- Department of Medical Equipment, Electronic and Information Technologies in Healthcare, Medical University of Varna, Varna, Bulgaria
Introduction: The development and optimization of novel diagnostic imaging prototypes heavily rely on experimental work. In radiology, this experimental work involves the use of phantoms. When testing novel techniques to demonstrate their advantages, anthropomorphic phantoms are utilized. The aim of this study was to investigate seven materials for 3D printing to replicate the radiological properties of breast lesions.
Methods: To achieve this objective, we utilized three fused filament fabrication materials, namely, polylactic acid (PLA), acrylonitrile butadiene styrene (ABS), and polyethylene terephthalate glycol (PET-G), along with resins such as White v4 Resin, Flexible 80A v1 Resin, Model v2 Resin, and Wax40 v1 Resin, to 3D print seven irregularly shaped lesions. These lesions were used to prepare a set of seven physical phantoms, each filled with either water or liquid paraffin, and one of the printed lesions. The phantoms were then scanned using a mammography unit at 28 kVp. Additionally, six computational breast phantoms, replicating the shape of the physical phantoms, were generated. These computational models were assigned the attenuating properties of various breast tissues, including glandular tissue, adipose tissue, skin, and lesions. Mammography images were generated under the same experimental conditions as the physical scans. Both the simulated and experimental images were evaluated for their contrast-to-noise ratio (CNR) and contrast (C).
Discussion: The results indicated that the studied resins and filament-based materials are all suitable for replicating breast lesions. Among these, PLA and White v4 Resin exhibited the densest formations and can effectively approximate breast lesions that are slightly less attenuating than glandular tissue, while ABS and Flexible 80A v1 Resin were the least dense and can represent fat-containing breast lesions. The remaining materials provided good approximations for malignant lesions. These materials can be utilized for constructing phantoms for experimental work, rendering the model a valuable tool for optimizing mammography protocols, ensuring quality control of mammography X-ray equipment, and aiding in the diagnosis and assessment of breast cancer.
1 Introduction
The development and optimization of novel prototypes for diagnostic imaging heavily rely on experimental work. In the field of radiology, this involves extensive X-ray exposures, subsequent image evaluations, and the fine-tuning of X-ray system parameters. In this domain, physical phantoms enable the conduction of unlimited irradiation sessions, offering a robust platform for comprehensive system optimization. In the field of breast imaging, researchers already demonstrated techniques for manufacturing advanced breast models [1–3], used for testing novel imaging techniques, such as tomosynthesis at synchrotron facilities [4], dual-energy, spectral mammography and tomosynthesis [5–10], breast computed tomography [11–13], evaluation of detection performance and novel reconstruction algorithms [4, 14], assessing the image quality of the X-ray breast imaging systems [12, 15–25], and evaluating developed CAD systems [26].
Advanced physical models of the breast not only accurately replicate the anthropomorphic shape of the breast but also simulate specific anatomical structures such as glandular tissue, adipose tissues, and Cooper ligaments, with materials exhibiting X-ray attenuation properties close to the real anatomical tissues [1–3]. Materials approximating glandular tissue using fused filament fabrication (FFF) and stereolithographic (SLA) 3D printers include PLA stone (polylactic acid filled with powdered stone), PLA wood (polylactic acid filled with wood powder), PET-G (polyethylene terephthalate glycol), ASA (acrylonitrile styrene acrylate), PVA (polyvinyl acetate), and EasyWood [27–29], as well as different resins [10, 30, 31]. For simulating adipose tissue, researchers have found acrylonitrile butadiene styrene (ABS)-based filaments, high-impact polystyrene (HIPS)-based filaments, and nylon to be suitable [10, 27, 29, 30, 32], while the skin can be represented by either polyethylene terephthalate (PET) or PLA [33]. By uniquely combining these materials, anthropomorphic phantoms of the breast can be produced [10, 12, 20, 33–35]. A critical component of these models is the tumor representation, where significant effort is dedicated to creating realistic lesion models. This is accomplished at both computational [36–38] and physical levels [15, 20]. Current efforts are focused on the creation of advanced phantoms incorporating lesion models, enhancing their reliability to real situations.
Realistic breast lesions can be derived through various mathematical approaches, or by segmenting lesions from three-dimensional imaging modalities, such as CT and magnetic resonance imaging (MRI) [38, 39]. These advancements may be exploited to manufacture realistic breast lesions and, thus, can contribute to the creation of sophisticated anthropomorphic breast phantoms; however, this requires research in both appropriate materials and printing technologies [24, 40].
Studies have focused on investigating the radiological properties of the available 3D printing materials [27, 30, 31, 41]. These studies serve as a foundation for efforts directed at mimicking the radiological characteristics of physically fabricated lesions [14, 20, 24, 40]. For instance, [14] proposed irregularly printed lesions from four resin types, using SLA technology, the performance of which was tested under an automatic exposure control (AEC) setting at clinical mammography and tomosynthesis units. [40] proposed a method for the production of realistic lesions based on FFF technology, tested for CT imaging. The materials used included ASA, PLA, nylon, and PET-G with corresponding densities of 1.08 (g/cm3), 1.29 (g/cm3), 1.18 (g/cm3), and 1.30 (g/cm3). By varying the infill ratio during modeling and printing, they achieved different Hounsfield units (HU), effectively replicating lesions with variable densities. Spheres with diameters of 3.2 mm and 4.8 mm, representing low-contrast abnormalities, were used in an experimental study carried out at a non-mammographic imaging setup with a 55-kVp X-ray beam by [10]. Recently, [15] used polytetrafluorethylene (PTFE) ellipsoids as a tissue substitute for masses and soybean for lesions. These models were integrated into a phantom, imaged at several clinical breast imaging systems. [16] used a mixture of acrylic resin and calcium bicarbonate to represent the spherical (regular) and amorphous (irregular) mass structures, respectively. These were incorporated into a slab, placed in a phantom, and imaged using a clinical mammography unit configured in the AEC mode. The incident beam was set to 28 kVp, utilizing a tungsten/rhodium anode/filter combination.
This study builds on extensive prior research by further exploring materials used with 3D printing that replicate the radiological properties of lesions. It extends this research by producing lesions with irregular shapes, incorporated into phantoms, imaged under clinical mammographic conditions. It uses modeling, simulation techniques, and experimental approaches to investigate and advance the understanding of these materials. The resulting breast phantoms are specifically designed for comparative studies, evaluating the performance of a prototype X-ray breast system under development against commercial mammography systems.
2 Materials and methods
Figure 1 summarizes the process of simulation and production. Initially, the 3D lesion model and the breast shape in the form of a semi-cylinder are computationally developed. Six computational breast phantoms were created, approximating compressed breasts of different densities. These models were subjected to mammography simulations. Then, the computational lesion model and the breast shape were converted to stl format and printed with both FFF and SLA printers. The printed lesions were inserted into the breast shape and imaged at a mammography unit.
