- Metabolomics and Biomarkers, Department of BioAnalytical Science, Nestlé Research Center, Nestec Ltd., Lausanne, Switzerland
Dietary preferences and nutrients composition have been shown to influence human and gut microbial metabolism, which ultimately has specific effects on health and diseases’ risk. Increasingly, results from molecular biology and microbiology demonstrate the key role of the gut microbiota metabolic interface to the overall mammalian host’s health status. There is therefore raising interest in nutrition research to characterize the molecular foundations of the gut microbial–mammalian cross talk at both physiological and biochemical pathway levels. Tackling these challenges can be achieved through systems biology approaches, such as metabolomics, to underpin the highly complex metabolic exchanges between diverse biological compartments, including organs, systemic biofluids, and microbial symbionts. By the development of specific biomarkers for prediction of health and disease, metabolomics is increasingly used in clinical applications as regard to disease etiology, diagnostic stratification, and potentially mechanism of action of therapeutical and nutraceutical solutions. Surprisingly, an increasing number of metabolomics investigations in pre-clinical and clinical studies based on proton nuclear magnetic resonance (1H NMR) spectroscopy and mass spectrometry provided compelling evidence that system wide and organ-specific biochemical processes are under the influence of gut microbial metabolism. This review aims at describing recent applications of metabolomics in clinical fields where main objective is to discern the biochemical mechanisms under the influence of the gut microbiota, with insight into gastrointestinal health and diseases diagnostics and improvement of homeostasis metabolic regulation.
System Biology Approaches to Decipher the Complex Metabolic Networks of Mammalian Organisms
Recent advances in nutrition research aims at determining and further our understanding on the biochemical effects of nutrients and foods in order to promote beneficial nutritional and health status at individual and population level. In this, the mammalian gut microbial relationship is a key determinant by performing multiple digestive, immune, and metabolic functions (Nicholson et al., 2005; Dethlefsen et al., 2007; Ley et al., 2008). The gastrointestinal tract of adult humans contain around 1.5 k of biomass composed by gut microbial symbiotic and commensal organisms that are in intimate communication with the host, which is the result of a long period of co-adaptation between the host genotype and the gut microbiome (Gill et al., 2006). The gut microbiota provides to the host specific capacities ranging from dietary energy recovery from nutrients, generating digestible carbohydrates and short chain fatty acids (SCFAs) from otherwise non-digestible fibers, amino acids, and vitamins, to protection against infectious diseases (Cummings et al., 1987; Gilmore and Ferretti, 2003; Xu et al., 2003; Tannock, 2005). Gut microbial activities were shown to be extremely specific, as exemplified via its essential role in the development and maintenance of the mucosal innate and adaptive immune system (Guigoz et al., 2008). In parallel, there is increasing awareness that many health disorders involve a significant perturbation of immune and energy metabolism which are intrinsically linked to the gut functionally ecology (Dunne, 2001; Turnbaugh et al., 2006; Dethlefsen et al., 2007).
The consortium of symbiotic gut microorganisms can be viewed as a metabolically adaptable, rapidly renewable, and metabolically flexible ecosystem varying in addition with the host’s age, diet, and health status. Consequently, the microbiome is a nutritional target today and might also become the foundation of future drug targeting and interventions (Jia et al., 2008). Systems biology approaches have emerged over the last two decades as a novel way forward to provide insights into the role of mammalian gut microbial metabolic interactions in individual susceptibility to health and disease outcomes. In particular, metabolomics offers new opportunities to explore individual needs, foods, and nutrients functionalities and formulate tangible biological hypotheses that can be followed-up and tested at individual and population scale.
Nowadays, metabolomics is based on the quantitative measurement of dynamic metabolic changes of living systems in response to genetic modifications or physiological stimuli, including nutrients, and drugs. Metabolites are the products and by-products of the many intricate biosynthetic and catabolic pathways existing in all living systems. Therefore, monitoring the resulting metabolic variations provides a unique insight into intra- and extra-cellular regulatory processes involved in our metabolic homeostasis. By the study of low molecular weight compounds in biofluids (blood and urine), stools, and tissues (intact biopsies or extracts; Beckonert et al., 2010), metabolomics assures the characterization of metabolic fingerprints that can be associated with individual phenotypes, which encompass dietary or disease status (Figure 1). Metabolomics employs mainly two analytical techniques based on 1H nuclear magnetic resonance (NMR) spectroscopy, and mass spectrometry (MS). MS methods are also commonly employed for global and targeted profiling and are inherently more sensitive, but require a more comprehensive sample preparation (Bruce et al., 2009) with separation of the metabolites components using either gas chromatography (GC) or liquid chromatography (LC). Both methods are then comprehensively used to generate high density data, from which meaningful biological information are recovered using advanced statistical tools (Eriksson et al., 2004; Montoliu et al., 2009). One recent analytical revolution – lipidomics – now enables the elucidations of molecular processes that involve the disruption of lipid metabolic enzymes and pathways. State-of-the art methods for characterizing the lipidome encompasses non-targeted profiling approach using a global shotgun approach (Ejsing et al., 2009), or alternatively, main lipid category separation using LC–MS approaches (Pietilainen et al., 2007). The emergence of “Nutrimetabolomics” illustrates the mutual link among nutrition and metabolomics research to study the effects of specific ingredients and food components elucidating the molecular mechanisms behind individual metabolic responses. However, deciphering food-induced metabolic reactions is very challenging since they vary greatly among individuals as differences in endogenous factors such as diet, stress, age, environment, genetics, lifestyle, and gut microbiota. In particular, recent advances in cellular and molecular biology have provided compelling evidence that the intestinal microbiota contribution to the overall health status of the host has been underestimated so far. Both system wide (i.e., whole organism) and organ-specific changes in metabolic profiles may have components indeed driven by gut microbial activities (Nicholson et al., 2005; Zheng et al., 2011). This review therefore aims at describing current advances in metabolomic studies where main objective is to discern the molecular pathways and mechanisms under the influence of gut microbial metabolism in the context of gastrointestinal diseases. Furthermore, emphasis is given to nutritional approaches, where the quest for homeostatic balance is dependent not only on the host but also on the nutritional modulation of the gut microbiota–host metabolic interactions.
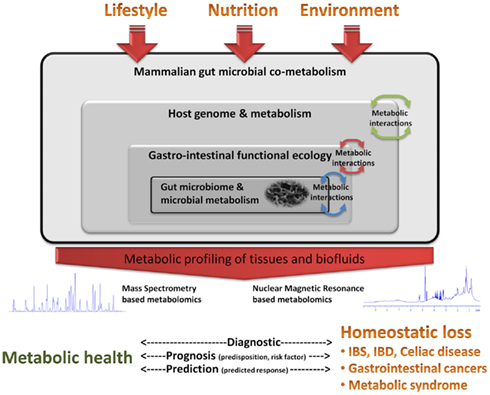
Figure 1. Conceptualization of nutritional metabonomics for gastrointestinal health and risk management. The metabolic status of individuals results from gene, environment, lifestyle, food, and gut microbiota continuous interactions. The relationship between host and gut microbiota, with the metabolic influence of gut symbionts at different level of biological organization, underpins the depth of symbiotic control over multiple host cell metabolic regulatory functions.
Gut Flora and Host Metabolism
The gastrointestinal tract is a complex ecosystem host to a diverse and highly evolved microbial community composed of hundreds of different microbial species. The human body contains circa 10 trillion parenchymal cells, but the gut contains approximately 100 trillion of microbial organisms, which vary in community composition through life according to lifestyle and nutrition (Beale, 2002; Xu and Gordon, 2003; Tannock, 2005; Xu et al., 2007). Increasing scientific evidence has been reported on the fundamental role of gut microbiota in both positive and negative triggers of specific metabolic states of individuals and populations (Dunne, 2001; Verdu et al., 2004; Backhed et al., 2005; Gill et al., 2006). Therefore, it is today critical to understand the molecular foundations of the impact of the gut microbial activity on human health and nutritional status. For instance, mammalian–microbial symbiosis can be influential in the etiology and development of several diseases, including metabolic syndrome (Ley et al., 2006), insulin resistance (Dumas et al., 2006), inflammatory bowel disease (IBD; Gupta et al., 2000), irritable bowel syndrome (IBS; Sartor, 2004), and gastrointestinal cancers (Dunne, 2001).
Both system wide (i.e., whole organism) and organ-specific changes in metabolic profiles may have components driven by gut microbial activities (Martin et al., 2009a, 2010a). The current omics revolution offers an unprecedented opportunity to explore how our gut symbionts contribute to our physiology and how they can be exploited to develop new therapeutic and nutritional strategies. Optimal systems biology approaches lies in the combination of state-of-the-art microbial and metabolic monitoring and discovery approaches, including metagenomics and metabolomics. In particular, rRNA and metagenomic sequencing offers novel means to decipher the content, diversity, and functioning of the microbial gut community, with the ultimate aims to help further the understanding of the impact of the gut microbes on human health (Qin et al., 2010). Recently, Qin et al. (2010) reported an exhaustive gene catalog containing virtually all of the prevalent gut microbial genes in large human cohort, and reported to which extent many bacterial species are shared by different individuals. Moreover the authors also pinpointed how such an approach could be used for global characterization of the genetic potential of ecologically complex environments (Qin et al., 2010).
