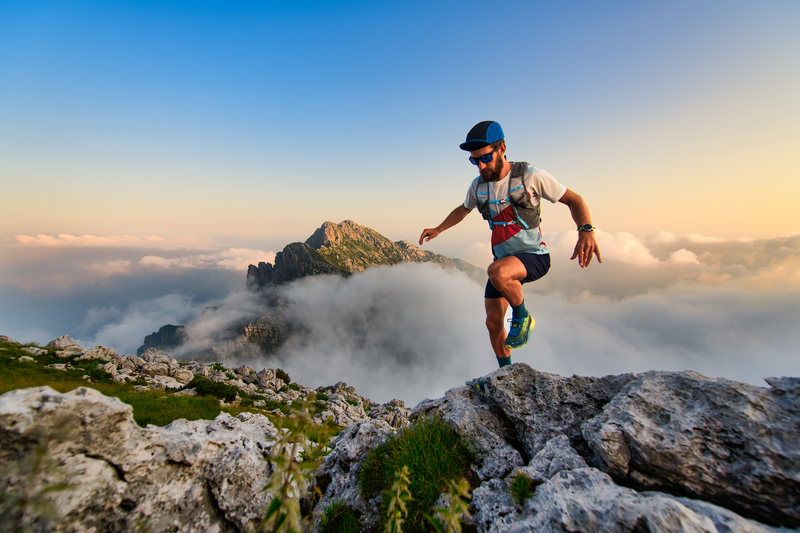
95% of researchers rate our articles as excellent or good
Learn more about the work of our research integrity team to safeguard the quality of each article we publish.
Find out more
ORIGINAL RESEARCH article
Front. Phys. , 26 February 2025
Sec. Social Physics
Volume 13 - 2025 | https://doi.org/10.3389/fphy.2025.1537110
With the development of transport and information technology (IT), urban innovation linkages are becoming increasingly close and are key factors in regional economic growth. Despite previous studies analyzing urban network characteristics, structures, and factors that influence them, there has been little exploration of their socioeconomic effects. Based on unique patent transaction data, this study analyzes the spatiotemporal evolution of urban innovation networks and their impacts and internal mechanisms on regional green total factor productivity (GTFP) using social network analysis, data envelopment analysis, and a negative binomial model. It was found that (1) the enhancement of intercity innovation linkages and their networked development have significantly improved urban GTFP and the innovation network’s embeddedness helps obtain network externalities to improve urban GTFP. (2) The innovation network promotes inter-regional production factor flow and optimal allocation and generates knowledge spillover effects to improve green technology innovation capacity, thus improving urban GTFP. (3) Spatial heterogeneity exists in the impact of the innovation network on the GTFP of different regions and economically developed big cities by gathering more innovation resources, forming closer innovation links, improving the level of green technology innovation and production efficiency, and contributing to urban GTFP. Economically developed big cities can have more innovation resources and form closer innovation links, improve green technology innovation and production efficiency, and have a stronger promotional effect on improving urban GTFP.
Since the early 21st century, the rapid growth of globalization and informationization, has strengthened inter-city linkages, giving rise to the emergence of mobile spaces [1]. Urban networks have reshaped global-local relations, led to new types of territorial spatial organizations, such as city clusters, and triggered a revolution in geospatiality [2]. Since the relational turn, urban networks have become a central focus across various disciplines, including geography, economics, and planning [3]. Scholars at home and abroad have analyzed the characteristics, structures, and influencing factors of different urban networks at multiple scales, such as global, national, and regional, based on the flow of traffic [4], capital [5], and information [6]. However, few studies have explored the socio-economic effects of urban networks, and there is a lack of a systematic theoretical framework or a consensus in the existing research [7]. Although some scholars have preliminarily analyzed the impact of urban networks on regional economic growth by borrowing concepts such as scale [8], urban networks are mainly measured using static attribute data, such as gravitational models and enterprise branches, which do not comprehensively and realistically reflect the diversified inter-city linkages [9].
In the knowledge economy era, innovation is the core factor determining the long-term growth of regional economies [10]. As spatial carriers of innovation, cities provide proximity, density, and diversity that enable innovation agents to interact, collaborate, and compete, generating innovation through knowledge spillover [11]. However, the innovation resources of a city alone are limited, and new knowledge can only be generated by embedding it in innovation networks and acquiring external knowledge [12]. Owing to a lack of micro-level data on innovation linkages, scholars have conducted relatively insufficient research on innovation network establishment and measurement [13]. Some scholars have indirectly measured innovation linkages through alternative variables, such as dissertation cooperation [14], talent flow [15], research and development (R&D) cooperation [16], international merchandise trade, and foreign investment [17]. Wang et al. [18] found that talent mobility through spatial spillovers significantly contributes to innovation and high-tech industries.