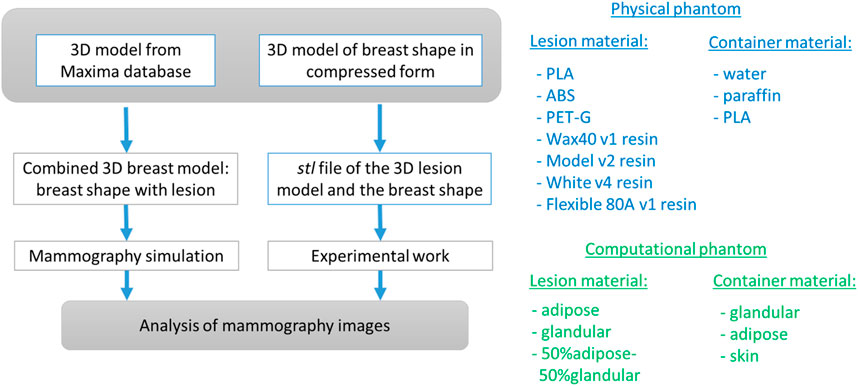
Figure 1. Overall approach of the study. The breast lesion model is taken from the Maxima database [36].
2.1 Creation of the computational model and images
The computational breast phantom is designed from 3D geometrical primitives, as shown in Figure 2. XRAYImagingSimulator was used, which is a computational software program for modeling and simulations in X-ray imaging [42]. This application can create computational models of any object with simple and complex shapes, to assign material properties, and generate X-ray images based on various acquisition geometries.
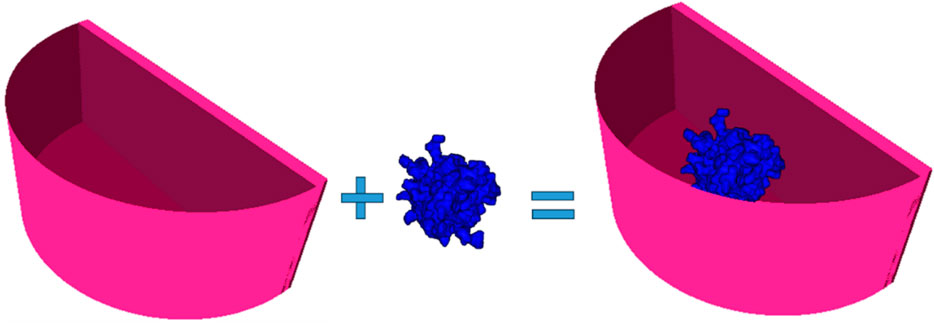
Figure 2. Created computational breast phantom by using XRayImagingSimulator [42]: a semi-cylindrical container housing a lesion composed of specific breast tissue. The phantom is filled with either adipose tissue, glandular tissue, or 50% adipose–50% glandular tissue mixture.
Two main computational objects were separately created: one resembling a semi-cylindrical container, representing the shape of a breast under compression during mammography imaging, and the other mimicking a breast lesion. The first component of this phantom, the breast shape, was modeled as a semi-cylinder with a thickness of 50 mm. The skin shape is obtained by the difference of two semi-cylinders with the same height of 50 mm and a base of 60 mm and 55 mm, respectively. Thus, the resulted skin thickness is 5 mm, with assigned elemental composition of the skin. The intersection of the semi-cylinders is filled with a homogeneous material, corresponding to adipose tissue, glandular tissue, and 50% adipose–50% glandular tissues. The characteristics of the simulated breast tissues are listed in Table 1. Data on the density and chemical composition were obtained from [30].
The computational model of the breast lesion was chosen from the Maxima database [36]. The irregular-shaped lesion was placed in a matrix with a size of 100 × 100 × 100 pixels, each pixel with a size of 0.2 mm × 0.2 mm × 0.2 mm. The lesion was created by initially using Brownian motion, followed by a low-frequency filter to smooth the shape. Furthermore, a procedure was created to insert the computational lesion model into the center of the modeled container. The complete phantom was voxelized and used in a simulation experiment, which included three breast glandularities and three types of breast lesions, each differing in their chemical composition. The outputs of this simulation are X-ray images of the computational phantoms.
The geometry used in the simulations is shown in Figure 3.
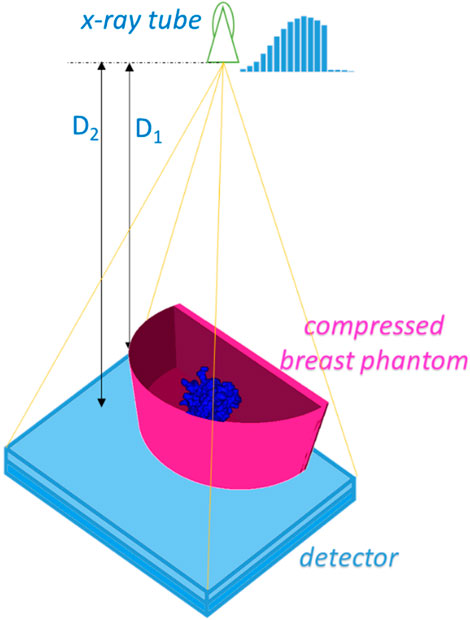
Figure 3. Simulated X-ray mammography geometry. D1 and D2 are the distances from the X-ray tube to the center of rotation and to the detector surface and were set to 600 mm and 650 mm, respectively.
The simulation is implemented using XRAYImagingSimulator [42] and includes the generation of X-ray images of the six phantoms for the mammography setup. The generated images are represented as a matrix with values corresponding to the evaluation of the line integrals calculated for each X-ray emerging from the X-ray tube toward each detector pixel. The exploited relationship is the Beer–Lambert law (Equation 1), reflecting the attenuation of the X-rays while passing the phantom and the properties of the objects in that phantom.
In this equation, I0 represents the intensity of the initial X-ray beam, while I denotes the intensity of the beam detected after traversing a path of length l through a material with a linear attenuation coefficient μ(E). Images had a size of 2,000 pixels × 2,000 pixels, with a pixel size of 0.1 mm × 0.1 mm. The images were generated for distances, 600 mm and 650 mm, which correspond to the distances from the source to the center of rotation and from the source to the detector plate, respectively. One image per phantom was generated with these settings, resulting in six X-ray images, which were subjected to evaluation. The simulated X-ray spectrum was used in the experimental work, i.e., 28 kVp with a W/Rh anode/filter combination. Quantum and detector noise was not simulated.
2.2 Printed models and experimental images
The computational lesion model shown in Figure 4A is used with ImageJ [43] to obtain its stl version, as shown in Figure 4B, which was then used for 3D printing. The model was printed using two 3D printers, namely, the Formlabs Form 3 printer (Formlabs, Somerville, Massachusetts), which is SLA-based, and the Raise3D Pro3 Plus printer (Raise3D, Costa Mesa, California), which is FFF-based. Seven physical lesion models were produced using four different resins: Wax40 v1 Resin, Model v2 Resin, White v4 Resin, and Flexible 80A v1 Resin. Additionally, three filament materials were used: PLA, ABS, and PET-G. These materials are summarized in Table 2.
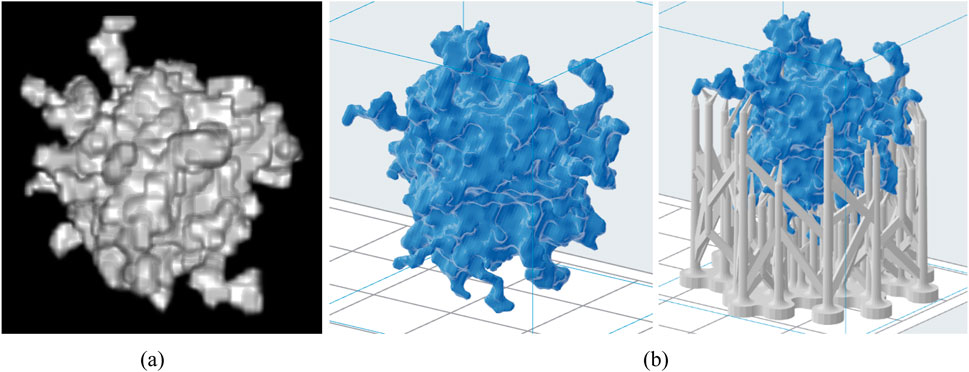
Figure 4. Computational lesion model (A) and the respective stl models without and with support structures (B).