Recently, applications of top-down system biology approaches described the depth and width of gut microbial influence on host metabolic functions, resulting in modulation of host lipid, carbohydrate, and amino acid metabolism at organ and system levels (Martin et al., 2009a; Claus et al., 2011; Merrifield et al., 2011; Mestdagh et al., 2011). For instance, the specific metabolic activities of a single gut bacterial species can provide the host with new biochemical compounds in sufficient amount to be detected in the general blood circulation (Wikoff et al., 2009). Martin et al. exemplified how the gut microbial modulation of the gastrointestinal system (Martin et al., 2009b) and extensive microbial–mammalian co-metabolism may fine-tune host metabolic processes and may induce metabolic deregulations (Martin et al., 2007a). In particular, the authors highlighted the different bacterial modulation of the bile acid metabolism and enterohepatic cycle, with consequent effects on the absorption of dietary fat and concomitant to lipid accumulation in the livers of animals harboring a non-adapted gut microbiota. In these investigations, the gut microbiota exerts deep and long-range control over multiple host cell metabolic pathways impacting on health and nutritional outcomes (Martin et al., 2007a; Clayton et al., 2009) via, for instance, the co-metabolism of bile acids and inferred modulation of lipid and cholesterol metabolism (Swann et al., 2011). Together, these studies suggest that controlling the dynamics of the gut microbiome to maintain or re-establish a balanced and well-adapted microbiota could help in preventing some microbial-related metabolic disorders, such as hepato-gastrointestinal diseases.
The Role of Gut Microbes in Determining Gastrointestinal Functional Ecology
The intestinal tract is one of the most important interface between mammalian metabolism and the environment along which the varying metabolic activities of the gut microbiota not only determine absorption, digestion, metabolism, and excretion of dietary nutrients but also shape regio-specifically the surrounding and distant host cell biochemical processes (Hooper et al., 2002). Advances in metabolomic applications are providing novel insights into the molecular foundations of these host–microbial relationships and their influence onto health and disease risks (Martin et al., 2009b, 2010b). In particular, a series of investigations in humans (Wang et al., 2007), rats (Wang et al., 2005), and gnotobiotic mice (Martin et al., 2007b, 2009b) have provided a set of reference metabolic profiles of gut intestinal biopsies that can be used not only to assess compartment structure and function but also the gut microbial impact at the tissue level (Martin et al., 2007b). These studies therefore indicated that the type of gut microbiota may be a key factor in the determination of the intestinal homeostasis, osmo-protection, motility, and calorie recovery from the diet. For instance, the ileum is regarded as the major site for absorption of luminal bile acids and emulsified dietary lipids, and the report of higher concentrations of glycerophospholipids, glutathione, taurine, and betaine in this tissue was consistent with its role in lipid and bile acid metabolism. The jejunum of the mice harboring a non-adapted microbiota showed metabolic similarities to the ileal profile, marked by higher levels of glutathione, and lower concentrations of its precursors in γ-glutamyl cycle when compared to conventional animals. Such an observation illustrates the essential role of the gut microbiota to influence surrounding tissue metabolic pathways, to ultimately shape a host environment that fosters their implantation and persistence. The investigations also provided evidence that microbial-dependent variations along the upper intestine were observed, which is often underestimated due to low bacterial populations. These signatures described that the type of gut bacteria affects the utilization efficiency of dietary proteins and amino acids and their subsequent availability to extra-intestinal tissues.
Metabolomics Applications to Inflammatory Bowel Disorders: IBD, IBS, and Celiac Disease
The influence of the gut microorganisms in the progression of human diseases is nowadays a main concern in the etiology and/or maintenance of gut dysfunctions, such as IBS (Madden and Hunter, 2002) or IBD (Bickston et al., 2003). The incidence and prevalence of IBD continue to rise worldwide and are the cause of much morbidity and disability (Loftus Jr., 2004). The clinically defined and idiopathic forms of IBD) encompassing ulcerative colitis (UC) and Crohn’s disease (CD), are spontaneously relapsing and immunologically mediated chronic disorders of the gastrointestinal tract (Shanahan, 2001; Bickston et al., 2003). Both manifestations are mediated by common and distinct mechanisms influenced by multiple environmental factors and specific genetic predispositions. Moreover, the involvement of gut microbiota in the chronic mucosal immune activation underlying the IBD pathogenesis have been widely documented, in particular as a key component in the duration and the prevention/treatment of chronic intestinal inflammation (Swidsinski et al., 2002; Sartor, 2004; Haller, 2006). Advancing knowledge regarding the mechanisms of IBD has led to the development of different therapeutic solutions based on surgery (Harper et al., 1983; Korelitz et al., 1985), cannabinoids (Duncan et al., 2005), immunosuppression (Remicade, Mesalamine, etc.; Siemanowski and Regueiro, 2007; Actis et al., 2008), and alternatively probiotic supplementation (Reid et al., 2003). However, the IBD diagnosis is only possible at an advanced disease state when symptoms are detectable by radiology, endoscopy with biopsy of pathological lesions (Ahmadi and Polyak, 2007). There is therefore a need to develop new strategies for early prognosis and treatment of IBD.
There is increasing awareness in gastrointestinal science for the prognostic, diagnostic, and monitoring potential of metabolomics, as well as the opportunity to provide new insights into IBD pathogenesis (Lin et al., 2011). A consistent approach to define early biomarkers of pathogenesis to be used for disease surveillance is the comprehensive analysis of the real endpoints of physiological regulatory processes, the metabolites, which may be associated with different IBD determinants. Winterkamp et al. (2002) reported previously how N-methylhistamine, a key metabolite in mast cell metabolism involved in the pathogenesis of IBD, could be used as an indicator of disease activity in patients. Mast cells are indeed present all along the gastrointestinal tract within all layers of the gut wall, and may influence acute and chronic inflammation episodes (IBD, celiac disease, infectious diarrheas) by releasing an array of mediators. Functional studies revealed the release of elevated amounts of mast cell mediators such as histamine and tryptase in the intestinal mucosa as well as increased levels of histamine in the gut lumen of patients with IBD, both indicating a local up-regulation of mast cells in these inflammatory conditions of yet unknown origin (Winterkamp et al., 2002). In this study the urinary excretion of N-methylhistamine was associated with elevated histamine production and metabolism in CD and UC, and could be used as a reliable diagnostic tool to monitor clinical and endoscopic disease activity in IBD.
Metabolomics was also proven to be a valuable diagnostic tool to differentiate CD from UC, but also active and quiescent UC, as per the analysis of intact gut biopsies and colonocytes (Bezabeh et al., 2001; Bjerrum et al., 2010). These studies showed that the metabolic profiles of biopsies and colonocytes can be used to differentiate active UC from controls. In the biopsies from patients with active UC, higher concentrations of glutathione and of a range of amino acids were associated with lower levels of lipid, glycerophosphocholine, myo-inositol, and betaine. The changes in glycerophospholipids and inositol were further confirmed in colonocytes studies. The authors also described that 20% of inactive UC patients had similar profiles to those who were in an active state. This study demonstrates the possibilities of metabolomics as a diagnostic tool in active and quiescent UC and provides new insight into pathophysiological mechanisms. In addition, Williams et al. (2009) demonstrated how urinary metabolic profiling could be employed to distinguish between CD from UC, which is critical for disease management. The most discriminant metabolites included hippurate, 4-cresol sulfate, and formate, which are well known for their gut microbial co-metabolism origin (Marchesi et al., 2007). In CD, UC, and controls, there is a gradual decrease in the concentration of aromatic metabolites, respectively, whilst formate gradually increased.
These metabolomics observations are in agreement with other studies where stool microbial and metabolic composition was investigated in gastrointestinal diseases. All together, the monitoring of the fecal metabolome was proven to be of relevance to unravel diagnostic information for IBD (Marchesi et al., 2007). Such measurable outcomes provide some essential insights – yet limited – into a small range of microbial activities within the colon. Marchesi et al. (2007) described the first characterization of fecal extracts of IBD patients using metabolomics as a diagnostic tool for differentiating patients affected with UC and CD, hence improving disease surveillance. The fecal extracts of both CD and UC patients were characterized by reduced levels of butyrate, acetate, methylamine, and trimethylamine in comparison with a control population, suggesting changes in the gut microbial community. Also, elevated quantities of amino acids were present in the feces from both disease groups, implying malabsorption caused by the inflammatory disease or an element of protein losing enteropathy. Furthermore, glycerol resonances were a dominant feature of fecal spectra from patients with CD but were present in much lower intensity in the control and UC groups. Recently, Le Gall et al. (2011) described through metabolomics analysis of fecal extracts combined with microbial profiling the alteration of both population and metabolic activity of the gut microbiota in UC and IBS. The authors followed-up a populations of healthy controls, IBS, and UC patients over a period of 4 years. NMR-based metabolomics resulted in a good prediction of UC against controls, but could not discriminate robustly enough IBS from the two other conditions. Whereas the fecal excretion of SCFAs and amino acids remain stable across the groups, strong differences were detected in the content of taurine, cadaverine, bile acid, and branched chain fatty acids. The difference in the metabolic profiles observed in fecal water extracts of UC and healthy controls was correlated with the distinct difference in the composition of the gut microbiota, and demonstrate relationships between the disease state and the handling of dietary lipids. Jansson et al. (2009) also illustrated the potential of ion cyclotron resonance Fourier transform MS (ICR-FT/MS) to determine the contribution of metabolites produced by the gut microbiota toward disease status of the host via fecal monitoring. Biochemical pathways involved in the metabolism and or synthesis of amino acids, fatty acids, bile acids, and arachidonic acid were also highlighted in colonic and ileal CD patients. Hong et al. (2010) reported the use of fecal metabolomics to assess the effects of lactic acid probiotic in a mouse model of acute colitis induced with dextran sulfate sodium (DSS). DSS treatment was associated with a significant decrease of several amino acids, as well as butyrate, uracil, and hypoxanthine. These changes were correlated with increases of monosaccharides, glucose, and trimethylamine in the feces. Histological damage, myeloperoxidase activity, and malondialdehyde content of colon tissue were reduced, whereas colon length increased in mice supplemented with the probiotics. Interestingly, the probiotic preventive treatment resulted in increased levels of acetate, butyrate, and glutamine and decreased levels of trimethylamine concomitant to the protective effects against DSS-induced colitis, which suggests the modulation of the gut microbiota is of importance.
