- 1Key Laboratory of Disaster Prevention and Structural Safety of Ministry of Education, Guangxi Key Laboratory of Disaster Prevention and Structural Safety, College of Civil Engineering and Architecture, Scientific Research Center of Engineering Mechanics, Guangxi University, Nanning, China
- 2The 923th Hospital of PLA Joint Logistic Support Force, Nanning, China
- 3Guangxi Nanning Ruifeng Medical Devices Co., Ltd., Nanning, China
- 4Department of Thoracic Surgery, The First Affiliated Hospital, Sun Yat-sen University, Guangzhou, China
Introduction: Esophageal stenting is a widely used treatment for esophageal diseases, which can also be used for adjuvant therapy and feeding after chemotherapy for esophageal cancer. The structural parameters of the stent have a significant impact on its mechanical properties and patient comfort.
Methods: In the present work, we reconstructed the esophagus model based on the patient’s computed tomography (CT) data, and designed stents with different structural parameters. We used 3D printing technology to achieve rapid production of the designed stents by using Thermoplastic polyurethane (TPU)/Poly-ε-caprolactone (PCL) blends as the materials. The mechanical properties and effects on the esophagus of polymer stents with four different structural parameters of diameter, wall thickness, length and flaring were investigated by in vitro tests of radial compression and migration of the stents, as well as by finite element simulations of the stent implantation process in the esophagus and of the stent migration process. An artificial neural network model was established to predict the radial force of the stent and the maximum equivalent stress of the esophagus during implantation based on these four structural parameters.
Results: The results show that wall thickness was the structural parameter that had the greatest impact on the radial force of the stent (statistically significant, p < 0.01), and flaring was the structural parameter that had the greatest impact on the maximum equivalent stress of the esophageal wall after stent implantation (statistically significant, p < 0.01). No. 6 stent had a maximum radial force of 18.07 N, which exceeded that of commercial esophageal stents and had good mechanical properties. And the maximum equivalent force on the esophagus caused by its implantation was only 30.39 kPa, which can improve patient comfort. The predicted values of the constructed back propagation (BP) neural network model had an error of less than 10% from the true values, and the overall prediction accuracies were both above 97%, which can provide guidance for optimizing the design of the stent and for clinical research.
Discussion: 3D printing technology presents a wide range of applications for the rapid fabrication of personalized TPU/PCL blend stents that are more suitable for individual patients.
1 Introduction
Esophageal cancer is one of the most common malignant tumors worldwide, with extremely high morbidity and mortality. It is rarely cured in the advanced or terminal stage [1–3]. Esophageal stricture is a common symptom of esophageal cancer patients. Due to cancer cells, the local wall of the esophagus of patients will be unevenly thickened, causing esophageal stenosis and difficulty in swallowing, seriously affecting the quality of life of patients [4]. Despite surgical treatment or chemotherapy, the incidence of secondary esophageal stricture is still high [5, 6].
Esophageal stenting is an effective palliative treatment for esophageal disease, that can help patients with advanced esophageal cancer to relieve the esophageal obstruction and improve the swallowing function [7, 8]. After surgery or chemotherapy, esophageal stenting can be used to protect the treated area of the esophagus in feeding, and reduce the likelihood of secondary strictures for adjunctive treatment [9]. Esophageal stents include self-expanding metal stents (SEMS) and self-expanding plastic stents (SEPS) [10]. Currently, SEMS are widely used in clinical treatment of esophageal diseases due to their excellent shape memory properties and mechanical properties [11, 12]. However, metal stents can cause complications such as tumor tissue growth, perforation, bleeding, stent fall, and secondary strictures to varying degrees [13]. SEPS are effective in reducing esophageal injury and reducing the risk of esophageal restenosis, as well as being easy to remove from the esophagus [14]. However, it has been demonstrated that the migration rate of SEPS is significantly higher than that of SEMS [15]. Moreover, the sizes of commercial esophageal stents circulating are fixed and cannot be fully adapted to the patient, which will reduce the patient’s comfort and affect the therapeutic effect. This puts forward higher demands for esophageal stents in terms of materials, structure and humanized design.