Patent transactions, as a key method of innovation resource flow, involve the transfer of ownership or rights of patents from the transferor to the transferee through market mechanisms. These transactions have become a primary vehicle for technology transfer and innovation linkage. Therefore, based on micro patent transaction data, this article analyzes the influence of innovation networks on urban GTFP and its internal mechanism of action using super-efficient SBM, social network analysis, and negative binomial model analysis methods. Heterogeneity analysis explored the differential impact of innovation networks on the GTFP in different regions and provided policy recommendations for regional sustainable development.
The main contributions of this study are as follows: First, it accurately measures urban GTFP using the improved super-efficient SBM model to measure urban green development, compensating for the inadequacy of existing studies that unilaterally utilize a single indicator to measure GTFP. Second, it measures inter-city innovation linkages and the evolution of their network structures based on unique patent transaction data, which deepens the understanding of the evolution law of the innovation network structure. Third, while existing studies analyze the factors influencing innovation networks, they do not analyze their socioeconomic effects. This study analyses and explores the impact of innovation networks on urban GTFP using a combination of social network analysis and negative binomial modeling, thus providing empirical evidence to understand the environmental effects of innovation networks.
Cities can access innovation resources in a larger spatial scope by embedding innovation networks, compensating for regional shortcomings in capital, technology, and information, reducing R&D risks and production costs, and improving resource allocation efficiency and its GTFP [19]. First, based on the consumer city theory and industrial division of labor theory, in the mature stage of urban agglomeration development, the function of the central city as a manufacturing center gradually diminishes [20], while its function as a consumption and service center grows [21].
Innovation cooperation between cities can improve the efficiency of resource allocation by increasing regional specialization. Second, the development of inter-city transportation infrastructure increases the frequency of people moving between cities, and neighboring cities can provide a wider range of innovation resources, such as talent, capital, and information, to the central city, thus making a greater contribution to its innovation development [22]. Finally, geographic proximity and similar regional cultures contribute to knowledge exchange and innovation cooperation, and neighboring cities can take advantage of the spatial spillover effects of knowledge and industries from the central city to improve the efficiency of innovation resource allocation [23]. Therefore, we propose the following hypothesis.
H1: Innovation resources help improve resource allocation efficiency, thus promoting urban GTFP.
Cities can export patented technologies to cities with low node intensity through technological cooperation and exchange. This will narrow the “technology gap” between these cities, improve their green innovation capacity, and improve GTFP [24]. First, more important cities often have better access to resources than other network members, meaning they receive information faster, making it easier for them to access technological, market, and managerial knowledge [25]. Second, more important cities tend to have a higher social status and power, making it easier for them to gain support from other network members [8]. Also, more important cities have more control over other network members, which means they are more capable of controlling, digesting, and integrating technological innovation resources than other cities. When new knowledge and technologies emerge from cities of equal status in China, they are more likely to develop further in these areas, thereby increasing the green innovation capacity [26]. Therefore, the following research hypothesis is proposed.
H2: An innovation network increases innovation through knowledge spillovers, thus driving urban GTFP improvement.
The patent transfer is a voluntary market transaction behavior between the demand and supply sides, which objectively reflects the flow of technology and knowledge sharing between cities and has become an important way to establish an innovation network [27]. First, based on big data, the patent information service platform website was obtained to collect detailed patent transfer data from 2005 to 2020, including the title of the patent, before and after the transfer of the rights holder and address, and the time of application-authorization-transfer, which are rich in vectorial (direction of the transfer), spatial-temporal (time of the transfer), subjective (transfer), and objective (patent rights holder). Second, the geocoding function of Python software was used to match the inventor’s patent address information to the corresponding city, and the data were verified by random sampling and cross-checking to ensure the scientificity and uniformity of the data.
Finally, based on the principle of graph theory, the weighted asymmetric matrix of intercity technology transfer (Equation 1) is established by taking prefecture-level and higher cities as nodes (N) and the number of patent transfers between cities as edges (E), where Wij represents the number of patents transferred from city i to city j, to characterize the strength of city innovation links. On this basis, the OD linkage network was established and spatially visualized using GIS software, with the strength of intercity innovation linkages as the weight of network edges and the total amount of city innovation linkages as the weight of nodes. Innovation networks are directed weighted networks, where cities are nodes, inter-city links are links, and link strengths are link weights.
In Formula 1, W is the matrix relationship, and w is the weight of the aviation and innovation connections in the matrix relationship.