A large majority of studies have focused on UC in different animal models by exploring plasma (Schicho et al., 2010), urine (Murdoch et al., 2008; Lin et al., 2010; Schicho et al., 2010; Otter et al., 2011), and biopsies (Balasubramanian et al., 2008; Sharma et al., 2010). However, most of the studies describe metabolic phenotypes at an advanced disease state when symptoms can already be diagnosed by endoscopy. Recent applications on the monitoring of metabolic alterations before and during the development of the disease were reported in pre-clinical studies, and suggest that metabolomics might help to define early IBD biomarkers. Metabolomics was also successfully applied to studying UC associated metabolism, using blood plasma (Martin et al., 2009c) or urine (Murdoch et al., 2008) metabolic profiling. In both studies, the gradual development of colitis was monitored from the age of 1 up to 24 weeks using Interleukin 10 deficient (IL-10−/−) mice in combination with histopathology analyses. Overall, chronic inflammation appears from 8 weeks onward, and was associated with altered cecum and colon morphologies and increased inflammatory cell infiltration into the mucosa and the submucosa. Blood plasma metabolic profiling showed marked energy homeostasis loss with impaired metabolism of lipoproteins and glycosylated proteins (Martin et al., 2009c). In particular, IL-10−/− mice were characterized by decreased levels of VLDL and increased concentrations of LDL and polyunsaturated lipids, which are related to the etiology of IBD. Moreover, higher levels of lactate, pyruvate, citrate, and lowered concentration of circulating glucose suggested increased fatty acid oxidation and glycolysis. In addition, UC was associated with higher plasma levels of free amino acids that can reflect increased muscle atrophy protein breakdown and use of amino acids as metabolic fuel. Moreover, after week 8, the IL-10 gene-deficient mice also showed major urinary metabolic differences with marked increased concentrations of fucose and metabolites modulated by the gut microbiota, namely trimethylamine and trimethylamine-N-oxide, as well as a decreased in Krebs cycle intermediates (Murdoch et al., 2008). The authors suggested that the increased urinary fucose excretion could be related to the lower incorporation of fucose into red blood cell membranes and subsequent increased serum concentration associated with glycoproteins. Alternatively, the changes in urinary fucose excretion could be associated with changes in the gut microflora modulation of novo production of fucose for fucosylated glycans.
Recently, Baur et al. (2011) monitored the effects and consequences of inflammatory processes associated with the gradual evolution of CD-like ileitis on the local metabolism taking advantage of TNFΔARE/WT mice. The TNFΔARE/WT mouse model displays similarity with the immune and tissue-related phenotype of human CD ileitis. The authors employed non-targeted 1H NMR spectroscopy and targeted LC–MS based metabolic profiling techniques in combination with histological, body weight and composition analysis and fecal energy loss to characterize site specific and systemic metabolic phenotypes along CD-like ileitis development. The results describe different biological processes associated to the disease onset, including modifications of the general cell membrane composition, energy homeostasis loss, and higher levels of inflammatory lipid mediators (cytokines and eicosanoids). Tissue pathology was associated with local expression of pro-inflammatory cytokines (i.e., TNF, IFNγ, and IL-6) and increased concentrations of cholesterol, plasmalogens, sphingomyelins, glycerophospholipids, and PUFAs. The authors suggested that lipid accumulation could be subsequent to the reported massive immune cell infiltration leading to an alteration of the overall lipid membrane composition. CD-induced alterations of cholesterol and PUFAs levels could be associated to relative changes in lipid rafts of intestinal epithelial cells with possible implication in the increased paracellular permeability reported in the pathophysiology of CD. Taken together, this new set of metabolic findings on IBD in both pre-clinical and clinical studies could be used to generate mechanistic hypotheses on the intimate cross talk between inflammation, gut microbiota, and host metabolism that is ultimately foreseen to lead to new early onset biomarkers and therapeutic targets and solutions.
Irritable bowel syndrome is another major multifactorial digestive disorders affecting around 20% of the industrialized adult population for which underlying mechanisms must be further elucidated (Loftus Jr., 2004). The pathology is generally associated to a broad range of symptoms including abdominal pain, bowel habit disturbance, changes in stool frequency and consistency. The role of abnormal microbiota composition in the pathogenesis of IBS is also supported by clinical and experimental data (Madden and Hunter, 2002; Si et al., 2004; Malinen et al., 2005; Matto et al., 2005) although causal relationships with IBS development is not yet established. Attempt was made to study IBS with a combination of microbial and metabolomic outcomes using stool analysis (Ponnusamy et al., 2011). Using a GC–MS methodology, the authors highlighted higher levels of specific amino acids (alanine and pyroglutamate) and phenolic compounds (hydroxyphenyl acetate and hydroxyphenyl propionate) with IBS. The authors associated these metabolic changes of protein and carbohydrate energy metabolism in the gut with alterations of specific gut microbial populations, including the abundance of lactobacilli and Clostridium. Martin et al. (2006) employed a NMR-based metabolomics of plasma, jejunal wall, and myenteric plexus–longitudinal muscle tissues collected from a post-infective IBS model to assess molecular changes in relation to the disease and nutritional interventions. Post-infective IBS was associated with a modification of energy metabolism to meet the energy requirements due to the intestinal muscular hyper-contractility, as noted with changes in energy metabolic intermediates, lipid, and amino acid metabolism. In addition, the jejunal wall tissues showed variations in the levels of gut microbiota-related metabolites that were correlated to the disruption of the gut microbiota observed in IBS. This study also confirmed the role of Lactobacillus paracasei probiotics in normalizing the muscular activity and the disturbed energy metabolism modulating glycogenesis and lipid oxidation. In addition, the effects of the probiotic L. paracasei and L. paracasei-free spent culture medium on the IBS mouse model were also evaluated. Treating the mice with L. paracasei probiotics alleviated gut muscle hyper-contractility and normalized the levels of immune markers suggesting amelioration of the inflammatory response (Verdu et al., 2004).
Celiac disease (CeD) is another multifactorial gastrointestinal disorder strongly associated with specific dietary features, which have both genetic and environmental determinants. CeD is broadly defined as an immune-mediated enteropathy induced in response to the ingestion of wheat gluten or related rye and barley proteins in genetically susceptible individuals. Bertini et al. (2009) demonstrated that combinatorial metabolomic analysis of blood sera and urine could help further the understanding of CeD. The metabolomic analysis of the biofluids collected from 34 CeD patients and 34 healthy controls. The classification accuracy of CeD and healthy control groups was 79.7–83.4% for serum and 69.3% for urine. CeD was associated with a significant reduction of the circulating levels of amino acids, lipids, pyruvate, and choline, and increased levels of glucose and the ketone body 3-hydroxybutyric acid, which described a major alteration of energy homeostatic regulation. Moreover, the urine metabolic signature provided a complementary description of metabolic processes under the influence of the gut microbial changes induced by CeD, marked by altered urinary excretion of indoxyl sulfate, meta-hydroxyphenylpropionate, and phenylacetylglycine. The highlighted changes in gut microbial co-metabolites may be associated to aberrant microbiota previously characterized in the small bowel of subjects suffering of CeD (Tjellstrom et al., 2005).
Clinical Applications for Metabolic Syndrome and Bariatric Surgery
The world wide pandemic rise of obesity prevalence is strongly linked to incidence of type 2 diabetes (T2D) and cardiovascular disorders. Recently, Newgard et al. (2009) have described a branched chain amino acids (BCAAs) related metabolic signature contributing to insulin resistance in obese human subjects. Furthermore, Suhre et al. (2010) recently applied metabolomic analyses to the study of an epidemiological cohort in which diabetes-related complications could be detected already under sub-clinical conditions. In addition to previously reported T2D biomarkers, including sugar metabolites, ketone bodies, and BCAA, metabolites resulting from perturbations of metabolic pathways linked to kidney dysfunction (3-indoxyl sulfate), lipid metabolism (glycerophospholipids, free fatty acids), and bile acid metabolism. Moreover, metabolomics was employed to decipher indicators of early onsets of pre-diabetes status, marked by alterations in fatty acid, tryptophan, uric acid, bile acid, and gut microbial metabolism (Zhao et al., 2010). Furthermore, non-alcoholic fatty liver disease (NAFLD) is increasingly considered as a main pathological determinant in various obesity and insulin resistance related diseases (Johnson et al., 2008; Fabbrini et al., 2010), with metabolomics and lipidomics being of great potential to further the understanding of disease etiology and progression. For instance, a remarkable study by Barr et al. (2010) integrated the analysis of human sera from non-diabetic, morbidly obese, biopsy-proven NAFLD patients, and a NAFLD mouse model. The study demonstrated reduced levels of creatine and increased concentrations in bile acids and eicosanoids associated with liver dysfunctions. More recently, metabolomics was successfully employed to demonstrate a relationship between gut microbial metabolism of dietary phosphatidylcholine and cardiovascular pathogenesis in humans (Wang et al., 2011). In particular, Wang et al. described how the circulating levels of choline, trimethylamine-N-oxide, and betaine were shown to predict the risk for cardiovascular events, which offers novel perspectives for therapeutical and nutraceutical approaches. Existing therapeutic strategies to obesity lies in changing dietary habits and lifestyle or pharmacotherapies, yet, with only marginally beneficial effects for morbidly obese patients. In such a context, bariatric surgery appears as an efficient therapeutic approach to counteract type 2 diabetes and morbid obesity (Li et al., 2011a,b). Nevertheless, if bariatric surgery clearly leads to rapid improvement in glucose metabolism, the molecular mechanisms of this so-called “metabolic surgery” remain poorly understood (Li et al., 2011a,b). Li et al. (2011a) have reported a metabolomic study in non-obese rats recovering from bariatric surgery. Intriguingly, substantial shifts of the main gut bacteria phyla toward higher concentrations of Proteobacteria, and lower concentrations of Firmicutes and Bacteroidetes in comparison with sham-operated rats were reported. Bariatric surgery was associated with profound alterations of gut microbial metabolism as noted by changing fecal and/or urinary levels of bile acids, amines, and cresols. Moreover, increased protein putrefaction was suggested by the concomitant changes in decreased tyrosine and increased putrescine and diaminoethane in feces. These changes in the gut functional ecology were associated with a modulation of the energy metabolism, as evidenced by a decreased urinary excretion of several intermediates of Krebs cycle.