3D printing is a technology that uses layers of discrete materials to print three-dimensional objects based on three-dimensional digital models. The products manufactured by 3D printing technology have a short cycle and can save costs. More importantly, 3D printing is not only independent of product complexity and size limitations, but also allows for innovative and personalized autonomous production. With the development of 3D printing technology, 3D printing has shown advantages in the field of biomaterials and medical devices [16, 17]. For example, Farzin et al. fabricated of sugar-based stents with ideal geometry and size for facilitating arterial surgical anastomosis by 3D printing [18]. And Matheus et al. developed 3D printed bioresorbable nitric oxide-releasing vascular stents [19]. Currently, an increasing number of scholars have begun to use 3D printing technology to develop blend esophageal stents that are more suitable for patients and have excellent mechanical properties [20]. For example, Lin et al. developed a new 3D-printed flexible PLA/TPU tubular polymeric stent with spirals that exhibits excellent self-expansion and anti-migration properties, and the performance is modulated by changing the ratio of PLA to TPU [21].
The structure and mechanical properties of esophageal stents are the most important factors in determining the interaction between the stent and the esophageal wall. At the same time, the structure of the stent also influences on its mechanical properties. Therefore, it is necessary to study the impact of esophageal stent implantation with different structural parameters on the individual esophagus of specific patients. However, it is impossible to test stent implantation into the human esophagus in the clinic, which in turn makes it difficult to accurately predict the effects of stent implantation.
Finite element method (FEM) is widely used in the biomedical field as an important and effective research method [22, 23]. For instance, Alkentar et al. investigated the performance of Ti6Al4V lattice structures designed for biomedical implants by using the FEM [24]. FEM is not be limited by environment and cost as experiments. It can also well solve various nonlinear problems encountered in the biomedical field, and achieve results very similar to the real situation. The accuracy of FEM can be improved through model reconstruction of medical cases [25]. Therefore, it is necessary to perform inverse modeling of the patient’s esophagus and FEM of the process of stent implantation into the esophagus with different structural parameters, so as to provide physicians with important guidance in designing stents and treating patients.
In this paper, we extracted the esophageal model from the computed tomography (CT) images and 3D printed it with thermoplastic elastomer (TPE). TPE is a new polymer material between rubber and resin, so it has the dual properties of rubber and plastic: high strength, high elasticity and injection molding [26, 27]. At the same time, TPE is an environmentally friendly, non-toxic and safe material with excellent weather resistance, fatigue resistance and temperature resistance. It also has a wide range of hardness from ultra-soft to 90A, which can meet the hardness needs of different products [28, 29]. Therefore, TPE is not only used in the manufacture of daily necessities and industrial products, but also in the manufacture of medical devices, such as tourniquets, nebulizer hoses, human tissue anatomy models, etc. [30]. Wu et al. used TPE to prepare high sensitivity capacitive pressure sensor of medical devices [31]. Fischenich et al. discovered that TPE hydrogels have elastic and viscous components that make them ideal for soft tissue replacements [32]. Esophageal model materials need to be as close as possible to the biomechanical properties of the human esophagus. In existing research, thermoplastic elastomers have been used for artificial blood vessels and artificial esophagus [33, 34]. TPE can be used to make artificial blood vessels [35]. Similarly, TPE can meet this basic requirement by adjusting its hardness, and as a commonly used soft material for 3D printing, it allows esophageal models to be molded quickly.
The stent designed in this paper consists of thermoplastic polyurethane (TPU) and poly-ε-caprolactone (PCL). TPU is a biocompatible polymer with high tensile strength, abrasion resistance, tear resistance, and low-temperature flexibility, enables provides the feasibility of designing elastic products with potential applications in medicine and tissue engineering [36–38]. PCL is a biodegradable polyester with the advantages of high availability and tensile strength, degradability and biocompatibility, and thus has been used in the field of tissue engineering [39, 40]. Some scholars used TPU and PCL blend materials for the manufacturing of various medical equipment, such as Pinto et al. prepared TPU/PCL blend for biomedical application [41]. Thus, TPU/PCL blends make it easier to manufacture flexible esophageal stents that are better suited to individual patients.
Back propagation (BP) neural network is a multilayer feedforward neural network trained according to the error back propagation algorithm. BP neural network has strong nonlinear mapping ability and flexible network structure, no need to determine the mathematical equation of the mapping relationship between the input and output in advance. It only learns a certain rule through its own training and gets the closest result to the desired output value when the input value is given [42–44]. Using BP neural network to predict the structural parameters of 3D printed esophageal stents can quickly and accurately obtain the index values of unknown combinations of structural parameters based on historical data and influencing factors, which can be fed back into the optimized design of esophageal stents for individual patients.
