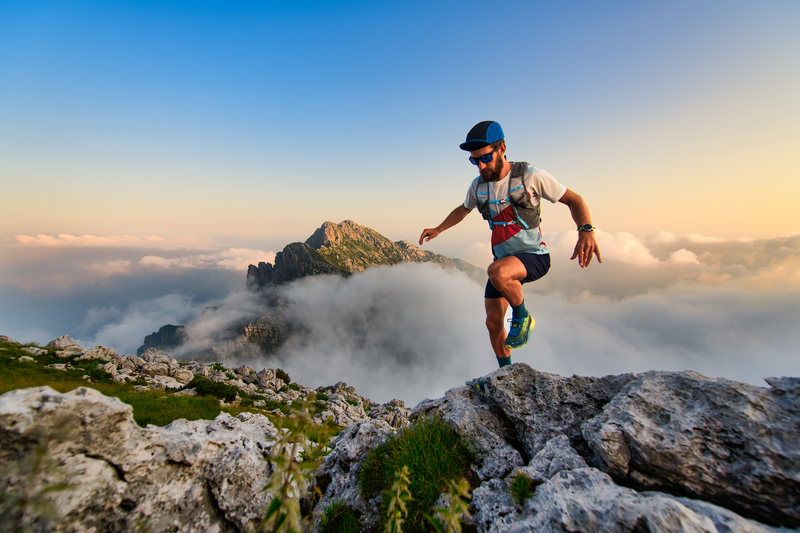
95% of researchers rate our articles as excellent or good
Learn more about the work of our research integrity team to safeguard the quality of each article we publish.
Find out more
METHODS article
Front. Physiol. , 10 April 2012
Sec. Integrative Physiology
Volume 3 - 2012 | https://doi.org/10.3389/fphys.2012.00061
This article is part of the Research Topic Autonomic dysfunction in heart failure and renal disease View all 7 articles
The immature autonomic nervous system (ANS) in premature infants regulates heart rate (HR) and respiration different during quiet sleep (QS) and active sleep (AS). Little information is available about ANS regulation in these subjects. The aim of this study was to investigate changes in autonomic regulation and cardiorespiratory coupling during AS and QS in five very preterm neonates with gestational age (GA) 26–31 weeks, applying univariate and bivariate linear and non-linear dynamics methods to the recorded cardiorespiratory signals. During QS univariate linear indices revealed lower standard deviations and entropies, indicating decreased heart rate (HR) variability. More balanced sympatho-vagal behavior of the ANS was revealed by decreased low frequency (LF), increased high frequency (HF), and a trend toward lower ratio LF/HF in QS. Applied non-linear indices (probabilities, entropies, and fractal measures) quantifying the complexity and scaling behavior of HR regulation processes were significantly altered in QS in comparison to AS. This reflects a lower short-term variability, less complexity, and a loss of fractal-like correlation properties of HR dynamics in QS. One major finding is that cardiorespiratory coupling is not yet completely developed in very preterm neonates with 26–31 weeks GA. Significantly different regulation patterns in bivariate oscillations of HR and respiration during AS and QS could be recognized. These patterns were characterized on the one hand by predominant monotonous regulating sequences originating from respiration independently from HR time series in AS, and to a minor degree in QS, and on the other hand by some prominent HR regulation sequences in QS independent of respiratory regulation. We speculate that these findings might be suitable for monitoring preterm neonates and for detecting disorders in the developing cardiorespiratory system.
The first postnatal period for preterm infants is characterized by periods of active sleep (AS) and quiet sleep (QS) states, with intermediate undetermined sleep phases. Preterm newborns have an immature autonomic nervous system (ANS) regulating brain and metabolic activity, heart rate (HR), respiration, blood pressure, and body temperature. Initially, the newborn uses primitive brainstem-visceral circuits via ingestive behaviors as the primary mechanism to regulate physiological state (bodily functions; Porges and Furman, 2011). However, in addition to progressive vagal maturation, cortical regulation of the brainstem develops during the first year of life (Longin et al., 2006). In QS, a decrease of HR variability (HRV) accompanied by less chaotic HR fluctuations has been investigated by applying linear and non-linear methods in healthy full-term and diseased preterm neonates (Andriessen et al., 2005; Doyle et al., 2009; Vandeput et al., 2009; Krueger et al., 2010).
Although cardiorespiratory coupling is weak at the earliest stages of human development, studies conducted in late preterm infants confirmed their presence and integrity (Indic et al., 2011). However, specifically targeted standards and recommended methods to assess cardiorespiratory functions in this rather early stage of life are still required. For example, standard definitions of frequency bands for HRV analysis [low and high frequency, (LF, HF)] that already exist for adults (Task Force, 1996) have not yet been established for infants. Multivariate autoregressive analysis has revealed higher coherence values in the ultra HF band (0.7–1.5 Hz) in late preterm infants. This suggests significantly stronger coupling in the frequency range associated with regular breathing, indicating a mild, but present, respiratory sinus arrhythmia (RSA) (Indic et al., 2008). In an immature system, both the cardiovascular and respiratory mechanisms may exhibit transient synchronizations, but due to the system’s vulnerability, the couplings are fast changing, not continuous, and not persistent. It has been shown that sleep states and HR regulation have a predominant influence on respiratory variability in non-healthy preterm infants, such that the ventilatory frequency and its variability are greater in AS (Elder et al., 2010, 2011) than in QS. Furthermore, in diseased preterm newborns, HR and respiration are more intermittently coupled in QS than in AS (Elder et al., 2010). In healthy full-term neonates higher HR complexity and cardiorespiratory coupling could be observed in QS on the basis of higher coherence and cross-correlation maxima (Frasch et al., 2007). Thus, cardiorespiratory coupling is less complex in QS in the HF (0.2–2 Hz) band only and shows different, probably sympathetically mediated, oscillations influencing the HR and respiration regulation.
