that choose the behavior that is enacted. This codetermination is also another pairing of regularity and randomness or order and disorder, like those that characterize pink noise and other aspects of complex systems.
Cognitive science is well underway as complexity science, with wide implications for how to conceptualize and investigate human nature. Already, changes in the organization of behavioral activity, as evidenced by the measured dynamics, are revealing of the nature of an organ or organism (e.g., Lipsitz and Goldberger, 1992; Vaillancourt and Newell, 2002; Van Orden et al., 2011; Dixon et al., 2012; Riley et al., 2012). Regarding investigation, however, all aspects of widely applied measurement protocols must be reconsidered, given the capacity of the participant to mirror our protocols in soft-assembly. In other words, for distinct component functions of memory, language, or motor control, substitute constraints that can create or pick out the behaviors that we give these names to. Practically, this way of thinking promotes research that systematically varies a hierarchy of time scaled contexts. A systematic understanding of control, and how it changes in different contexts, will be had by observing changes in the organization of behaviors estimated by scaling relations or order parameters.
Conflict of Interest Statement
The authors declare that the research was conducted in the absence of any commercial or financial relationships that could be construed as a potential conflict of interest.
Acknowledgments
Supported by NSF grants BCS #0843133 and DHB #0728743 to Guy Van Orden. Sebastian Wallot acknowledges funding from the Marie Curie TESIS network. We thank the MINDLab at Aarhus University and the UNIK initiative of the Danish Ministry for Research and Innovation.
Guy Van Orden’s journey into complexity science started with the question, “How would I ever know that I am wrong?” This statement was aimed at what is today called classical cognitive science. Guy reasoned that the answer was not found in particular outcomes of studies, but in how mathematics was used to describe observations. In particular, the identification of components of the mind hinges on independent sources of variability, which can be identified using linear statistics. However, the pervasiveness of interaction effects in behavioral data suggested to him that independent sources of variability are an exception case of human behavior.
In his later work, complexity science provided Guy with an alternative framework, and in particular the concepts of pink noise and criticality were of twofold importance. Pink noise showed what violations of independent contributions of variability look like and criticality offered an alternative set of concepts and statistics to build a science of phenomena that deviate from the classical assumptions. This article highlights some of his last thoughts on the role of critical fluctuations in brain and behavior, and sketches out new routes for a complexity science of cognition.
Guy passed away on May 11th 2012. Guy was unique and wonderful in his roles as scientist, mentor, and colleague. He will be sorely missed.
References
Abbs, J. H., and Gracco, V. L. (1984). Control of complex motor gestures: orofacial muscle responses to load perturbations of lip during speech. J. Neurophysiol. 51, 705–723.
Bauer, A., Jancke, L., and Kalveram, K. T. (1995). Mechanical perturbation of jaw movements during speech: effects on articulation and phonation. Percept. Mot. Skills 80, 1108–1112.
Belen’kii, V., Gurfinkel, V., and Pal’tsev, Y. (1967). Elements of control of voluntary movements. Biophysics (Oxf.) 12, 154–156.
Birkeland, C., Cheng, L., and Lewis, R. A. (1981). Mobility of dideminid ascidian colonies. Bull. Mar. Sci. 31, 170–173.
Carello, C., Silva, P. L., Kinsella-Shaw, J. M., and Turvey, M. T. (2008). Muscle-based perceptions: theory, research and implications for rehabilitation. Rev. Bras. Fisioter 12, 339–350.
Clarke, D. D., and Sokoloff, L. (1999). “Circulation and energy metabolism of the brain,” in Basic Neurochemistry, Molecular, Cellular and Medical Aspects, 6th Edn, eds B. W. Agranoff and G. J. Siegel (Philadelphia: Lippincott-Raven), 637–670.
Davia, C. J. (2005). “Life, catalysis and excitable media: a dynamic systems approach to metabolism and cognition,” in The Physical Basis for Consciousness, ed. J. Tuszynski (Heidelberg: Springer), 229–260.
Delignieres, D., Fortes, M., and Ninot, G. (2004). The fractal dynamics of self-esteem and physical self. Nonlinear Dynamics Psychol. Life Sci. 8, 479–510.