Social network analysis software, UCINET, was used to topologize the urban network and measure indicators, such as node degree centrality, to analyze the characteristics of Chinese urban networks. In network research, scholars have primarily used degree centrality, betweenness centrality, closeness centrality, and the K-shell to measure the importance of nodes [28]. Degree centrality characterizes nodes’ control and mediation ability and is an important indicator for analyzing the influence and status of urban nodes in a network [29]. The node degree centrality is calculated according to Formula 2, where i denotes the number of connections with other nodes; g is the number of possible connections of the node x [13,30]. The formula used is as follows:
Green Total Factor Productivity (GTFP) means achieving the highest output with the least resource input while protecting the environment [31]. GTFP is a core indicator of regional economic growth and ecological environmental protection. In this study, we calculated the urban GTFP and its decomposition for Chinese cities using MATLAB software (Table 1). The calculation process involved input, desired output, and carbon emission variables. The relevant indicators and data processing are explained as follows: (1) Capital input: This study uses social fixed asset investment instead and the perpetual inventory method. (2) Labor input: Given data availability, this study uses the number of employed people in each province at the end of the year to measure labor input. (3) Energy input: Concerning most of the literature, the total energy consumption converted using the standard coal method was chosen as a proxy variable for energy input in units of 100,000 tons of standard coal. (000) Desired output: In this study, the GDP of each province, which is deflated to the price index for 4 years, is used to indicate the desired output.
Carbon emissions: Because carbon emissions data for China’s cities are not directly available and fossil energy consumption is the main source of carbon emissions, this study used Calculation Method one provided by the IPCC to calculate carbon emissions. These emissions were based on the China Energy Statistics Yearbook and targeted coal, coke, crude oil, gasoline, diesel, kerosene, fuel oil, and natural gas.
Gravity, negative binomial, and stochastic exponential models are widely used to measure the effects of innovation networks. Since the number of patents is a non-negative integer and the data are often characterized by “over-dispersion” [32], obtaining an effective unbiased estimate using the benchmark OLS regression is difficult. Therefore, this study adopted a negative binomial model to explore the impact of intercity innovation linkages and their resulting networked development on urban GTFP.
In addition, regional green economic development is a complex and dynamic process that is not only affected by innovation linkages and their networked development but is also constrained by other factors such as resource endowment, agglomeration economy, and environmental regulation [33]. Environmental regulations profoundly impact urban GTFP by affecting firms’ production costs and technological innovation performance through production costs and Porter effects, respectively. Therefore, this study considers resource endowment, agglomeration economy, and environmental regulation as important control variables for improving the accuracy of the estimation results. The benchmark model established in this study is as follows:
Equation 3 denotes lnGTFPit regional green total factor, LKit denotes inter-city innovation linkages to characterize the degree of inter-city innovation networking in China, ERit denotes environmental regulation, Xit denotes a series of control variables such as resource endowment, agglomeration economy, and the level of openness to the outside world, Uit denotes the individual effect and is used to control the individual characteristics of the city, εit denotes the error term of the model, a0 denotes the intercept term.
Since the explanatory variables are all urban GTFP, the control variables of environmental regulation, resource endowment, industrial agglomeration, industrial structure, and energy consumption structure were selected regarding other studies [34]. Table 2 presents the names of the main variables and their measurements.
The statistical data used in this study were mainly obtained from the China Regional Statistical Yearbook and China Urban Statistical Yearbook. in addition, some missing data were supplemented and improved by examining the city statistical bulletins of the corresponding years. Descriptive statistics for the main variables are presented.
To identify the pattern and characteristics of innovation network linkages, urban innovation linkages are graded and visualized based on the natural discontinuity grading method, finding that urban innovation networks are characterized by hierarchy and imbalance. In Figure 1, the thicker and darker the line, the greater the intensity of urban innovation linkages. Specifically, it includes the following aspects.
(1) China’s urban innovation linkages are increasing, but the overall network linkages are weak and characterized by obvious hierarchy and path dependence. In the early stages of the network, most of the inter-city innovation linkages were generally below 50, and only the innovation linkages between Beijing and Shenzhen exceeded 10; thus, the overall development level of the innovation network was relatively low. 2010 has seen the formation of high-level innovation linkages between economically developed large cities such as Beijing and Shenzhen and neighboring regions such as Shanghai-Suzhou, Shenzhen-Dongguan, etc. Dongguan and neighboring regions have seen higher-ranking innovation links, reflecting that the innovation network has hierarchical diffusion among high-ranking cities and proximity contacts among neighboring cities. However, high-intensity innovation linkages are still concentrated between a few large cities, such as Beijing and Shenzhen. In contrast, the intensity of innovation linkages in most cities is still low, resulting in an obvious hierarchical nature of the innovation network.
(2) The spatial distribution of inter-city linkages in China is uneven and shows decreasing characteristics in the East-Central-West zone. The eastern region has more high-level inter-city innovation links and a higher network density. However, only a few core cities in the central and western regions, such as Chengdu, Chongqing, and Wuhan, have formed high-level inter-regional innovation links. In contrast, most other cities have fewer innovation links and lower linkage intensity. Border cities such as Heihe, Turpan, and Dehong have long been in spatial isolation of China’s innovation linkages, lacking channels for the flow of innovation factors with cities in the eastern region, reflecting the high degree of imbalance in the spatial distribution of China’s urban linkages.