The aim of this study was to investigate if typical cardiorespiratory regulation patterns and coupling already exist in very preterm neonates with a gestational age (GA) of 26–31 weeks. Different sleep states (AS and QS) were investigated to quantify regulation patterns of the ANS in these different stages. Autonomic regulation was characterized by separately analyzing cardiac (HRV) and respiratory signals and their interactions (coupling) with linear and non-linear methods. Coupling analyzes presuppose non-linear methods because of the non-stationary behavior of the underlying control systems and the high complexity of the recorded signals (Hoyer et al., 1998). Therefore, we applied non-linear methods, which are more appropriate to investigate the complex behavior of the autonomous cardiorespiratory system than conventional linear methods (Voss et al., 2009). For cardiovascular and cardiorespiratory coupling analyses we selected the joint symbolic dynamics (JSD) method that has been successfully applied in some clinical studies and contributed to a better understanding of autonomous regulation, leading to improved risk stratification in diseased patients (Baumert et al., 2002; Voss et al., 2006; Caminal et al., 2010; Schulz et al., 2010).
Findings from this study could be useful in pediatrics for monitoring the cardiorespiratory system in preterm infants to recognize normal or abnormal changes in autonomic regulation at an early stage of life.
In this study five very preterm neonates (conceptual age 30.0 weeks, 27–32; birth weight 1416 g, 1170–1750; 5 min Apgar-score 8.6, 6–10) at postnatal age between 3 and 8 days were enrolled (Eiselt et al., 1997). All infants were clinically and neurologically normal. Recordings proceeded during sleep between 09.00 and 12.00 h a.m., immediately after feeding. All newborns lay in an incubator adapted to maintain normal body temperature. Tracings lasted on average of 104.8 ± 7.6 min (range 97–116 min) and included recordings of electrocardiogram (ECG), respiration signal, and electroencephalogram (EEG, leads: Fp2–C4, C4–O2, Fp1–T3, and C3–O1) with a sampling frequency of 281 Hz. The respiration signal was either detected by means of nasal thermistor spirography or by a strain gage placed around the thorax and abdomen. The ECG was recorded from two electrodes placed on the right and left lateral thorax. More detailed information can be seen in Eiselt et al. (1997).
Sleep states were coded through the concordance of EEG-patterns and the presence or absence of rapid eye movement (Curzi-Dascalova and Mirmiran, 1996), whereas simultaneous patterns of high amplitude spike waves (bursts) in the EEG were assigned by trained physicians. AS was characterized by continuous or semi-continuous EEG with rapid eye movements (REM), whereas QS was indicated by discontinuous non-REM EEG with predominantly burst activity. Markers (time stamps) for AS and QS states as well as for the bursts onset were simultaneously available. According to the markers several time series of 5 min duration with appropriate signal quality (continuous ECG and respiration signals without artifacts) were manually selected from AS states (mainly without bursts) and from QS states (with burst activity) for each newborn (Table 1).
Table 1. Demographic characteristics and number of extracted sleep states of the five preterm newborns.
Heart rate time series consisting of successive beat-to-beat intervals (BBI) were automatically extracted from all ECG recordings to obtain normal-to-normal (NN) heart rate time series. Time series of successive breath durations (RESP) were extracted automatically from the respiratory signal and, thereafter, visually inspected, and if necessary, edited. For coupling analyses both time series (BBI, RESP) were resampled with a frequency of 3 Hz to obtain synchronized time series (Figure 1).
Figure 1. Examples of a ECG and a respiration signal from one preterm newborn (A) and of BBI and RESP time series for active sleep (B) and quiet sleep (C) states.
Heart rate variability was univariate quantified by indices of time- and frequency domain and non-linear dynamics extracted from BBI time series. For bivariate cardiorespiratory coupling analyses (BBI–RESP) bivariate methods specifically from non-linear dynamics were applied.
Time and frequency domain indices [as recommended by the Task Force for adults (Task Force, 1996)] were adapted to temporal and spectral features of neonatal cardiorespiratory signals (Fortrat, 2002; Longin et al., 2006; Doyle et al., 2009; Krueger et al., 2010) because standards have not yet been established for infants. It should be noted that due to the relatively low sampling frequency of 281 Hz, findings from HRV analysis could be slightly overestimated. However, with proper pre-processing even a 100 Hz sampling rate can be sufficient (Task Force, 1996). The following time domain indices were calculated: meanNN and sdNN as the mean value and the standard deviation (SD) of NN intervals, pNN5 as the proportion of NN5 (number of interval differences > 5 ms) divided by total number of all NNs and Shannon_BBI as the Shannon entropy of the histogram (density distribution of all NN intervals). The Shannon entropy describes the statistical properties of a time series and represents a measure of uncertainty of a time series. The higher the Shannon_BBI, the higher is the HRV.