Dickinson, M. H., Farley, C. T., Full, R. J., Koehl, M. A. R., Kram, R., and Lehman, S. (2000). How animals move: an integrative view. Science 288, 100–106.
Dixon, J. A., Holden, J. G., Mirman, D., and Stephen, D. G. (2012). Multifractal dynamics in the emergence of cognitive structure. Top. Cogn. Sci. 4, 51–62.
Dutilh, G., Wagenmakers, E. J., Visser, I., and van der Maas, H. L. J. (2011). A phase transition model for the speed-accuracy trade-off in response time experiments. Cogn. Sci. 35, 211–250.
Engel, S. A., Glover, G. H., and Wandell, B. A. (1997). Retinotopic organization in human visual cortex and the spatial precision of functional MRI. Cereb. Cortex 7, 181–192.
Engel, S. A., Rumelhart, D. E., Wandell, B. A., Lee, A. T., Glover, G. H., Chichilnisky, E. J., and Shadlen, M. N. (1994). fMRI of human visual cortex. Nature 369, 525.
Engel, S. A., Rumelhart, D. E., Wandell, B. A., Lee, A. T., Glover, G. H., Chichilnisky, E. J., Shadlen, M. N., and Newsome, W. T. (1993). Functional MRI measurements of human striate cortex topography. Soc. Neurosci. Abst. 19, 335.
Fabre-Thorpe, M., Delorme, A., Marlot, C., and Thorpe, S. (2001). A limit to the speed of processing in ultra-rapid visual categorization of novel natural scenes. J. Cogn. Neurosci. 13, 1–10.
Folkins, J. W., and Zimmermann, G. N. (1982). Lip and jaw interaction during speech: responses to perturbation of lower-lip movement prior to bilabial closure. J. Acoust. Soc. Am. 71, 1225–1233.
Fowler, C. A., and Turvey, M. T. (1980). Immediate compensation for bite-block vowels. Phonetica 37, 306–326.
Fuchs, A., Jirsa, V. K., Haken, H., and Kelso, J. A. S. (1996). Extending the HKB-model of coordinated movement to oscillators with different eigenfrequencies. Biol. Cybern. 74, 21–30.
Fuchs, A., Kelso, J. A. S., and Haken, H. (1992). Phase transitions in the human brain: spatial mode dynamics. Int. J. Bifurcat. Chaos 2, 917–939.
Gonzales, L. M., Hessler, E. E., and Amazeen, P. G. (in press). Perceptual constraints on frequency ratio performance in motor-respiratory coordination. Ecol. Psychol.
Haken, H. (1977). Synergetics: An Introduction. Nonequilibrium Phase Transitions and Self-Organization in Physics, Chemistry, and Biology. Berlin: Springer-Verlag.
Harris-Warrick, R. M., and Marder, E. (1991). Modulation of neural networks for behavior. Annu. Rev. Neurosci. 14, 39–57.
Hasson, U., Nusbaum, H. C., and Small, S. L. (2009). Task-dependent organization of brain regions active during rest. Proc. Natl. Acad. Sci. U.S.A. 106, 10841–10846.
Hausdorff, J. M., Zemany, L., Peng, C.-K., and Goldberger, A. L. (1999). Maturation of gait dynamics: stride-to-stride variability and its temporal organization in children. J. Appl. Physiol. 86, 1040–1047.
Hendriks, A. W., and Kolk, H. H. J. (1997). Strategic control in developmental dyslexia. Cogn. Neuropsychol. 14, 321–366.
Hofstede, B. T. M., and Kolk, H. J. (1994). The effects of task variation on the production of grammatical morphology in Broca’s aphasia: a multiple case study. Brain Lang. 46, 278–328.
Hollis, G., Kloos, H., and Van Orden, G. (2009). “Origins of order in cognitive activity,” in Chaos and Complexity in Psychology, eds S. J. Guastello, M. Koopmans and D. Pincus (Cambridge: Cambridge University Press), 206–241.
Hooper, S. L. (2001). “Central pattern generators,” in Encyclopedia of Life Sciences (Chichester: John Wiley & Sons), Available at: http://www.ELS.net [doi 10.1038/npg.els.0000032]
Järvilehto, T. (1998). The theory of the organism-environment system: I. Description of the theory. Integr. Psychol. Behav. Sci. 33, 321–334.