(3) Significant differences in urban innovation capacity reflect the distribution of urban hierarchies to a certain extent and significantly impact regional economic patterns. In particular, first-tier cities, such as Beijing, Shanghai, Guangzhou, and Shenzhen, always have the highest degree of centrality, become the source of influence and radiation of the innovation network, and are at the top level of the urban system. By their superiority as provincial capitals, central and western cities such as Changsha have also formed a highland of innovation resource concentration and played an intermediary role at the second level of the urban system. However, despite small and medium-sized cities becoming integrated into the innovation system due to their lower centrality, they face significant challenges in retaining innovation resources, remaining at the bottom of the innovation network.
Therefore, this study uses the super-efficient SBM model to measure China’s urban GTFP from 2005 to 2020 and uses GIS software for spatial visualization to excavate the spatiotemporal evolution characteristics and patterns of the urban GTFP. In Figure 2, the darker the color, the higher the GTFP, where red indicates the highest GTFP and blue indicates the lowest GTFP.
(1) In 2005, China’s urban GTFP was generally high in the east and low in the central and western regions. In particular, the GTFP of the Beijing-Tianjin-Hebei, Yangtze River Delta, and Pearl River Delta city clusters on the eastern coast were generally high and showed obvious spatial agglomeration characteristics. These economically developed city clusters attract many high-tech and high-quality enterprises through superior location conditions and good infrastructure, and the strong regional innovation ability and more developed tertiary industries have promoted the improvement of urban GTFP. However, the GTFP of most cities in Sichuan, Guizhou, Gansu, and Shaanxi is generally low, and the regional green innovation capacity and industrial structure are also low, even becoming an agglomeration of some polluting enterprises, which seriously affects the high-quality development of the central and western regions. Some cities in economically developed coastal areas have a low GTFP, reflecting the seriousness of unbalanced and uncoordinated regional development.
(2) In 2010, the spatial differences in China’s urban GTFP continued to expand, indicating an evident Matthew effect. The Beijing-Tianjin-Hebei, Shandong Peninsula, and Yangtze River Delta urban agglomerations still had relatively high GTFP, showing obvious agglomeration characteristics. Although the Pearl River Delta region is more innovative, the city’s vigorous economic development and the introduction of a large number of foreign-funded enterprises have increased the total amount and intensity of pollution emissions, reducing the urban GTFP and affecting sustainable development, reflecting that there are still inconsistencies and imbalances in China’s regional economic development.
(3) In 2015, China’s urban GTFP showed a spatial pattern of being high in the east and low in the central and western parts of the country; however, the GTFP of most cities increased. Since 2015, economically developed regions increasingly focused on environmental pollution and introduced regulatory measures, such as sewage charging, to curb emissions. As a result, some enterprises have relocated to neighboring areas of Guangdong Province to lower production costs and reduce the number of polluting enterprises and regional pollution. This shift has improved the green economy of developed regions.
(4) In 2020, the GTFP of most cities in China improved but was still characterized by spatial agglomeration. In particular, with the Beautiful China strategy, economically developed coastal cities, such as BTH, YRD, and PRD, increased their environmental protection efforts and urged enterprises to carry out green technological innovations to reduce pollutant emissions, which has promoted the transformation and upgrading of the industrial structure of developed coastal cities and the improvement of urban GTFP. However, Huizhou City and Shanwei City in Guangdong Province, which have taken over more pollution-intensive enterprises from Guangzhou and Shenzhen, still have a low GTFP and have become weak areas for the high-quality development of Guangdong’s economy, which urgently needs to strengthen environmental protection and pollution control efforts to improve urban GTFP.
The estimated coefficients of innovation linkages are significantly positive in the different regression models; however, the negative binomial model has the best fit. In the negative binomial model, for every percentage point increase in innovation linkage, urban GTFP increases by 0.016, reflecting that the enhancement of inter-regional innovation linkage and its networked development significantly contributes to the improvement of green total factor productivity. The enhancement of inter-city innovation links contributes to the dissemination and exchange of invisible knowledge and improves the innovation ability and production efficiency of enterprises through the knowledge spillover effect, which raises the important micro-foundation for the technological upgrading of regional industries and the improvement of the green economy. At the same time, enhanced innovation linkages between cities promote the cross-regional flow of innovative resources, such as talent, capital, and technology; improve the efficiency of innovation resource allocation and utilization; and reduce resource and energy consumption and regional pollution emissions (Table 3).