To estimate the power spectra of the BBI time series, Fast Fourier Transform with a Blackman Harris window function was applied. The following frequency domain indices were estimated from the spectrum: LFn – normalized low frequency (LF; 0.04–0.2 Hz) power = LF/(LF + HF) [arb. units], HFn – normalized HF (0.2–1 Hz) power = HF/(LF + HF) [arb. units] and the ratio LF/HF. The frequency domain indices LFn and HFn emphasize the controlled and balanced behavior of the two branches of the ANS. LFn most likely reflects a mix of both parasympathetic and sympathetic influences whereas HFn is a well-accepted non-invasive measure of cardiac parasympathetic activity in humans.
Non-linear dynamics within short-term time series were quantified by indices from symbolic dynamics (SD), compression entropy (CE), detrended fluctuation analysis (DFA), Poincaré plot analysis (PPA), and conditional entropy (CEn) (Voss, 2009).
Symbolic dynamics classifies dynamic changes within time series (e.g., BBI) whereas this time series was transformed into a symbol sequence of four symbols with a given alphabet A = {0, 1, 2, 3}. From this symbol sequence words were created consisting of three successive symbols (64 different word types). The following variability and complexity indices were calculated (Voss et al., 1996): wpsum02 as the number of word types consisting only of symbols “0” and “2” indicating lower HRV; wsdvar as the SD of the word sequence and wd_Renyi as the Renyi entropy of the word distribution (measure for complexity).
The CE method represents a non-linear index describing the complexity of a time series (Baumert et al., 2004), indicating to what extent a time series (BBI) can be compressed (Ziv and Lempel, 1977) using the detection of repetitive sequences (e.g., heartbeats; CE_BBI). Reduced short-term fluctuations of HRV result in an increased compression (lower compression entropy values), which reflects a complexity reduction. The highest complexity (time series cannot be compressed) generates the value 1.
Detrended fluctuation analysis (Peng et al., 1995) provides a short-term scaling exponent alpha1 over the range of 4 ≤ n ≤ 16 samples (e.g., heartbeats, alpha1_BBI) quantifying the presence or absence of fractal correlation properties in non-stationary time series.
PPA is a two-dimensional graphical representation (scatter plots) of each BBI plotted against the subsequent BBI. The shape of the plot is assumed to be influenced by changes in the vagal and sympathetic modulation and provides detailed beat-to-beat information on the behavior of the heart (Kamen and Tonkin, 1995). In this study we calculated the index SD1/SD2_BBI, quantifying the ratio between short-term (SD1) and long-term (SD2) variability from cardiac time series.
Conditional entropy (Porta et al., 1999) is based on the Shannon entropy and quantifies the information content inside a time series. Based on CEn, one can define the index of regularity (RI_BBI, RI_RESP) as a measure of a time series’ complexity.
Analysis of cardiorespiratory coupling was performed by applying cross conditional entropy (CCEn) (Porta et al., 1999), cross-correlation, mutual information, and JSD (Baumert et al., 2002; Caminal et al., 2010).
Cross conditional entropy estimates the degree of coupling between cardiorespiratory signals. Based on CCEn, one can define a synchronization index that quantifies the amount of information included in one time series when another time series is given. The larger the synchronization index (SI_BBI_RESP), the more coupled are the two time series (BBI and RESP).
We further applied the traditional cross-correlation function (CCF) and mutual information (trans-information) methods (MUI) to investigate cardiorespiratory interdependencies (linear and non-linear) between BBI and RESP time series. Thereby, CCF analysis considers only linear dependencies between the two time series whereas MUI considers linear and non-linear dependencies between the two time series (Pompe, 1993).
The JSD method as an enhanced version of SD characterizes the non-linear behavior of short-term cardiorespiratory coupling by means of symbols. The JSD transforms the BBI and the inspiratory interval time series (xBBI, xRESP) from a bivariate sample vector X (Eq. 1) into a bivariate symbol vector S (Eq. 2) the latter consisting of a sequence of symbols (sBBI, sRESP) of a given alphabet A = {0, 1}.
Here symbol “1” represents increasing values and symbol “0” decreasing and unchanged values applying the threshold level l equal to zero (Eqs 3 and 4).
Thus, short patterns (words, w) of symbol sequences with a length equal to three were formed. From all bivariate word type combinations the probability [p(wi,j)] of occurrence was estimated using an 8 × 8 word distribution density matrix W (columns represent RESP, rows represent BBI) ranging from [000, 000]T to [111, 111]T (Figure 2). To avoid the influence of different length of time series, the sum of all word type probabilities was normalized to 1. The following indices were estimated: normalized probability occurrences of bivariate word type combinations “XXX” of BBI time series and simultaneous “YYY” of inspiratory interval time series (RESP; e.g., BBI-111/RESP-000), the sum of each row (combinations with equal BBI word – BBIXXX), the sum of each column (combinations with equal RESP word – RESPYYY) and the Shannon entropy (JSDshannon, Eq. 5) of the word distribution density matrix as a measure of the overall complexity of the cardiorespiratory coupling. The higher the JSD Shannon entropy, the more variable and complex are the cardiorespiratory short-term fluctuations.