Juarrero, A. (1999). Dynamics in Action: Intentional Behavior as a Complex System. Cambridge, MA: MIT Press.
Kello, C. T., and Plaut, D. C. (2000). Strategic control in word reading: evidence from speeded responding in the tempo naming task. J. Exp. Psychol. Learn Mem. Cogn. 26, 719–750.
Kello, C. T., and Van Orden, G. (2009). Soft-assembly of sensorimotor function. Nonlinear Dynamics Psychol. Life Sci. 13, 57–78.
Kelso, J. A. S. (1995). Dynamic Patterns: The Self-Organization of Brain and Behavior. Cambridge, MA: MIT Press.
Kelso, J. A. S. (1998). “From Bernstein’s physiology of activity to coordination dynamics,” in Progress in Motor Control, Vol. 1, Bernstein’s Traditions in Movement Studies, ed. M. L. Latash (Champaign, IL: Human Kinetics), 203–219.
Kelso, J. A. S. (2009). “Synergies: atoms of brain and behavior,” in Progress in Motor Control: A Multidisciplinary Perspective, ed. D. Sternad (New York: Springer), 83–91.
Kelso, J. A. S., and Fuchs, A. (1995). Self-organizing dynamics of the human brain: critical instabilities and Sil’nikov chaos. Chaos 5, 64–69.
Kelso, J. A. S., Tuller, B., Vatikiotis-Bateson, E., and Fowler, C. A. (1984). Functionally specific articulatory cooperation following jaw perturbations during speech: evidence for coordinative structures. J. Exp. Psychol. Hum. Percept. Perform. 10, 812–832.
Kiefer, A. W., Riley, M. A., Shockley, K., Villard, S., and Van Orden, G. (2009). Walking changes the dynamics of cognitive estimates of time intervals. J. Exp. Psychol. Hum. Percept. Perform. 35, 1532–1541.
Kloos, H., and Van Orden, G. (2009). “Soft-assembled mechanisms for the unified theory,” in Toward a Unified Theory of Development: Connectionism and Dynamic Systems Theory Re-Considered, eds J. P. Spencer, M. Thomas and J. McClelland (New York: Oxford University Press), 253–267.
Kolk, H. H. J., and Heeschen, C. (1992). Agrammatism, paragrammatism and the management of language. Lang. Cogn. Process. 7, 89–129.
Kolk, H. H. J., and Hofstede, B. T. M. (1994). The choice for ellipsis: a case study of stylistic shifts in an agrammatic speaker. Brain Lang. 47, 507–509.
Kolk, H. H. J., Van Grunsven, M. H. F., and Keyser, A. (1985). “On parallelism between production and comprehension in agrammatism,” in Agrammatism, ed. M. Kean (New York: Academic Press), 165–206.
Kugler, P. N., Kelso, J. A. S., and Turvey, M. T. (1980). “On the concept of coordinative structures as dissipative structures: I. Theoretical lines of convergence,” in Tutorials in Motor Behavior, eds G. E. Stelmach and J. Requin (New York: North-Holland), 3–47.
Kugler, P. N., Kelso, J. A. S., and Turvey, M. T. (1982). “On coordination and control in naturally developing systems,” in The Development of Movement Control and Coordination, eds J. A. S. Kelso and J. E. Clark (New York: Wiley), 5–78.
Kugler, P. N., and Turvey, M. T. (1987). Information, Natural Law, and the Self-Assembly of Rhythmic Movement. Hillsdale, NJ: Lawrence Erlbaum Associates.
Latash, M. L., Scholz, J., and Schöner, G. (2002). Motor control strategies revealed in the structure of motor variability. Exerc. Sport Sci. Rev. 30, 26–31.
Levin, S. N. (2002). The tensegrity-truss as a model for spine mechanics: biotensegrity. J. Mech. Med. Biol. 2, 375–388.
Maguire, E. A., Gadian, N. G., Johnsrude, I. S., Good, C. D., Ashburner, J., Frackowiak, R. S., and Frith, C. D. (2000). Navigation-related structural changes in the hippocampi of taxi drivers. PNAS 97, 4398–4403.