Figure 5 shows the 3D-printed tumor models and the corresponding materials used.
The printed lesion models were placed in a container composed of two semi-cylinders (Figure 6A). The white and black containers were printed from PLA. The thickness of the white container was 3 mm. The black container was an envelope, with a thickness of 2 mm, and when combined with the white container, a breast skin thickness of 5 mm can be obtained. Then, the container was used to prepare 14 breast phantoms: 7 breast phantoms with water and 7 breast phantoms with liquid paraffin, each one scanned using a mammography imaging system, as shown in Figures 6B, C.
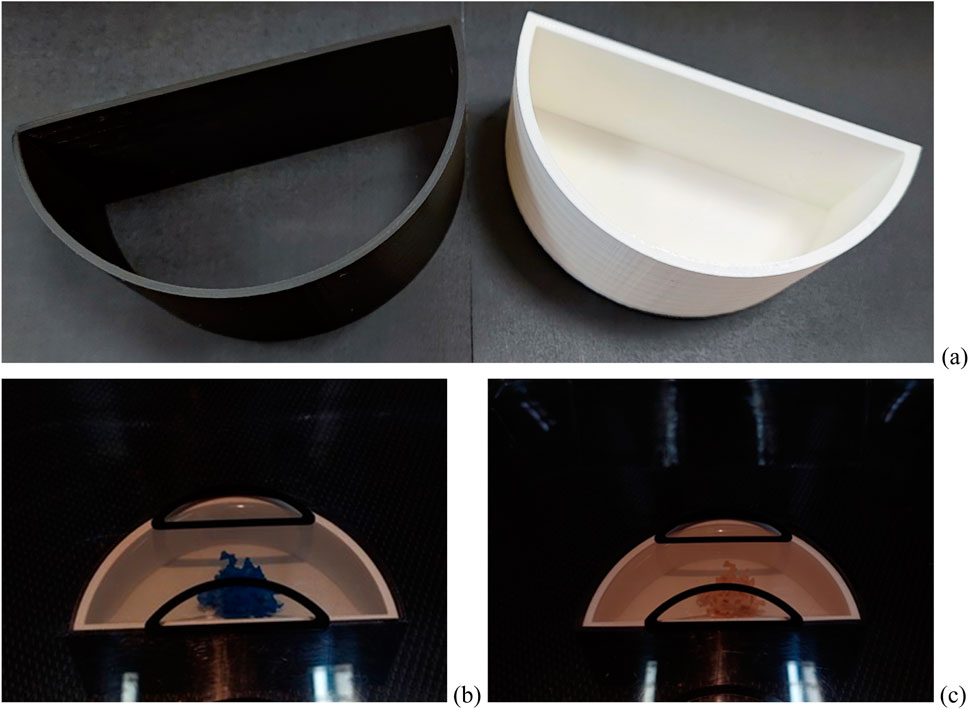
Figure 6. Phantom components: (A) components of the skin: white container—printed from PLA simulating a compressed breast—and black component—printed from PLA simulating breast skin; (B and C) physical phantoms with lesion formations placed in water/paraffin on a mammography machine.
The experiments were conducted on a Siemens Mammomat mammography system, operated in the AEC mode, allowing the radiographer to manually select the kVp for obtaining X-ray mammographic images. The anode voltage was set to 28 kVp, and the used anode/filter combination was W/Rh. Fourteen experiments were performed corresponding to the number of phantoms. Each lesion phantom was imaged five times, and the contrast and contrast-to-noise calculations were presented as average values. In this way, the resultant image was obtained with decreased quantum noise.
2.3 Quantitative evaluation of models
The processing of the X-ray images includes the calculation of two parameters, the contrast of the lesion, contrast (Clesion), and the contrast-to-noise ratio (CNRlesion), calculated as suggested by [44]. These two features provide quantitative measures of the visibility and detectability of different materials used in printing lesion models, similar to the approach of other research groups exploiting in their research anthropomorphic physical phantoms [45–47]. By keeping kVp constant, variations in the CNR and contrast directly reflect differences in the phantoms themselves rather than changes in the imaging setup. Additionally, we attempted to position the lesions in the same location for each experiment in order to ensure that the comparison is fair and that any observed differences are attributable to the characteristics of the phantoms.
The following areas on the mammographic images are defined, in which the mean and the standard deviation were measured in order to calculate C and CNR:
where Imlesion is the average pixel intensity value in the region of the lesion (ROIlesion), Imbg is the average pixel intensity value in the background region of interest (ROIbackground), and σbg and σlesion are the standard deviations of the background and the lesion pixel intensity values, respectively. The ROIlesion area is of size 100 pixels × 100 pixels, while ROIbackground is defined as an area of 250 pixels × 200 pixels. The CNR for the simulated lesion is calculated by a slightly different formula, as suggested by Equation 3, imposed by the fact that the images are free of noise. The ImageJ application was used for this purpose.
3 Results and discussion
3.1 Simulation study
Simulated X-ray images of the phantoms with the lesion made from different percentage ratios between the glandular and adipose tissues are shown in Figure 7. These phantoms represent scenarios obtained from the literature, based on both simulated and patient data. Specifically, the breast density and the attenuation of the breast mass lesion were obtained from several research studies [38, 39, 48–50].
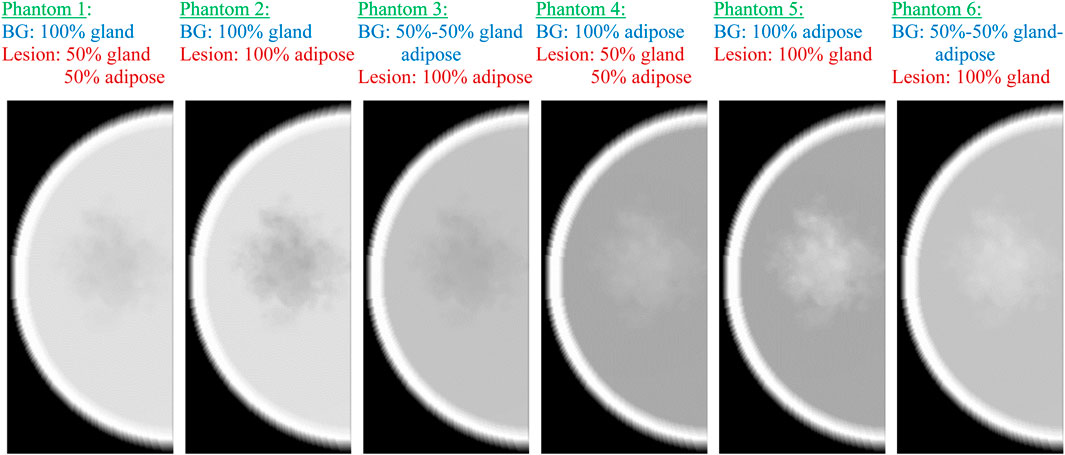
Figure 7. Simulated mammography images of the six computational phantoms, replicating different breast composition scenarios. BG, background.
