- 1 Department of Chemical Engineering, University of Michigan, Ann Arbor, MI, USA
- 2 Department of Microbiology and Immunology, University of Michigan Medical School, Ann Arbor, MI, USA
The NF-κB signaling pathway is central to the body’s response to many pathogens. Mathematical models based on cell culture experiments have identified important molecular mechanisms controlling the dynamics of NF-κB signaling, but the dynamics of this pathway have never been studied in the context of an infection in a host. Here, we incorporate these dynamics into a virtual infection setting. We build a multi-scale model of the immune response to the pathogen Mycobacterium tuberculosis (Mtb) to explore the impact of NF-κB dynamics occurring across molecular, cellular, and tissue scales in the lung. NF-κB signaling is triggered via tumor necrosis factor-α (TNF) binding to receptors on macrophages; TNF has been shown to play a key role in infection dynamics in humans and multiple animal systems. Using our multi-scale model, we predict the impact of TNF-induced NF-κB-mediated responses on the outcome of infection at the level of a granuloma, an aggregate of immune cells and bacteria that forms in response to infection and is key to containment of infection and clinical latency. We show how the stability of mRNA transcripts corresponding to NF-κB-mediated responses significantly controls bacterial load in a granuloma, inflammation level in tissue, and granuloma size. Because we incorporate intracellular signaling pathways explicitly, our analysis also elucidates NF-κB-associated signaling molecules and processes that may be new targets for infection control.
Introduction
The transcription factor NF-κB is a central inflammatory mediator that is essential for the induction of a variety of inflammatory genes in response to various pathogens and inflammatory cytokines. One such cytokine is tumor necrosis factor-α (TNF), a key regulator of host responses to infection, in particular immune response to Mycobacterium tuberculosis (Mtb), the causative agent of tuberculosis (TB). TNF affects the immune response to Mtb through several mechanisms, including induction of macrophage activation to efficiently kill bacteria (Gutierrez et al., 2008; Harris et al., 2008; Mosser and Edwards, 2008), induction of chemokine and cytokine expression (Algood et al., 2004), and apoptosis (Beg and Baltimore, 1996; Van Antwerp et al., 1996; Keane et al., 1997, 2002). These activities, regulated by the NF-κB signaling pathway, have made TNF a key factor for restricting bacterial growth in granulomas, aggregates of bacteria and immune cells within the lung that form as a result of the immune response (Algood et al., 2003; Turner et al., 2003; Ulrichs et al., 2004; Lin et al., 2006; Morel et al., 2006; Tsai et al., 2006; Davis and Ramakrishnan, 2008). Hence, the TNF-induced NF-κB signaling pathway is central to the Mtb immune response, and regulation of intracellular NF-κB signaling dynamics may be key to controlling Mtb infection.
Granulomas are the key pathological feature of TB. If granulomas are capable of containing mycobacteria growth and spread, humans develop a clinically latent infection (Flynn and Klein, 2010; Russell et al., 2010; Flynn et al., 2011). However, if granulomas are impaired in function, infection progresses, granulomas enlarge, and bacteria seed new granulomas; this results in progressive pathology and disease, i.e., active TB. In clinical latency, immunologic perturbation at the level of the granuloma can result in reactivation of infection (Lin et al., 2010). Several experimental (Flynn et al., 1995; Bean et al., 1999; Roach et al., 2002; Chakravarty et al., 2008; Clay et al., 2008; Lin et al., 2010) and theoretical (Marino et al., 2007, 2012; Ray et al., 2009; Fallahi-Sichani et al., 2010, 2011, 2012) studies have confirmed the principal role of TNF in containment of bacteria within TB granulomas.
NF-κB in resting cells is bound to IκB proteins that hold it latent in cytoplasm. Binding of TNF to TNF receptor type 1 (TNFR1) results in activation of IκB kinase (IKK) and IKK-mediated phosphorylation of IκB proteins that ultimately leads to ubiquitination and proteasome-mediated degradation of IκB. Free NF-κB then accumulates in the nucleus and mediates the transcription of target genes (Hayden and Ghosh, 2008; Baltimore, 2011). These genes include extracellular signaling molecules such as TNF and chemokines, intracellular proteins such as macrophage-activating molecules (referred to here as ACT) and inhibitor of apoptosis proteins (IAPs), as well as negative regulators of NF-κB such as IκBα and A20 (Pahl, 1999; Hoffmann and Baltimore, 2006; Gutierrez et al., 2008). The inhibitory impact of A20 on NF-κB results from its roles in attenuating TNFR1 activity and inhibiting IKK activation (Wertz et al., 2004). The regulation of NF-κB via multiple critical intracellular feedback mechanisms is important for the control of inflammation and immune activation (Hoffmann et al., 2002; Cheong et al., 2006, 2008; Kearns and Hoffmann, 2009). Further, the structural characteristics of the inflammatory genes induced by NF-κB, particularly stability of their corresponding mRNA transcripts, control the dynamics of NF-κB-mediated responses in cells (Hao and Baltimore, 2009). However, the significance of intracellular molecular mechanisms controlling the dynamics of TNF-induced NF-κB signaling in regulating the long-term immune response to Mtb infection is poorly characterized.
One can hypothesize that molecules such as NF-κB that have been shown to be critical to immunity against Mtb may have significant effects at the cell and tissue scale, namely on the formation and function of granulomas (Barry et al., 2009; Kirschner et al., 2010). However, these effects have not been identified. For example, it is unclear how the dynamics of NF-κB-mediated responses (i.e., expression of chemokines, TNF and IAPs, and activation of macrophages) affect formation and function of a granuloma. A critical requirement for such studies is the integration of biological information across multiple biological scales (molecular, cellular, and tissue; Figure 1). In this study, we describe a multi-scale computational model that includes: (i) molecular interactions describing the dynamics of the TNF-induced NF-κB signaling pathway, (ii) molecular interactions describing the dynamics of TNFR binding and trafficking, and (iii) cellular/tissue-scale dynamics of the immune response to Mtb. These processes altogether lead to formation of a granuloma. We incorporate a recent model of the NF-κB pathway developed by Tay et al. (2010) based on cell culture data but never explored in the context of an infection in a host. We show that dynamics of TNF-induced NF-κB signaling are critical to controlling bacterial load and inflammation levels at the tissue scale. Further, TNF-mediated activation of resting macrophages, in addition to infected macrophages, is required for a protective immune response, but must be optimally regulated by the immune system to prevent excessive inflammation. We also predict the impact of the dynamics (the extent and the timing) of various NF-κB-mediated responses (i.e., expression of chemokines, TNF, IAPs, and activation of macrophages) on both formation and function of a granuloma. Finally, we ask whether pharmacologically manipulating the NF-κB signaling pathway (for example, by affecting mRNA stability) can improve the outcome of a granuloma that is initially unable to control infection.
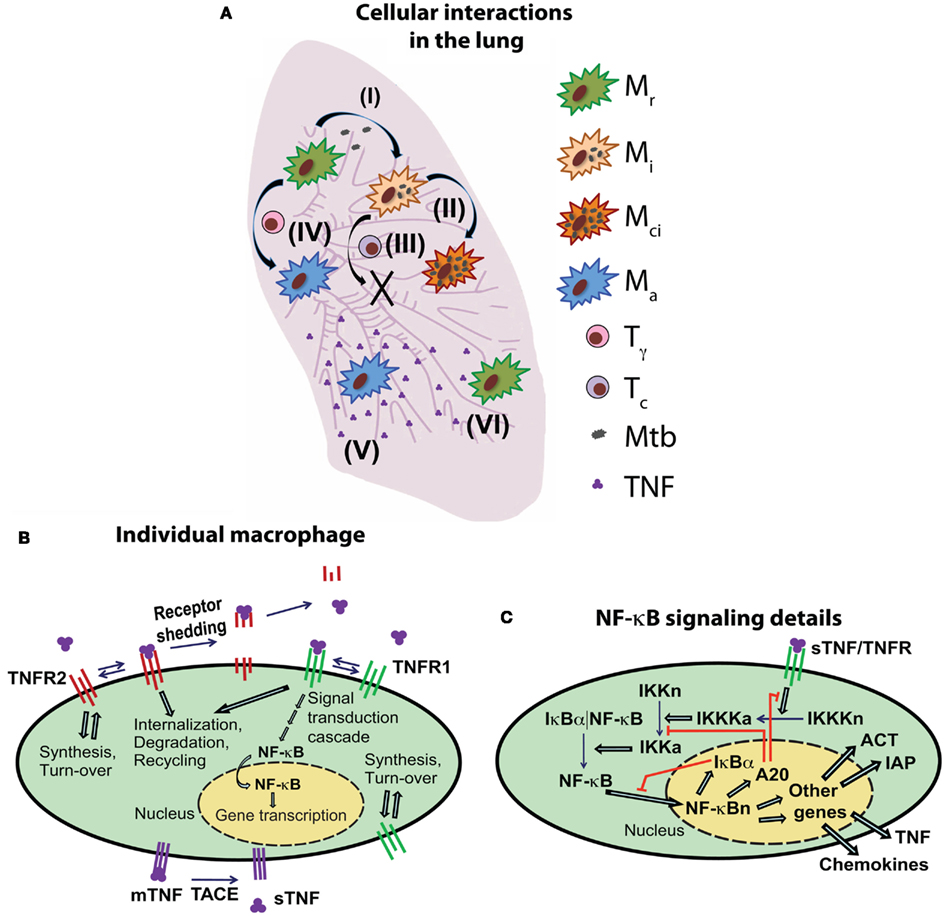
Figure 1. Schematic diagram of the multi-scale model of the immune response to Mtb infection in the lung. (A) An overview of selected cell- and tissue-level ABM rules based on known immunological activities and interactions (Mr, resting macrophage; Mi, infected macrophage; Mci, chronically infected macrophage; Ma, activated macrophage; Tγ, pro-inflammatory IFN-γ producing T cell; Tc, cytotoxic T cell). Example rules are: (I) infection of a resting macrophage after phagocytosis of extracellular Mtb, (II) intracellular growth of Mtb within an infected macrophage, (III) cytotoxic T cell-mediated killing of an infected macrophage, (IV) activation of a macrophage as a result of interaction with IFN-γ producing T cells and TNF, (V) secretion of TNF (and chemokines) from an activated macrophage and diffusion in tissue, (VI) TNF interactions with a macrophage and induction of feedback mechanisms that control TNF-mediated cell responses. For a full description of all ABM rules (see Fallahi-Sichani et al., 2011). (B) An overview of TNF/TNFR binding and trafficking interactions and reactions and the NF-κB signal transduction cascade at the level of individual cell. TNF/TNFR-associated processes are modeled in both macrophages and T cells. (C) Detailed description of the regulation of the TNF-induced NF-κB signaling pathway and NF-κB-mediated responses [expression of chemokines (CHEM), TNF, inhibitors of apoptosis (IAP), and macrophage-activating molecules (ACT)] for an individual macrophage.
Materials and Methods
Multi-Scale Granuloma Model
To address questions regarding TNF-regulated host immune responses to Mtb infection in the lung and the impact of NF-κB signaling dynamics on these responses, we developed a multi-scale computational model (Figure 1) that describes processes over three biological length scales: tissue, cellular, and molecular. Cellular and tissue-scale dynamics are captured via probabilistic rules for interactions between immune cells and Mtb using a stochastic two-dimensional agent-based model (ABM). Single-cell level molecular scale processes include TNF/TNFR binding and trafficking events (defined here to include synthesis, internalization, recycling, and degradation of ligand and receptors) as well as intracellular NF-κB signaling pathway interactions and reactions that are captured by non-linear ordinary differential equations (ODEs). We briefly describe these models below and then describe our approach for linking them.
Our ABM builds on our previous models that capture cellular scale interactions leading to a tissue-level readout, namely granuloma formation in response to Mtb infection in primates (Segovia-Juarez et al., 2004; Ray et al., 2009; Fallahi-Sichani et al., 2011). The ABM has the following components: agents (immune cells, bacteria, chemokines, and cytokines), the environment where agents reside (a two-dimensional grid representing a section of lung tissue), probabilistic rules that govern the dynamics of agents, including movement, actions, and interactions among agents and between agents and environment, and time-scales on which the rules are executed. Briefly, ABM events include: chemotactic movement and recruitment of immune cells from vascular sources to site of infection, intracellular and extracellular growth of Mtb, phagocytosis of bacteria by macrophages, cell death and apoptosis, macrophage/T-cell interactions such as cytolytic functions of cytotoxic T cells (Tc) and IFN-γ-mediated activation of macrophages by pro-inflammatory T cells (Tγ), down-regulation of immune cells by regulatory T cells (Treg), diffusion of chemokines and soluble TNF (sTNF), and caseation (formation of an area of dead tissue with a cheese-like appearance in the center of granuloma). Some of the ABM rules are shown in Figure 1A and a detailed description of these aspects of ABM structure and rules can be found in Fallahi-Sichani et al. (2011). ABM parameters that reflect known biological activities are provided in Table A1 in Appendix. We have now modified our ABM described in Fallahi-Sichani et al. (2011) to facilitate its linking to an NF-κB signaling dynamics model. We now include NF-κB-mediated macrophage activation, NF-κB-mediated chemokine and TNF expression, and NF-κB-mediated inhibition of apoptosis. All of these activities are now controlled as part of the NF-κB signaling dynamics model.
The ODE model describing kinetic processes of TNF/TNFR binding and trafficking occurring in individual cells follows our previous models (Fallahi-Sichani et al., 2010, 2011; Figure 1B; Tables A2 and A3 in Appendix). We modified the reactions associated with TNF expression in this model to capture the linkage between this process and the NF-κB signaling pathway.
In order to capture the molecular mechanisms that control TNF-mediated responses at the single-cell level, we first need to have a model describing intracellular NF-κB signaling pathway activation that follows TNFR activation due to TNF binding. Then, NF-κB activation must be linked to each of the NF-κB-mediated cell responses that include macrophage activation and expression of chemokines, TNF and IAPs. The single-cell level intracellular NF-κB signaling pathway interactions and reactions are captured by using the deterministic approximation of the two-compartment NF-κB dynamics model presented by Tay et al. (2010). This model combines the two-feedback NF-κB-IκBα-A20 regulatory module with the signal transduction cascade transmitting the signal from sTNF-bound TNFR1 receptors. TNFR1 activation results in an oscillatory NF-κB response that controls the dynamics of gene expression (Nelson et al., 2004). The model includes noise due to different levels of TNFRs and total NF-κB molecules across the cell population. This noise results from random assignment of initial values for TNFR densities and total NF-κB molecules to each single cell as described in Tay et al. (2010).