Marshall, J. C., and Newcombe, F. (1973). Patterns of paralexia: a psycholinguistic approach. J. Psycholinguist. Res. 2, 175–199.
Marshall, J. C., and Newcombe, F. (1977). “Variability and constraint in acquired dyslexia,” in Studies in Neurolinguistics, Vol. 3, eds H. Whitaker and H. A. Whitaker (New York: Academic Press), 257–283.
Moreno, M. A., Stepp, N., and Turvey, M. T. (2011). Whole body lexical decision. Neurosci. Lett. 490, 126–129.
Morton, D. W., and Chiel, H. J. (1994). Neural architectures for adaptive behavior. Trends Neurosci. 17, 413–420.
Newell, K. M., and Slifkin, A. B. (1998). “The nature of movement variability,” in Motor Behavior and Human Skill: A Multidisciplinary Approach, ed. J. P. Piek (Champaign, IL: Human Kinetics), 143–160.
Nicolis, G., and Rouvas-Nicolis, C. (2007). “Complex systems,” in Scholarpedia, 2, :1473. Available at: http://www.scholarpedia.org/article/ [accessed April 24, 2009].
Nishikawa, K., Biewener, A. A., Aerts, P., Ahn, A. N., Chiel, H. J., Daley, M. A., Daniel, T. L., Full, R. J., Hale, M. E., Hedrick, T. L., Lappin, A. K., Nichols, T. R., Quinn, R. D., Satterlie, R. A., and Szymik, B. (2007). Neuromechanics: an integrative approach for understanding motor control. Integr. Comp. Biol. 47, 16–54.
Pellecchia, G., Shockley, K., and Turvey, M. T. (2005). Concurrent cognitive task modulates coordination dynamics. Cogn. Sci. 29, 531–557.
Raichle, M. E., and Gusnard, D. A. (2005). Intrinsic brain activity sets the stage for expression of motivated behavior. J. Comp. Neurol. 493, 167–176.
Riccio, G. E., and Stoffregen, T. A. (1988). Affordances as constraints on the control of stance. Hum. Mov. Sci. 7, 265–300.
Riley, M. A., Richardson, M. J., Shockley, K., and Ramenzoni, V. C. (2011). Interpersonal synergies. Front. Move. Sci. Sport Psychol. 2:38. doi:10.3389/fpsyg.2011.00038
Riley, M. A., Shockley, K., and Van Orden, G. (2012). Learning from the body about the mind. Top. Cogn. Sci. 4, 21–34.
Riley, M. A., and Turvey, M. T. (2002). Variability and determinism in motor behavior. J. Mot. Behav. 34, 99–125.
Saltzman, E., Löfqvist, A., Kay, B., Kinsella-Shaw, J., and Rubin, P. (1998). Dynamics of intergestural timing: a perturbation study of lip-larynx coordination. Exp. Brain Res. 123, 412–424.
Schiepek, G., and Haken, H. (2006). Synergetik in der Psychologie. (Synergetics in Psychology). Göttingen: Hogrefe.
Schmit, J. M., Regis, D., and Riley, M. A. (2005). Dynamic patterns of postural sway in ballet dancers and track athletes. Exp. Brain Res. 163, 370–378.
Schmit, J. M., Riley, M. A., Dalvi, A., Sahay, A., Shear, P. K., Shockley, K. D., and Pun, R. Y. K. (2006). Deterministic center of pressure patterns characterize postural instability in Parkinsons’s disease. Exp. Brain Res. 168, 357–367.
Scholz, J. P., and Schöner, G. (1999). The uncontrolled manifold concept: identifying control variables for a functional task. Exp. Brain Res. 126, 289–306.
Shockley, K., and Turvey, M. T. (2005). Encoding and retrieval during bimanual rhythmic coordination. J. Exp. Psychol. Learn Mem. Cogn. 31, 980–990.
Shockley, K., and Turvey, M. T. (2006). Dual-task influences on strategic retrieval and coordination dynamics. Psychon. Bull. Rev. 13, 985–990.
Simon, H. A. (1973). “The organization of complex systems,” in Hierarchy Theory: The Challenge of Complex Systems, ed. H. H. Pattee (New York: Braziller), 1–27.