In phantoms 1 and 2, the breast density is 100%, resulting in a lesion appearing as lower-density regions due to their lower attenuation coefficient than that of glandular tissue. The optimal differentiation between the lesion and the background tissue occurs when the lesion has an attenuation coefficient similar to glandular tissue and the surrounding tissue is entirely adipose, as observed in phantom 5, which simulates the breast tissue composition in women with fatty breasts [51]. This is well-supported by the quantitative results given in Table 3, demonstrating both maximum contrast and CNR (calculated by Equations 2, 3) for phantom 5, which represents a combination of fatty background and 100% glandular lesion. Thus, this phantom is suitable for representing the fatty breasts in experimental work.
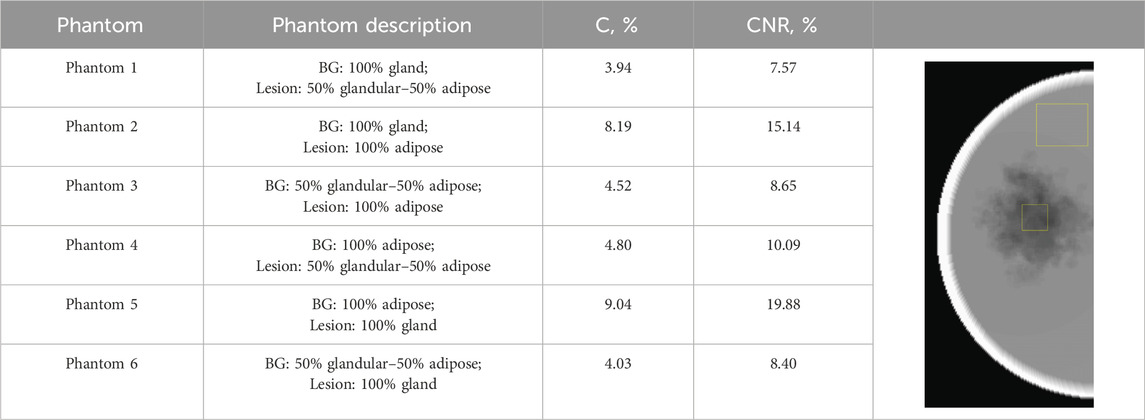
Table 3. Calculated CNR and C parameters for the images of the six computational phantoms. The image shows the region of interest used in the calculation of these parameters.
The lowest CNR and contrast are observed for phantom 1, where the lesion is modeled as a combination of 50% gland–50% adipose tissue inserted in a 100% glandular tissue, simulating the case of a 100% dense breast. However, this case is not very realistic. [52] reported that the mean compositions, expressed as percent fibroglandular tissue with the skin, range from 13.7% to 25.6% among various breast type groups. Despite this, some investigators still use breast models with glandularity of up to 75% [49] and 100% [48] in their experimental work. [39] modeled breast lesions with 86% of the absorption of glandular tissue, while [50] modeled the attenuation of the computational irregular mass to be equal to that of glandular tissue. This instantly shows that the configurations of phantom 2 and phantom 3 are not realistic phantom combinations for implementation in experimental work.
3.2 Experimental study
Each physical phantom was imaged five times, with input parameters recorded for each session, and is shown in Table 4.
Figure 8 presents the mammographic images of the imaged physical phantoms: the images in the upper row correspond to phantoms filled with liquid paraffin (fatty breasts), while the images in the lower (second) row correspond to mammographic images of physical phantoms filled with water (dense breasts).
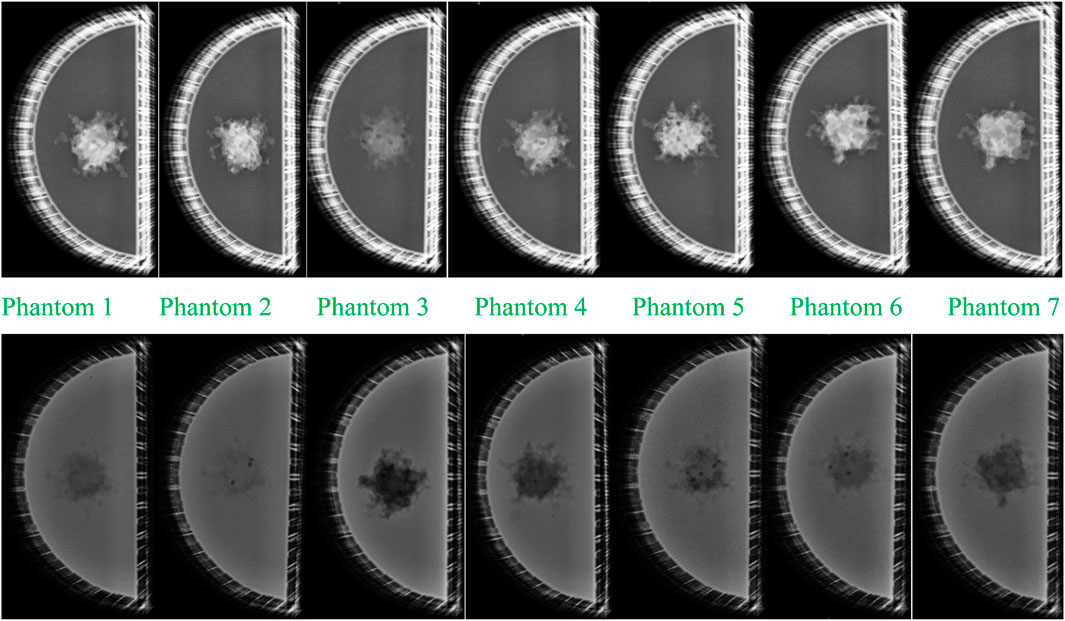
Figure 8. Mammographic images of the imaged physical phantoms: upper row: images of the seven phantoms with liquid paraffin; lower row: images of the seven phantoms with water. Images 1–7 correspond to images of phantom 1 with lesion from White v4 Resin; phantom 2 with lesion from PLA; phantom 3 with lesion from ABS; phantom 4 with lesion from Flexible 80A v1 Resin; phantom 5 with lesion from PET-G; phantom 6 with lesion from Model v2 Resin; phantom 7 with lesion from Wax40 v1 Resin.
The comparison of the mammograms of the printed tumor formations shows that all the materials from which the lesions are printed are denser than paraffin. Of the materials from which the tumor formations are made, ABS (phantom 3) is closest in density to that of paraffin, which is visually demonstrated by the apparent small contrast. All other materials, used for the tumor representation, appear on the mammograms denser than the paraffin, demonstrated visually by the greater contrast. The visual assessment shows that Model v2 Resin (phantom 6), PET-G (phantom 5), White v4 Resin (phantom 1), and PLA (phantom 2) demonstrate greater contrast regarding the paraffin background than Flexible 80A v1 Resin (phantom 4) and Wax40 v1 Resin (phantom 7). This is a logical output, taking into account the difference in the densities of these two models regarding the denser materials.