Bariatric surgery also suggested the induction of a cytotoxic chemical environment in the gut content in pre-clinical studies (Li et al., 2011b). The fecal extracts obtained from rats after Roux-en-Y gastric bypass (RYGB) showed a high toxicity to rodent cells (mouse lymphoma cell line) when compared to sham-operated animals. The authors associated this toxicity to the gross structure of the microbiome, which was also strongly correlated with specific urinary and metabolic signatures. The rats after RYGB indeed showed a very strong alteration of the urinary excretion of gut bacterial co-metabolites, namely phenylacetylglycine and indoxyl sulfate in urine and gamma-aminobutyrate, putrescine, tyramine, and uracil in feces. This profound co-dependent response of mammalian and microbial metabolism suggests that RYGB exerts local and global metabolic effects which may have an influence on long-term cancer risk and cytotoxic load.
Clinical Applications for Cancer Diagnosis
Metabolomics is today vastly applied for diseases diagnosis, with cancer being one of the major applications. Conventional tumor markers are relatively unsuitable for detecting carcinoma at an early stage and such novel insights may become critical in the development of tailored approaches to reduce disease burden. Using plasma free amino acids profiling, Miyagi et al. (2011) proposed a novel diagnostic measurements for lung, gastric, colorectal, breast, and prostate cancer. Cancer patients and controls could be discriminated using multivariate analysis based on plasma free amino acids profiles. Interestingly, tryptophan was identified as one of the most important amino acids in relation to cancer progression. Ikeda et al. (2011) also investigated serum metabolic signatures in patients suffering of gastrointestinal cancers. Human serum samples from esophageal, gastric, and colorectal cancer (CRC) patients were compared to healthy volunteers. Amongst the most significantly altered metabolites, malonate, and serine were specific to esophageal cancer; 3-hydroxypropionate and pyruvate for gastric cancer and alanine, glutamine, and glucuronic lactone for CRC. Very recently, Song et al. (2011a,b) applied metabolomics to both sera and tissues biopsies to investigate biomarkers of gastric cancer that could help predicting carcinogenesis and metastasis at an early stage. Their analysis of biopsies using a GC–MS approach provided a set of 15 metabolites associated with the gastric cancer morbidity (Song et al., 2011a). This metabolic signature encompassed significant alterations of glycolysis, fatty acid oxidation, cholesterol, and amino acid metabolism (Song et al., 2011a). A similar approach was applied to monitor serum metabolic composition of gastric cancer patients, which confirmed these metabolic alterations in addition to nucleotide metabolism at systemic level (Song et al., 2011b).
Colorectal cancer, a very common cause of cancer morbidity, particularly suffers from the absence of non- or minimally invasive diagnostics for early detection. Over 1 million cases of CRC are diagnosed each year worldwide making it one of the most spread cancer type (Cunningham et al., 2010). CRC is responsible for approximately 529,000 deaths each year, 60% of those occurring in developed countries (Goldberg, 2005). Numerous studies reported that the general population of intestinal bacteria is associated with initiation of colon cancer via the production of carcinogens, co-carcinogens, or pro-carcinogen substances (O’Keefe, 2008). Recently, Ritchie et al. (2010a) applied metabolomics to study CRC in American and Japanese populations using MS analyses of serum samples. Comprehensive metabolomic analyses revealed significantly reduced levels of 28–36 carbon-containing hydroxylated polyunsaturated ultra long-chain fatty acids (hPULCFAs). Structure elucidation studies on the C28 molecules revealed two molecular families harboring two or three hydroxyl groups and various unsaturation levels. The authors discussed the sensitivity and specificity of these putatively new CRC serum markers regardless of ethnic or geographic background. In a follow-up study, the authors investigated the effect of CRC therapy on the serum level of hPULCFAs (Ritchie et al., 2010b). The analyses indicated that the circulating levels of these hPULCFAs were not affected by conventional CRC treatment and hypothesized the occurrence of these markers in early stage of CRC development. 1H NMR spectroscopy was also applied to attempt to characterize the metabolic signature of malignant colon mucosa. Significant increased levels of taurine, choline-containing compounds, and lipids were reported in malignant tissues (Moreno et al., 1993). More recently, a proteomic study (Bi et al., 2006) reported alterations of multiple metabolic pathways namely purine and pyrimidine, glycolysis, gluconeogenesis, glucuronic acid synthesis, and tricarboxylic acid cycle also occur in CRC tissue samples. Chan et al. (2009) compared the metabolic profile of biopsied colorectal tumors and their matched normal mucosa obtained from 31 CRC patients using high-resolution magic angle spinning–NMR and GC–MS and obtained 31 marker metabolites that were able to distinguish normal from malignant samples and further colon from rectal cancers. These metabolic alterations were associated with CRC-induced metabolic perturbations such as increased tissue hypoxia, glycolysis, nucleotide biosynthesis, lipid metabolism, inflammation, and steroid metabolism. Moreover, the application of NMR metabolic profiling to fecal water extracts has interesting potential as a diagnostic tool for detecting CRC (Monleon et al., 2009). NMR metabolic profiles of fecal water extracts from CRC patients were markedly distinct from controls with lower concentrations of SCFAs (acetate and butyrate) and higher levels of proline and cysteine, the later being two major components of most colonic epithelium mucus glycoproteins.
Metabolic Assessment of Probiotics, Prebiotics, and Synbiotics Nutritional Interventions: Understanding the Molecular Foundations of Gut Microbial–Mammalian Metabolic Interactions for Disease Prevention
Food products are nowadays more and more commonly employed to modulate the composition of the gut microbiota with the main objective to prevent disease development and contribute to the health status’ maintenance. The effects of consuming live microbial supplements (probiotics) on the microbial ecology, and on human health and nutritional status have been extensively investigated over the past years (Collins and Gibson, 1999). Probiotic supplementation aims at replacing or reducing the number of potentially pathogenic bacteria in the intestine by enriching the populations of beneficial strains that ferment carbohydrates and that have little proteolytic activity. As an alternative, prebiotics, non-digestible food ingredients, generally oligosaccharides, modify the balance of the intestinal microbiota by stimulating the activity of health beneficial bacteria, such as lactobacilli and bifidobacteria (Gibson and Roberfroid, 1995; Collins and Gibson, 1999). Eventually, the combined use of prebiotics and probiotics, also called synbiotics, may offer superior effects in health maintenance through modulating the microbial functional ecology (Collins and Gibson, 1999; Schrezenmeir and de Vrese, 2001). Nowadays, clinical trials support the claims of efficacy of pro- and prebiotic nutritional intervention with regard to various proposed beneficial health effects, and this has raised the requirement for providing additional evidence and for elucidation of the molecular bases of their action. This can only be captured effectively by studying the global system response of an organism to an intervention using top-down systems biology approaches, as commented above under various gastrointestinal conditions. Such investigations can be conducted using germfree and gnotobiotic animals to evaluate the effects on host functions after colonizing the gut with a single microbial species (Falk et al., 1998). Martin et al. (2007b) employed such an approach to generate a region-specific metabolic mapping of the gut tract of a gnotobiotic mouse, in which they assessed the effects of mouse supplementation with L. paracasei. Colonization with live L. paracasei induced region-dependent changes in the metabolic profiles of all intestinal tissues, except for the colon, consistent with modulation of intestinal digestion, absorption of nutrients, energy metabolism, lipid synthesis, and protective functions. The results demonstrated the importance of the transgenomic metabolic interactions between L. paracasei and the host to modulate the gut functions.
In a series of related studies (Martin et al., 2008a,b, 2009b), probiotics effects were assessed on the mammalian metabolism using humanized mouse models. In a first study, integration of metabolic changes across diverse metabolic compartments including biofluids, tissues, and cecum levels of SCFAs in relation to microbial population modulation generated a novel top-down systems biology view of the host response to probiotic intervention (Martin et al., 2008b). Probiotic exposure resulted in altered hepatic lipid metabolism coupled with lowered plasma lipoprotein levels and apparent stimulated glycolysis in animals harboring a non-adapted gut microbiota. Probiotic treatments also altered a diverse range of pathways outcomes including amino acid metabolism, methylamines, and SCFAs. In a second study, it is the combination of probiotics (L. paracasei or L. rhamnosus), and two galactosyl-oligosaccharide prebiotics on the microbiome–mammalian metabolic interactions that was investigated (Martin et al., 2008a). The nutritionally induced microbial changes were associated with the modulation of a range of host metabolic pathways, namely gluconeogenesis, lipid, amino acid, and methylamine metabolism associated to different bacterial fermentation of carbohydrates. Finally, as envisioned through the complex arrays of host metabolic functions impacted by the nature and the activity of our gut microbiota, Martin et al. (2009a) described that the modulation of gut microbial metabolic function using pro-, pre-, or synbiotics results in significant perturbations in the metabolic pathways across the many individual biological compartments of a mammalian organism. In particular, they reported, using an extensive multi-compartmental modeling approach with metabolic imprints from 10 tissue/fluid samples that induced microbial changes affected host lipids, carbohydrates, and amino acids metabolism in every major mammalian biological analyzed compartment. These studies has showed the breadth and the depth of gut microbiome modulations of the intestinal metabolite pathways and indicate that metabolomics combined with key physiological indicators is a powerful and reliable approach to tackle the challenge to understand the molecular foundations of the beneficial or detrimental role of gut microbiota for health and disease prevention. Current scientific evidence, from pre-clinical studies, suggests that probiotic supplementation may appear as an potential nutritional solution to contribute to reducing tumor occurrence and precancerous lesions in the colon (Shahani and Ayebo, 1980). For instance, recent studies on the rat–azoxymethane model (Le Leu et al., 2005) displayed that the synbiotic combination of prebiotics and Bifidobacterium lactis probiotics significantly protected against the development of CRC. The study demonstrated that ingested prebiotics acted as a preferential metabolic substrate that enabled the selective growth of B. lactis and significantly contributed to exert its pro-apoptotic action. Because the synbiotic combination of these agents facilitates the apoptotic response to DNA damage by a cancer initiator in the colon of rats, the authors suggested that this warrants further study for its capacity to protect against CRC.