In this study, we link the molecular scale NF-κB dynamics model described above to four major NF-κB-mediated cell responses in macrophages (Figure 1C). These responses are: TNF expression, chemokine expression, macrophage activation, and inhibition of apoptosis. To do this, we incorporate NF-κB-mediated expression of genes corresponding to TNF, chemokines, a generic IAP, and a generic macrophage-activating molecule (ACT), translation of their mRNA transcripts, and secretion of translated TNF and chemokines into the single-cell level NF-κB dynamics model. The generic IAP represents a family of proteins that serve as inhibitors of apoptosis (e.g., cellular inhibitors of apoptosis, c-IAPs) via binding and inhibiting caspase activities (Karin and Lin, 2002). The generic ACT represents various molecules (e.g., membrane trafficking molecules or lysosomal enzyme) that are induced by NF-κB and are required for activation of a macrophage to efficiently kill bacteria (Gutierrez et al., 2008). The reactions, parameters, and equations describing intracellular NF-κB signaling pathway processes and NF-κB-mediated responses for an individual cell are listed in Tables A4–A6 in Appendix. The full range of parameter values explored is given in Table A5 in Appendix; values in parentheses indicate baseline model values, which are intermediate values in the ranges explored and yield the containment outcome.
Linking the Single-Cell Molecular Scale NF-κB Signaling Dynamics to the TNF/TNFR Kinetic Model and the Cellular/Tissue-Scale Model
The activation of TNF-induced NF-κB signaling pathway requires sTNF binding to cell surface TNFR1. It is this process that links the TNF/TNFR kinetic model to the intracellular NF-κB signaling dynamics model. The activation of the NF-κB signaling pathway initiates four major cellular responses: induction of chemokine expression, TNF expression, macrophage activation (to efficiently kill bacteria), and inhibition of apoptosis. These responses serve as the link between the single-cell molecular scale NF-κB signaling dynamics model and the cellular/tissue-scale model (Figure 1). Secretion of chemokines and TNF by macrophages into extracellular spaces follows NF-κB-mediated expression of their genes and translation of their mRNA transcripts as described in the NF-κB signaling equations (see Tables A4 and A6 in Appendix). Recent studies on NF-κB activation and apoptosis have shown that these are processes with discrete nature at the single-cell level, with more cells responding to higher doses of stimuli and longer periods of stimulation (Albeck et al., 2008; Tay et al., 2010). Accordingly, we describe NF-κB-mediated activation of a macrophage as a Poisson process with a probability determined within each time-step (Δt), based on a Poisson rate parameter that is a function of the macrophage activation rate constant (kACT), intracellular concentration of ACT protein [ACT], and the ACT concentration threshold for macrophage activation (τACT):
Similarly, we model TNF-induced apoptosis for each individual cell by:
We use a Poisson process with a probability computed as a function of the apoptosis rate constant (kapopt), the concentration of internalized sTNF/TNFR1 complexes (sTNF/TNFR1i), and the concentration threshold for internalized sTNF/TNFR1 (τapopt). The inhibitory impact of the NF-κB activation on macrophage apoptosis is captured by:
The magnitude of kapopt is a function of the intracellular concentration of IAP, the apoptosis inhibition coefficient (kIAP), and the intrinsic TNF-induced apoptosis rate constant Parameters introduced in Eqs 1–3 are listed in Table A5 in Appendix.
Computer Simulations and Model Outputs
The multi-scale computational model is used to simulate the immune response to Mtb and granuloma formation in the lung for 200 days post-infection. Simulations are initiated following placement of one infected macrophage with one intracellular bacterium at the center of a grid representing a section of lung tissue (see Fallahi-Sichani et al., 2011 for details). Cell-cell interactions governed by ABM rules are updated within every ABM time-step (Δt = 10 min). Molecular scale processes, including TNF/TNFR dynamics and NF-κB signaling dynamics at the single-cell level, are updated within shorter time-steps (dt = 0.5 s).
We use several model outputs to track formation and function of a granuloma during the immune response to Mtb. Granuloma size and total number of macrophages and T cells in tissue are used as readouts to track granuloma formation. We also track total number of bacteria and total number of activated macrophages as readouts for quantifying granuloma function. These outputs represent the ability of a granuloma to control infection and inflammation, respectively. Other outputs of interest include chemokine and TNF concentrations in tissue, and caseation area.
We previously showed that the efficacy of TNF in controlling Mtb infection is strongly affected by whether or not macrophages stimulated by TNF are infected (Fallahi-Sichani et al., 2011). To analyze how NF-κB signaling affects infected versus uninfected (resting) macrophages in a granuloma, we define infected/resting cell ratios, Rapoptosis and Ractivation, as follows. Rapoptosis is defined as the ratio of the number of infected macrophages that undergo TNF-mediated apoptosis to the number of resting macrophages that undergo TNF-mediated apoptosis during a 200-day period post-infection. Ractivation is similarly defined as the number of infected macrophages that become activated (to efficiently kill bacteria) to the number of resting macrophages that become activated during a 200-day period post-infection.
Parameter Estimation
We estimate ABM parameter values from literature data or by using uncertainty analysis as described in detail in Marino et al. (2008); Ray et al. (2009); Fallahi-Sichani et al. (2011). Cell-specific TNFR densities and rate constants for TNF/TNFR processes are estimated based on experimental data from our group (Fallahi-Sichani et al., 2010) and other groups as indicated in Table A3 in Appendix. Intracellular NF-κB signaling parameters are as in Tay et al. (2010; Table A5 in Appendix). Values of parameters used to describe TNF-induced apoptosis and NF-κB-mediated cell responses, including induction of expression of chemokines and TNF, macrophage activation and inhibition of apoptosis, are estimated via uncertainty analysis. This is done by varying parameter values in ranges that are consistent with experimental and modeling data on time-scales of events associated with these responses (Fotin-Mleczek et al., 2002; Rangamani and Sirovich, 2007; Albeck et al., 2008; Hao and Baltimore, 2009; Tay et al., 2010). We specify a baseline set of parameter values (containment baseline values as listed in Tables A1, A3, and A5 in Appendix) that robustly leads to control of infection in granulomas with organized structures as reported for humans and non-human primates.
Model Validation
Immunity to Mtb in humans and animal studies has been attributed to activities of a variety of factors, including specific immune cells (e.g., macrophages and T cells), cytokines (e.g., TNF and IFN-γ), chemokines (e.g., CCL2, CCL5, CXCL9/10/11), immune receptors (e.g., TNFR1), and signaling pathways (e.g., NF-κB). Our new multi-scale computational model [resulting from the incorporation of the single-cell level NF-κB signaling dynamics (Tay et al., 2010), as indicated in Figure 1, into our previous generation model (Fallahi-Sichani et al., 2011)] must retain its ability to reproduce experimental findings regarding the importance of these factors in control of infection. Our model is able to recapitulate different types of granuloma with different abilities to control infection and inflammation (Figure 2). Using a baseline set of values for model parameters (Tables A1, A3, and A5 in Appendix), our model captures a state of equilibrium between the host and Mtb termed bacterial containment (Figure 2A). This state represents control of infection for more than 200 days within a well-circumscribed granuloma containing stable bacteria numbers (<103 total bacteria). Simulated containment granulomas closely represent experimentally characterized solid granulomas (Algood et al., 2003; Turner et al., 2003; Ulrichs et al., 2004; Lin et al., 2006; Morel et al., 2006; Tsai et al., 2006; Davis and Ramakrishnan, 2008) that are predominantly composed of uninfected macrophages surrounding a core of bacteria and infected and activated macrophages with T cells localized at the periphery. Varying values of important model parameters lead to other possibilities, including clearance of bacteria, uncontrolled growth of bacteria, or excessive inflammation.
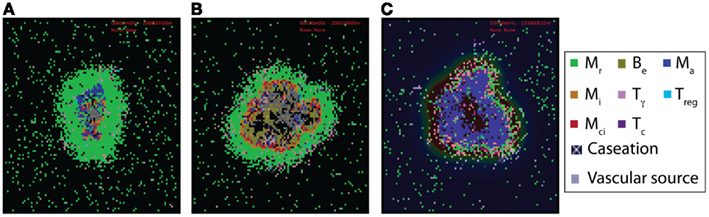
Figure 2. Examples of virtual control experiments for the multi-scale computational model of granuloma formation in response to Mtb infection. (A–C) Granuloma snapshots for (A) a scenario of containment (200 days post-infection), (B) a TNFR1 knockout (TNFR1mac = TNFR1Tcell = 0) scenario resulting in uncontrolled growth of bacteria 200 days post-infection, and (C) a scenario of blocking TNFR1 internalization (kint1 = 0) resulting in excessive inflammation 5 weeks post-infection, respectively. All other model parameter values used for these experiments are listed in Tables A1, A3, and A5 in Appendix. Cell types and status are shown by different color squares, as indicated on the right side of the figure (Mr, resting macrophage; Mi, infected macrophage; Mci, chronically infected macrophage; Ma, activated macrophage; Be, extracellular bacteria; Tγ, pro-inflammatory IFN-γ producing T cell; Tc, cytotoxic T cell; Treg, regulatory T cell). Caseation and vascular sources are also indicated.
We also perform virtual deletion and depletion experiments that mimic experimental gene knockout or molecule depletion studies. Loss of activity is achieved by setting relevant parameters (e.g., probabilities or rate constants) to zero or raising relevant thresholds to an unattainable level. Virtual deletion refers to the loss of activity from the beginning of simulation (such as a gene knockout) and virtual depletion refers to the loss of activity after establishment of a granuloma. Specifically, we simulate gene knockouts of previously identified essential components of the Mtb immune response (e.g., TNF, TNFR1, IFN-γ, and T cell knockouts). These simulation studies are used for testing the ability of the model to predict different infection outcomes under pathological conditions compatible with both experimental and previous modeling data on granuloma formation. Simulations of TNF or TNFR1 knockout (Figure 2B), IFN-γ gene knockout, and deletion of T cells (data not shown), in agreement with experimental data and our previous modeling studies (Flynn, 2004; Segovia-Juarez et al., 2004; Lin et al., 2007; Ray et al., 2009; Lin and Flynn, 2010; Fallahi-Sichani et al., 2011), lead to uncontrolled growth of Mtb and formation of granulomas with irregular structures that include very high numbers of extracellular bacteria, large numbers of infected macrophages, and widespread caseation. In contrast, inhibition of TNFR1 internalization, a process critical to control of TNF concentration and apoptosis (Fallahi-Sichani et al., 2010, 2011), leads to excessive inflammation by which we mean recruitment of a large number of immune cells in tissue, uncontrolled activation of macrophages, and very high concentrations of TNF (Figure 2C).
Sensitivity Analysis
A second approach to identify important processes that determine infection outcome is to use sensitivity analysis. We use sensitivity analysis to analyze the impact of parameters describing events at different scales (molecular, cellular, or tissue scales) on model outputs describing granuloma outcomes. In particular, we use sensitivity analysis techniques adapted for use in ABMs (Marino et al., 2008) to analyze the impact of NF-κB signaling-associated parameter values on model outputs such as bacteria numbers, macrophage and T cell numbers, chemokine and TNF concentrations in tissue, granuloma size, and caseation area. Latin hypercube sampling (LHS) is an algorithm that allows multiple parameters to be varied and sampled simultaneously in a computationally efficient manner (Blower and Dowlatabadi, 1994). The correlation of model outputs with each parameter is quantified via calculation of a partial rank correlation coefficient (PRCC). PRCC values vary between −1 (perfect negative correlation) and +1 (perfect positive correlation) and can be differentiated based on p-values derived from Student’s t test. Here, we performed 700-sample LHS simulations for each parameter. Each sampled parameter set was run four times (to account for stochasticity) and averages of the outputs were used to calculate PRCC values. The choice of the number of simulations is determined by the desired significance level for the PRCC (Blower and Dowlatabadi, 1994; Marino et al., 2008). Here, 700 runs imply that PRCC values above +0.13 or below −0.13 are significantly different from zero (p < 0.001).
Programming and Visualization
The model was implemented in C++. We use Qt, a C++ framework that runs our simulations on multiple platforms (Linux, Windows, and Mac OS) with a graphical user interface (GUI). Through the GUI, one can visualize and track different aspects of the granuloma, including the structure and molecular concentration gradients, as the granuloma forms and is maintained. Simulations can be run with or without graphical visualization. For more detailed description of the Qt framework applications in studying granuloma characteristics see (Marino et al., 2011).
Results
Contribution of NF-κB Signaling Factors to Control of Granuloma Outcomes
We know from both experimental data and our previous modeling studies that TNF availability and activities (i.e., macrophage activation, induction of TNF and chemokine expression, regulation of immune cell recruitment, and induction of apoptosis) within a granuloma are essential to control of infection (Keane et al., 2001; Winthrop, 2006; Marino et al., 2007; Chakravarty et al., 2008; Ray et al., 2009; Lin et al., 2010; Fallahi-Sichani et al., 2011). The NF-κB signaling pathway activated as a result of TNF binding to TNFR1 on the membrane of immune cells is critical for regulation of these activities. Having validated that our multi-scale model gives results consistent with experimental data (see Materials and Methods, Figure 2), we now predict the role of biochemical factors and interactions associated with the NF-κB signaling pathway on important outcomes at the granuloma level: number of bacteria, granuloma size and amount of caseation, and TNF concentration.
We analyze the impact of TNF-mediated NF-κB signaling-associated parameters in six groups as defined in Table A5 in Appendix: (1) concentration of intracellular signaling molecules [NF-κB, IκBα kinase (IKK), and IKK kinase (IKKK)], (2) processes associated with activation of the signal transduction cascade, (3) A20 and IκBα synthesis, (4) IκBα interactions, (5) NF-κB and IκBα transport between cytoplasm and nucleus, and (6) NF-κB-mediated cell responses (TNF and chemokine expression, macrophage activation, inhibition of apoptosis). Notably, parameters identified to have strong correlations with bacterial levels within a granuloma, i.e., granuloma function, belong to groups 1–3 and group 6 (see Table 1 and Tables A7 and A8 in Appendix). Processes within groups 4 and 5, although essential for NF-κB activation, have a less significant impact on model outputs as compared to other groups when they are all varied within a 10-fold range around their baseline values. Within group 1, increasing the average number of NF-κB molecules per macrophage significantly enhances macrophage activation and thus reduces bacterial numbers within a granuloma. This is consistent with the published data on the role of NF-κB in activating macrophages to kill mycobacteria (Gutierrez et al., 2008). Similarly, IKKK activation (from group 2), a key process in NF-κB signaling cascade that occurs following TNF binding to TNFR1, strongly and negatively correlates with bacterial load. Among group 3 parameters, the rate of NF-κB binding at A20 and IκBα gene promoters as well as the rates of A20 and IκBα mRNA synthesis and translation positively correlate with bacterial levels. In contrast, increasing A20 and IκBα mRNA and protein degradation rates impairs granuloma’s ability to control infection. These results highlight the important role that the NF-κB-IκBα-A20 feedback regulatory module plays in the regulation of the NF-κB-mediated cell responses (Cheong et al., 2008), and thus in the regulation of granuloma function.