Environmental regulation is also an essential factor affecting urban GTFP. With improvements in environmental regulation, the cost of pollution control for enterprises increased. However, it can force enterprises to carry out green technological innovation and reduce pollution emissions, thus promoting the green transformation and upgrading of industries and the improvement of urban GTFP. At the same time, the agglomeration economy significantly improves urban GTFP. Therefore, developing an agglomeration economy helps enterprises generate knowledge spillover effects through sharing, learning, and matching mechanisms to improve innovation and productivity. It also helps enterprises centralize their pollution control and common use of resources, thus reducing regional pollution emissions and improving their GTFP.
To solve the endogeneity problem of the model, this study measures the community structure of the innovation network through social network analysis. It regresses it as an instrumental variable to improve the model’s scientificity [35]. The regression results show that the regression coefficient of the instrumental variable and GTFP is 1.678 and significant at the 1% level, with a standard error of 0.213, indicating that the regression results of the instrumental variable are somewhat similar to those of the baseline regression, reflecting that the development of innovation networks promotes the improvement of GTFP. Meanwhile, the F-statistic in the one-stage regression was 26.13 and greater than 10, further indicating the validity of the instrumental variables.
As an important aspect of the comprehensive strength of cities, factors such as the level of urban innovation, urbanization, and size of cities have a certain impact on the improvement of urban GTFP. Specifically, urban innovation has a significant promotional effect on urban GTFP, but urbanization has a significant negative impact on urban GTFP. As the urbanization level increases, the population grows, and a large amount of industrial land appears, which leads to an increase in traffic congestion and pollution emissions, negatively affecting urban GTFP. In addition, city size negatively affects urban GTFP. With the increasing urban population, the congestion effect of cities increases and exacerbates environmental pollution and other problems, thus reducing urban GTFP.
This study uses the mediating effect model to analyze the influence mechanism of inter-city innovation linkages and the knowledge spillover effect it generates on urban GTFP from the dimensions of factor allocation efficiency and green technology progress. In the mediation effect model, this study uses the network weighting degree as the core explanatory variable to explore the impact of the strength of urban innovation linkages on urban GTFP. First, drawing on the practice of scholars [36], the regional capital mismatch index and labor mismatch index are calculated to analyze the impact of innovation networks on capital and labor mismatch. Second, the number of green patents was used to characterize green technological progress and analyze the impact of innovation networks on green technological progress. Finally, the impacts of capital mismatch, labor mismatch, and green technology progress on urban GTFP were analyzed separately.
In Equations 4–7, the relationships between resource mismatch and innovation linkages, and green technology progress and innovation linkages are measured, respectively.
First, inter-city innovation linkages have a significantly negative effect on regional capital mismatch (Table 4). With the continuous development of patent transactions, the flow of innovation resources such as capital, information, and technology between regions accelerates, thereby reducing the degree of capital mismatch. Cities can break through the distance limitation by embedding themselves in the innovation network to obtain sufficient capital and other innovation resources in a wider range and promote a more efficient flow of the innovation network under network externalities to improve the allocation efficiency of capital and other innovation resources. Additionally, although inter-city innovation links significantly promote urban GTFP, capital mismatch negatively affects urban GTFP, indicating that capital mismatch is an important factor hindering urban GTFP. With the enhancement of urban innovation links, innovation resource factors accelerate the flow and help alleviate the distortion of factor market prices, improve the efficiency of capital use and allocation, improve the productivity of enterprises, reduce production costs, and promote green development in the region.
Second, from the perspective of labor mismatch, intercity innovation linkages significantly reduce regional labor mismatches. This highlights that strengthening innovation linkages facilitates the free flow of key resources—such as capital, technology, and talent—which helps address labor imbalances. As intercity transportation infrastructure improves, high-quality labor moves more freely across regions, gradually relocating to areas or sectors offering higher marginal compensation. This fosters the agglomeration of innovation resources and enhances the efficiency of their allocation.
Moreover, inter-city innovation links significantly promote improved urban GTFP, but labor mismatch has a significantly negative effect on urban GTFP, indicating that labor resource mismatch also hinders the improvement of urban GTFP. With the enhancement of inter-city innovation links, the inter-regional flow of high-quality talent across regions is more frequent. It generates knowledge spillover effects, which help to improve the regional innovation level and improve the efficiency of allocation and utilization of innovation resources, such as capital and labor, thus promoting the improvement of urban GTFP.
Third, the estimated coefficient of innovation linkage on green technology progress is significantly positive, indicating that with the development of patent transactions, innovation factors accelerate the flow and promote the formation and development of regional innovation networks, which helps regions obtain more innovation resources and learn from each other through network channels. In the flow of innovation factors, a city absorbs external knowledge. It strengthens the interaction of local knowledge through the knowledge flow system of local buzzing and global channels, generating knowledge spillover and promoting green technology progress. At the same time, improving the regional green technology level promotes the manufacturing industry to reduce pollution and emissions. It also improves the efficiency of resource allocation and promotes its green transformation. In addition, the estimated coefficient of the spatial lag term of green technological progress was significantly positive, indicating a significant spatial spillover effect of green technological progress.