Conclusion: Metabolomics, Biomarkers, and Gastrointestinal Diseases
This non-exhaustive review on state-of-the art approaches to monitor changes in metabolite pattern demonstrate how powerful systems biology approaches are for assessing the clinical characteristics of gastrointestinal disorders. The current revolution in nutrigenomics sciences will at term offers new opportunities for preventive medicine, prognostic strategies and personalized healthcare, and nutritional management solutions. The rise in multifactorial disorders (e.g., IBS, IBD), the lack of understanding on the molecular processes at play (bariatric surgery) and the needs for disease prediction in asymptomatic conditions are some of the many questions that system biology approaches are well suited to address. Achieving this goal lies in our abilities to model and understand the complex web of metabolic interactions between genetics, metabolism, environmental factors, lifestyle, and nutrition. By opening a direct biochemical window into the metabolome, metabolomics is a unique science perfectly suited for the identification of biomarkers capable of providing better understanding of these complex metabolic phenomena. In such a context, new concepts joining biomarkers development and health monitoring are increasingly recognized as plausible strategies to provide new health oriented solution to the general population. In particular, major advances in immunology, metabolomics, and microbial ecology have shown that the contribution of the intestinal microbiota to the overall health status of the host has been so far underestimated. Through the rigorous characterization of interactions between the diet and the microbiota, metabolomics is providing new ventures for modulating the microbiota toward the improvement of human health. The prospective of preventing the progression of human diseases, by normalizing their effects, by specific nutritional intervention programs, such as probiotic supplementation, could benefit from the applications of nutrigenomics sciences for therapeutic surveillance and assessment of treatment efficacy.
Conflict of Interest Statement
The authors declare that the research was conducted in the absence of any commercial or financial relationships that could be construed as a potential conflict of interest.
References
Actis, G. C., Pazienza, P., and Rosina, F. (2008). Mesalamine for inflammatory bowel disease: recent reappraisals. Inflamm. Allergy Drug Targets 7, 1–5.
Ahmadi, A. A., and Polyak, S. (2007). Endoscopy/surveillance in inflammatory bowel disease. Surg. Clin. North Am. 87, 743–762.
Backhed, F., Ley, R., Sonnenburg, J., Peterson, D., and Gordon, J. (2005). Host-bacterial mutualism in the human intestine. Science 307, 1915–1920.
Balasubramanian, K., Kumar, S., Singh, R. R., Sharma, U., Ahuja, V., Makharia, G. K., and Jagannathan, N. R. (2008). Metabolism of the colonic mucosa in patients with inflammatory bowel diseases: an in vitro proton magnetic resonance spectroscopy study. Magn. Reson. Imaging 27, 79–86.
Barr, J., Vazquez-Chantada, M., Alonso, C., Perez-Cormenzana, M., Mayo, R., Galan, A., Caballeria, J., Martin-Duce, A., Tran, A., Wagner, C., Luka, Z., Lu, S. C., Castro, A., Le Marchand-Brustel, Y., Martinez-Chantar, M. L., Veyrie, N., Clement, K., Tordjman, J., Gual, P., and Mato, J. M. (2010). Liquid chromatography-mass spectrometry-based parallel metabolic profiling of human and mouse model serum reveals putative biomarkers associated with the progression of nonalcoholic fatty liver disease. J. Proteome Res. 9, 4501–4512.
Baur, P., Martin, F. P., Gruber, L., Bosco, N., Brahmbhatt, V., Collino, S., Guy, P., Montoliu, I., Rozman, J., Klingenspor, M., Tavazzi, I., Thorimbert, A., Rezzi, S., Kochhar, S., Benyacoub, J., Kollias, G., and Haller, D. (2011). Metabolic phenotyping of the Crohn’s disease-like IBD etiopathology in the TNF(DeltaARE/WT) mouse model. J. Proteome Res. 10, 5523–5535.
Beckonert, O., Coen, M., Keun, H. C., Wang, Y., Ebbels, T. M., Holmes, E., Lindon, J. C., and Nicholson, J. K. (2010). High-resolution magic-angle-spinning NMR spectroscopy for metabolic profiling of intact tissues. Nat. Protoc. 5, 1019–1032.
Bertini, I., Calabro, A., De, C. V., Luchinat, C., Nepi, S., Porfirio, B., Renzi, D., Saccenti, E., and Tenori, L. (2009). The metabonomic signature of celiac disease. J. Proteome Res. 8, 170–177.
Bezabeh, T., Somorjai, R. L., Smith, I. C., Nikulin, A. E., Dolenko, B., and Bernstein, C. N. (2001). The use of 1H magnetic resonance spectroscopy in inflammatory bowel diseases: distinguishing ulcerative colitis from Crohn’s disease. Am. J. Gastroenterol. 96, 442–448.
Bi, X., Lin, Q., Foo, T. W., Joshi, S., You, T., Shen, H. M., Ong, C. N., Cheah, P. Y., Eu, K. W., and Hew, C. L. (2006). Proteomic analysis of colorectal cancer reveals alterations in metabolic pathways: mechanism of tumorigenesis. Mol. Cell Proteomics 5, 1119–1130.
Bickston, S. J., Comerford, L. W., and Cominelli, F. (2003). Future therapies for inflammatory bowel disease. Curr. Gastroenterol. Rep. 5, 518–523.
Bjerrum, J. T., Nielsen, O. H., Hao, F., Tang, H., Nicholson, J. K., Wang, Y., and Olsen, J. (2010). Metabonomics in ulcerative colitis: diagnostics, biomarker identification, and insight into the pathophysiology. J. Proteome Res. 9, 954–962.
Bruce, S. J., Tavazzi, I., Parisod, V., Rezzi, S., Kochhar, S., and Guy, P. A. (2009). Investigation of human blood plasma sample preparation for performing metabolomics using ultrahigh performance liquid chromatography/mass spectrometry. Anal. Chem. 81, 3285–3296.
Chan, E. C., Koh, P. K., Mal, M., Cheah, P. Y., Eu, K. W., Backshall, A., Cavill, R., Nicholson, J. K., and Keun, H. C. (2009). Metabolic profiling of human colorectal cancer using high-resolution magic angle spinning nuclear magnetic resonance (HR-MAS NMR) spectroscopy and gas chromatography mass spectrometry (GC/MS). J. Proteome Res. 8, 352–361.
Claus, S. P., Ellero, S. L., Berger, B., Krause, L., Bruttin, A., Molina, J., Paris, A., Want, E. J., de Waziers, I., Cloarec, O., Richards, S. E., Wang, Y., Dumas, M. E., Ross, A., Rezzi, S., Kochhar, S., van, B. P., Lindon, J. C., Holmes, E., and Nicholson, J. K. (2011). Colonization-induced host-gut microbial metabolic interaction. MBio 2, e00271–10.
Clayton, T. A., Baker, D., Lindon, J. C., Everett, J. R., and Nicholson, J. K. (2009). Pharmacometabonomic identification of a significant host-microbiome metabolic interaction affecting human drug metabolism. Proc. Natl. Acad. Sci. U.S.A. 106, 14728–14733.
Collins, M. D., and Gibson, G. R. (1999). Probiotics, prebiotics, and synbiotics: approaches for modulating the microbial ecology of the gut. Am. J. Clin. Nutr. 69, 1052S–1057S.
Cummings, J. H., Pomare, E. W., Branch, W. J., Naylor, C. P., and Macfarlane, G. T. (1987). Short chain fatty acids in human large intestine, portal, hepatic and venous blood. Gut 28, 1221–1227.
Cunningham, D., Atkin, W., Lenz, H. J., Lynch, H. T., Minsky, B., Nordlinger, B., and Starling, N. (2010). Colorectal cancer. Lancet 375, 1030–1047.
Dethlefsen, L., Fall-Ngai, M., and Relman, D. A. (2007). An ecological and evolutionary perspective on human-microbe mutualism and disease. Nature 449, 811–818.
Dumas, M. E., Barton, R. H., Toye, A., Cloarec, O., Blancher, C., Rothwell, A., Fearnside, J., Tatoud, R., Blanc, V., Lindon, J. C., Mitchell, S. C., Holmes, E., McCarthy, M. I., Scott, J., Gauguier, D., and Nicholson, J. K. (2006). Metabolic profiling reveals a contribution of gut microbiota to fatty liver phenotype in insulin-resistant mice. Proc. Natl. Acad. Sci. U.S.A. 103, 12511–12516.
Duncan, M., Davison, J. S., and Sharkey, K. A. (2005). Review article: endocannabinoids and their receptors in the enteric nervous system. Aliment. Pharmacol. Ther. 22, 667–683.