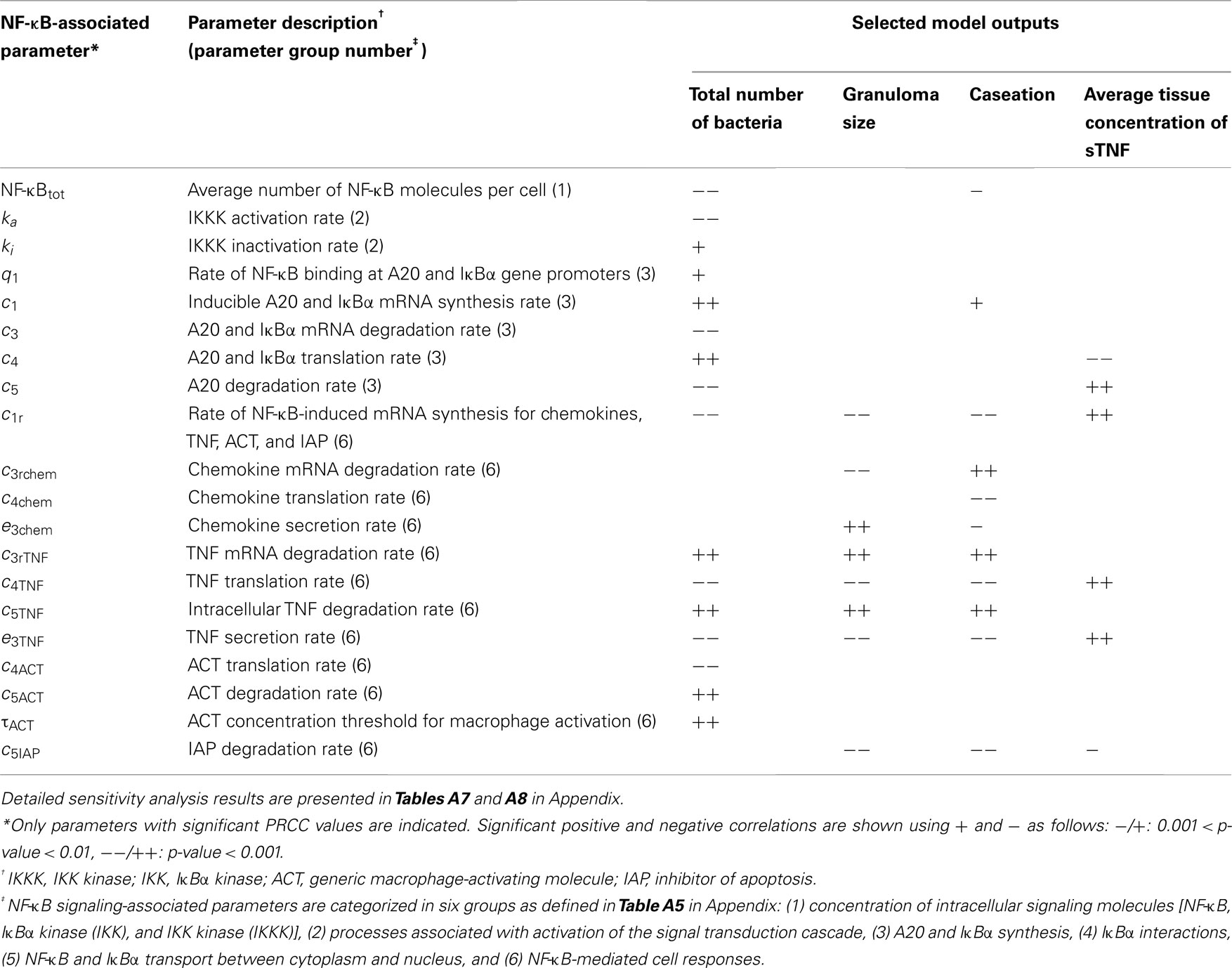
Table 1. NF-κB-associated model parameters significantly correlated with outputs of interest, i.e., bacterial numbers, granuloma size, caseation area, and TNF concentration at day 200 post-infection.
Finally, group 6 comprises important parameters with strong effects on most model outcomes. Parameters that control either TNF expression or macrophage activation significantly influence granuloma function and thus bacterial load within a granuloma. In contrast, parameters that only affect chemokine expression or apoptosis do control granuloma size (formation) but without exerting strong effects on bacterial load (see Table 1 and Table A8 in Appendix). This is consistent with our previous studies indicating that TNF-induced macrophage activation is a key mechanism for controlling bacterial growth (Ray et al., 2009). The rate of NF-κB-dependent mRNA synthesis for chemokines, TNF, the generic macrophage-activating molecule (ACT), and the inhibitor of apoptosis (IAP) is an important parameter. It strongly and positively correlates with all TNF-induced cellular responses in tissue (i.e., apoptosis, TNF and chemokine expression, and macrophage activation) and negatively correlates with bacterial load, caseation, and granuloma size. The stability of TNF mRNA, as well as TNF translation, degradation, and secretion significantly control granuloma outcomes. Increasing the rates of degradation of TNF mRNA and intracellular TNF or reducing the rates of TNF translation and secretion enhance bacterial numbers, caseation, and granuloma size. In addition, the ACT translation rate (negatively), and the ACT degradation rate as well as the ACT concentration threshold for macrophage activation (positively) correlate with bacterial load within a granuloma. Increasing the chemokine secretion rate or reducing the chemokine mRNA degradation rate elevates chemokine concentration in tissue, enhancing immune cell recruitment, and granuloma growth.
Overall, each of the above parameters identified as critical for formation and function of a granuloma represents a potential target for therapeutic modulation. Hence, we focus our next analysis on the potential effects of manipulation of each of these parameters.
Optimal Regulation of NF-κB Signaling Dynamics for Control of Infection without Inducing Excessive Inflammation
The analysis above highlights various NF-κB signaling pathway-associated biochemical factors and intracellular interactions that show significant impacts on infection outcomes at all scales (molecular, cellular, and tissue). How do these responses influence granuloma formation? Does manipulation of these mechanisms alter infection outcome at the granuloma level? The effects of manipulation of four important NF-κB-associated factors as identified by sensitivity analysis - (i) average number of NF-κB molecules per cell, NF-κBtot, (ii) IKKK inactivation rate constant, ki, (iii) A20 and IκBα mRNA degradation rate constant, c3, and (iv) TNF mRNA degradation rate constant, c3rTNF - on granuloma formation, total number of bacteria, sTNF concentration, and macrophage activation after Mtb infection are shown in Figure 3.
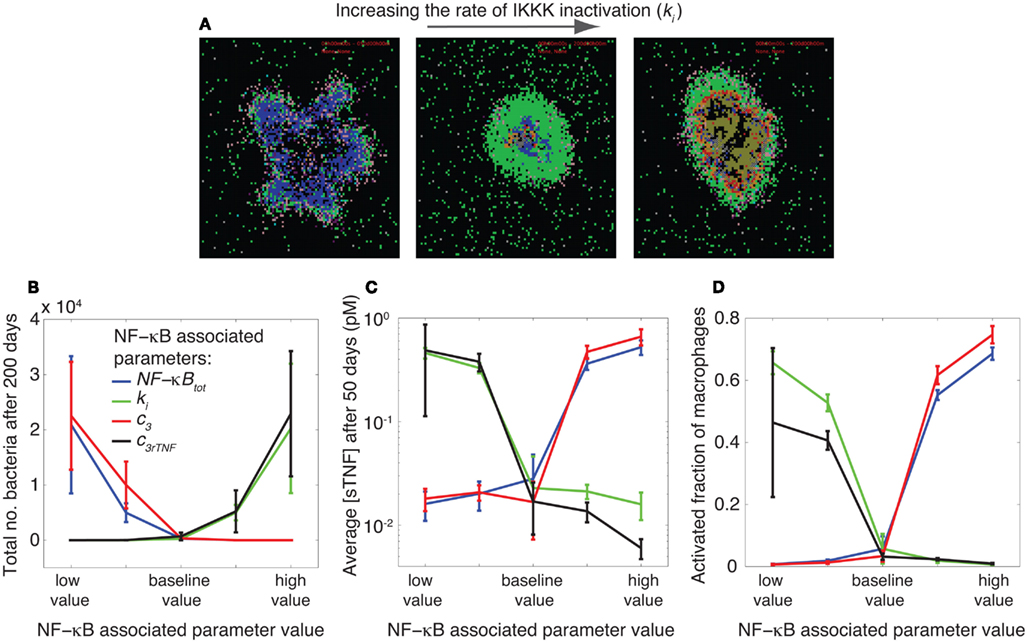
Figure 3. NF-κB signaling dynamics control bacterial growth and inflammation level in tissue. (A) Granuloma snapshots for slow (ki = 3.2 × 10−3 s−1), intermediate (ki = 10−2 s−1), and rapid (ki = 3.2 × 10−2 s−1) rates of IKKK inactivation. Slow rates of IKKK inactivation lead to uncontrolled macrophage activation and excessive inflammation. An intermediate value of ki results in control of infection in a stable granuloma containing small numbers of bacteria. Rapid rates of IKKK inactivation lead to large numbers of bacteria and infected macrophages as well as widespread caseation. The colors representing cells of different type and status in granuloma snapshots are the same as those shown and defined in Figure 2. (B–D) Simulation results showing the effects of four important parameters, as identified by sensitivity analysis, controlling NF-κB signaling dynamics on granuloma outcomes (total number of bacteria, tissue concentration of TNF, and macrophage activation). The parameters are: the average number of NF-κB molecules per cell (NF-κBtot), IKKK inactivation rate (ki), A20 and IκBα mRNA degradation rate (c3), and TNF mRNA degradation rate (c3rTNF). In each simulation, only one of these parameters is varied. The baseline (intermediate) values of these parameters lead to clearance or control of infection in stable granulomas with very low bacterial numbers, low levels of TNF, and low levels of macrophage activation. Perturbing the NF-κB signaling dynamics by varying values of these parameters impair the balance toward either uncontrolled growth of bacteria or excessive inflammation (high TNF concentrations and high levels of macrophage activation) in tissue. The baseline value of each parameter is as reported in Table A5 in Appendix and is as follows: NF-κBtot = 105, ki = 10−2 s−1, c3 = 7.5 × 10−4 s−1, c3rTNF = 3.8 × 10−4 s−1. The difference between the low value and high value presented in the figure is one order of magnitude.
The values of these parameters significantly determine the ability of a granuloma to control bacterial load. Small numbers of NF-κB molecules per cell, slow rates of A20 and IκBα mRNA degradation, rapid rates of IKKK inactivation, and rapid rates of TNF mRNA degradation all lead to uncontrolled growth of bacteria within a 200-day period post-infection (Figure 3B). These effects result from reduced rates of TNF-induced activation of macrophages, diminishing their ability to kill bacteria. Slowly altering the values of these parameters to intermediate levels reduces bacteria numbers and leads to containment of bacteria within a stable granuloma. Further increasing the values of parameters NF-κBtot and c3, or further reducing the values of parameters c3rTNF and ki from their containment-level values each further reduces bacterial numbers and increases the chance of infection clearance. However, these clearance outcomes are generally accompanied by uncontrolled rates of macrophage activation and cell infiltration as well as very high concentrations of TNF in tissue; markers of excessive inflammation and immunopathology (Figures 3C,D). Overall, as depicted in Figures 3A–D, intermediate (containment baseline) values of NF-κBtot, ki, c3 and c3rTNF (listed in Table A5 in Appendix) lead to control of infection in stable granulomas with very low bacteria numbers (and sometimes clearance), low levels of TNF, and low levels of macrophage activation. Perturbing NF-κB signaling dynamics by varying values of these parameters (i.e., rates at which these processes occur) impairs the balance toward either uncontrolled growth of bacteria or excessive inflammation in tissue. Hence, our model predicts that the optimal regulation of the TNF-mediated NF-κB signaling pathway is essential to controlling infection and inflammation in tissue. The balance between the NF-κB-mediated bacterial killing activities and the NF-κB-mediated inflammation results in an equilibrium state, i.e., containment of bacteria within a stable granuloma with minimal inflammation.
How Do NF-κB Signaling Dynamics Balance Inflammation and Bacterial Killing?
How do the NF-κB-associated intracellular processes identified above affect the balance of inflammation and bacterial killing activities within a granuloma? We previously showed that the impact of TNF concentration on granuloma outcomes is strongly correlated with whether or not macrophages stimulated by TNF are infected (Fallahi-Sichani et al., 2011). This motivates us to test whether there is a correlation between the effect of NF-κB signaling dynamics on granuloma function (as described in Figure 3) and the infection status of macrophages stimulated by TNF during the immune response. Thus, we analyze the infection status of macrophages that become activated or undergo apoptosis after Mtb infection by computing infected/resting cell ratios, Ractivation and Rapoptosis, as defined in Section “Materials and Methods.” Our model predicts a very significant effect of important NF-κB-associated parameters on both Ractivation and Rapoptosis (Figure 4). At small numbers of NF-κB molecules per cell, slow rates of A20 and IκBα mRNA degradation, rapid rates of IKKK inactivation, or rapid rates of TNF mRNA degradation, infected macrophages are the main cells that become activated or undergo apoptosis as a result of TNF activities (Ractivation and Rapoptosis ≫ 1). However, with one order of magnitude increase in each of these parameters, resting macrophages become the main responders to TNF signaling (Ractivation and Rapoptosis ≪ 1). Comparing these results with results from the previous section (Figure 3), we observe a significant correlation between infected/resting cell ratios, Ractivation and Rapoptosis, and the granuloma outcomes (i.e., bacterial load and inflammation). At large values of Ractivation and Rapoptosis (values of 1–10 or greater), we observe uncontrolled growth of Mtb. Small values of these ratios (smaller than ∼0.1) correlate with excessive inflammation in tissue. Intermediate values of infected/resting cell ratios (between 0.1 and 1) are correlated with control of infection without excessive inflammation. The absolute values of these ratios are calculated based on our two-dimensional simulations and might change in three-dimensional settings. These results suggest that a balance between the number of resting macrophages and infected macrophages responding to TNF signaling is required for control of infection and inflammation within a stable granuloma, and that such a balance is critically regulated by NF-κB signaling dynamics.
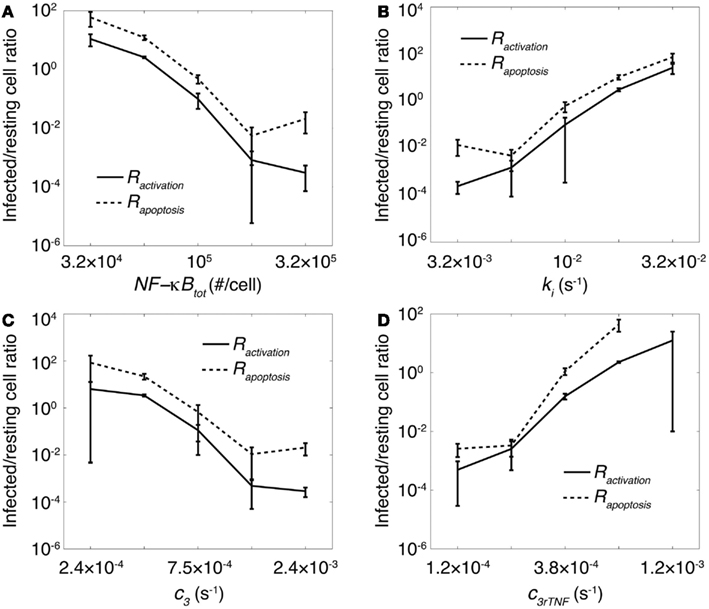
Figure 4. The impact of important processes associated with the NF-κB signaling dynamics on granuloma outcomes is correlated with status of macrophages that undergo apoptosis or become activated by TNF. Simulation results show the effect of (A) the average number of NF-κB molecules per cell, NF-κBtot, (B) IKKK inactivation rate, ki, (C) A20 and IκBα mRNA degradation rate, c3, and (D) TNF mRNA degradation rate, c3rTNF on infected/resting cell ratios Rapoptosis and Ractivation within a 200 day period after Mtb infection.