Mammographic images depicting lesions in water (simulating denser breast) revealed that the PLA-based lesion (phantom 2) exhibits the closest density resemblance to water, evident in the minimal contrast observed between the two materials in Figure 8. ABS (phantom 3) exhibits the most substantial contrast with water, supporting its status as the least dense material among those investigated, as discussed in the preceding paragraph. The visual assessment further emphasizes that Model v2 Resin, PET-G, White v4 Resin, and PLA exhibit higher densities than Flexible 80A v1 Resin and Wax40 v1 Resin, as indicated by their lower contrast than that of water. All dark spherical spots are observed in the lesion formations themselves, caused by air voids, which may arise during 3D printing.
Objective evaluation was implemented using ImageJ [43], using a custom macro that was created to calculate both the object and background averages. This macro was applied to all five images within a given imaging set, generating regions of interest (ROIs) as depicted shown in Table 5. In the same table, the measured mean pixel intensity gray values of the ROIs are reported.
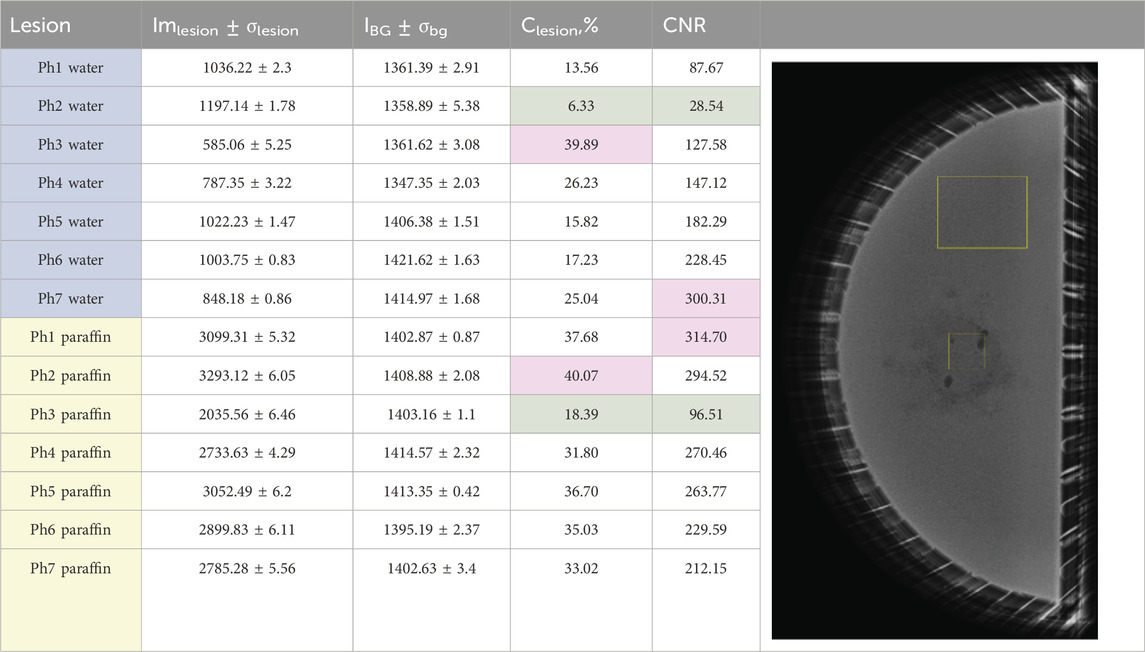
Table 5. Measured pixel intensity gray value data. The first seven phantoms Ph1–Ph7 are water-based, and the rest are liquid paraffin-based. The regions of interest (ROIs) on the image show the ROIs for object and background for the calculation of measured parameters with ImageJ.
The analysis of the X-ray images of the water-based phantoms shows that the lowest Clesion and CNR are observed for physical phantom 2, where the lesion is printed from PLA. This is clearly visualized in the mammographic image of the phantom, shown in the second row of Figure 8. The low CNR is due to the low contrast of the object in this case. This result also compares well to the simulated result of computational phantom 1, representing a 100% glandular background with a lesion made up of 50% gland and 50% adipose tissue, although this is not a realistic scenario according to the findings obtained by [52].
The maximum Clesion was observed for phantom 3, where the lesion was 3D-printed from ABS, which was expected, given the lower density of this material than that of water. This result associates well with the simulated image of computational phantom 2, composed of 100% dense breast tissue with a lesion represented by adipose tissue. Although a 100% glandular breast is not a realistic breast, an adipose breast lesion can represent a fat-containing breast lesion, and this may be useful in virtual investigations with other backgrounds. Finally, the maximum CNR was reported for physical phantom 7, with a lesion produced from Wax40 v1 Resin. The image of this phantom is characterized by higher lesion contrast and lower noise. This advantage of printing with this material must be taken into account when producing noise-reduced images of breast phantoms.
The analysis of the liquid paraffin phantoms showed that the highest contrast was observed for physical phantom 2, where the lesion was printed from PLA. This is clearly visualized in the mammographic image of the phantom shown in the first row of Figure 8. This result aligns well with the performance of computational phantom 5, which represents a fatty breast with a lesion composed of 100% glandular tissue, a realistic breast composition and imaging scenario. The maximum CNR was reported for physical phantom 1, with the lesion printed from White v4 Resin. This model is characterized by high contrast and low noise, leading to an increased CNR. The lesion produced with this resin had fewer air voids, resulting in lower noise levels.
Minimal contrast and CNR were observed for physical phantom 3, where the lesion was 3D-printed from ABS. This outcome is expected, given that ABS has a density close to that of paraffin. This result compares well to computational phantom 4, which represents a fatty breast with a lesion composed of 50% gland and 50% adipose tissue, another realistic breast composition and imaging scenario.
The mean intensities of the tumor entities are compared in Table 5. The single ANOVA test (data available on Zenodo) shows that there is a difference in the measured background values, which is explained with the variability of the input parameters. The X-ray images of the phantoms were taken on 4 different days within 2 consequent months, which may have led to variations in the positioning of some of the phantoms. For instance, the first three phantoms, phantom 1, phantom 2, and phantom 3, showed no significant differences in their intensity background values as they were scanned on the same day without being moved from the mammography units, with only the lesion models being changed. Additionally, slight variations in the water quantity within the phantoms could result in differences in pixel grayscale values. [53] demonstrated in an experimental study that under constant kVp and target/filter material of the mammography X-ray tube, even a 5-mm difference in phantom thickness leads to increased mAs and average glandular dose.
AEC in mammography systems establishes the optimal exposure conditions required to achieve the desired image quality based on the absorption characteristics of compressed breast tissue [54]. Studies have shown that under the same target/filter configuration, different AEC options tend to converge as the thickness increases, indicating that the benefits of the effective energy downshift in the incident beam are overwhelmed by the beam-hardening effect [55]. On the other hand, the modified AEC may be more effective than the default AEC for a typical breast thickness range of 4.5 cm–6 cm as it improves both the figure of merit and the signal difference-to-noise ratio.
The comparison of the measured mean intensity lesion values shows that the densest formations are PLA and White v4 Resin, and the least dense are ABS and Flexible 80A v1 Resin. Regarding object contrast, physical phantom 3, with an ABS lesion in water, exhibits the highest contrast, while physical phantom 2, with a PLA lesion in water, shows the lowest contrast. This results in minimal brightness variation across different parts of the image, creating a softer, more natural-looking appearance.
All resins used in this study demonstrate suitable radiological properties, making any of them appropriate for representing breast lesions. The most challenging scenario for screening is the PLA lesion in water, which reflects a dense breast with a lesion. However, this is not a realistic case.