Dunne, C. (2001). Adaptation of bacteria to the intestinal niche: probiotics and gut disorder. Inflamm. Bowel Dis. 7, 136–145.
Ejsing, C. S., Sampaio, J. L., Surendranath, V., Duchoslav, E., Ekroos, K., Klemm, R. W., Simons, K., and Shevchenko, A. (2009). Global analysis of the yeast lipidome by quantitative shotgun mass spectrometry. Proc. Natl. Acad. Sci. U.S.A. 106, 2136–2141.
Eriksson, L., Antti, H., Gottfries, J., Holmes, E., Johansson, E., Lindgren, F., Long, I., Lundstedt, T., Trygg, J., and Wold, S. (2004). Using chemometrics for navigating in the large data sets of genomics, proteomics, and metabonomics (gpm). Anal. Bioanal. Chem. 380, 419–429.
Fabbrini, E., Sullivan, S., and Klein, S. (2010). Obesity and nonalcoholic fatty liver disease: biochemical, metabolic, and clinical implications. Hepatology 51, 679–689.
Falk, P. G., Hooper, L. V., Midtvedt, T., and Gordon, J. I. (1998). Creating and maintaining the gastrointestinal ecosystem: what we know and need to know from gnotobiology. Microbiol. Mol. Biol. Rev. 62, 1157–1170.
Gibson, G. R., and Roberfroid, M. B. (1995). Dietary modulation of the human colonic microbiota: introducing the concept of prebiotics. J. Nutr. 125, 1401–1412.
Gill, S. R., Pop, M., Deboy, R. T., Eckburg, P. B., Turnbaugh, P. J., Samuel, B. S., Gordon, J. I., Relman, D. A., Fraser-Liggett, C. M., and Nelson, K. E. (2006). Metagenomic analysis of the human distal gut microbiome. Science 312, 1355–1359.
Gilmore, M. S., and Ferretti, J. J. (2003). The thin line between gut commensal and pathogen. Science 299, 1999–2002.
Goldberg, R. M. (2005). Advances in the treatment of metastatic colorectal cancer. Oncologist 10(Suppl. 3), 40–48.
Guigoz, Y., Dore, J., and Schiffrin, E. J. (2008). The inflammatory status of old age can be nurtured from the intestinal environment. Curr. Opin. Clin. Nutr. Metab. Care 11, 13–20.
Gupta, P., Andrew, H., Kirschner, B. S., and Guandalini, S. (2000). Is Lactobacillus GG helpful in children with Crohn’s disease? Results of a preliminary, open-label study. J. Pediatr. Gastroenterol. Nutr. 31, 453–457.
Haller, D. (2006). Intestinal epithelial cell signalling and host-derived negative regulators under chronic inflammation: to be or not to be activated determines the balance towards commensal bacteria. Neurogastroenterol. Motil. 18, 184–199.
Harper, P. H., Truelove, S. C., Lee, E. C., Kettlewell, M. G., and Jewell, D. P. (1983). Split ileostomy and ileocolostomy for Crohn’s disease of the colon and ulcerative colitis: a 20 year survey. Gut 24, 106–113.
Hong, Y. S., Ahn, Y. T., Park, J. C., Lee, J. H., Lee, H., Huh, C. S., Kim, D. H., Ryu do, H., and Hwang, G. S. (2010). 1H NMR-based metabonomic assessment of probiotic effects in a colitis mouse model. Arch. Pharm. Res. 33, 1091–1101.
Hooper, L. V., Midtvedt, T., and Gordon, J. I. (2002). How host-microbial interactions shape the nutrient environment of the mammalian intestine. Annu. Rev. Nutr. 22, 283–307.
Ikeda, A., Nishiumi, S., Shinohara, M., Yoshie, T., Hatano, N., Okuno, T., Bamba, T., Fukusaki, E., Takenawa, T., Azuma, T., and Yoshida, M. (2011). Serum metabolomics as a novel diagnostic approach for gastrointestinal cancer. Biomed. Chromatogr. 26, 548–558.
Jansson, J., Willing, B., Lucio, M., Fekete, A., Dicksved, J., Halfvarson, J., Tysk, C., and Schmitt-Kopplin, P. (2009). Metabolomics reveals metabolic biomarkers of Crohn’s disease. PLoS ONE 4, e6386. doi: 10.1371/journal.pone.0006386
Jia, W., Li, H., Zhao, L., and Nicholson, J. K. (2008). Gut microbiota: a potential new territory for drug targeting. Nat. Rev. Drug Discov. 7, 123–129.
Johnson, N. A., Walton, D. W., Sachinwalla, T., Thompson, C. H., Smith, K., Ruell, P. A., Stannard, S. R., and George, J. (2008). Noninvasive assessment of hepatic lipid composition: advancing understanding and management of fatty liver disorders. Hepatology 47, 1513–1523.
Korelitz, B. I., Cheskin, L. J., Sohn, N., and Sommers, S. C. (1985). The fate of the rectal segment after diversion of the fecal stream in Crohn’s disease: its implications for surgical management. J. Clin. Gastroenterol. 7, 37–43.
Le Gall, G., Noor, S. O., Ridgway, K., Scovell, L., Jamieson, C., Johnson, I. T., Colquhoun, I. J., Kemsley, E. K., and Narbad, A. (2011). Metabolomics of fecal extracts detects altered metabolic activity of gut microbiota in ulcerative colitis and irritable bowel syndrome. J. Proteome Res. 10, 4208–4218.
Le Leu, R. K., Brown, I. L., Hu, Y., Bird, A. R., Jackson, M., Esterman, A., and Young, G. P. (2005). A synbiotic combination of resistant starch and Bifidobacterium lactis facilitates apoptotic deletion of carcinogen-damaged cells in rat colon. J. Nutr. 135, 996–1001.
Ley, R., Turnbaugh, P., Klein, S., and Gordon, J. (2006). Microbial ecology: human gut microbes associated with obesity. Nature 444, 1022–1023.
Ley, R. E., Hamady, M., Lozupone, C., Turnbaugh, P. J., Ramey, R. R., Bircher, J. S., Schlegel, M. L., Tucker, T. A., Schrenzel, M. D., Knight, R., and Gordon, J. I. (2008). Evolution of mammals and their gut microbes. Science 320, 1647–1651.
Li, J. V., Ashrafian, H., Bueter, M., Kinross, J., Sands, C., le Roux, C. W., Bloom, S. R., Darzi, A., Athanasiou, T., Marchesi, J. R., Nicholson, J. K., and Holmes, E. (2011a). Metabolic surgery profoundly influences gut microbial-host metabolic cross-talk. Gut 60, 1214–1223.
Li, J. V., Reshat, R., Wu, Q., Ashrafian, H., Bueter, M., le Roux, C. W., Darzi, A., Athanasiou, T., Marchesi, J. R., Nicholson, J. K., Holmes, E., and Gooderham, N. J. (2011b). Experimental bariatric surgery in rats generates a cytotoxic chemical environment in the gut contents. Front. Microbiol. 2:183. doi: 10.3389/fmicb.2011.00183
Lin, H. M., Barnett, M. P., Roy, N. C., Joyce, N. I., Zhu, S., Armstrong, K., Helsby, N. A., Ferguson, L. R., and Rowan, D. D. (2010). Metabolomic analysis identifies inflammatory and noninflammatory metabolic effects of genetic modification in a mouse model of Crohn’s disease. J. Proteome Res. 9, 1965–1975.
Lin, H. M., Helsby, N. A., Rowan, D. D., and Ferguson, L. R. (2011). Using metabolomic analysis to understand inflammatory bowel diseases. Inflamm. Bowel Dis. 17, 1021–1029.
Loftus, E. V. Jr. (2004). Clinical epidemiology of inflammatory bowel disease: incidence, prevalence, and environmental influences. Gastroenterology 126, 1504–1517.
Madden, J. A., and Hunter, J. O. (2002). A review of the role of the gut microflora in irritable bowel syndrome and the effects of probiotics. Br. J. Nutr. 88(Suppl. 1), S67–S72.
Malinen, E., Rinttila, T., Kajander, K., Matto, J., Kassinen, A., Krogius, L., Saarela, M., Korpela, R., and Palva, A. (2005). Analysis of the fecal microbiota of irritable bowel syndrome patients and healthy controls with real-time PCR. Am. J. Gastroenterol. 100, 373–382.
Marchesi, J. R., Holmes, E., Khan, F., Kochhar, S., Scanlan, P., Shanahan, F., Wilson, I. D., and Wang, Y. (2007). Rapid and noninvasive metabonomic characterization of inflammatory bowel disease. J. Proteome Res. 6, 546–551.
Martin, F. P., Dumas, M. E., Wang, Y., Legido-Quigley, C., Yap, I. K., Tang, H., Zirah, S., Murphy, G. M., Cloarec, O., Lindon, J. C., Sprenger, N., Fay, L. B., Kochhar, S., van, B. P., Holmes, E., and Nicholson, J. K. (2007a). A top-down systems biology view of microbiome-mammalian metabolic interactions in a mouse model. Mol. Syst. Biol. 3, 112.
Martin, F. P., Wang, Y., Sprenger, N., Holmes, E., Lindon, J. C., Kochhar, S., and Nicholson, J. K. (2007b). Effects of probiotic Lactobacillus paracasei treatment on the host gut tissue metabolic profiles probed via magic-angle-spinning NMR spectroscopy. J. Proteome Res. 6, 1471–1481.
Martin, F. P., Sprenger, N., Montoliu, I., Rezzi, S., Kochhar, S., and Nicholson, J. K. (2010a). Dietary modulation of gut functional ecology studied by fecal metabonomics. J. Proteome Res. 9, 5284–5295.