The Stability of mRNA Transcripts Controls Bacterial Load, Inflammation, and Granuloma Size by Affecting the Dynamics of NF-κB-Mediated Responses
A key advantage of incorporating NF-κB signaling dynamics into our granuloma model is the ability to study the impact of the dynamics of NF-κB-mediated responses (i.e., macrophage activation, expression of chemokines, TNF, and inhibitors of apoptosis) on granuloma outcomes. These responses follow NF-κB oscillations (Nelson et al., 2004). The dynamics of these responses depend, to a large extent, on the stability of their corresponding mRNA transcripts (Hao and Baltimore, 2009). Thus, we analyzed the effect of varying the stability of mRNA transcripts corresponding to macrophage activation (ACT), and expression of chemokines (CHEM), TNF, and inhibitors of apoptosis (IAP) on granuloma outcomes, bacterial load, and inflammation level (represented by the activated fraction of macrophages). Varying the stability (half-life; t1/2) of mRNA transcripts significantly influences the dynamics of the NF-κB-mediated responses (e.g., chemokine secretion) in an individual cell (Figure 5A). Simulations show that the stability of mRNA transcripts for NF-κB-mediated responses, particularly ACT, TNF, and CHEM, significantly control bacteria numbers and inflammation level in tissue (Figures 5B,C). The impact of the IAP mRNA stability on these model outcomes is less significant.
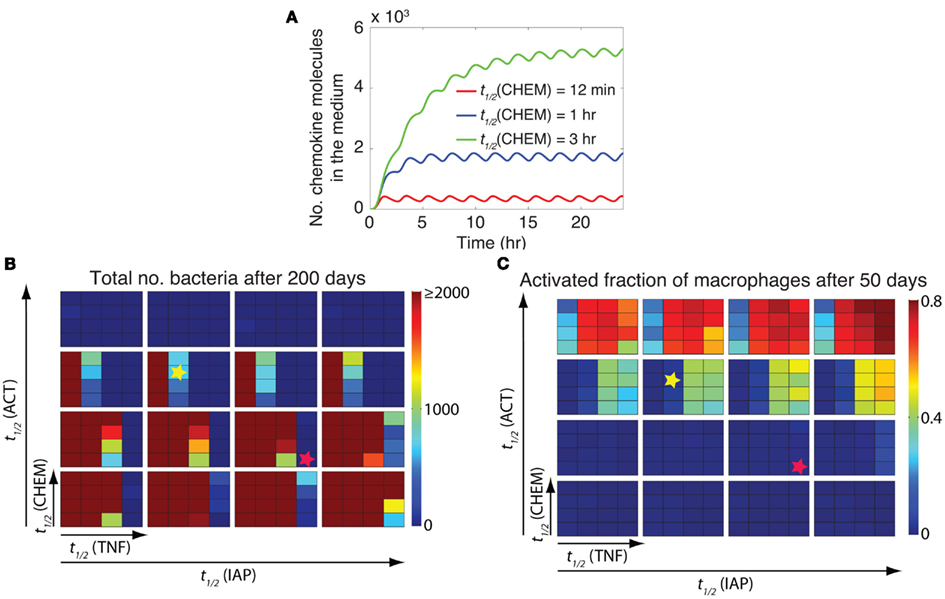
Figure 5. The stability of mRNA transcripts controls bacterial load and inflammation by affecting the dynamics of NF-κB-mediated responses. (A) The effect of the stability (half-life) of chemokine mRNA transcripts [t1/2(CHEM)] on the dynamics of chemokine secretion by an individual cell. Simulated results are produced using the single-cell level NF-κB signaling dynamics model for continuous stimulation of a cell by 1 ng/ml TNF, with parameters and equations as described in Tables A3, A5, and A6 in Appendix. A similar pattern of response can be observed when the effects of mRNA stability on the dynamics of other NF-κB-mediated responses (i.e., expression of ACT, IAP, and TNF) are studied (data not shown). (B,C) Simulation results for the effect of the stability of mRNA transcripts corresponding to major NF-κB-mediated responses, including macrophage activation [t1/2(ACT)], TNF expression [t1/2(TNF)], chemokine expression [t1/2(CHEM)], and inhibitor of apoptosis protein expression [t1/2(IAP)], on bacteria numbers (B) and on the activated fraction of macrophages (C) 200 days post-infection. Small squares represent different values of t1/2(CHEM) vertically and different values of t1/2(TNF) horizontally. Large boxes represent different values of t1/2(ACT) vertically and different values of t1/2(IAP) horizontally. Four values of mRNA half-life were tested in simulations: 12 min, 30 min, 1 h, and 3 h. Simulation results were averaged over 10 repetitions. Yellow stars represent an example scenario with containment outcome. This state represents control of infection for more than 200 days within a well-circumscribed granuloma containing stable bacteria numbers (<103 total bacteria). Red stars represent an example scenario that leads to clearance of Mtb (total bacteria = 0) without inducing excessive inflammation (activated fraction of macrophages <0.15).
Our analysis shows that there are combinations of TNF, ACT, CHEM, and IAP mRNA transcript half-lives that lead to distinct model outcomes such as control of infection within stable granulomas, clearance, uncontrolled growth of bacteria, or excessive inflammation (see Figure 2). For example, a containment outcome (as highlighted by yellow stars in Figures 5B,C) may result from the following parameter combination: mRNA transcript half-life of 30 min for TNF, mRNA transcript half-life of 1 h for ACT, mRNA transcript half-life of 1 h for CHEM, and mRNA transcript half-life of 30 min for IAP. Increasing mRNA transcript stabilities for TNF and ACT from these values increases the chance of extensive inflammation in tissue, whereas reducing their values significantly enhance bacterial load. Increasing mRNA transcript stabilities for CHEM from the suggested value also slightly enhances bacterial load as well as granuloma size (data not shown). Further, our results suggest that there are combinations of mRNA stabilities for TNF-mediated responses that lead to clearance of Mtb without inducing excessive inflammation (see red stars in Figures 5B,C as an example). This set of mRNA stability values significantly enhances the ability of granuloma to kill bacteria while limiting inflammation by controlling macrophage activation and apoptosis. Overall, these results suggest that the differential dynamics of NF-κB-mediated responses resulting from differential stabilities of their corresponding mRNA transcripts are essential to regulate granuloma’s ability to control infection and inflammation.
The Timing of NF-κB-Induced Macrophage Activation is Critical to Controlling Excessive Inflammation
In the previous section, we showed that stability of mRNA transcripts associated with NF-κB-mediated inflammatory molecules significantly affects the immune response to Mtb. The stability of mRNA controls both the extent and the timing of NF-κB-mediated responses in individual cells (Tay et al., 2010). However, it is not clear whether it is mostly the extent of response, the timing of response, or both that influence granuloma outcomes. In other words, how important is the speed of each individual macrophage’s response to TNF signals in determining the overall function of a granuloma? To address this question, we analyzed the effect on granuloma outcomes of varying the stability of ACT, CHEM, TNF, and IAP mRNA transcripts while maintaining the average extent of these responses at their containment baseline levels (determined in the previous section). To maintain the average extent of each response as its corresponding mRNA stability is varied, we simultaneously vary another parameter associated with a process downstream of mRNA translation. Parameters varied to adjust the extent of the four NF-κB-mediated responses are: TNF secretion rate (e3TNF), chemokine secretion rate (e3chem), ACT concentration threshold for macrophage activation (τACT), macrophage activation rate constant (kACT), and apoptosis inhibition constant (kIAP). For example, we increase the chemokine mRNA half-life [t1/2(CHEM)] and decrease the chemokine secretion rate (e3chem) simultaneously to achieve the same average number of chemokine molecules secreted in tissue by an individual macrophage (Figure 6A).
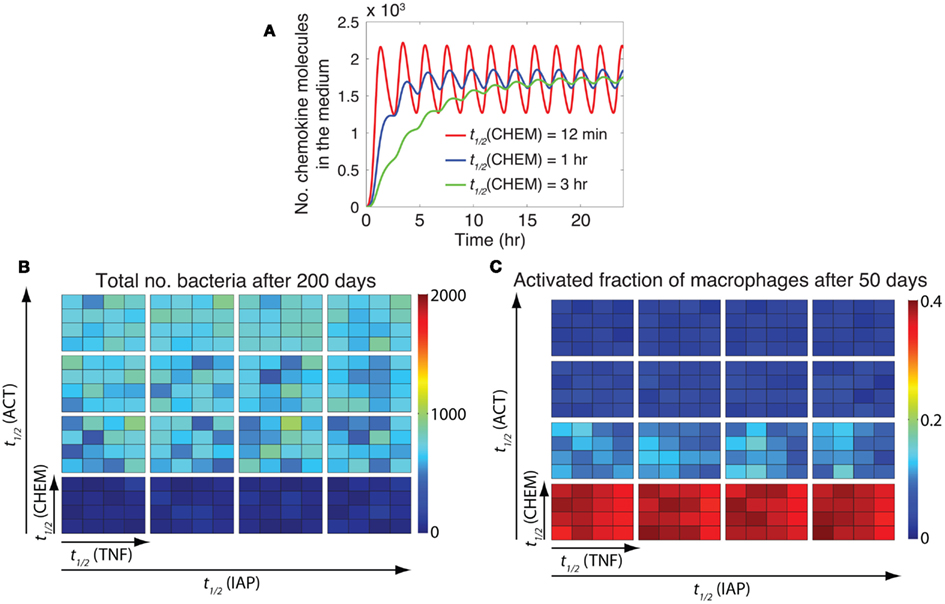
Figure 6. The timing of NF-κB-induced macrophage activation is critical to control of inflammation. (A) Varying the chemokine mRNA half-life [t1/2(CHEM): 12 min, 1 h, and 3 h, respectively] and the chemokine secretion rate (e3chem: 7.65 × 10−5 s−1, 1.39 × 10−5 s−1, 4.52 × 10−6 s−1, respectively) by an individual macrophage simultaneously leads to secretion of the same average number of chemokine molecules, but with distinct temporal patterns of chemokine secretion. Simulated results are produced using the single-cell level NF-κB signaling dynamics model for continuous stimulation of a cell by 1 ng/ml TNF, with parameters and equations as described in Tables A3, A5, and A6 in Appendix. A similar pattern of response can be observed when the effects of mRNA stability on the timing of other NF-κB-mediated responses (i.e., expression of ACT, IAP, and TNF) are studied (data not shown). (B,C) Simulation results for the effect of the timing of NF-κB-mediated responses, including macrophage activation [regulated by t1/2(ACT)], TNF expression [regulated by t1/2(TNF)], chemokine expression [regulated by t1/2(CHEM)], and inhibitor of apoptosis protein expression [regulated by t1/2(IAP)], on bacteria numbers (B), and on the activated fraction of macrophages (C) at 200 days post-infection. Small squares represent different values of t1/2(CHEM) vertically and different values of t1/2(TNF) horizontally. Large boxes represent different values of t1/2(ACT) vertically and different values of t1/2(IAP) horizontally. Four values of mRNA half-life were tested in simulations: 12 min, 30 min, 1 h, and 3 h. Simulation results were averaged over 10 repetitions.
Analysis of granuloma simulations indicates that among the four major NF-κB-mediated responses studied here (TNF, CHEM, ACT, and IAP), only the timing of ACT response, i.e., macrophage activation, is critical to control of inflammation in tissue as well as bacterial load within a granuloma (Figures 6B,C). Early NF-κB-mediated activation of macrophages that occurs because of highly unstable ACT mRNA transcripts lead to uncontrolled activation of macrophages and excessive inflammation in tissue. This suggests that both extent and timing of NF-κB-mediated macrophage activation are critical to control of the immune response to Mtb.
Can Manipulating TNF-Mediated NF-κB Signaling Dynamics Improve Granuloma Function?
Above we showed that optimal regulation of NF-κB signaling dynamics is critical to control of infection within a granuloma and control of inflammation in lung tissue. Thus, impairing NF-κB activation leads to uncontrolled growth of bacteria that is in agreement with NF-κB knockout experimental studies (Yamada et al., 2001). The repression of NF-κB signaling in infected macrophages is also a mechanism that pathogenic mycobacteria use to enhance their survival and growth (Gutierrez et al., 2008). An important question is then: can we find a hypothetical treatment strategy that affects TNF-mediated NF-κB signaling in a granuloma to improve ability to control bacteria? We first simulate formation of a granuloma that is unable to control bacterial growth due to impaired NF-κB signaling (e.g., at high rates of IKKK inactivation, ki) for 100 days. Then, we change one or more of the NF-κB-associated parameters to restore NF-κB activities within the granuloma and resume simulation for another 100 days.
Our analysis, as depicted in Figure 7, indicates that reducing ki (IKKK inactivation rate constant) from high values to intermediate (containment-level) values (Treatment I) enhances the ability of a granuloma to control bacteria. However, average bacteria levels for a 200-day granuloma after changing ki are generally higher than bacteria levels resulting from simulating a containment scenario. A further decrease in the value of ki (Treatment II) is more successful in killing bacteria. However, it leads to uncontrolled activation of macrophages and excessive inflammation in tissue. This suggests that targeting the process of IKKK inactivation alone is not sufficient for infection control at the granuloma scale. In another set of simulations (Treatment III), decreasing ki to intermediate values, together with manipulating stability of mRNA transcripts associated with NF-κB-mediated responses (based on results from Figure 5) leads to better outcomes. Increasing the half-life of TNF mRNA transcripts to 3 h, reducing the half-life of ACT mRNA transcripts to 30 min, and setting the IAP mRNA transcripts to 1 h improves the granuloma outcome, inducing efficient killing of bacteria without excessive inflammation. Overall, this suggests that manipulating the dynamics of NF-κB-mediated responses, particularly macrophage activation, TNF and IAP expression, can improve the function of a TB granuloma.