Figure 2. Joint symbolic dynamics – transformation of the bivariate sample vector X [BBI, beat-to-beat intervals; RESP, inspiratory interval time series (s)] into the bivariate symbol vector S (1: increasing values; 0: decreasing and unchanged values) leading to the word distribution density matrix W (8 × 8).
For a more detailed visualization of the predominantly occurring word type combinations within the word distribution density matrix we applied a threshold level ThL (0, 1, and 1.5%) to all probabilities of occurrence. Thus, we stepwise reduced (subtraction) the low variations within the word distribution density matrix.
Indices were calculated for all selected time series (BBI, RESP) and averaged for each newborn in each sleep state. The parametric paired t-test was applied for statistical evaluation of differences between the sleep states in preterm newborns. Univariate significances were considered for values of p < 0.05 and high significances for values of p < 0.01. Descriptive statistics were used to present the basic features of the data in terms of mean value (mean) and SD.
The mean value of NN intervals meanNN was not significant different between QS and AS (Table 2). The index sdNN showed significantly (p = 0.03) lower mean values for QS than for AS which was confirmed by a decreased mean value for pNN5 in QS. The index Shannon_BBI was significantly (p = 0.008) reduced in QS in comparison to AS.
Table 2. Univariate and bivariate indices comparing between active sleep (AS) and quiet sleep (QS) states.
Reduced normalized LF power and increased normalized HF power (p < 0.05) were found in QS. As a trend (not significant) the frequency domain index LF/HF was decreased in newborns within QS compared to AS (6.4 ± 2.7 vs. 13.3 ± 12.2 a.u.).
The SD method revealed significant lower (wsdvar_BBI, p = 0.002) and significant higher (wpsum02_BBI, p = 0.004) indices during QS in comparison to AS for BBI time series. The SD complexity index of the word distribution wd_Renyi, was highly significant (p = 0.0003) reduced in newborns during QS.
CE_BBI quantifying the degree of compressibility was significant (p = 0.0164) diminished in QS in comparison to AS.
Detrended fluctuation analysis and PPA revealed only significant differences for BBI time series. Thereby, the mean value of the short-term DFA scaling exponent alpha1_BBI was significantly (p < 0.01) decreased whereas the PPA index (SD1/SD2_BB) was significantly (p < 0.01) higher in the QS than in the AS state. In addition, univariate indices from CEn revealed no significant differences between QS and AS and nearly unchanged mean values for the regularity indices RI_BBI and RI_RESP.
Bivariate investigations of cardiorespiratory coupling by CCEn revealed a low and not significant coupling index SI_BBI_RESP between AS and QS. Analysis of short-term cardiorespiratory coupling by JSD demonstrated that word type combinations with “000” or “111” for BBI and particularly for RESP, indicating lower variability, were the most dominant word types in both sleep states but were declined in QS. However, the proportion of word type combinations with “100,” “001,” “011,” and “110,” marking higher variability, were mainly found in QS (Figure 3A, ThL = 0%). Further on, the BBI words “011” and “110” in combination with all other word types of RESP were significantly increased in QS.
Figure 3. Probability distributions of word types from JSD for cardiorespiratory coupling (BBI, beat-to-beat intervals; RESP, inspiratory interval time series) from preterm newborns during active sleep (AS) and quiet sleep (QS) states. (A) Complete 8 × 8 word distribution density matrix from BBI-000/RESP-000 to BBI-111/RESP-111. (B) Zoomed 8 × 6 word distribution density matrix after excluding the columns RESP-000 and RESP-111 and subtracting a threshold of 1% (0.01) from the amplitude (normalized probability of occurrence) to reduce the low variations. (C) Zoomed 8 × 6 word distribution density matrix after subtracting 1.5% (0.015).
After excluding the outer columns RESP-000 and RESP-111 from the JSD word distribution density matrix and applying ThL = 1% (reduction of low varying word types) some complete BBI rows (e.g., BBI110, p < 0.05) remained, especially within the reduced JSD matrix (Figure 3B) in QS. This behavior was even more obvious when ThL was set to 1.5% (Figure 3C). Within the reduced JSD matrix we further observed that word types consisting of alternating symbol sequences as “101” and “010” were rarely present for both BBI and RESP time series and even fewer were AS (Figure 3B) than in QS.
In this study the cardiorespiratory system in very preterm neonates during active and QS states was analyzed applying linear (time and frequency domain, correlation) and non-linear dynamics (symbolic dynamics, compression entropy, detrended fluctuation analysis, Poincaré plot analysis, mutual information, conditional entropy, and JSD) methods (Table 2). Significantly different regulation patterns of HR and respiration between active and QS states could be recognized The respiratory patterns represent a pronounced and monotonously increasing or decreasing regulation in both AS and QS. The HRV regulation patterns in QS are independent from respiratory regulation and probably representing an increasing vagal modulation. Finally it could be demonstrated that cardiorespiratory coupling is not significantly developed in very preterm neonates with 26–31 weeks GA.