Research on materials for replicating the radiological properties of the human tissues confirmed that ABS and nylon may be successfully used to replicate the adipose tissue, while 100% PLA is good in replicating soft tissues [10, 29]. The results of our experimental study indicate that all tested materials are suitable for replicating breast lesions, depending on the specific type of lesion desired for simulation. This conclusion is well-supported by the results of several experimental studies. For instance, [30] demonstrated that for mammography purposes, resins and PET-G are suitable for representing properties of breast mass when the mass absorption is lower than that of glandular tissue, as suggested by [39]. [16] used a mixture of acrylic resin and calcium bicarbonate to represent amorphous breast lesion structures in a new physical breast phantom. Furthermore, [15] utilized PTFE, soybean, and nylon to represent mass, tumor, and fibroglandular structures in a novel breast phantom designed for digital mammography, contrast-enhanced digital mammography, and digital breast tomosynthesis. Their use is also based on experimental data obtained from [30] and the theoretical study by [56]. Additionally, PLA was used by [20] in preparing lesion phantoms for mammography and tomosynthesis studies.
During experimentation, we faced various problems with the materials used for replicating the lesion and the used background. These are summarized in Table 6 as most of the problems were due to presence of air voids. In the case of PET-G, the visible air voids on the mammography image may be due to entrapped air during 3D printing and surface porosity, which is valid for many polymer materials. In addition, PET-G is hygroscopic, similar to PLA, and absorbs moisture from the environment. If the material has absorbed moisture, the heat during printing can cause the moisture to vaporize, leading to the formation of air voids [57]. Therefore, the calculated values in this study reflect not only the material properties but also the shape and orientation of the individual lesion and the specifics of the printing and filling processes. For instance, during the cooling process, ABS may experience issues such as shrinkage and warping [58], which may result in a slightly smaller shape of the printed lesion. Furthermore, PLA is reported to absorb approximately 1 wt% moisture at 21 °C within 1 day of printing, and this level remains relatively constant for the next 10 days [59]. However, this moisture absorption could potentially alter the composition of the printed lesions and consequently affect the absorption properties of the material.
Other limitations to this study include the challenge of consistently positioning the tumors within the phantom and placing the phantom itself in the same mammography position. Variability in placement was unavoidable due to the difficulty in precisely locating the lesions in water and paraffin.
4 Conclusion
This study focused on investigating seven materials used in 3D printing, alongside simulations. The modeled lesions served as the basis for constructing 3D physical breast phantom configurations, which were analyzed in parallel with simulations. The results demonstrated that all the studied materials are suitable for breast phantoms containing lesions. The densest materials were PLA and White v4 Resin, while the least dense materials were ABS and Flexible 80A v1 Resin. Wax40 v1 Resin and White v4 Resin exhibited less noise due to the absence of air voids, a feature that will be beneficial in future heterogeneous breast models. However, taking into account the challenges during the experimental work, the best material for representing low- and high-contrast lesions turned out to be the resins, such as Flexible 80A v1 Resin and White v4 Resin. The findings will be utilized in creating a mammary tumor phantom and producing realistic X-ray images that closely resemble patient mammograms of breast carcinoma. This makes the model a valuable tool for optimizing mammography protocols, performing quality control of mammography X-ray equipment, and aiding in the diagnosis and assessment of breast cancer.
Data availability statement
The original contributions presented in the study are publicly available. This data can be found here: Zenodo repository, https://zenodo.org/records/12737920.
Author contributions
KB: conceptualization, data curation, formal analysis, funding acquisition, investigation, methodology, resources, software, supervision, validation, visualization, writing–original draft, and writing–review and editing. ND: conceptualization, data curation, investigation, methodology, resources, software, visualization, and writing–review and editing. OT-V: data curation, formal analysis, investigation, methodology, resources, visualization, and writing–review and editing. ZB: methodology, project administration, resources, and writing–review and editing.
Funding
The author(s) declare that financial support was received for the research, authorship, and/or publication of this article. This study is financed by the European Union NextGenerationEU, through the National Recovery and Resilience Plan of the Republic of Bulgaria, project no. BG-RRP-2.004-0009. The work was implemented by the ELPIDA research group, part of the MUVE-TEAM project.
Conflict of interest
The authors declare that the research was conducted in the absence of any commercial or financial relationships that could be construed as a potential conflict of interest.
Publisher’s note
All claims expressed in this article are solely those of the authors and do not necessarily represent those of their affiliated organizations, or those of the publisher, the editors, and the reviewers. Any product that may be evaluated in this article, or claim that may be made by its manufacturer, is not guaranteed or endorsed by the publisher.
References
1. Glick S. J., Ikejimba L. C. Advances in digital and physical anthropomorphic breast phantoms for x-ray imaging. Med Phys (2018) 45:e870–85. doi:10.1002/mp.13110
2. Sarno A., Valero C., Tucciariello R. M., Dukov N., Costa P. R., Tomal A. Physical and digital phantoms for 2D and 3D x-ray breast imaging: review on the state-of-the-art and future prospects. Radiat Phys Chem (2023) 204:110715. doi:10.1016/j.radphyschem.2022.110715
3. Bliznakova K., The advent of anthropomorphic three-dimensional breast phantoms for X-ray imaging. Phys Med (2020) 79:145–61. doi:10.1016/j.ejmp.2020.11.025
4. Bliznakova K., Kolitsi Z., Speller R. D., Horrocks J. A., Tromba G., Pallikarakis N. Evaluation of digital breast tomosynthesis reconstruction algorithms using synchrotron radiation in standard geometry. Med Phys (2010) 37:1893–903. doi:10.1118/1.3371693
5. Carton A. K., Bakic P., Ullberg C., Derand H., Maidment A. D. A., Development of a physical 3D anthropomorphic breast phantom. Med Phys (2011) 38:891–6. doi:10.1118/1.3533896