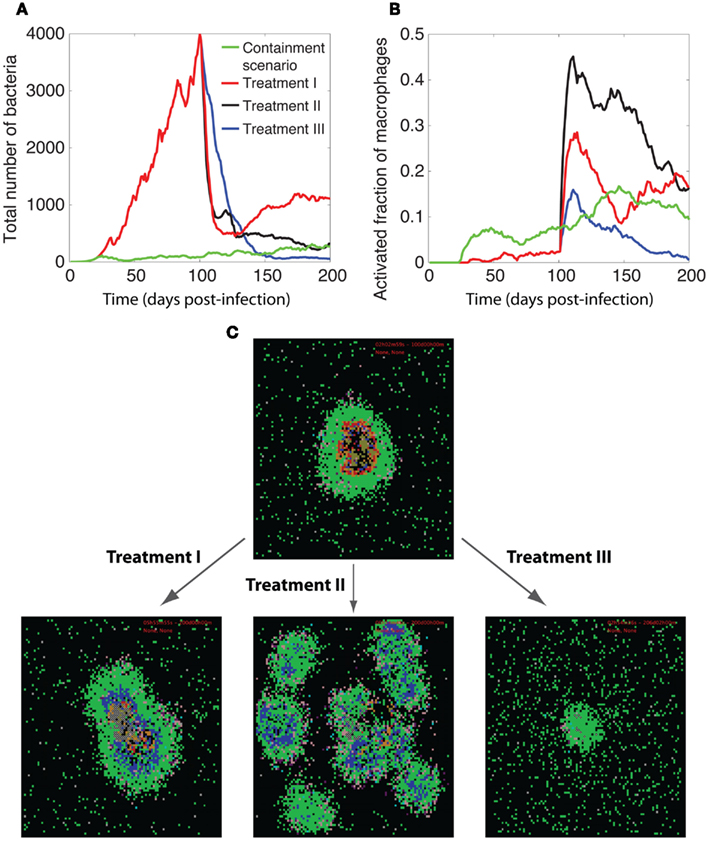
Figure 7. Manipulation of TNF-mediated NF-κB signaling for improving granuloma function. Comparison of the dynamics of (A) bacteria growth, (B) activated fraction of macrophages, and (C) granuloma snapshots among three different treatment methods for enhancing NF-κB activities. In all treatments, we first simulate formation of a granuloma that is unable to control bacteria growth due to impaired NF-κB signaling at high rates of IKKK inactivation (ki = 3.16 × 10−2 s−1) for 100 days (all other parameter values are as listed in Tables A1, A3, and A5 in Appendix). Then, we change one or more of the NF-κB-associated parameters to restore NF-κB activities within the granuloma and resume simulation for another 100 days. Parameter changes in each treatment are as follows: treatment I: ki = 1 × 10−2 s−1, Treatment II: ki = 3.16 × 10−3 s−1, Treatment III: ki = 1 × 10−2 s−1, t1/2(TNF) = 3 h, t1/2(ACT) = 30 min, t1/2(TNF) = 1 h. Simulation results were averaged over 10 repetitions. The colors representing cells of different type and status in granuloma snapshots are the same as those shown and defined in Figure 2.
Discussion
Systems biology approaches have been increasingly helpful for studying the interactions between the components of biological systems, and understanding how these interactions give rise to the function of the system. These approaches are particularly essential for studying systems that consist of several components on different spatial and temporal scales, as they are extremely challenging to study using traditional experimental methods. An important example is to study the role that the dynamics of intracellular signaling pathways, with time-scales of seconds to hours, play in the long-term immune response of a host to a pathogen. In this work, we focus on this problem by asking if simulations of the immune response can successfully capture both short and long-term dynamics over length scales that range from molecular to tissue. We build and simulate a multi-scale model to explore the impact of NF-κB dynamics on the long-term immune response to the pathogen Mtb. NF-κB plays an important role in coordinating both innate and adaptive immunity. A recently published study of the pathway uses data from cells in culture to elucidate the kinetics of the pathway and to identify critical intracellular mechanisms controlling the NF-κB response in a single cell (Tay et al., 2010). A recent modeling study has also shown how NF-κB response can control cytokine waves in tissue (Yde et al., 2011). Yet it is unclear how these mechanisms affect the immune response in tissue, where immune cells and bacteria interact with each other and determine the outcome of infection.
Immune responses induced by Mtb infection are myriad and complex, and it remains incompletely understood which responses are required for protection and which contribute to pathology (Cooper, 2009; Lin and Flynn, 2010). Indeed, there is significant overlap among protective and pathological responses. An important example, as dissected in this study, is TNF-induced NF-κB activation. Figure 8 summarizes our results showing how NF-κB-mediated responses are critical for restricting bacterial growth in a granuloma, but excessive activation of the NF-κB pathway in macrophages leads to pathological inflammation in tissue. Containment of bacteria, particularly at the level of the granuloma, is achieved when a balance exists between the NF-κB-mediated bacterial killing activities and the NF-κB-mediated inflammation. Such a balance is controlled by a combination of molecular scale biochemical processes identified in detail in this study, such as IKKK activity, A20 and IκBα interactions, and stability of mRNA transcripts associated with NF-κB-mediated responses. Optimal regulation of these processes, in the presence of an efficient T cell-meditated response, can lead to clearance of bacteria. Further, we find that processes controlling the dynamics of NF-κB signaling critically regulate whether resting macrophages or infected macrophages are the major targets for TNF signaling within a granuloma. Unless sufficient numbers of resting macrophages relative to infected macrophages become activated by TNF, uncontrolled growth of Mtb occurs. On the other hand, excessive activation of resting macrophages leads to uncontrolled inflammation. These findings highlight the potential importance of NF-κB-associated processes as targets in future studies examining approaches to controlling both TB infection and pathology.
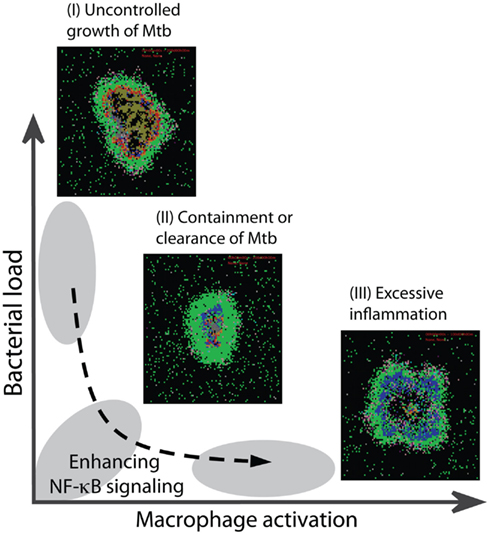
Figure 8. Optimal regulation of the TNF-mediated NF-κB signaling dynamics is essential for optimal granuloma outcomes. Impaired NF-κB activity leads to uncontrolled growth of bacteria within a granuloma (outcome I). Containment or clearance of bacteria (outcome II) is achieved when the NF-κB-mediated responses are regulated such that small, but sufficient numbers of macrophages become activated to kill bacteria. Uncontrolled macrophage activation due to over-activity of NF-κB leads to excessive inflammation in tissue (outcome III).
Another interesting finding from our study is that the stability of mRNA transcripts corresponding to NF-κB-mediated responses, particularly macrophage activation and expression of TNF and chemokines, significantly affects bacterial load in a granuloma, inflammation level in tissue, and granuloma size. This is due to the impact of mRNA stability on the kinetics of these responses (Hao and Baltimore, 2009). Tay et al. (2010) have also described how differences in stability of NF-κB-induced mRNA transcripts and TNF concentration influence the dynamics of expression of different inflammatory genes. We find that both the extent and the timing of NF-κB-mediated macrophage activation are critical to control of the immune response to Mtb. However, the significance of the stability of TNF and chemokine mRNA transcripts is mostly due its effect on the extent of these responses. This is the first study, to our knowledge, that reveals the importance of the dynamics of various NF-κB-mediated responses on immunity to Mtb. Further, we show that manipulating the dynamics of these responses in a granuloma that is unable to contain infection due to, for example, pathogen-induced inhibition of NF-κB activation can significantly improve granuloma function.
Finally, our approach is an initial step toward understanding the molecular targets at the level of intracellular signaling pathways for control of the tissue-scale outcomes of the immune response to Mtb, particularly granuloma formation. We anticipate that other factors, including crosstalk between signaling mediated by the Mtb bacteria and other cytokines through various types of receptors and different signaling pathways (Basak and Hoffmann, 2008) in various types of cells, or the noise resulting from discrete regulation of TNFR activity and transcription regulation (Lipniacki et al., 2007) will further influence the ability of a granuloma to contain infection. Importantly, our unique multi-scale approach provides a platform for discovering which intracellular interventions may enhance immunity to Mtb, and has implications for testing and optimizing new vaccine and therapeutic strategies that minimize non-specific or off-target side effects.
Conflict of Interest Statement
The authors declare that the research was conducted in the absence of any commercial or financial relationships that could be construed as a potential conflict of interest.
Acknowledgments
This work was supported by National Institutes of Health Grants R33 HL092844 (Jennifer J. Linderman), R33 HL092853 and R01 HL106804 (Denise E. Kirschner), and R01 EB012579 (awarded to both Denise E. Kirschner and Jennifer J. Linderman), and by a University of Michigan Rackham Predoctoral Fellowship awarded to Mohammad Fallahi-Sichani. We thank Simeone Marino for helpful discussions, and Paul Wolberg for software engineering and programming.
References
Albeck, J. G., Burke, J. M., Spencer, S. L., Lauffenburger, D. A., and Sorger, P. K. (2008). Modeling a snap-action, variable-delay switch controlling extrinsic cell death. PLoS Biol. 6, 2831–2852. doi:10.1371/journal.pbio.0060299
Algood, H. M., Chan, J., and Flynn, J. L. (2003). Chemokines and tuberculosis. Cytokine Growth Factor Rev. 14, 467–477.
Algood, H. M., Lin, P. L., Yankura, D., Jones, A., Chan, J., and Flynn, J. L. (2004). TNF influences chemokine expression of macrophages in vitro and that of CD11b+ cells in vivo during Mycobacterium tuberculosis infection. J. Immunol. 172, 6846–6857.
Barry, C. E., Boshoff, H. I. 3rd, Dartois, V., Dick, T., Ehrt, S., Flynn, J., Schnappinger, D., Wilkinson, R. J., and Young, D. (2009). The spectrum of latent tuberculosis: rethinking the biology and intervention strategies. Nat. Rev. Microbiol. 7, 845–855.
Basak, S., and Hoffmann, A. (2008). Crosstalk via the NF-kappaB signaling system. Cytokine Growth Factor Rev. 19, 187–197.
Bean, A. G., Roach, D. R., Briscoe, H., France, M. P., Korner, H., Sedgwick, J. D., and Britton, W. J. (1999). Structural deficiencies in granuloma formation in TNF gene-targeted mice underlie the heightened susceptibility to aerosol Mycobacterium tuberculosis infection, which is not compensated for by lymphotoxin. J. Immunol. 162, 3504–3511.
Beg, A. A., and Baltimore, D. (1996). An essential role for NF-kappaB in preventing TNF-alpha-induced cell death. Science 274, 782–784.
Blower, S. M., and Dowlatabadi, H. (1994). Sensitivity and uncertainty analysis of complex models of disease transmission: an HIV model, as an example. Int. Stat. Rev. 62, 229–243.
Chakravarty, S. D., Zhu, G., Tsai, M. C., Mohan, V. P., Marino, S., Kirschner, D. E., Huang, L., Flynn, J., and Chan, J. (2008). Tumor necrosis factor blockade in chronic murine tuberculosis enhances granulomatous inflammation and disorganizes granulomas in the lungs. Infect. Immun. 76, 916–926.
Cheong, R., Bergmann, A., Werner, S. L., Regal, J., Hoffmann, A., and Levchenko, A. (2006). Transient IkappaB kinase activity mediates temporal NF-kappaB dynamics in response to a wide range of tumor necrosis factor-alpha doses. J. Biol. Chem. 281, 2945–2950.
Cheong, R., Hoffmann, A., and Levchenko, A. (2008). Understanding NF-kappaB signaling via mathematical modeling. Mol. Syst. Biol. 4, 192.
Clay, H., Volkman, H. E., and Ramakrishnan, L. (2008). Tumor necrosis factor signaling mediates resistance to mycobacteria by inhibiting bacterial growth and macrophage death. Immunity 29, 283–294.
Cooper, A. M. (2009). Cell-mediated immune responses in tuberculosis. Annu. Rev. Immunol. 27, 393–422.
Davis, J. M., and Ramakrishnan, L. (2008). “The very pulse of the machine”: the tuberculous granuloma in motion. Immunity 28, 146–148.
Fallahi-Sichani, M., El-Kebir, M., Marino, S., Kirschner, D. E., and Linderman, J. J. (2011). Multiscale computational modeling reveals a critical role for TNF-{alpha} receptor 1 dynamics in tuberculosis granuloma formation. J. Immunol. 186, 3472–3483.
Fallahi-Sichani, M., Flynn, J. L., Linderman, J. J., and Kirschner, D. E. (2012). Differential risk of tuberculosis reactivation among anti-TNF therapies is due to drug binding kinetics and permeability. J. Immunol. 188, 3169–3178.
Fallahi-Sichani, M., Schaller, M. A., Kirschner, D. E., Kunkel, S. L., and Linderman, J. J. (2010). Identification of key processes that control tumor necrosis factor availability in a tuberculosis granuloma. PLoS Comput. Biol. 6, e1000778. doi:10.1371/journal.pcbi.1000778
Flynn, J. L. (2004). Immunology of tuberculosis and implications in vaccine development. Tuberculosis (Edinb) 84, 93–101.
Flynn, J. L., Chan, J., and Lin, P. L. (2011). Macrophages and control of granulomatous inflammation in tuberculosis. Mucosal Immunol. 4, 271–278.
Flynn, J. L., Goldstein, M. M., Chan, J., Triebold, K. J., Pfeffer, K., Lowenstein, C. J., Schreiber, R., Mak, T. W., and Bloom, B. R. (1995). Tumor necrosis factor-alpha is required in the protective immune response against Mycobacterium tuberculosis in mice. Immunity 2, 561–572.
Flynn, J. L., and Klein, E. (2010). “Pulmonary tuberculosis in monkeys,” in A Color Atlas of Comparative Pulmonary Tuberculosis Histopathology, eds. J. Leong, V. Dartois, and T. Dick (Boca Raton: CRC Press, Taylor & Francis Publishers), 83–106.
Fotin-Mleczek, M., Henkler, F., Samel, D., Reichwein, M., Hausser, A., Parmryd, I., Scheurich, P., Schmid, J. A., and Wajant, H. (2002). Apoptotic crosstalk of TNF receptors: TNF-R2-induces depletion of TRAF2 and IAP proteins and accelerates TNF-R1-dependent activation of caspase-8. J. Cell. Sci. 115, 2757–2770.
Gutierrez, M. G., Mishra, B. B., Jordao, L., Elliott, E., Anes, E., and Griffiths, G. (2008). NF-kappa B activation controls phagolysosome fusion-mediated killing of mycobacteria by macrophages. J. Immunol. 181, 2651–2663.
Hao, S., and Baltimore, D. (2009). The stability of mRNA influences the temporal order of the induction of genes encoding inflammatory molecules. Nat. Immunol. 10, 281–288.
Harris, J., Hope, J. C., and Keane, J. (2008). Tumor necrosis factor blockers influence macrophage responses to Mycobacterium tuberculosis. J. Infect. Dis. 198, 1842–1850.