Therefore, to explore the regional heterogeneity of the impact of innovation networks on the GTFP in different regions, this study divides these cities into eastern, central, and western cities and regresses them separately using a negative binomial model (Table 5).
Inter-city innovation linkages significantly impact the GTFP in different regions, but the degree of impact is heterogeneous. Among them, innovation linkages have the strongest impact on GTFP in cities in the eastern region, followed by cities in the central region, and have the least impact on GTFP in the western region. The eastern region is more economically developed and generally has many innovative resources, such as universities and high-tech enterprises. Cities have a higher degree of centrality and innovation linkages, and it is easier for them to obtain more resources and conduct patent-trading activities through innovation networks, thereby promoting tacit knowledge dissemination.
At the same time, the eastern region attracts a large inflow of innovation resources from other cities in the central and western regions through a good innovation environment and transportation facilities. It becomes a highland of innovation resource concentration and radiation, making it easier to realize the region’s economic development and green technological innovation through network and agglomeration externalities, thus improving the region’s economic performance. However, the economies of the central and western regions are generally more backward, the intensity of innovation links in most cities is low, and innovation resources are concentrated only in a few core cities such as Chongqing, Chengdu, and Xi’an. This makes it difficult to effectively exert the radiation effect of invisible knowledge dissemination and innovation links and, to a certain extent, restricts the development of regional green technological innovation and economic activities.
Based on unique patent transaction data, this study reveals the spatiotemporal pattern of innovation networks and urban GTFP using the improved super-efficient SBM model and social network analysis. Furthermore, this study uses a negative binomial model to explore innovation networks’ impact on the urban GTFP and its mechanism of action.
(1) The innovation network significantly improves urban GTFP, and the conclusion still holds after endogeneity and robustness tests. Environmental regulations also significantly contribute to GTFP.
(2) The innovation network mainly promotes the urban GTFP by alleviating regional labor and capital mismatches and improving green technology. With the enhancement of intercity innovation linkage, the innovation network is gradually formed and developed, which not only promotes the free flow of innovation factors and industrial agglomeration but also promotes the application of energy-saving and emission-reduction technologies through the knowledge spillover effect.
(3) The innovation network impacts GTFP differently in different regions, in which economically developed big cities attract more innovation resources under priority linkage through good infrastructure and interaction and form closer innovation linkages, obtaining fuller agglomeration and network externalities.
This study has important policy implications for regional innovation systems and green transformation. First, the government should strengthen the construction of a cross-regional technology market and increase the strength of inter-regional innovation linkages. Second, governments should adopt differentiated technology transaction regulatory policies in different regions. Finally, the government should actively introduce relevant policies to optimize the spatial allocation of innovation resources and give full play to the critical role of industrial agglomeration in promoting regional green economy performance.
This study measures GTFP through the super-efficient SBM model compared to previous studies. It analyzes the impact of inter-city innovation linkages on urban GTFP using the negative binomial model, which clarifies the socioeconomic effects of innovation networks and provides policy recommendations. However, there are some limitations. This study mainly focuses on inter-city patent transactions and their technology factor flows. Still, it lacks attention to patent transaction activities and their impact on regional green development at different scales, such as inter-country and inter-county. Therefore, future research could use big data technology to obtain more detailed data on the number and amount of patent transactions at the national, city, and county scales to characterize interregional innovation linkages fully.
The datasets presented in this study can be found in online repositories. The names of the repository/repositories and accession number(s) can be found in the article/supplementary material.
DZ: Methodology, Validation, Resources, Writing–review and editing. CT: Conceptualization, Methodology, Writing–original draft. XH: Writing–review and editing.
The author(s) declare that financial support was received for the research, authorship, and/or publication of this article. This study was funded by the Basic Education Research Project of Jiangxi Province (Research on the Reconstruction and Implementation of the Teaching Content of the Compulsory High School Language Curriculum under the New Curriculum Standard. NO:GZYW2024-0478) and Social Science Planning Project of Shanghai (No: 2018BJL005).
The authors would like to thank the editors and reviewers for their helpful comments and suggestions.
The authors declare that the research was conducted in the absence of any commercial or financial relationships that could be construed as a potential conflict of interest.
The author(s) declare that no Generative AI was used in the creation of this manuscript.
All claims expressed in this article are solely those of the authors and do not necessarily represent those of their affiliated organizations, or those of the publisher, the editors and the reviewers. Any product that may be evaluated in this article, or claim that may be made by its manufacturer, is not guaranteed or endorsed by the publisher.