Various studies found a slowing down in breathing and respiratory variability during QS compared to AS (Lewicke et al., 2008; Elder et al., 2011). However, in our study the mean value and the standard variation of the respiratory frequency were not significantly different between AS and QS, whereas a reduced respiratory variability in QS could be confirmed. According to HRV analysis the mean HR was not significant different between AS and QS, whereby the notable significantly lower SD of NN intervals indicated a decreased HRV in QS. Further, declined HRV indices as pNNi (i < 5 ms) and Shannon_BBI affirmed a reduced HRV by greater portions of smaller BBI differences respectively a diminished information content included in BBI time series in QS.
Regarding frequency domain analysis of BBI, the reduced LFn and increased HFn power suggested a sympathetic activity withdrawal accompanied by a progressively increased vagal activity during QS. This was also confirmed by the decline (trend) of the index LF/HF from 13.3 ± 12.2 a.u. in AS to 6.4 ± 2.7 a.u. in QS. However, one should consider that these indices have previously been mainly investigated in human adults. Pagani et al. (1997) showed that during sympathetic activation in normal humans, there is a predominance in the LF oscillation of blood pressure, RR interval, and sympathetic nerve activity whereas during sympathetic inhibition, the HF component of cardiovascular variability predominates. For very preterm neonates one should consider that they may not have the full complement of adrenergic receptors in the sinoatrial node or may not have a fully developed baroreflex that affects cardiac sympathetic activity.
The results from linear analysis were in accordance with previous other findings (Doyle et al., 2009; Vandeput et al., 2009) where a decrease in HRV (lower HR, sdNN, rmssd), LFn, LF/HF, and an increase in HFn during QS were demonstrated. However, in contrast to our study, these studies analyzed cardiac data from more mature preterm infants with GA ≥ 36 weeks.
In this study, univariate analyses of cardiorespiratory signals applying methods from non-linear dynamics revealed considerable differences between AS and QS states, especially for BBI time series. Indices from SD (wpsum02 and wsdvar) demonstrated a decrease in HRV much clearer than linear indices from the time domain. In addition, the increase of word types, only consisting of symbols “0” and “2,” in QS confirmed a reduced HRV. Moreover, the lowered value of wd_Renyi complemented the distinct decrease in HR complexity within QS compared to AS. Similar to this finding Vandeput et al. (2009) could show that the RR interval series are less chaotic during non-REM sleep.
Time series of less variability and complexity often include similar and repetitive patterns leading to reduced compression entropy. Therefore, the lower value of CE_BBI during QS due to an increased compressibility of cardiac time series represented a reduced complexity in HR regulation. In accordance with higher compressibility and less complexity, the reduction of the mean values of alpha1_BBI from DFA (even the DFA requires longer time series) and SD1_BBI and SD2_BBI from PPA suggested a loss of fractal-like dynamics in short-term as well as long-term HRV in QS. A decrease in SD2, but not in SD1, could also be confirmed comparing AS and QS in healthy newborns > 37 weeks GA by Doyle et al. (2009). In another study (Lewicke et al., 2011), indices from DFA, PPA, and fractal dimension were observed, comparing phases with and without cardiorespiratory events (CREs), like bradycardia and apnea, each within AS and QS states in preterm infants with ≥ 35 weeks conceptional age. Thereby a reduced DFA index alpha1 appeared to be a promising predictor of CRE in QS state.
Additionally, in our study CEn showed no significantly changed regularity indices (RI_BBI and RI_RESP) between AS and QS. The low values of the synchronization index SI_BBI_RESP represented nearly no cardiorespiratory coupling and did not differ significantly between AS and QS.
Elder et al. (2010) demonstrated that cardiorespiratory coupling represented by a reduced Shannon entropy of intervals between inspiration and preceding R-peaks is more frequently seen in QS than in AS. However, there was a significant difference at the boundary to a predefined range of uncoupling, probably avoiding a clear conclusion about cardiorespiratory coupling during different sleep states. Moreover, the investigated preterm newborns suffered from restricted growth at birth, smoking mothers, severe respiratory illness, or chronic lung disease. These disorders likely had an influence on autonomic regulation and cardiorespiratory coupling, and are therefore, not comparable with our results.
Another study (Frasch et al., 2007) investigated healthy full-term neonates, and suggested higher cardiorespiratory coupling in QS than in AS on the basis of increased complexity measures from autonomic information flow on local and global time scales. However, healthy full-term neonates are characterized by a more mature ANS than preterm newborns and this may cause marked changes in cardiorespiratory coupling during different sleep states. In our study, correlation and mutual information analysis revealed significant differences only for univariate HR time series expressing a less predictable HR regulation in QS. Bivariate applications (cross-correlation and cross-trans-information function) did not indicate any significant differences between QS and AS.