Martin, F. P., Montoliu, I., Kochhar, S., and Rezzi, S. (2010b). Chemometric strategy for modeling metabolic biological space along the gastrointestinal tract and assessing microbial influences. Anal. Chem. 82, 9803–9811.
Martin, F. P., Sprenger, N., Yap, I. K., Wang, Y., Bibiloni, R., Rochat, F., Rezzi, S., Cherbut, C., Kochhar, S., Lindon, J. C., Holmes, E., and Nicholson, J. K. (2009a). Panorganismal gut microbiome-host metabolic crosstalk. J. Proteome Res. 8, 2090–2105.
Martin, F. P., Wang, Y., Yap, I. K., Sprenger, N., Lindon, J. C., Rezzi, S., Kochhar, S., Holmes, E., and Nicholson, J. K. (2009b). Topographical variation in murine intestinal metabolic profiles in relation to microbiome speciation and functional ecological activity. J. Proteome Res. 8, 3464–3474.
Martin, F. P., Rezzi, S., Philippe, D., Tornier, L., Messlik, A., Holzlwimmer, G., Baur, P., Quintanilla-Fend, L., Loh, G., Blaut, M., Blum, S., Kochhar, S., and Haller, D. (2009c). Metabolic assessment of gradual development of moderate experimental colitis in IL-10 deficient mice. J. Proteome Res. 8, 2376–2387.
Martin, F. P., Verdu, E. F., Wang, Y., Dumas, M. E., Yap, I. K., Cloarec, O., Bergonzelli, G. E., Corthesy-Theulaz, I., Kochhar, S., Holmes, E., Lindon, J. C., Collins, S. M., and Nicholson, J. K. (2006). Transgenomic metabolic interactions in a mouse disease model: interactions of Trichinella spiralis infection with dietary Lactobacillus paracasei supplementation. J. Proteome Res. 5, 2185–2193.
Martin, F.-P. J., Wang, Y., Sprenger, N., Yap, I. K. S., Rezzi, S., Ramadan, Z., Pere-Trepat, E., Rochat, F., Cherbut, C., van Bladeren, P., Fay, L. B., Kochhar, S., Lindon, J. C., Holmes, E., and Nicholson, J. K. (2008a). Top-down systems biology integration of conditional prebiotic transgenomic interactions in a humanized microbiome mouse model. Mol. Syst. Biol. 4, 205.
Martin, F. P., Wang, Y., Sprenger, N., Yap, I. K., Lundstedt, T., Lek, P., Rezzi, S., Ramadan, Z., van, B. P., Fay, L. B., Kochhar, S., Lindon, J. C., Holmes, E., and Nicholson, J. K. (2008b). Probiotic modulation of symbiotic gut microbial-host metabolic interactions in a humanized microbiome mouse model. Mol. Syst. Biol. 4, 157.
Matto, J., Maunuksela, L., Kajander, K., Palva, A., Korpela, R., Kassinen, A., and Saarela, M. (2005). Composition and temporal stability of gastrointestinal microbiota in irritable bowel syndrome – a longitudinal study in IBS and control subjects. FEMS Immunol. Med. Microbiol. 43, 213–222.
Merrifield, C. A., Lewis, M., Claus, S. P., Beckonert, O. P., Dumas, M. E., Duncker, S., Kochhar, S., Rezzi, S., Lindon, J. C., Bailey, M., Holmes, E., and Nicholson, J. K. (2011). A metabolic system-wide characterisation of the pig: a model for human physiology. Mol. Biosyst. 7, 2577–2588.
Mestdagh, R., Dumas, M. E., Rezzi, S., Kochhar, S., Holmes, E., Claus, S. P., and Nicholson, J. K. (2011). Gut microbiota modulate the metabolism of brown adipose tissue. J. Proteome Res. 11, 620–630.
Miyagi, Y., Higashiyama, M., Gochi, A., Akaike, M., Ishikawa, T., Miura, T., Saruki, N., Bando, E., Kimura, H., Imamura, F., Moriyama, M., Ikeda, I., Chiba, A., Oshita, F., Imaizumi, A., Yamamoto, H., Miyano, H., Horimoto, K., Tochikubo, O., Mitsushima, T., Yamakado, M., and Okamoto, N. (2011). Plasma free amino acid profiling of five types of cancer patients and its application for early detection. PLoS ONE 6, e24143. doi: 10.1371/journal.pone.0024143
Monleon, D., Morales, J. M., Barrasa, A., Lopez, J. A., Vazquez, C., and Celda, B. (2009). Metabolite profiling of fecal water extracts from human colorectal cancer. NMR Biomed. 22, 342–348.
Montoliu, I., Martin, F. P., Collino, S., Rezzi, S., and Kochhar, S. (2009). Multivariate modeling strategy for intercompartmental analysis of tissue and plasma 1H NMR spectrotypes. J. Proteome Res. 8, 2397–2406.
Moreno, A., Rey, M., Montane, J. M., Alonso, J., and Arus, C. (1993). 1H NMR spectroscopy of colon tumors and normal mucosal biopsies; elevated taurine levels and reduced polyethyleneglycol absorption in tumors may have diagnostic significance. NMR Biomed. 6, 111–118.
Murdoch, T. B., Fu, H., Macfarlane, S., Sydora, B. C., Fedorak, R. N., and Slupsky, C. M. (2008). Urinary metabolic profiles of inflammatory bowel disease in interleukin-10 gene-deficient mice. Anal. Chem. 80, 5524–5531.
Newgard, C. B., An, J., Bain, J. R., Muehlbauer, M. J., Stevens, R. D., Lien, L. F., Haqq, A. M., Shah, S. H., Arlotto, M., Slentz, C. A., Rochon, J., Gallup, D., Ilkayeva, O., Wenner, B. R., Yancy, W. S. Jr., Eisenson, H., Musante, G., Surwit, R. S., Millington, D. S., Butler, M. D., and Svetkey, L. P. (2009). A branched-chain amino acid-related metabolic signature that differentiates obese and lean humans and contributes to insulin resistance. Cell Metab. 9, 311–326.
Nicholson, J. K., Holmes, E., and Wilson, I. D. (2005). Gut microorganisms, mammalian metabolism and personalized health care. Nat. Rev. Microbiol. 3, 431–438.
O’Keefe, S. J. (2008). Nutrition and colonic health: the critical role of the microbiota. Curr. Opin. Gastroenterol. 24, 51–58.
Otter, D., Cao, M., Lin, H. M., Fraser, K., Edmunds, S., Lane, G., and Rowan, D. (2011). Identification of urinary biomarkers of colon inflammation in IL10-/- mice using Short-Column LCMS metabolomics. J. Biomed. Biotechnol. 2011, 974701.
Pietilainen, K. H., Sysi-Aho, M., Rissanen, A., Seppanen-Laakso, T., Yki-Jarvinen, H., Kaprio, J., and Oresic, M. (2007). Acquired obesity is associated with changes in the serum lipidomic profile independent of genetic effects – a monozygotic twin study. PLoS ONE 2, e218. doi: 10.1371/journal.pone.0000218
Ponnusamy, K., Choi, J. N., Kim, J., Lee, S. Y., and Lee, C. H. (2011). Microbial community and metabolomic comparison of irritable bowel syndrome faeces. J. Med. Microbiol. 60(Pt 6), 817–827.
Qin, J., Li, R., Raes, J., Arumugam, M., Burgdorf, K. S., Manichanh, C., Nielsen, T., Pons, N., Levenez, F., Yamada, T., Mende, D. R., Li, J., Xu, J., Li, S., Li, D., Cao, J., Wang, B., Liang, H., Zheng, H., Xie, Y., Tap, J., Lepage, P., Bertalan, M., Batto, J. M., Hansen, T., Le, P. D., Linneberg, A., Nielsen, H. B., Pelletier, E., Renault, P., Sicheritz-Ponten, T., Turner, K., Zhu, H., Yu, C., Li, S., Jian, M., Zhou, Y., Li, Y., Zhang, X., Li, S., Qin, N., Yang, H., Wang, J., Brunak, S., Dore, J., Guarner, F., Kristiansen, K., Pedersen, O., Parkhill, J., Weissenbach, J., Bork, P., Ehrlich, S. D., and Wang, J. (2010). A human gut microbial gene catalogue established by metagenomic sequencing. Nature 464, 59–65.
Reid, G., Jass, J., Sebulsky, M. T., and McCormick, J. K. (2003). Potential uses of probiotics in clinical practice. Clin. Microbiol. Rev. 16, 658–672.
Ritchie, S. A., Ahiahonu, P. W., Jayasinghe, D., Heath, D., Liu, J., Lu, Y., Jin, W., Kavianpour, A., Yamazaki, Y., Khan, A. M., Hossain, M., Su-Myat, K. K., Wood, P. L., Krenitsky, K., Takemasa, I., Miyake, M., Sekimoto, M., Monden, M., Matsubara, H., Nomura, F., and Goodenowe, D. B. (2010a). Reduced levels of hydroxylated, polyunsaturated ultra long-chain fatty acids in the serum of colorectal cancer patients: implications for early screening and detection. BMC Med. 8, 13. doi: 10.1186/1741-7015-8-13
Ritchie, S. A., Heath, D., Yamazaki, Y., Grimmalt, B., Kavianpour, A., Krenitsky, K., Elshoni, H., Takemasa, I., Miyake, M., Sekimoto, M., Monden, M., Tomonaga, T., Matsubara, H., Sogawa, K., Matsushita, K., Nomura, F., and Goodenowe, D. B. (2010b). Reduction of novel circulating long-chain fatty acids in colorectal cancer patients is independent of tumor burden and correlates with age. BMC Gastroenterol. 10, 140. doi: 10.1186/1471-230X-10-140
Sartor, R. B. (2004). Therapeutic manipulation of the enteric microflora in inflammatory bowel diseases: antibiotics, probiotics, and prebiotics. Gastroenterology 126, 1620–1633.