1. Glückler J. Economic geography and the evolution of networks. J Econ Geogr (2007) 7(5):619–34. doi:10.1093/jeg/lbm023
2. Sun D, Lu D, Sun B, Zhang W. From network description to network performance--Preface to the album “Urban network externalities”. Geogr Res (2022) 41(09):2325–9. doi:10.11821/dlyj02022081
3. Sheng Y, Gou Q, Song J. Research on the network structure of innovation linkages and innovation efficiency of urban agglomerations--Taking Beijing-Tianjin-Hebei, Yangtze River Delta and Pearl River Delta urban agglomerations as an example. Scientia Geographica Sinica (2020) 40(11):1831–9. doi:10.11821/dlxb202206005
4. Ma X, Tang C. Spatial linkages and patterns of networked cities in China - a big data analysis based on high-speed rail passenger flow. Econ Geogr (2020) 38(04):55–64. doi:10.15957/j.cnki.jjdl.2018.04.007
5. Ma XLR. Spatial linkage structure of networked cities in China - a study based on bank outlet data. Adv Geosci (2017) 36(04):393–403. doi:10.18306/dlkxjz.2017.04.001
6. Zhao Y, Gao X, Jiang B. Hierarchical structure of network links in Baidu index of cities in three northeastern provinces. Econ Geogr (2015) 35(05):32–7. doi:10.15957/j.cnki.jjdl.2015.05.005
7. Jin X, Hu G, Ding H, Ye S, Lin J. Evolution of spatial structure patterns of urban network in the Yangtze River Economic Belt from the perspective of corporate pledge linkage. Growth and Change (2020) 3(51):833–51. doi:10.1111/grow.12371
8. Bai X, Wu J, Liu Y, Yang Y, Wang M. Research on the impact of global innovation network on corporate performance. Technology Anal and Strateg Management (2021) 34(5):518–34. doi:10.1080/09537325.2021.1912317
9. Breschi S, Lissoni F. Mobility of skilled workers and co-invention networks: An anatomy of localized knowledge flows. Journal of Economic Geography (2009) 9:439–468. doi:10.1093/jeg/lbp008
10. Sun D, Yuan Y. Collaborative innovation and regional industrial upgrading under the perspective of spatial spillover. Stat Res (2019) 36(10):100–14. doi:10.19343/j.cnki.11-1302/c.2019.10.008
11. Lu J, Mao WF. The rise of urban network externalities: a new mechanism for high-quality integrated development of regional economy. The Economist (2020)(12) 62–70. doi:10.16158/j.cnki.51-1312/f.2020.12.007
12. Zhong Z, Wang Z. Diffusion of innovation and optimization of global industrial structure - a study based on Agent simulation. Sci Res (2017) 35(04):611–24. doi:10.16192/j.cnki.1003-2053.2017.04.014
13. Duan D, Du D, Grimes S. The faster the better? Economic effects of the speed of inter-city technology transfer in China. Growth and Change (2019) 50(9):1085–101. doi:10.1111/grow.12309
14. Liu C, Niu C. Spatial evolution and influencing factors of inter-city technology transfer networks in three northeastern provinces. J Geogr (2019) 74(10):2092–107. doi:10.11821/dlxb201910010
15. Zheng J, Sun D, Dai W, Shi L. Multi-polarization variation in the spatial structure of global invention talent mobility network. Finance Trade Econ (2023) 44(10):109–25. doi:10.19795/j.cnki.cn11-1166/f.20231010.001
16. Ma H, Huang X, Li Y. Evolutionary process and mechanism of knowledge polycenters in the city clusters of Guangdong, Hong Kong and Macao Greater Bay Area. J Geogr (2018) 73(12):2297–314. doi:10.11821/dlxb201812003
17. Qi W, Li Q. Study on the dynamic evolution characteristics and influencing factors of global new energy vehicle trade network. World Geogr Res (2024) 33(02):1–14. doi:10.3969/j.issn.1004-9479.2024.02.20220415
18. Wang C, Wang L, Xue Y, Li R. Revealing spatial spillover effect in high-tech industry agglomeration from a high-skilled labor flow network perspective. J Syst Sci Complex (2022) 35(05):839–59. doi:10.1007/s11424-022-1056-1
19. Ma H. Urban relationship construction and innovation network simulation in knowledge flow space. J Geogr (2020) 75(04):708–21. doi:10.11821/dlxb202004004
20. Duan D, Chen Y, Du D. Research on regional integration development of China's three major city clusters under the perspective of technology transfer. Scientia Geographica Sinica (2019) 39(10):1581–91. doi:10.13249/j.cnki.sgs.2019.10.007
21. Dahesh MB, Tabarsa G, Zandieh M, Hamidizadeh M. Reviewing the intellectual structure and evolution of the innovation systems approach. A social network analysis. Technology Soc (2020) 63(05):101399. doi:10.1016/j.techsoc.2020.101399