Sporns, O. (2007) “Complexity,” in Scholarpedia, 2, :1623. Available at: http://www.scholarpedia.org/article/Complexity [accessed April 24, 2009].
Temprado, J. J., Zanone, P. G., Monno, A., and Laurent, M. (1999). Attentional load associated with performing and stabilizing preferred bimanual patterns. J. Exp. Psychol. Hum. Percept. Perform. 25, 1579–1594.
Temprado, J. J., Zanone, P. G., Monno, A., and Laurent, M. (2001). A dynamical framework to understand performance trade-offs and interference in dual tasks. J. Exp. Psychol. Hum. Percept. Perform. 27, 1303–1313.
Thelen, E., Schöner, G., Scheier, C., and Smith, L. B. (2001). The dynamics of embodiment: a field theory of infant perseverative reaching. Behav. Brain Sci., 24, 1–34.
Thorpe, S. J. (2002). Ultra-rapid scene categorization with a wave of spikes. Lect. Notes Comput. Sci. 2525, 1–15.
Tomassian, R. (1997). The Stiff, the Sagging, the Supple: The Possibility of Flexible Integrity in Architecture. Masters thesis, University of Cincinnati, Cincinnati.
Tsonis, A. A. (2008). Randomicity: Rules and Randomness in the Realm of the Infinite. London: Imperial College Press.
Tur, J. M. M., and Juan, S. H. (2009). Tensegrity frameworks: dynamic analysis review and open problems. Mech. Mach. Theory 44, 1–18.
Ulanowicz, R. E. (2006). A Third Window: Natural Life beyond Newton and Darwin. West Conshohocken, PA: Templeton Press.
Vaillancourt, D. E., and Newell, K. M. (2002). Changing complexity in human behavior and physiology through aging and disease. Neurobiol. Aging 23, 1–11.
van Lieshout, P. H. H. M. (2004). “Dynamical systems theory and its application in speech,” in Speech Motor Control in Normal and Disordered Speech, eds B. Maassen, R. Kent, H. Peters, P. van Lieshout and W. Hulstijn (Oxford: Oxford University Press), 51–82.
van Lieshout, P. H. H. M., Bose, A., Square, P. A., and Steele, C. M. (2007). Speech motor control in fluent and dysfluent speech production of an individual with apraxia of speech and Broca’s aphasia. Clin. Linguist. Phon. 21, 159–188.
Van Orden, G., Kloos, H., and Wallot, S. (2011). “Living in the pink: intentionality, wellness, and complexity,” in Handbook of the Philosophy of Science, Vol. 10, Philosophy of Complex Systems, ed. C. A. Hooker (Amsterdam: Elsevier), 639–684.
van Rooij, M., and Van Orden, G. (2011). It’s about space, it’s about time, neuroeconomics and the brain sublime. J. Econ. Perspect. 25, 31–56.
West, B. J. (2006). Where Medicine Went Wrong. Rediscovering the Path to Complexity. London: World Scientific.
Wijnants, M. L., Cox, R. F. A., Hasselman, F., Bosman, A. M. T., and Van Orden, G. (in press). A trade-off study revealing nested timescales of constraint. Front. Fractal Physiol.
Keywords: control, synergetics, self-organization, 1/f scaling, mind and body
Citation: Van Orden G, Hollis G and Wallot S (2012) The blue-collar brain. Front. Physio. 3:207. doi: 10.3389/fphys.2012.00207
Received: 28 January 2012; Accepted: 24 May 2012;
Published online: 18 June 2012.
Edited by:
Tjeerd W. Boonstra, University of New South Wales, AustraliaReviewed by:
Didier Delignieres, University Montpellier 1, FranceCees Van Leeuwen, Katholieke Universiteit Leuven, Belgium
Copyright: © 2012 Van Orden, Hollis and Wallot. This is an open-access article distributed under the terms of the Creative Commons Attribution Non Commercial License, which permits non-commercial use, distribution, and reproduction in other forums, provided the original authors and source are credited.
*Correspondence: Guy Van Orden, CAP Center for Cognition, Action and Perception, Department of Psychology, University of Cincinnati, Cincinnati, OH 45221-0376, USA. e-mail:Z3V5LnZhbi5vcmRlbkB1Yy5lZHU=