Schicho, R., Nazyrova, A., Shaykhutdinov, R., Duggan, G., Vogel, H. J., and Storr, M. (2010). Quantitative metabolomic profiling of serum and urine in DSS-induced ulcerative colitis of mice by (1)H NMR spectroscopy. J. Proteome Res. 9, 6265–6273.
Schrezenmeir, J., and de Vrese, M. (2001). Probiotics, prebiotics, and synbiotics – approaching a definition. Am. J. Clin. Nutr. 73(Suppl. 2), 361S–364S.
Shahani, K. M., and Ayebo, A. D. (1980). Role of dietary lactobacilli in gastrointestinal microecology. Am. J. Clin. Nutr. 33(Suppl. 11), 2448–2457.
Shanahan, F. (2001). Inflammatory bowel disease: immunodiagnostics, immunotherapeutics, and ecotherapeutics. Gastroenterology 120, 622–635.
Sharma, U., Singh, R. R., Ahuja, V., Jagannathan, N. R., and Makharia, G. K. (2010). Similarity in the metabolic profile in macroscopically involved and un-involved colonic mucosa in patients with inflammatory bowel disease: an in vitro proton ((1)H) MR spectroscopy study. Magn. Reson. Imaging 28, 1022–1029.
Si, J. M., Yu, Y. C., Fan, Y. J., and Chen, S. J. (2004). Intestinal microecology and quality of life in irritable bowel syndrome patients. World J. Gastroenterol. 10, 1802–1805.
Siemanowski, B., and Regueiro, M. (2007). Efficacy of infliximab for extraintestinal manifestations of inflammatory bowel disease. Curr. Treat. Options Gastroenterol. 10, 178–184.
Song, H., Wang, L., Liu, H. L., Wu, X. B., Wang, H. S., Liu, Z. H., Li, Y., Diao, D. C., Chen, H. L., and Peng, J. S. (2011a). Tissue metabolomic fingerprinting reveals metabolic disorders associated with human gastric cancer morbidity. Oncol. Rep. 26, 431–438.
Song, H., Peng, J. S., Dong-Sheng, Y., Yang, Z. L., Liu, H. L., Zeng, Y. K., Shi, X. P., and Lu, B. Y. (2011b). Serum metabolic profiling of human gastric cancer based on gas chromatography/mass spectrometry. Braz. J. Med. Biol. Res. 45, 78–85.
Suhre, K., Meisinger, C., Doring, A., Altmaier, E., Belcredi, P., Gieger, C., Chang, D., Milburn, M. V., Gall, W. E., Weinberger, K. M., Mewes, H. W., Hrabe de, A. M., Wichmann, H. E., Kronenberg, F., Adamski, J., and Illig, T. (2010). Metabolic footprint of diabetes: a multiplatform metabolomics study in an epidemiological setting. PLoS ONE 5, e13953. doi: 10.1371/journal.pone.0013953
Swann, J. R., Want, E. J., Geier, F. M., Spagou, K., Wilson, I. D., Sidaway, J. E., Nicholson, J. K., and Holmes, E. (2011). Systemic gut microbial modulation of bile acid metabolism in host tissue compartments. Proc. Natl. Acad. Sci. U.S.A. 108(Suppl. 1), 4523–4530.
Swidsinski, A., Ladhoff, A., Pernthaler, A., Swidsinski, S., Loening-Baucke, V., Ortner, M., Weber, J., Hoffmann, U., Schreiber, S., Dietel, M., and Lochs, H. (2002). Mucosal flora in inflammatory bowel disease. Gastroenterology 122, 44–54.
Tannock, G. W. (2005). New perceptions of the gut microbiota: implications for future research. Gastroenterol. Clin. North Am. 34, 361–382.
Tjellstrom, B., Stenhammar, L., Hogberg, L., Falth-Magnusson, K., Magnusson, K. E., Midtvedt, T., Sundqvist, T., and Norin, E. (2005). Gut microflora associated characteristics in children with celiac disease. Am. J. Gastroenterol. 100, 2784–2788.
Turnbaugh, P., Ley, R., Mahowald, M., Magrini, V., Mardis, E., and Gordon, J. (2006). An obesity-associated gut microbiome with increased capacity for energy harvest. Nature 444, 1027–1031.
Verdu, E. F., Bercik, P., Bergonzelli, G. E., Huang, X. X., Blennerhasset, P., Rochat, F., Fiaux, M., Mansourian, R., Corthesy-Theulaz, I., and Collins, S. M. (2004). Lactobacillus paracasei normalizes muscle hypercontractility in a murine model of postinfective gut dysfunction. Gastroenterology 127, 826–837.
Wang, Y., Holmes, E., Comelli, E. M., Fotopoulos, G., Dorta, G., Tang, H., Rantalainen, M. J., Lindon, J. C., Corthesy-Theulaz, I. E., Fay, L. B., Kochhar, S., and Nicholson, J. K. (2007). Topographical variation in metabolic signatures of human gastrointestinal biopsies revealed by high-resolution magic-angle spinning (1)H NMR spectroscopy. J. Proteome Res. 6, 3944–3951.
Wang, Y., Tang, H., Holmes, E., Lindon, J. C., Turini, M. E., Sprenger, N., Bergonzelli, G., Fay, L. B., Kochhar, S., and Nicholson, J. K. (2005). Biochemical characterization of rat intestine development using high-resolution magic-angle-spinning 1H NMR spectroscopy and multivariate data analysis. J. Proteome Res. 4, 1324–1329.
Wang, Z., Klipfell, E., Bennett, B. J., Koeth, R., Levison, B. S., Dugar, B., Feldstein, A. E., Britt, E. B., Fu, X., Chung, Y. M., Wu, Y., Schauer, P., Smith, J. D., Allayee, H., Tang, W. H., DiDonato, J. A., Lusis, A. J., and Hazen, S. L. (2011). Gut flora metabolism of phosphatidylcholine promotes cardiovascular disease. Nature 472, 57–63.
Wikoff, W. R., Anfora, A. T., Liu, J., Schultz, P. G., Lesley, S. A., Peters, E. C., and Siuzdak, G. (2009). Metabolomics analysis reveals large effects of gut microflora on mammalian blood metabolites. Proc. Natl. Acad. Sci. U.S.A. 106, 3698–3703.
Williams, H. R., Cox, I. J., Walker, D. G., North, B. V., Patel, V. M., Marshall, S. E., Jewell, D. P., Ghosh, S., Thomas, H. J., Teare, J. P., Jakobovits, S., Zeki, S., Welsh, K. I., Taylor-Robinson, S. D., and Orchard, T. R. (2009). Characterization of inflammatory bowel disease with urinary metabolic profiling. Am. J. Gastroenterol. 104, 1435–1444.
Winterkamp, S., Weidenhiller, M., Otte, P., Stolper, J., Schwab, D., Hahn, E. G., and Raithel, M. (2002). Urinary excretion of N-methylhistamine as a marker of disease activity in inflammatory bowel disease. Am. J. Gastroenterol. 97, 3071–3077.
Xu, J., Bjursell, M. K., Himrod, J., Deng, S., Carmichael, L. K., Chaing, H. C., Hooper, L. V., and Gordon, J. I. (2003). A genomic view of the human-Bacteroides thetaiotaomicron symbiosis. Science 299, 2074–2076.
Xu, J., and Gordon, J. I. (2003). Inaugural article: honor thy symbionts. Proc. Natl. Acad. Sci. U.S.A. 100, 10452–10459.
Xu, J., Mahowald, M. A., Ley, R. E., Lozupone, C. A., Hamady, M., Martens, E. C., Henrissat, B., Coutinho, P. M., Minx, P., Latreille, P., Cordum, H., Van, B. A., Kim, K., Fulton, R. S., Fulton, L. A., Clifton, S. W., Wilson, R. K., Knight, R. D., and Gordon, J. I. (2007). Evolution of symbiotic bacteria in the distal human intestine. PLoS Biol. 5, e156. doi: 10.1371/journal.pbio.0050156
Zhao, X., Fritsche, J., Wang, J., Chen, J., Rittig, K., Schmitt-Kopplin, P., Fritsche, A., Haring, H. U., Schleicher, E. D., Xu, G., and Lehmann, R. (2010). Metabonomic fingerprints of fasting plasma and spot urine reveal human pre-diabetic metabolic traits. Metabolomics 6, 362–374.
Keywords: gastrointestinal, gut microbiota, nutritional metabolomics, metabotypes, personalized nutrition
Citation: Martin F-PJ, Collino S, Rezzi S and Kochhar S (2012) Metabolomic applications to decipher gut microbial metabolic influence in health and disease. Front. Physio. 3:113. doi: 10.3389/fphys.2012.00113
Received: 13 December 2011; Accepted: 05 April 2012;
Published online: 26 April 2012.
Edited by:
Stephen J. Pandol, University of California Los Angeles, USAReviewed by:
Dwaipayan Bharadwaj, Institute of Genomics and Integrative Biology, IndiaHongxiang Hui, Southern Medical University, China
Copyright: © 2012 Martin, Collino, Rezzi and Kochhar. This is an open-access article distributed under the terms of the Creative Commons Attribution Non Commercial License, which permits non-commercial use, distribution, and reproduction in other forums, provided the original authors and source are credited.
*Correspondence: Sunil Kochhar, Metabolomics and Biomarkers, Department of BioAnalytial sciences, Nestlé Research Center, Nestec Ltd., Vers-chez-les-Blanc, CH-1000 Lausanne 26, Switzerland. e-mail: sunil.kochhar@rdls.nestle.com