6. Koukou V., Martini N., Fountos G., Michail C., Sotiropoulou P., Bakas A, et al. Dual energy subtraction method for breast calcification imaging. Nucl Instrum Methods Phys Res Sect A (2017) 848:31–8. doi:10.1016/j.nima.2016.12.034
7. Koukou V., Martini N., Velissarakos K., Gkremos D., Fountzoula C., Bakas A., et al. PVAL breast phantom for dual energy calcification detection. In: International conference on bio-medical instrumentation and related engineering and physical sciences (biomep 2015) (2015). p. 637. doi:10.1088/1742-6596/637/1/012013
8. Badal A., Clark M., Ghammraoui B., Reproducing two-dimensional mammograms with three-dimensional printed phantoms. J Med Imaging (2018) 5:1. doi:10.1117/1.jmi.5.3.033501
9. Clark M., Ghammraoui B., Badal A., Reproducing 2D breast mammography images with 3D printed phantoms. Med Imaging 2016: Phys Med Imaging (2016) 9783. doi:10.1117/12.2217215
10. Malliori A, Daskalaki A, Dermitzakis A, Pallikarakis N. Development of physical breast phantoms for x-ray imaging employing 3D printing techniques. Open Med Imaging J (2020) 12:1–10. doi:10.2174/1874347102012010001
11. Salad J., Ikejimba L. C., Makeev A., Graff C. G., Ghammraoui B., Glick S. J., Development of an anthropomorphic breast phantom for objective task-based assessment of dedicated breast CT systems (2018) In: 14th international workshop on breast imaging (iwbi 2018). doi:10.1117/12.2318524
12. Kiarashi N., Nolte A. C., Sturgeon G. M., Segars W. P., Ghate S. V., Nolte L. W., et al. Development of realistic physical breast phantoms matched to virtual breast phantoms based on human subject data. Med Phys (2015) 42:4116–26. doi:10.1118/1.4919771
13. di Franco F., Mettivier G., Sarno A., Varallo A., Russo P., Manufacturing of physical breast phantoms with 3D printing technology for X-ray breast imaging. IEEE Nuclear Science Symposium and Medical Imaging Conference (NSS/MIC), Manchester, United Kingdom (2019):1–5. doi:10.1109/NSS/MIC42101.2019.9059986
14. Cockmartin L., Marshall N. W., Zhang G., Lemmens K., Shaheen E., Van Ongeval C., et al. Design and application of a structured phantom for detection performance comparison between breast tomosynthesis and digital mammography. Phys Med Biol (2017) 62:758–80. doi:10.1088/1361-6560/aa5407
15. Zhang C., Fu J., A new breast phantom suitable for digital mammography, contrast-enhanced digital mammography and digital breast tomosynthesis. Phys Med Biol (2023) 68:045015. doi:10.1088/1361-6560/acb636
16. Flores M. B., Moura A. P., del Rio M. C., Breast phantom made of acrylic slabs for tests in mammography DR. Appl Radiat Isotopes (2022) 188:110389. doi:10.1016/j.apradiso.2022.110389
17. Zhao C., Solomon J., Sturgeon G. M., Gehm M. E., Catenacci M., Wiley B. J., et al. Third generation anthropomorphic physical phantom for mammography and DBT: incorporating voxelized 3D printing and uniform chest wall QC region. In: Medical imaging 2017: physics of medical imaging (2017). doi:10.1117/12.2256091
18. Ikejimba L., Farooqui A., Ghazi P., Hyperia: a novel methodology of developing anthropomorphic breast phantoms for X-ray imaging modalities - Part I: concept and initial findings. Med Phys (2023) 50:702–18. doi:10.1002/mp.16045
19. Balta C., Bouwman R. W., Sechopoulos I., Broeders M. J. M., Karssemeijer N., van Engen R. E., et al. A model observer study using acquired mammographic images of an anthropomorphic breast phantom. Med Phys (2018) 45:655–65. doi:10.1002/mp.12703
20. Dukov N., Bliznakova K., Teneva T., Marinov S., Bakic P., Bosmans H., et al. Experimental evaluation of physical breast phantoms for 2D and 3D breast X-ray imaging techniques. In: T Jarm, A Cvetkoska, S. Mahnič-Kalamiza, and D. Miklavcic, editors. IFMBE proceedings. Springer Science and Business Media Deutschland GmbH (2021). p. 544–552. doi:10.1007/978-3-030-64610-3_62
21. Mainprize J. G., Carton A. K., Klausz R., Li Z. J., Hunter D. M., Mawdsley G. E., et al. Development of a physical 3D anthropomorphic breast texture model using selective laser sintering rapid prototype printing. Med Imaging 2018: Phys Med Imaging (2018):10573. doi:10.1117/12.2293560
22. Mainprize J. G., Mawdsley G. E., Carton A. K., Li Z. J., Klausz R., Muller S., et al. Full-size anthropomorphic phantom for 2D and 3D breast x-ray imaging. Proc Spie (2020) 11513. doi:10.1117/12.2560358
23. Rossman A. H., Catenacci M., Zhao C., Sikaria D., Knudsen J. E., Dawes D., et al. Three-dimensionally-printed anthropomorphic physical phantom for mammography and digital breast tomosynthesis with custom materials, lesions, and uniform quality control region. J Med Imaging (2019) 6:1. doi:10.1117/1.jmi.6.2.021604
24. Dukov N., Bliznakova K., Okkalidis N., Teneva T., Encheva E., Bliznakov Z., Thermoplastic 3D printing technology using a single filament for producing realistic patient-derived breast models. Phys Med Biol (2022) 67:045008. doi:10.1088/1361-6560/ac4c30
25. Schopphoven S., Cavael P., Bock K., Fiebich M., Mäder U., Breast phantoms for 2D digital mammography with realistic anatomical structures and attenuation characteristics based on clinical images using 3D printing. Phys Med Biol (2019) 64:215005. doi:10.1088/1361-6560/ab3f6a
26. Sousa M. A. Z., Matheus B. R. N., Schiabel H., Development of a structured breast phantom for evaluating CADe/Dx schemes applied on 2D mammography. Biomed Phys Eng Expr (2018) 4:045018. doi:10.1088/2057-1976/aac2f2
27. Ma X., Buschmann M., Unger E., Homolka P., Classification of X-ray attenuation properties of additive manufacturing and 3D printing materials using computed tomography from 70 to 140 kVp. Front Bioeng Biotechnol (2021) 9:763960. doi:10.3389/fbioe.2021.763960
28. Okkalidis F., Chatzigeorgiou C., Okkalidis N., Dukov N., Milev M., Bliznakov Z., et al. Characterization of commercial and custom-made printing filament materials for Computed Tomography imaging of radiological phantoms. Technologies (2024) 12:139. doi:10.3390/technologies12080139
29. Kunert P., Trinkl S., Giussani A., Reichert D., Brix G., Tissue equivalence of 3D printing materials with respect to attenuation and absorption of X-rays used for diagnostic and interventional imaging. Med Phys (2022) 49:7766–78. doi:10.1002/mp.15987
30. Ivanov D., Bliznakova K., Buliev I., Popov P., Mettivier G., Russo P., et al. Suitability of low density materials for 3D printing of physical breast phantoms. Phys Med Biol (2018) 63:175020. doi:10.1088/1361-6560/aad315
31. Esposito G., Mettivier G., Bliznakova K., Bliznakov Z., Bosmans H., Bravin A., et al. Investigation of the refractive index decrement of 3D printing materials for manufacturing breast phantoms for phase contrast imaging. Phys Med Biol (2019) 64:075008. doi:10.1088/1361-6560/ab0670
32. Solc J., Vrba T., Burianova L., Tissue-equivalence of 3D-printed plastics for medical phantoms in radiology. J Instrumentation (2018) 13:P09018. doi:10.1088/1748-0221/13/09/p09018
33. Varallo A., Sarno A., Castriconi R., Mazzilli A., Loria A., del Vecchio A., et al. Fabrication of 3D printed patient-derived anthropomorphic breast phantoms for mammography and digital breast tomosynthesis: imaging assessment with clinical X-ray spectra. Phys Med (2022) 98:88–97. doi:10.1016/j.ejmp.2022.04.006