Hoffmann, A., and Baltimore, D. (2006). Circuitry of nuclear factor kappaB signaling. Immunol. Rev. 210, 171–186.
Hoffmann, A., Levchenko, A., Scott, M. L., and Baltimore, D. (2002). The IkappaB-NF-kappaB signaling module: temporal control and selective gene activation. Science 298, 1241–1245.
Karin, M., and Lin, A. (2002). NF-kappaB at the crossroads of life and death. Nat. Immunol. 3, 221–227.
Keane, J., Balcewicz-Sablinska, M. K., Remold, H. G., Chupp, G. L., Meek, B. B., Fenton, M. J., and Kornfeld, H. (1997). Infection by Mycobacterium tuberculosis promotes human alveolar macrophage apoptosis. Infect. Immun. 65, 298–304.
Keane, J., Gershon, S., Wise, R. P., Mirabile-Levens, E., Kasznica, J., Schwieterman, W. D., Siegel, J. N., and Braun, M. M. (2001). Tuberculosis associated with infliximab, a tumor necrosis factor alpha-neutralizing agent. N. Engl. J. Med. 345, 1098–1104.
Keane, J., Shurtleff, B., and Kornfeld, H. (2002). TNF-dependent BALB/c murine macrophage apoptosis following Mycobacterium tuberculosis infection inhibits bacillary growth in an IFN-gamma independent manner. Tuberculosis (Edinb) 82, 55–61.
Kearns, J. D., and Hoffmann, A. (2009). Integrating computational and biochemical studies to explore mechanisms in NF-{kappa}B signaling. J. Biol. Chem. 284, 5439–5443.
Kirschner, D. E., Young, D., and Flynn, J. L. (2010). Tuberculosis: global approaches to a global disease. Curr. Opin. Biotechnol. 21, 524–531.
Lin, P. L., and Flynn, J. L. (2010). Understanding latent tuberculosis: a moving target. J. Immunol. 185, 15–22.
Lin, P. L., Myers, A., Smith, L., Bigbee, C., Bigbee, M., Fuhrman, C., Grieser, H., Chiosea, I., Voitenek, N. N., Capuano, S. V., Klein, E., and Flynn, J. L. (2010). Tumor necrosis factor neutralization results in disseminated disease in acute and latent Mycobacterium tuberculosis infection with normal granuloma structure in a cynomolgus macaque model. Arthritis Rheum. 62, 340–350.
Lin, P. L., Pawar, S., Myers, A., Pegu, A., Fuhrman, C., Reinhart, T. A., Capuano, S. V., Klein, E., and Flynn, J. L. (2006). Early events in Mycobacterium tuberculosis infection in cynomolgus macaques. Infect. Immun. 74, 3790–3803.
Lin, P. L., Plessner, H. L., Voitenok, N. N., and Flynn, J. L. (2007). Tumor necrosis factor and tuberculosis. J. Investig. Dermatol. Symp. Proc. 12, 22–25.
Lipniacki, T., Puszynski, K., Paszek, P., Brasier, A. R., and Kimmel, M. (2007). Single TNFalpha trimers mediating NF-kappaB activation: stochastic robustness of NF-kappaB signaling. BMC Bioinformatics 8, 376. doi:10.1186/1471-2105-8-376
Marino, S., El-Kebir, M., and Kirschner, D. (2011). A hybrid multi-compartment model of granuloma formation and T cell priming in Tuberculosis. J. Theor. Biol. 280, 50–62.
Marino, S., Fallahi-Sichani, M., Linderman, J. J., and Kirschner, D. E. (2012). “Mathematical Models of Anti-TNF Therapies and their Correlation with Tuberculosis,” in Antibody-Mediated Drug Delivery Systems: Concepts, Technology and Applications, eds. Y. Pathak, and S. Benita (Hoboken, NJ: John Wiley & Sons, Inc.), 83–104.
Marino, S., Hogue, I. B., Ray, C. J., and Kirschner, D. E. (2008). A methodology for performing global uncertainty and sensitivity analysis in systems biology. J. Theor. Biol. 254, 178–196.
Marino, S., Sud, D., Plessner, H., Lin, P. L., Chan, J., Flynn, J. L., and Kirschner, D. E. (2007). Differences in reactivation of tuberculosis induced from anti-TNF treatments are based on bioavailability in granulomatous tissue. PLoS Comput. Biol. 3, 1909–1924. doi:10.1371/journal.pcbi.0030194
Morel, P. A., Ta’asan, S., Morel, B. F., Kirschner, D. E., and Flynn, J. L. (2006). New insights into mathematical modeling of the immune system. Immunol. Res. 36, 157–165.
Mosser, D. M., and Edwards, J. P. (2008). Exploring the full spectrum of macrophage activation. Nat. Rev. Immunol. 8, 958–969.
Nelson, D. E., Ihekwaba, A. E., Elliott, M., Johnson, J. R., Gibney, C. A., Foreman, B. E., Nelson, G., See, V., Horton, C. A., Spiller, D. G., Edwards, S. W., McDowell, H. P., Unitt, J. F., Sullivan, E., Grimley, R., Benson, N., Broomhead, D., Kell, D. B., and White, M. R. (2004). Oscillations in NF-kappaB signaling control the dynamics of gene expression. Science 306, 704–708.
Pahl, H. L. (1999). Activators and target genes of Rel/NF-kappaB transcription factors. Oncogene 18, 6853–6866.
Rangamani, P., and Sirovich, L. (2007). Survival and apoptotic pathways initiated by TNF-alpha: modeling and predictions. Biotechnol. Bioeng. 97, 1216–1229.
Ray, J. C., Flynn, J. L., and Kirschner, D. E. (2009). Synergy between individual TNF-dependent functions determines granuloma performance for controlling Mycobacterium tuberculosis infection. J. Immunol. 182, 3706–3717.
Roach, D. R., Bean, A. G., Demangel, C., France, M. P., Briscoe, H., and Britton, W. J. (2002). TNF regulates chemokine induction essential for cell recruitment, granuloma formation, and clearance of mycobacterial infection. J. Immunol. 168, 4620–4627.
Russell, D. G., Barry, C. E. III, and Flynn, J. L. (2010). Tuberculosis: what we don’t know can, and does, hurt us. Science 328, 852–856.
Segovia-Juarez, J. L., Ganguli, S., and Kirschner, D. (2004). Identifying control mechanisms of granuloma formation during M. tuberculosis infection using an agent-based model. J. Theor. Biol. 231, 357–376.
Tay, S., Hughey, J. J., Lee, T. K., Lipniacki, T., Quake, S. R., and Covert, M. W. (2010). Single-cell NF-kappaB dynamics reveal digital activation and analogue information processing. Nature 466, 267–271.
Tsai, M. C., Chakravarty, S., Zhu, G., Xu, J., Tanaka, K., Koch, C., Tufariello, J., Flynn, J., and Chan, J. (2006). Characterization of the tuberculous granuloma in murine and human lungs: cellular composition and relative tissue oxygen tension. Cell. Microbiol. 8, 218–232.
Turner, O.C., Basaraba, R. J., Frank, A. A., and Orme, I. M. (2003). “Granuloma formation in mouse and guinea pig models of experimental tuberculosis,” in Granulomatous Infections and Inflammations: Cellular and Molecular Mechanisms, ed. D. L. Boros (Washington, DC: ASM Press), 65–84.
Ulrichs, T., Kosmiadi, G. A., Trusov, V., Jorg, S., Pradl, L., Titukhina, M., Mishenko, V., Gushina, N., and Kaufmann, S. H. (2004). Human tuberculous granulomas induce peripheral lymphoid follicle-like structures to orchestrate local host defence in the lung. J. Pathol. 204, 217–228.
Van Antwerp, D. J., Martin, S. J., Kafri, T., Green, D. R., and Verma, I. M. (1996). Suppression of TNF-alpha-induced apoptosis by NF-kappaB. Science 274, 787–789.
Wertz, I. E., O’Rourke, K. M., Zhou, H., Eby, M., Aravind, L., Seshagiri, S., Wu, P., Wiesmann, C., Baker, R., Boone, D. L., Ma, A., Koonin, E. V., and Dixit, V. M. (2004). De-ubiquitination and ubiquitin ligase domains of A20 downregulate NF-kappaB signalling. Nature 430, 694–699.
Winthrop, K. L. (2006). Risk and prevention of tuberculosis and other serious opportunistic infections associated with the inhibition of tumor necrosis factor. Nat. Clin. Pract. Rheumatol. 2, 602–610.
Yamada, H., Mizuno, S., Reza-Gholizadeh, M., and Sugawara, I. (2001). Relative importance of NF-kappaB p50 in mycobacterial infection. Infect. Immun. 69, 7100–7105.
Yde, P., Mengel, B., Jensen, M. H., Krishna, S., and Trusina, A. (2011). Modeling the NF-kappaB mediated inflammatory response predicts cytokine waves in tissue. BMC Syst. Biol. 5, 115–123.
Appendix
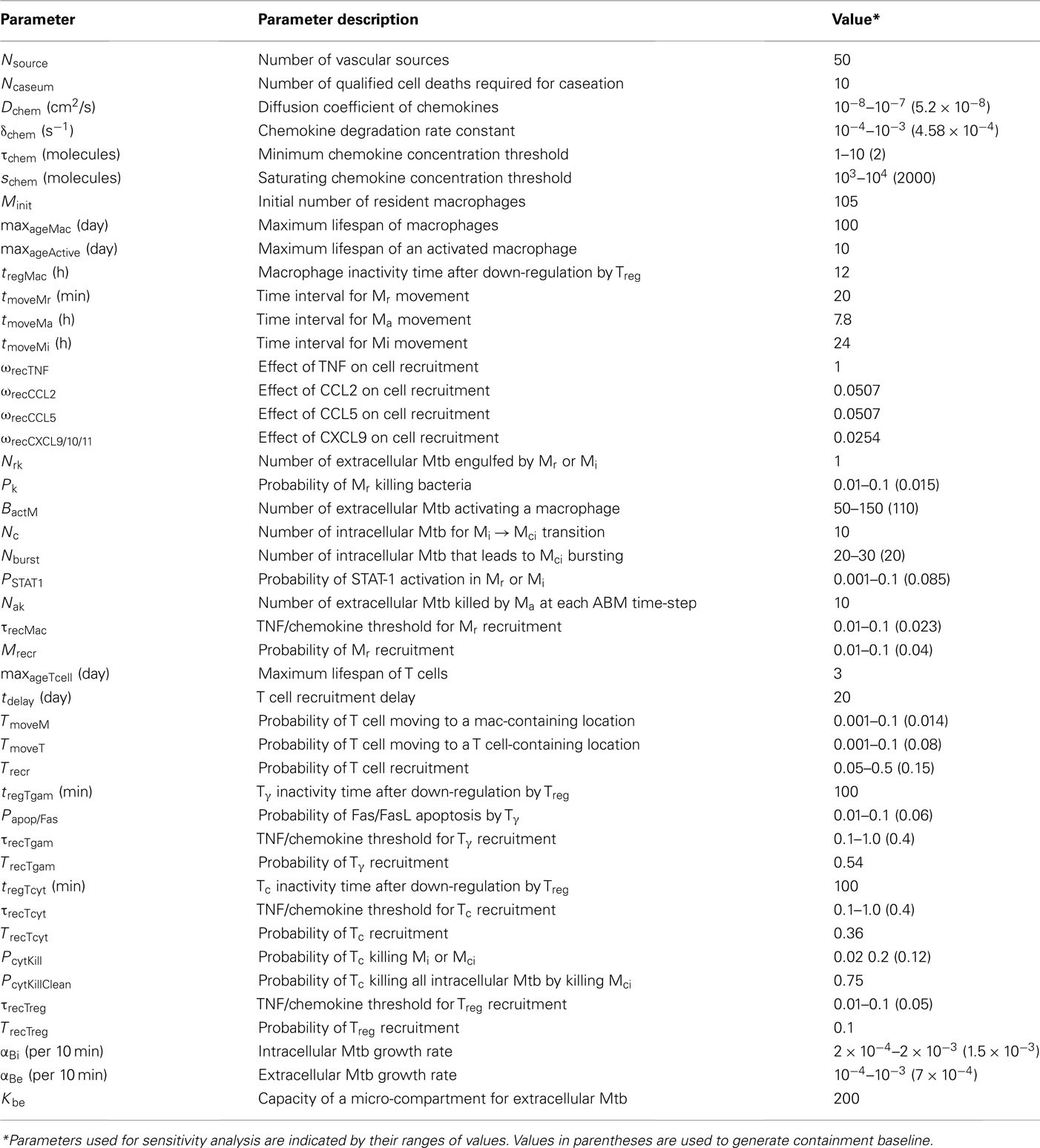
Table A1. TNF-independent and cellular/tissue-scale parameters, definitions, and values estimated from literature or approximated via uncertainty analysis as described in Ray et al. (2009); Fallahi-Sichani et al. (2011).
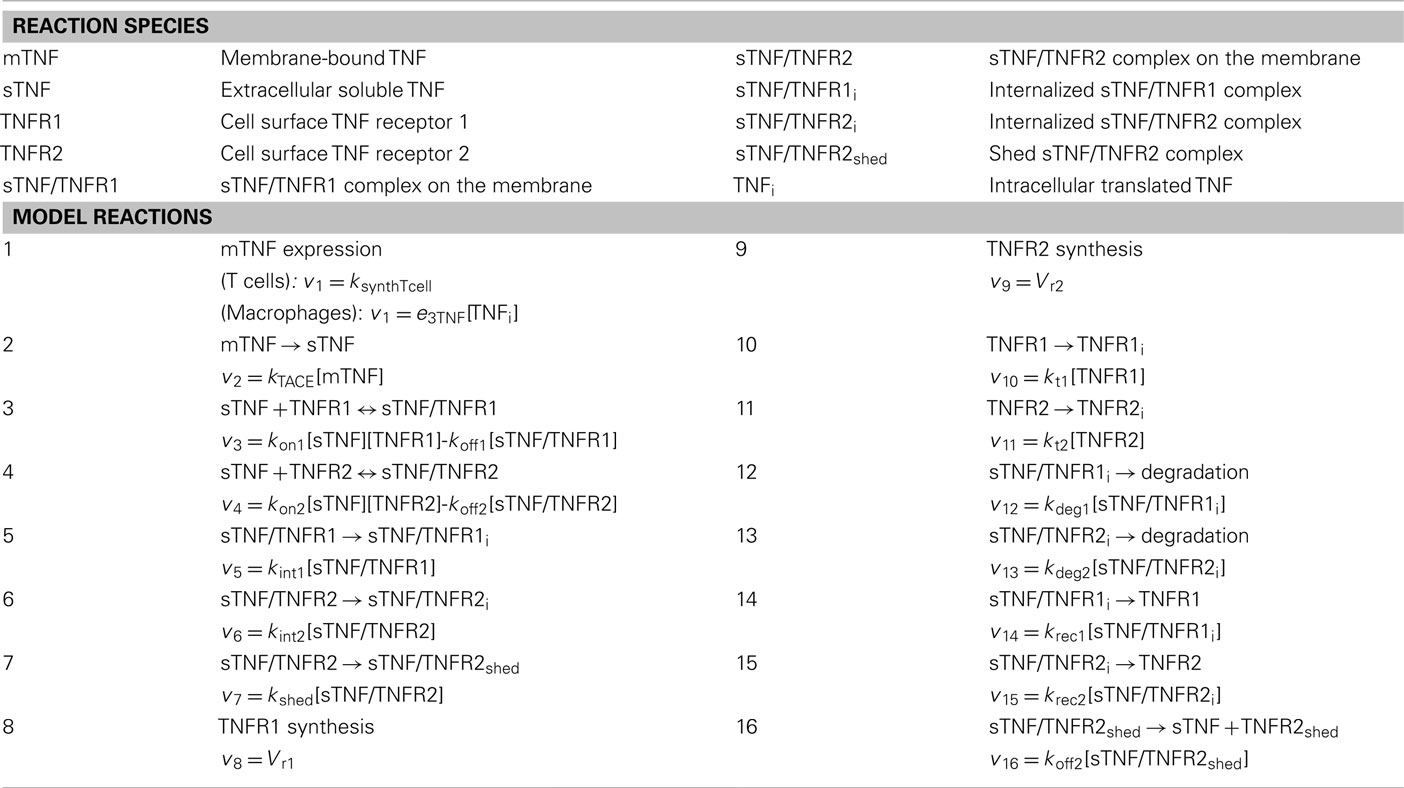
Table A2. Definition of reaction species, reactions describing TNF/TNFR processes and their rates (vi).