Bivariate analysis of short-term cardiorespiratory coupling applying JSD revealed various significant indices differentiating AS and QS states. The most occurring indices [p(wBBI, wRESP) ≥ 0.015] represented mainly monotonous word types “111” and “000,” especially in RESP, indicating a consecutive increasing (“111”) respectively unchanging or decreasing (“000”) of RESP. The two prominent RESP word types (111, 000; first and last column within the JSD matrix) occurred mostly (26–30%) in combination with all other BBI word types in both AS and QS, but more frequently in AS (Figure 3A, first and last column). These RESP patterns occur independently from the BBI patterns and reflect a sustained behavior of cardiorespiratory regulation. Hence, these results indicated a restricted short-term variation in respiration and an absence of clear cardiorespiratory coupling in very preterm neonates for AS and QS. The absence of cardiorespiratory coupling in preterm infants was confirmed by CCEn, correlation, and mutual information analysis. Moreover, the specific occurrences of RESP patterns could be considered as a marker for a restricted RSA. Interestingly, there are only few studies that investigated interactions between HR and respiration in preterm infants. These studies revealed inconsistent results related to absence (Giddens and Kitney, 1985; Longin et al., 2006) or presence (Thompson et al., 1993; Rassi et al., 2005) of RSA, depending on age and degree of development. Thus, the exact relationship between HR and respiration (RSA) in preterm infants remained elusive and seemed to be not yet completely developed, but can commonly be found in mature newborns, children, and adults (Indic et al., 2011). Due to the fact that the preterm newborns in this study were of very young age (26–31 weeks GA) it might be assumed that the development of RSA is still at the initial stage and in progress.
Considering only word types with a low probability of occurrence inside the JSD matrix (Figures 3B,C) there are two remarkable BBI rows in QS. Word types in these rows (e.g., BBI110, p < 0.05) occurred more often in QS and were independent from RESP patterns (Figure 3C). These BBI rows represented two specific HR regulation patterns of unidirectional HR change. This could be interpreted as vagal activity (a change of HR every two to three heart beats). Furthermore, short-term alternating patterns (word types “010” and “101”) were nearly not present suggesting a low or missing beat-to-beat vagal modulated HR in QS. The indices HFn and LFn represented a slight shift toward an increased vagal modulation of HR in QS that may be interpreted as early sign of a developing baseline vagal activity and is congruent to the upper JSD result.
Furthermore, the number of monotonous word types (“000,” ”111”) decreased, and seldom occurring bivariate word types [p(wBBI, wRESP) < 0.015] with simultaneous and more fluctuating patterns (“100,” “110”) increased (Figure 3B) in QS in comparison to AS. The decrease of most occurring word types (23%) and the increase of seldom occurring word types (37%) were a sign of less complexity in cardiorespiratory regulation in QS than in AS. This reduction of complexity was confirmed by the results from SD and compression entropy.
In full-term newborns (humans and animals) and in late preterm infants cardiorespiratory coupling can be demonstrated. Moreover, during the first days of life in full-term newborns cardiorespiratory coupling is nearly symmetric, while the direction is mainly determined by respiratory frequency (Mrowka et al., 2003). Due to higher respiratory frequencies (>0.6 Hz), which only occur at very young ages, the direction is less pronounced or even abolished. In an animal study on newborn piglets significant differences for cardiorespiratory coupling was revealed by indices from JSD due to hypoxia following reoxygenation (Reulecke et al., 2011; Schulz et al., 2011). Hypoxia initially led to greater HR complexity and to stronger cardiovascular and respiratory coupling, maintaining life in the newborn piglets. After reoxygenation, cardiovascular complexity parameters returned to pre-hypoxic values whereas regulating mechanisms related to respiration were prolonged and ongoing. Such possible life-threatening events like hypoxia might occur in human newborns as well, leading to a modified coupling and could be monitored by applying the above mentioned methods. In our study, however, due to the relatively young age of preterm infants (early development stage) no alteration of respiratory frequency (0.5–1.2 Hz) and no clear cardiorespiratory coupling could be found during the different sleep states. However, specific prominent regulation patterns of HR, probably caused in part by an increased vagal modulation were demonstrated in QS.
One limitation of this study is the small sample size (N = 5) of analyzed preterm newborns. Therefore, the data must be interpreted with caution. However, we circumvented this limitation somewhat by using averaging indices from multiple phases (AS: n = 23; QS: n = 20) to reduce the influence of uncertainties in statistical data. Another limitation is that the association of EEG bursts with HR accelerations might have a remaining influence on BBI regularity that was not proved. In a further study, this association of EEG bursts with HR accelerations should be investigated in combination with cardiorespiratory coupling analysis and causality analysis.
In conclusion, in various studies investigating cardiorespiratory coupling in late preterm infants, result indicate that depending on the development stage of infants, this coupling is more or less clearly visible. In this study we provide evidence by applying non-linear dynamics methods that cardiorespiratory coupling is not yet completely developed in very preterm neonates with 26–31 weeks GA. Furthermore, significantly different regulation patterns in bivariate oscillations of HR and respiration during active and QS states could be recognized. These patterns represent on the one hand a pronounced respiratory regulation in both AS and QS and on the other hand some prominent HR regulation patterns in QS that are independent from respiratory regulation and probably representing an increasing vagal modulation. Findings from this study could be useful in pediatrics for the purpose of monitoring very preterm neonates and detecting disorders in time by real-time tracking of cardiorespiratory signal alterations.
The authors declare that the research was conducted in the absence of any commercial or financial relationships that could be construed as a potential conflict of interest.