22. Tang C, Guan M, Dou J. Understanding the impact of High Speed Railway on urban innovation performance from the perspective of agglomeration externalities and network externalities. Technology Soc (2021) 67(9):101760. doi:10.1016/j.techsoc.2021.101760
23. Xie Q, Su J. The spatial-temporal complexity and dynamics of research collaboration: evidence from 297 cities in China (1985- 2016). Technol Forecast Soc Change (2021) 162(07):120390. doi:10.1016/j.techfore.2020.120390
24. Yang L, Zeng D, Zou S, Zhao S. Scientific cooperation networks, knowledge diversity and firms' technological innovation performance. Sci Res (2021) 39(05):867–75. doi:10.16192/j.cnki.1003-2053.20201204.002
25. Amoroso S, Diodato D, Hall BH, Moncada-Paternò-Castello P. Technological relatedness and industrial transformation. J Technol Transf (2023) 48(6):469–75. doi:10.1007/s10961-022-09941-1
26. Fan F, Lian H, Wang S. Can regional collaborative innovation improve innovation efficiency? An empirical study of Chinese cities. Growth and Change (2020) 51(6):440–63. doi:10.1111/grow.12346
27. Wang Y, Wang C. Urban innovation linkage network and radiation ability in Yangtze River Delta. Economic Geography (2018) 38(09):130–137. doi:10.15957/j.cnki.jjdl.2018.09.015
28. Li R, Liang J, Cheng C, Zhang X, Zhao L, Zhao C, et al. The evolution of k-shell in syndication networks reveals financial performance of venture capital institutions. Social Networks (2024) 76(1):191–202. doi:10.1016/j.socnet.2023.09.006
29. Opsahl T, Agneessens F, Skvoretz J. Node centrality in weighted networks: generalizing degree and shortest paths. Soc Networks (2010) 32(3):245–51. doi:10.1016/j.socnet.2010.03.006
30. Antonio A, Gianmaria M, Flavio P, Davide S. Air connectivity and spatial effects: regional differences in Europe. Reg Stud (2020) 33(06):1748–60. doi:10.1080/00343404.2020.1768231
31. Fu S, Ma Z, Ni B, Peng J, Zhang L, Fu Q. Research on the spatial differences of pollution-intensive industry transfer under the environmental regulation in China. Ecol Indicators (2021) 129(07):107921. doi:10.1016/j.ecolind.2021.107921
32. Zhang W, Derudder B, Liu X, Sun B, Wang Y. Defining “centres” in analyses of polycentric urban regions: the case of the Yangtze River Delta. Reg Stud (2021) 25(06):87–98. doi:10.1080/00343404.2021.1912725
33. Wang J, Liu B. Environmental regulation and firms’ total factor productivity—an empirical analysis based on data from Chinese industrial firms. China Ind Economy (2014) 33(03):44–56. doi:10.19581/j.cnki.ciejournal.2014.03.004
34. Shao S, Fan M, Yang L. Economic structural adjustment, green technology progress and China's low-carbon transition development-an empirical investigation based on the perspective of overall technological frontier and spatial spillover effects. Management World (2022) 38(02):46–69. doi:10.3969/j.issn.1002-5502.2022.02.005
35. Shi D, Shang F, Chen B, Expert P, Lü L, Eugene H, et al. Local dominance unveils clusters in networks. Commun Phys (2024) 170(5):170–12. doi:10.1038/s42005-024-016354
Keywords: innovation network, network effect, knowledge spillover, green economic performance, social network analysis
Citation: Zhao D, Tang C and Huang X (2025) Innovation network, knowledge spillover, and urban green economic performance. Front. Phys. 13:1537110. doi: 10.3389/fphy.2025.1537110
Received: 30 November 2024; Accepted: 10 February 2025;
Published: 26 February 2025.
Edited by:
Michele Bellingeri, University of Parma, ItalyReviewed by:
Francesco Scotognella, Polytechnic University of Turin, ItalyCopyright © 2025 Zhao, Tang and Huang. This is an open-access article distributed under the terms of the Creative Commons Attribution License (CC BY). The use, distribution or reproduction in other forums is permitted, provided the original author(s) and the copyright owner(s) are credited and that the original publication in this journal is cited, in accordance with accepted academic practice. No use, distribution or reproduction is permitted which does not comply with these terms.
*Correspondence: Chenghui Tang, MjY1OTMwNjU0NUBxcS5jb20=
Disclaimer: All claims expressed in this article are solely those of the authors and do not necessarily represent those of their affiliated organizations, or those of the publisher, the editors and the reviewers. Any product that may be evaluated in this article or claim that may be made by its manufacturer is not guaranteed or endorsed by the publisher.
Research integrity at Frontiers
Learn more about the work of our research integrity team to safeguard the quality of each article we publish.