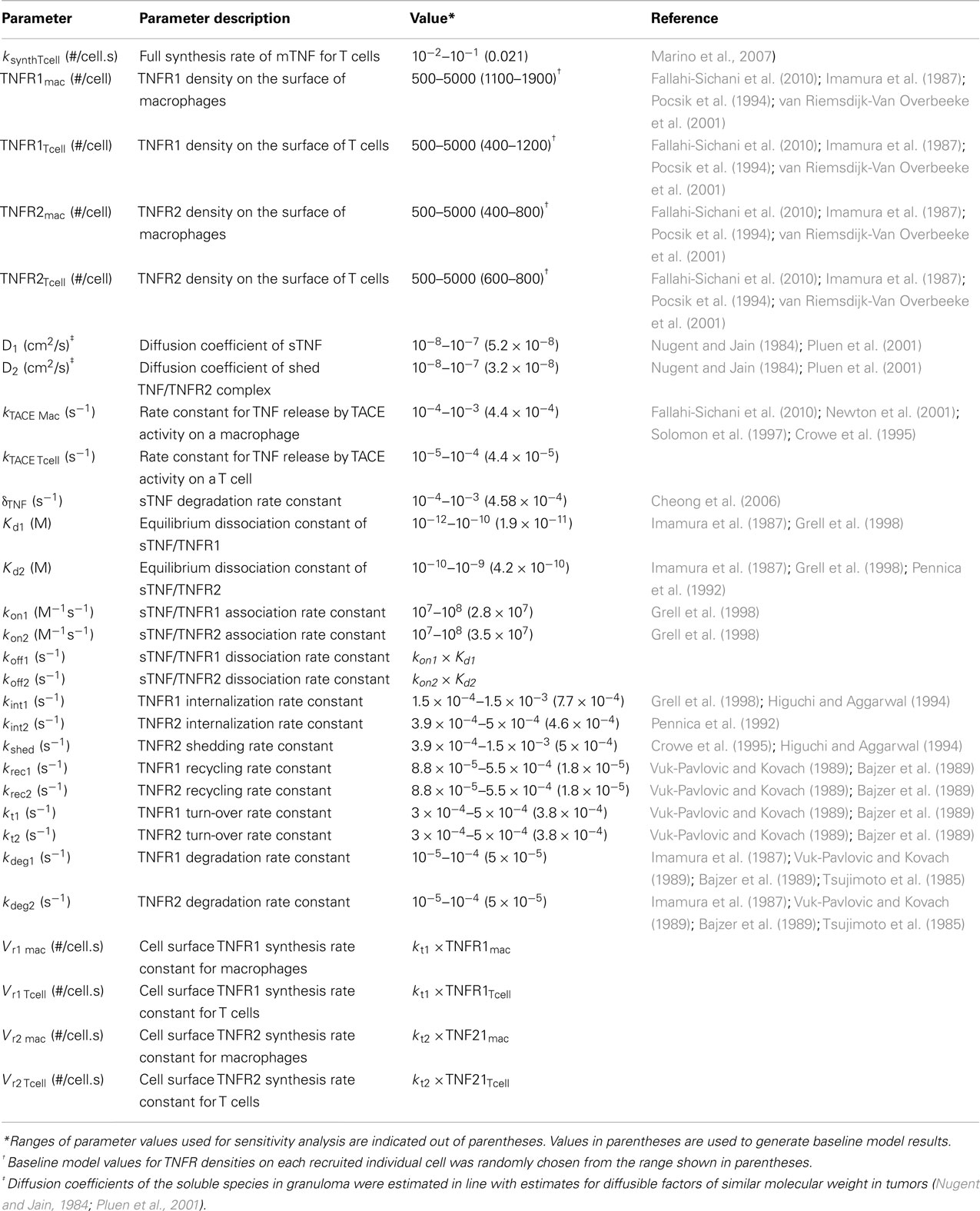
Table A3. Molecular/single-cell scale TNF/TNFR parameters, definitions and values estimated from literature.
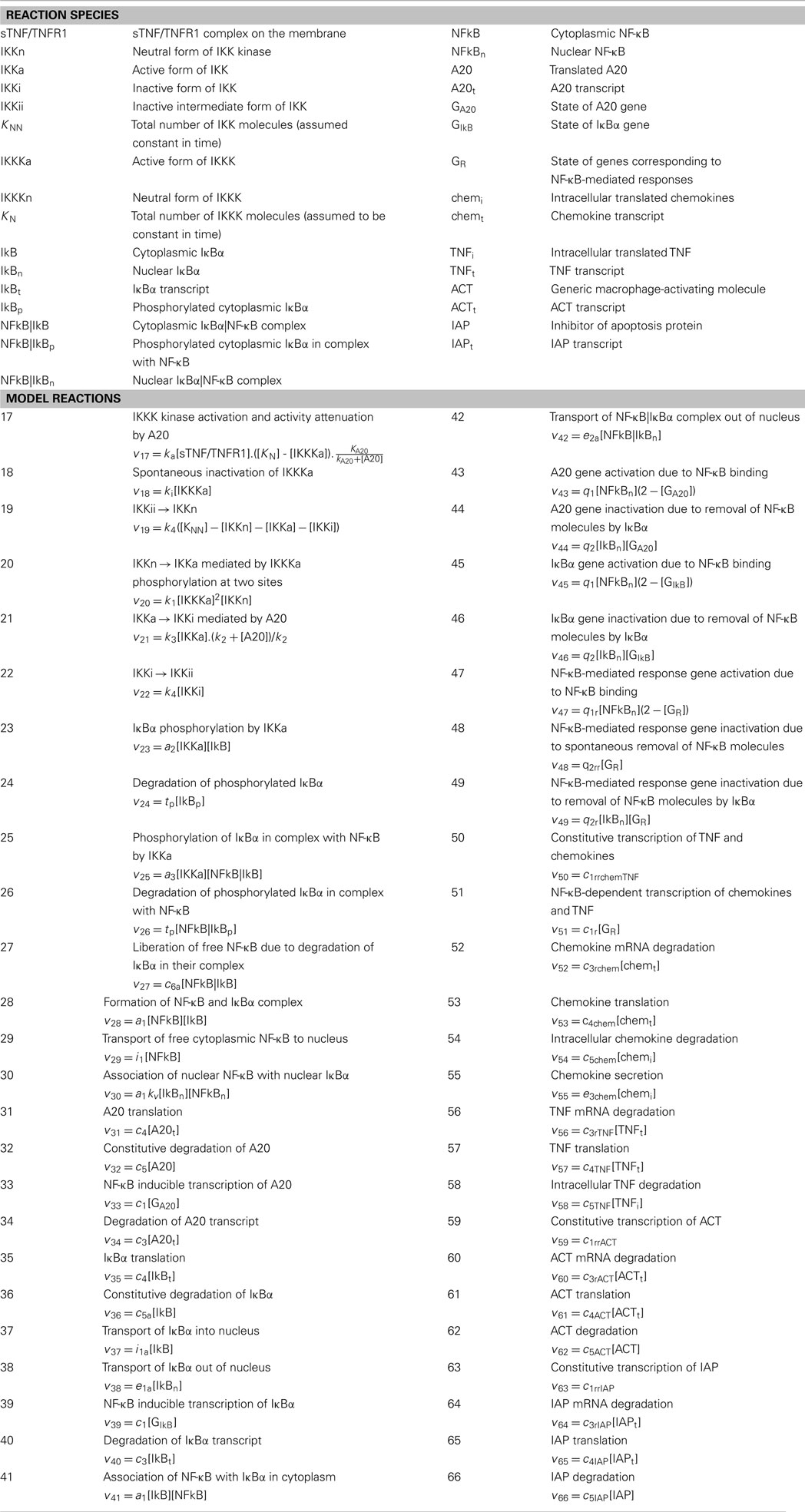
Table A4. Definition of reaction species, reactions describing NF-κB signaling and response-associated processes in macrophages and their rates (vi).
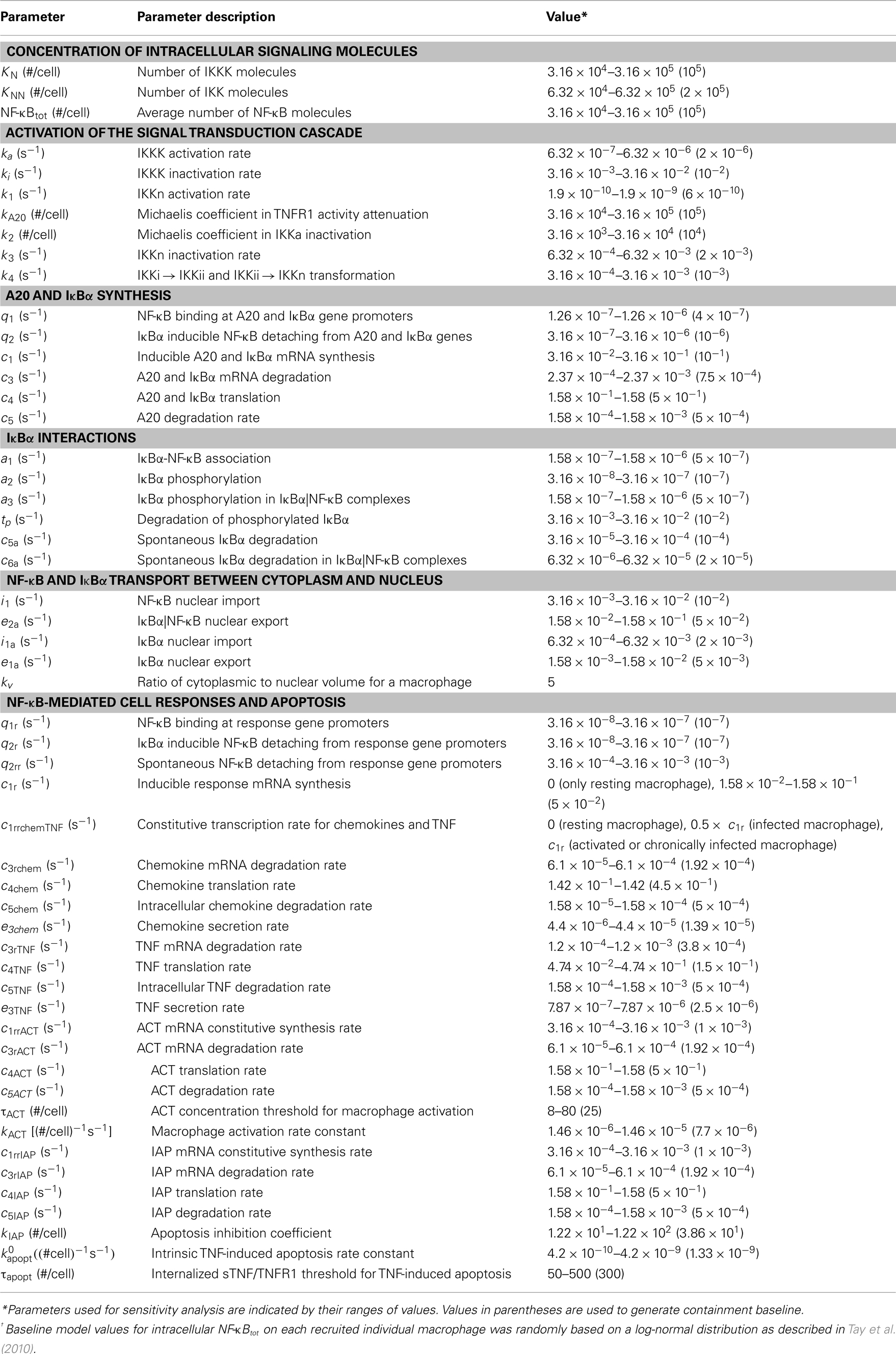
Table A5. Molecular/single-cell scale NF-κB signaling-associated parameters, definitions and values from Tay et al. (2010), or approximated via uncertainty analysis.