We thank Dr. Eiselt, Dr. Schwab, and Prof. Witte from the Institute of Medical Statistics, Computer Sciences and Documentation, Friedrich-Schiller-University, Jena, Germany for providing and preparing the newborns data. This study was partly supported by grants from the Deutsche Forschungsgemeinschaft (DFG-VO 505/8-1 and DFG-VO 505/8-2).
Andriessen, P., Oetomo, S. B., Peters, C., Vermeulen, B., Wijn, P. F., and Blanco, C. E. (2005). Baroreceptor reflex sensitivity in human neonates: the effect of postmenstrual age. J. Physiol. (Lond.) 568, 333–341.
Baumert, M., Walther, T., Hopfe, J., Stepan, H., Faber, R., and Voss, A. (2002). Joint symbolic dynamic analysis of beat-to-beat interactions of heart rate and systolic blood pressure in normal pregnancy. Med. Biol. Eng. Comput. 40, 241–245.
Baumert, M., Baier, V., Haueisen, J., Wessel, N., Meyerfeldt, U., Schirdewan, A., and Voss, A. (2004). Forecasting of life threatening arrhythmias using the compression entropy of heart rate. Methods Inf. Med. 43, 202–206.
Caminal, P., Giraldo, B. F., Vallverdu, M., Benito, S., Schroeder, R., and Voss, A. (2010). Symbolic dynamic analysis of relations between cardiac and breathing cycles in patients on weaning trials. Ann. Biomed. Eng. 38, 2542–2552.
Curzi-Dascalova, L., and Mirmiran, M. (1996). Manual of Methods of Recording and Analyzing Sleep-wakefulness States in Preterm and Full-Term Infants. Paris: Les Editions INSERM.
Doyle, O. M., Korotchikova, I., Lightbody, G., Marnane, W., Kerins, D., and Boylan, G. B. (2009). Heart rate variability during sleep in healthy term newborns in the early postnatal period. Physiol. Meas. 30, 847–860.
Eiselt, M., Schendel, M., Witte, H., Dorschel, J., Curzi-Dascalova, L., D’allest, A. M., and Zwiener, U. (1997). Quantitative analysis of discontinuous EEG in premature and full-term newborns during quiet sleep. Electroencephalogr. Clin. Neurophysiol. 103, 528–534.
Elder, D. E., Campbell, A. J., Larsen, P. D., and Galletly, D. (2011). Respiratory variability in preterm and term infants: effect of sleep state, position and age. Respir. Physiol. Neurobiol. 175, 234–238.
Elder, D. E., Larsen, P. D., Galletly, D. C., and Campbell, A. J. (2010). Cardioventilatory coupling in preterm and term infants: effect of position and sleep state. Respir. Physiol. Neurobiol. 174, 128–134.
Fortrat, J. O. (2002). Inaccurate normal values of heart rate variability spectral analysis in newborn infants. Am. J. Cardiol. 90, 346.
Frasch, M. G., Zwiener, U., Hoyer, D., and Eiselt, M. (2007). Autonomic organization of respirocardial function in healthy human neonates in quiet and active sleep. Early Hum. Dev. 83, 269–277.
Giddens, D. P., and Kitney, R. I. (1985). Neonatal heart rate variability and its relation to respiration. J. Theor. Biol. 113, 759–780.
Hoyer, D., Bauer, R., Walter, B., and Zwiener, U. (1998). Estimation of nonlinear couplings on the basis of complexity and predictability – a new method applied to cardiorespiratory coordination. IEEE Trans. Biomed. Eng. 45, 545–552.
Indic, P., Bloch-Salisbury, E., Bednarek, F., Brown, E. N., Paydarfar, D., and Barbieri, R. (2011). Assessment of cardio-respiratory interactions in preterm infants by bivariate autoregressive modeling and surrogate data analysis. Early Hum. Dev. 87, 477–487.
Indic, P., Salisbury, E., Paydarfar, D., Brown, E., and Barbieri, R. (2008). Interaction between heart rate variability and respiration in preterm infants. Comput. Cardiol. 35, 57–60.
Kamen, P. W., and Tonkin, A. M. (1995). Application of the Poincare plot to heart rate variability: a new measure of functional status in heart failure. Aust. N. Z. J. Med. 25, 18–26.
Krueger, C., Van Oostrom, J. H., and Shuster, J. (2010). A longitudinal description of heart rate variability in 28–34-week-old preterm infants. Biol. Res. Nurs. 11, 261–268.
Lewicke, A., Corwinb, M., Schuckers, M., Xud, X., Neumane, M., and Schuckers, S. (2011). Analysis of heart rate variability for predicting cardiorespiratory events in infants. Biomed. Signal Process. Control.
Lewicke, A., Sazonov, E., Corwin, M. J., Neuman, M., and Schuckers, S. (2008). Sleep versus wake classification from heart rate variability using computational intelligence: consideration of rejection in classification models. IEEE Trans. Biomed. Eng. 55, 108–118.
Longin, E., Gerstner, T., Schaible, T., Lenz, T., and Konig, S. (2006). Maturation of the autonomic nervous system: differences in heart rate variability in premature vs. term infants. J. Perinat. Med. 34, 303–308.