34. Okkalidis N., Bliznakova K., A voxel-by-voxel method for mixing two filaments during a 3D printing process for soft-tissue replication in an anthropomorphic breast phantom. Phys Med Biol (2022) 67:245019. doi:10.1088/1361-6560/aca640
35. Feradov F., Marinov S., Bliznakova K., Physical breast phantom dedicated for mammography studies. Ifmbe Proc (2020) 76:344–352. doi:10.1007/978-3-030-31635-8_41
36. Bliznakova K., Dukov N., Feradov F., Gospodinova G., Bliznakov Z., Russo P., et al. Development of breast lesions models database. Phys Med (2019) 64:293–303. doi:10.1016/j.ejmp.2019.07.017
37. Dukov N., Bliznakova K., Feradov F., Ridlev I., Bosmans H., Mettivier G., et al. Models of breast lesions based on three-dimensional X-ray breast images. Phys Med (2019) 57:80–7. doi:10.1016/j.ejmp.2018.12.012
38. Van Camp A., Houbrechts K., Cockmartin L., Woodruff H. C., Lambin P., Marshall N. W, et al. The creation of breast lesion models for mammographic virtual clinical trials: a topical review. Prog Biomed Eng (2023) 5:012003. doi:10.1088/2516-1091/acc4fc
39. Tomic H., Costa A. C., Bjerkén A., Vieira M. A. C., Zackrisson S., Tingberg A., et al. Simulation of breast lesions based upon fractal Perlin noise. Physica Med (2023) 114:102681. doi:10.1016/j.ejmp.2023.102681
40. Hatamikia S., Gulyas I., Birkfellner W., Kronreif G., Unger A., Oberoi G., et al. Realistic 3D printed CT imaging tumor phantoms for validation of image processing algorithms. Phys Med (2023) 105:102512. doi:10.1016/j.ejmp.2022.102512
41. Dancewicz O. L., Sylvander S. R., Markwell T. S., Crowe S. B., Trapp JV. Radiological properties of 3D printed materials in kilovoltage and megavoltage photon beams. Phys Med (2017) 38:111–8. doi:10.1016/j.ejmp.2017.05.051
42. Bliznakova K., Speller R., Horrocks J., Liaparinos P., Kolitsi Z., Pallikarakis N., Experimental validation of a radiographic simulation code using breast phantom for X-ray imaging. Comput Biol Med (2010) 40:208–14. doi:10.1016/j.compbiomed.2009.11.017
43. Schneider C. A., Rasband W. S., Eliceiri K. W., NIH Image to ImageJ: 25 years of image analysis. Nat Methods (2012) 9:671–5. doi:10.1038/nmeth.2089
44. Malliori A., Bliznakova K., Speller R. D., Horrocks J. A., Rigon L., Tromba G., et al. Image quality evaluation of breast tomosynthesis with synchrotron radiation. Med Phys (2012) 39:5621–34. doi:10.1118/1.4747268
45. Im J. Y., Halliburton S. S., Mei K., Perkins A. E., Wong E., Roshkovan L., et al. Patient-derived PixelPrint phantoms for evaluating clinical imaging performance of a deep learning CT reconstruction algorithm. Phys Med Biol (2024) 69:115009. doi:10.1088/1361-6560/ad3dba
46. Kusk M. W., Stowe J., Hess S., Gerke O., Foley S., Low-cost 3D-printed anthropomorphic cardiac phantom, for computed tomography automatic left ventricle segmentation and volumetry – a pilot study. Radiography (2023) 29:131–8. doi:10.1016/j.radi.2022.10.015
47. LaRochelle E. P. M., Streeter S. S., Littler E. A., Ruiz A. J., 3D-Printed tumor phantoms for assessment of in vivo fluorescence imaging analysis methods. Mol Imaging Biol (2023) 25:212–20. doi:10.1007/s11307-022-01783-5
48. Malkov S., Wang J., Kerlikowske K., Cummings S. R., Shepherd J. A., Single x-ray absorptiometry method for the quantitative mammographic measure of fibroglandular tissue volume. Med Phys (2009) 36:5525–36. doi:10.1118/1.3253972
49. Chang T. Y., Lai K. J., Tu C. Y., Wu J., Three-layer heterogeneous mammographic phantoms for Monte Carlo simulation of normalized glandular dose coefficients in mammography. Scientific Rep (2020) 10:2234. doi:10.1038/s41598-020-59317-4
50. Elangovan P., Alrehily F., Pinto R. F., Rashidnasab A., Dance D. R., Young K. C., et al. Simulation of spiculated breast lesions. In: D Kontos, JY Lo, and TG Flohr, editors. Progress in biomedical optics and imaging - proceedings of SPIE, SPIE (2016). doi:10.1117/12.2216227
51. Checka C. M., Chun J. E., Schnabel F. R., Lee J., Toth H., The relationship of mammographic density and age: implications for breast cancer screening. Am J Roentgenol (2012) 198:W292–5. doi:10.2214/ajr.10.6049
52. Yaffe M. J., Boone J. M., Packard N., Alonzo-Proulx O., Huang S. Y., Peressotti C. L., et al. The myth of the 50-50 breast. Med Phys (2009) 36:5437–43. doi:10.1118/1.3250863
53. Patidar D., Yap L. B. C., Begum H., Soh B. P., Manual or auto-mode: does this affect radiation dose in digital mammography without compromising image quality? Radiography (Lond) (2022) 28:1064–70. doi:10.1016/j.radi.2022.08.004
54. Gennaro G., Del Genio S., Manco G., Caumo F., Phantom-based analysis of variations in automatic exposure control across three mammography systems: implications for radiation dose and image quality in mammography, DBT, and CEM. Eur Radiol Exp (2024) 8:49. doi:10.1186/s41747-024-00447-z
55. Zhou Y., Scott A., Allahverdian J., Frankel S., Evaluation of automatic exposure control options in digital mammography. J Xray Sci Technol (2014) 22:377–94. doi:10.3233/XST-140433
56. Karellas A., Vedantham S., Breast cancer imaging: a perspective for the next decade. Med Phys (2008) 35:4878–97. doi:10.1118/1.2986144
57. Patel K. S., Shah D. B., Joshi S. J., Aldawood F. K., Kchaou M., Effect of process parameters on the mechanical performance of FDM printed carbon fiber reinforced PETG. J Mater Res Technology (2024) 30:8006–18. doi:10.1016/j.jmrt.2024.05.184
58. Rosli A. A., Shuib R. K., Ishak K. M. K., Hamid Z. A. A., Abdullah M. K., Rusli A., Influence of bed temperature on warpage, shrinkage and density of various acrylonitrile butadiene styrene (ABS) parts from fused deposition modelling (FDM). AIP Conf Proc (2020) 2267. doi:10.1063/5.0015799
Keywords: 3D-printed breast lesions, breast phantoms, breast imaging, mammography, experimental images, modeling and simulations
Citation: Bliznakova K, Dukov N, Toshkova-Velikova O and Bliznakov Z (2024) Assessment of a method for manufacturing realistic breast lesions for experimental investigations. Front. Phys. 12:1441740. doi: 10.3389/fphy.2024.1441740
Received: 31 May 2024; Accepted: 13 August 2024;
Published: 29 August 2024.
Edited by:
Alexander Sommer, University Hospital Münster, GermanyReviewed by:
Marcus Kaar, Medical University of Vienna, AustriaElisabeth Salomon, Medical University of Vienna, Austria
Copyright © 2024 Bliznakova, Dukov, Toshkova-Velikova and Bliznakov. This is an open-access article distributed under the terms of the Creative Commons Attribution License (CC BY). The use, distribution or reproduction in other forums is permitted, provided the original author(s) and the copyright owner(s) are credited and that the original publication in this journal is cited, in accordance with accepted academic practice. No use, distribution or reproduction is permitted which does not comply with these terms.
*Correspondence: Kristina Bliznakova, a3Jpc3RpbmEuYmxpem5ha292YUBnbWFpbC5jb20=