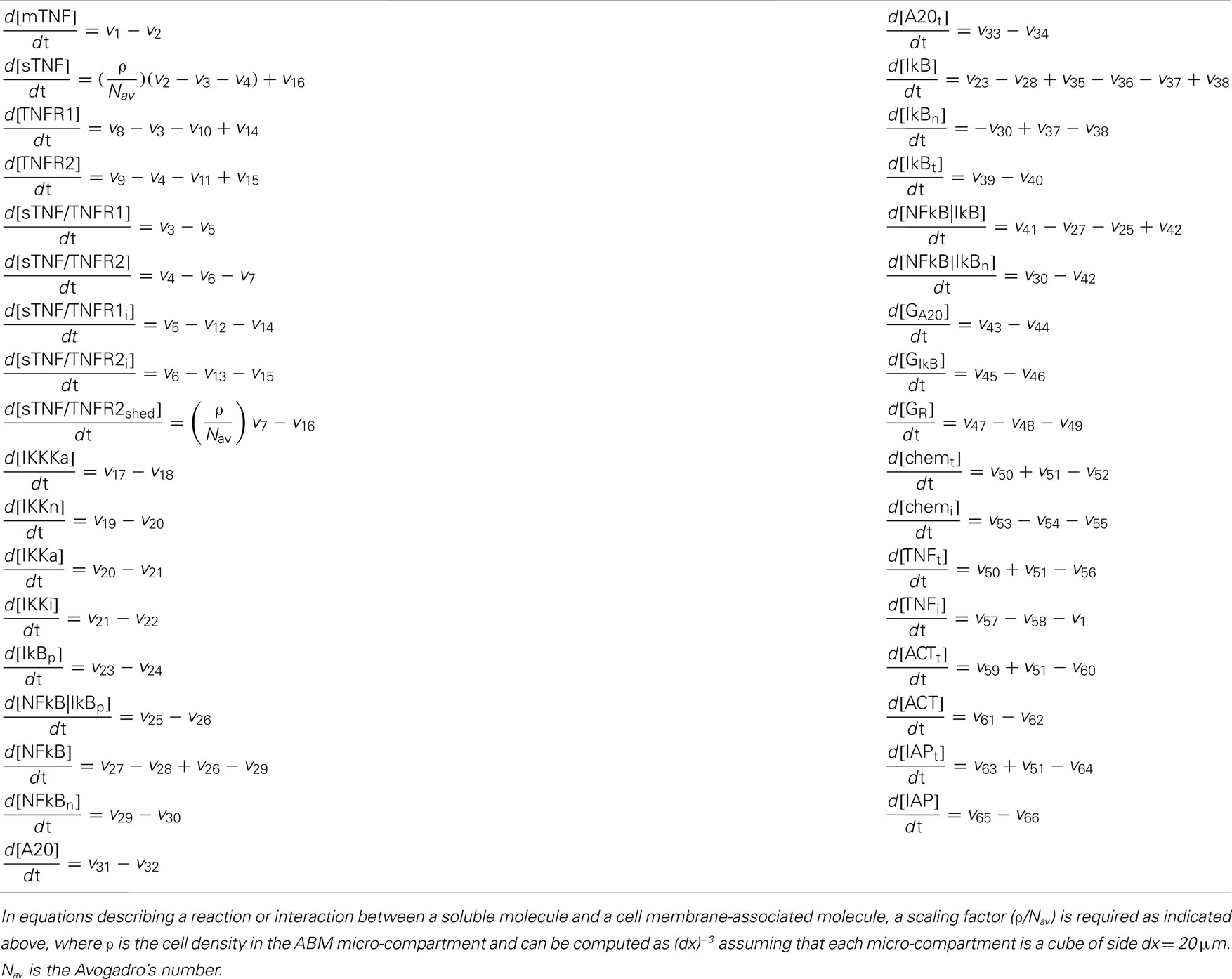
Table A6. Differential equations describing molecular single-cell scale TNF/TNFR and NF-κB signaling and response-associated processes.
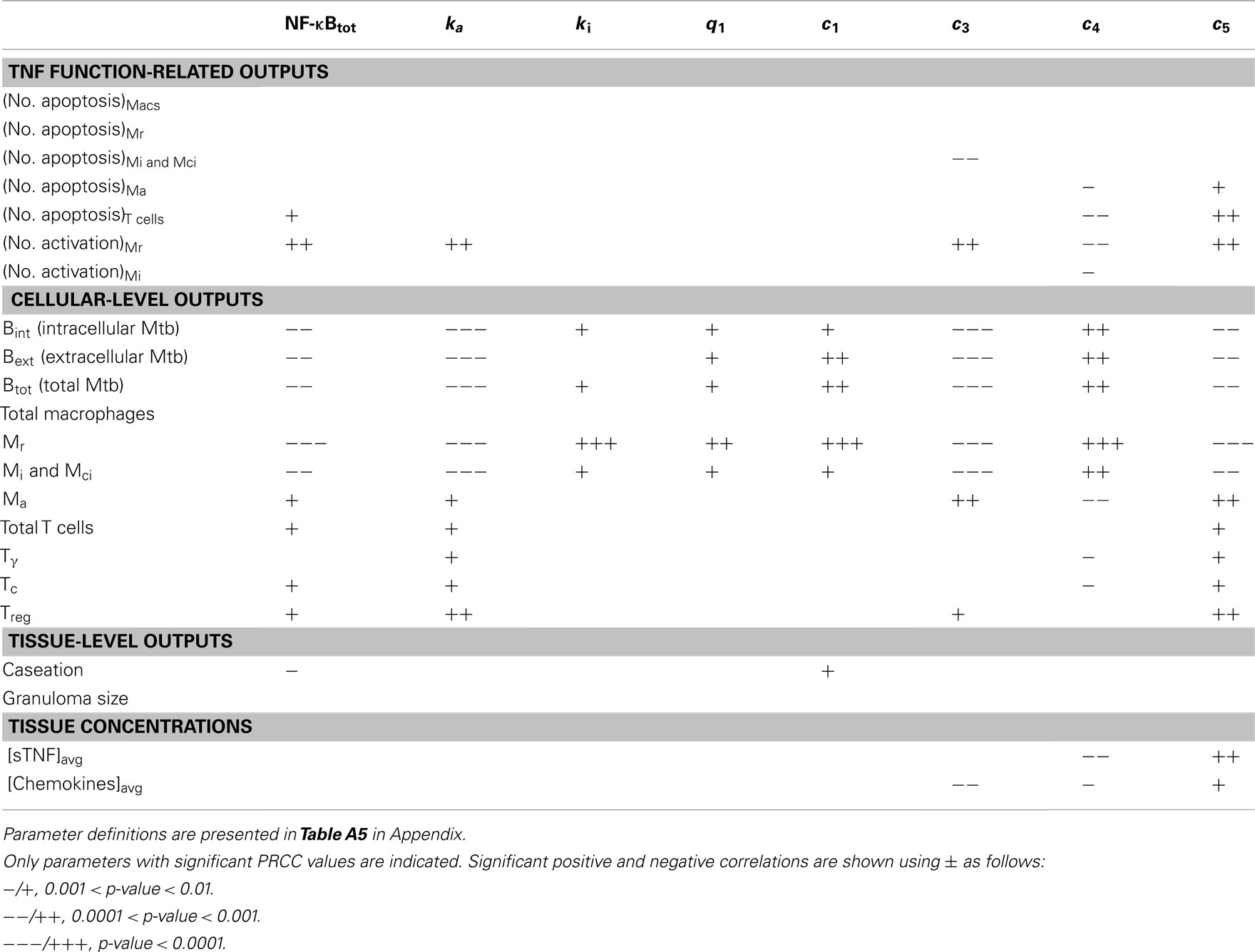
Table A7. LHS sensitivity analysis results for the effect of important NF-κB-associated model parameters (groups 1–3) on model outputs at day 200 post-infection.
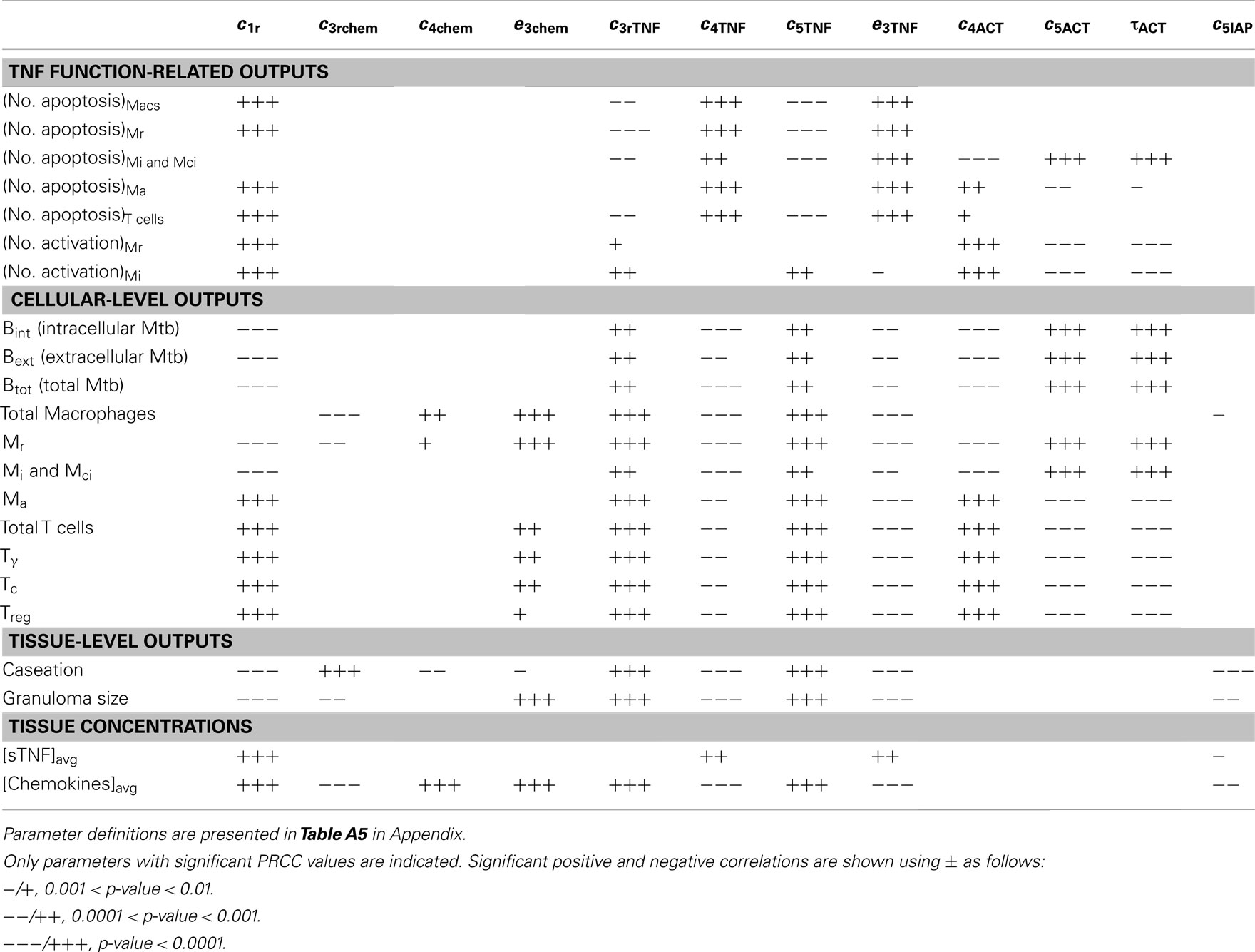
Table A8. LHS sensitivity analysis results for the effect of important NF-κB-associated model parameters (group 6) on model outputs at day 200 post-infection.
References
Bajzer, Z., Myers, A. C., and Vuk-Pavlovic, S. (1989). Binding, internalization, and intracellular processing of proteins interacting with recycling receptors. A kinetic analysis. J. Biol. Chem. 264, 13623–13631.
Crowe, P. D., Walter, B. N., Mohler, K. M., Otten-Evans, C., Black, R. A., and Ware, C. F. (1995). A metalloprotease inhibitor blocks shedding of the 80-kD TNF receptor and TNF processing in T lymphocytes. J. Exp. Med. 181, 1205–1210.
Grell, M., Wajant, H., Zimmermann, G., and Scheurich, P. (1998). The type 1 receptor (CD120a) is the high-affinity receptor for soluble tumor necrosis factor. Proc. Natl. Acad. Sci. U.S.A. 95, 570–575.
Higuchi, M., and Aggarwal, B. B. (1994). TNF induces internalization of the p60 receptor and shedding of the p80 receptor. J. Immunol. 152, 3550–3558.
Imamura, K., Spriggs, D., and Kufe, D. (1987). Expression of tumor necrosis factor receptors on human monocytes and internalization of receptor bound ligand. J. Immunol. 139, 2989–2992.
Newton, R. C., Solomon, K. A., Covington, M. B., Decicco, C. P., Haley, P. J., Friedman, S. M., and Vaddi, K. (2001). Biology of TACE inhibition. Ann. Rheum. Dis. 60(Suppl. 3), iii25–iii32.
Nugent, L. J., and Jain, R. K. (1984). Extravascular diffusion in normal and neoplastic tissues. Cancer Res. 44, 238–244.
Pennica, D., Lam, V. T., Mize, N. K., Weber, R. F., Lewis, M., Fendly, B. M., Lipari, M. T., and Goeddel, D. V. (1992). Biochemical properties of the 75-kDa tumor necrosis factor receptor. Characterization of ligand binding, internalization, and receptor phosphorylation. J. Biol. Chem. 267, 21172–21178.
Pluen, A., Boucher, Y., Ramanujan, S., McKee, T. D., Gohongi, T., di Tomaso, E., Brown, E. B., Izumi, Y., Campbell, R. B., Berk, D. A., and Jain, R. K. (2001). Role of tumor-host interactions in interstitial diffusion of macromolecules: cranial vs. subcutaneous tumors. Proc. Natl. Acad. Sci. U.S.A. 98, 4628–4633.
Pocsik, E., Mihalik, R., Ali-Osman, F., and Aggarwal, B. B. (1994). Cell density-dependent regulation of cell surface expression of two types of human tumor necrosis factor receptors and its effect on cellular response. J. Cell. Biochem. 54, 453–464.
Solomon, K. A., Covington, M. B., DeCicco, C. P., and Newton, R. C. (1997). The fate of pro-TNF-alpha following inhibition of metalloprotease-dependent processing to soluble TNF-alpha in human monocytes. J. Immunol. 159, 4524–4531.
Tsujimoto, M., Yip, Y. K., and Vilcek, J. (1985). Tumor necrosis factor: specific binding and internalization in sensitive and resistant cells. Proc. Natl. Acad. Sci. U.S.A. 82, 7626–7630.
van Riemsdijk-Van Overbeeke, I. C., Baan, C. C., Knoop, C. J., Loonen, E. H., Zietse, R., and Weimar, W. (2001). Quantitative flow cytometry shows activation of the TNF-alpha system but not of the IL-2 system at the single cell level in renal replacement therapy. Nephrol. Dial. Transplant. 16, 1430–1435.
Keywords: tuberculosis, granuloma, NF-κB signaling pathway, tumor necrosis factor, systems biology, multi-scale modeling
Citation: Fallahi-Sichani M, Kirschner DE and Linderman JJ (2012) NF-κB signaling dynamics play a key role in infection control in tuberculosis. Front. Physio. 3:170. doi: 10.3389/fphys.2012.00170
Received: 19 February 2012; Paper pending published: 26 March 2012;
Accepted: 09 May 2012; Published online: 06 June 2012.
Edited by:
Jennie Larkin, National Heart, Lung, and Blood Institute, USAReviewed by:
Sriram Neelamegham, State University of New York at Buffalo, USADonald Paul Gaver, Tulane University, USA
Copyright: © 2012 Fallahi-Sichani, Kirschner and Linderman. This is an open-access article distributed under the terms of the Creative Commons Attribution Non Commercial License, which permits non-commercial use, distribution, and reproduction in other forums, provided the original authors and source are credited.
*Correspondence: Denise E. Kirschner, Department of Microbiology and Immunology, University of Michigan Medical School, Ann Arbor, MI 48109, USA. e-mail:a2lyc2NobmVAdW1pY2guZWR1; Jennifer J. Linderman, Department of Chemical Engineering, University of Michigan, Ann Arbor, MI 48109, USA. e-mail:bGluZGVybWFAdW1pY2guZWR1
†Present address: Mohammad Fallahi-Sichani, Department of Systems Biology, Harvard Medical School, Boston, MA 02115, USA.