Mrowka, R., Cimponeriu, L., Patzak, A., and Rosenblum, M. G. (2003). Directionality of coupling of physiological subsystems: age-related changes of cardiorespiratory interaction during different sleep stages in babies. Am. J. Physiol. Regul. Integr. Comp. Physiol. 285, R1395–R1401.
Pagani, M., Montano, N., Porta, A., Malliani, A., Abboud, F. M., Birkett, C., and Somers, V. K. (1997). Relationship between spectral components of cardiovascular variabilities and direct measures of muscle sympathetic nerve activity in humans. Circulation 95, 1441–1448.
Peng, C. K., Havlin, S., Stanley, H. E., and Goldberger, A. L. (1995). Quantification of scaling exponents and crossover phenomena in nonstationary heartbeat time series. Chaos 5, 82–87.
Porges, S. W., and Furman, S. A. (2011). The early development of the autonomic nervous system provides a neural platform for social behavior: a polyvagal perspective. Infant Child Dev. 20, 106–118.
Porta, A., Baselli, G., Lombardi, F., Montano, N., Malliani, A., and Cerutti, S. (1999). Conditional entropy approach for the evaluation of the coupling strength. Biol. Cybern. 81, 119–129.
Rassi, D., Mishin, A., Zhuravlev, Y. E., and Matthes, J. (2005). Time domain correlation analysis of heart rate variability in preterm neonates. Early Hum. Dev. 81, 341–350.
Reulecke, S., Schulz, S., Bauer, R., Witte, H., and Voss, A. (2011). “Quantification of cardiovascular and cardiorespiratory coupling during hypoxia with joint symbolic dynamics,” in International Conference of the IEEE Engineering in Medicine and Biology Society, Boston.
Schulz, S., Koschke, M., Bar, K. J., and Voss, A. (2010). The altered complexity of cardiovascular regulation in depressed patients. Physiol. Meas. 31, 303–321.
Schulz, S., Reulecke, S., Eiselt, M., Schwab, K., Witte, H., Walter, B., Bauer, R., and Voss, A. (2011). Quantification of compensatory processes of postnatal hypoxia in newborn piglets applying short-term nonlinear dynamics analysis. Biomed. Eng. Online 10, 88.
Task Force. (1996). Heart rate variability. Standards of measurement, physiological interpretation, and clinical use. Task Force of the European Society of Cardiology and the North American Society of Pacing and Electrophysiology. Eur. Heart J. 17, 354–381.
Thompson, C. R., Brown, J. S., Gee, H., and Taylor, E. W. (1993). Heart rate variability in healthy term newborns: the contribution of respiratory sinus arrhythmia. Early Hum. Dev. 31, 217–228.
Vandeput, S., Naulaers, G., Daniels, H., and Van Huffel, S. (2009). Heart rate variability during REM and non-REM sleep in preterm neonates with and without abnormal cardiorespiratory events. Early Hum. Dev. 85, 665–671.
Voss, A., Kurths, J., Kleiner, H. J., Witt, A., Wessel, N., Saparin, P., Osterziel, K. J., Schurath, R., and Dietz, R. (1996). The application of methods of non-linear dynamics for the improved and predictive recognition of patients threatened by sudden cardiac death. Cardiovasc. Res. 31, 419–433.
Voss, A., Schroeder, R., Truebner, S., Baumert, M., Goernig, M., Hagenow, A., and Figulla, H. R. (2006). Multivariate and multidimensional analysis of cardiovascular oscillations in patients with heart failure. Biomed. Tech. (Berl.) 51, 163–166.
Voss, A., Schulz, S., Schroeder, R., Baumert, M., and Caminal, P. (2009). Methods derived from nonlinear dynamics for analysing heart rate variability. Philos. Transact. A Math. Phys. Eng. Sci. 367, 277–296.
Keywords: autonomic regulation, cardiorespiratory coupling, preterm newborns, sleep states, non-linear dynamics
Citation: Reulecke S, Schulz S and Voss A (2012) Autonomic regulation during quiet and active sleep states in very preterm neonates. Front. Physio. 3:61. doi: 10.3389/fphys.2012.00061
Received: 04 November 2011; Accepted: 29 February 2012;
Published online: 10 April 2012.
Edited by:
Jacqueline Kathleen Phillips, Macquarie University, AustraliaReviewed by:
Karie Scrogin, Loyola University Chicago, USACopyright: © 2012 Reulecke, Schulz and Voss. This is an open-access article distributed under the terms of the Creative Commons Attribution Non Commercial License, which permits non-commercial use, distribution, and reproduction in other forums, provided the original authors and source are credited.
*Correspondence: Andreas Voss, Department of Medical Engineering and Biotechnology, University of Applied Sciences Jena, Carl-Zeiss-Promenade 2, 07745 Jena, Germany. e-mail:dm9zc0BmaC1qZW5hLmRl
Disclaimer: All claims expressed in this article are solely those of the authors and do not necessarily represent those of their affiliated organizations, or those of the publisher, the editors and the reviewers. Any product that may be evaluated in this article or claim that may be made by its manufacturer is not guaranteed or endorsed by the publisher.
Research integrity at Frontiers
Learn more about the work of our research integrity team to safeguard the quality of each article we publish.