Based on the patient’s CT scan data, we reversed model, optimized and simplified the patient’s esophageal geometric model through medical software, and designed 12 esophageal stents with different structural parameters. By conducting in vitro tests and FEM on the 3D printed simulated esophagus and 12 esophageal stents, we analyzed the characteristics of structural parameters affecting the support performance, safety and comfort of 3D printed blend esophageal stents from a mechanical perspective. We established an artificial neural network model to predict the radial force of the stent and the maximum equivalent stress in the esophagus after stent implantation, and optimized the structural parameters of the esophageal stent for a specific patient. It provided physicians with a reference for the selection and design of esophageal stents, as well as the prediction and selection of auxiliary treatment with stent implantation after chemotherapy.
2 Materials and methods
2.1 CT reverse modeling and simplification of the esophagus
The main flowchart of esophageal model acquisition is shown in Figure 1.
2.1.1 Extraction of esophageal model
The esophageal model was extracted from CT scans of a patient with esophageal cancer who underwent chemotherapy. The purpose of the extraction was to design a stent that could improve the patient’s comfort by covering the chemotherapy area and extending slightly beyond it. The chemotherapy area was determined by positron emission computed tomography (PET)-CT fusion imaging, which showed the metabolic activity of the tumor cells. The extracted esophagus included the chemotherapy area and 8 additional tomograms (each 1.25 mm thick) at both ends of the chemotherapy area.
The raw data of the esophageal model in this paper comes from the PET-CT enhancement scan images of the patient. The patient is an elderly male diagnosed with esophageal cancer in a hospital in Zhongshan, Guangdong Province, based on the provided medical imaging data in digital image and communication on medicine (DICOM) format.
The patient’s DICOM data file before chemotherapy was imported into a medical image reading software called Xiaosaikankan DICOM Viewer, and PET-CT fusion browsing was used to obtain the metabolic information of each cell in the body. Based on the principle that tumor cells are metabolically active and their ability to uptake contrast agent is 2–10 times higher than normal cells, the location of the patient’s esophageal tumor and the CT section range of the chemotherapy area were accurately and rapidly determined.
The DICOM data file of the patient’s post-chemotherapy review was identified and imported by the medical image processing software called Mimics. After self-processing of the data by the software, three views of the patient’s CT-enhanced scan data were obtained. The Soft tissue scale was selected in the Grayscale of the Contrast workspace below, so that the soft tissues including the esophagus could be displayed in high contrast, which facilitated the segmentation of the human body tissues.
In Mimics, the extraction method for esophagus was mainly divided into the following steps:
1. After the DICOM data file was successfully imported, a new two-dimensional mask was created using the New Mask in the SEGMENT function area, and the Threshold gray value range was adjusted to separate the esophagus from other tissues in the image, resulting in a preliminary segmentation of the human body tissues.
2. Since the gray value range of the esophagus also included other tissues within this range, such as liver, kidney, and stomach. The Crop Mask was used to roughly segment the desired extraction region of the esophagus after the gray value segmentation. The Edit Masks mask editing tool was used to extract the roughly segmented esophagus to obtain a relatively complete esophagus model.
3. Due to the software’s inability to accurately distinguish more complex content during the gray value segmentation stage, there are still some problems with the esophageal model at this point, such as voids at the borders. The details of the model were refined using 2D mask editing. The Edit Masks and Multiple Slice Edit 2D editing tools were used to repair the details of the esophagus layer by layer in the 2D mask. The Mask 3D Preview function was used to preview the entire extraction process in the 3D view box and generate a 3D esophagus model from the mask. Finally, the Calculate Part tool was used to generate a 3D solid model of the esophagus from the edited mask.
2.1.2 Smoothing and simplification of the esophagus model
The extracted esophageal model had a complex and rough surface structure. To improve the 3D printing quality and reduce the computational complexity and time of the FEM, the model needed to be smoothed and simplified. The Smooth tool in the 3D TOOLS function area of Mimics software was not sufficient to achieve a satisfactory smoothing effect, so the model was further optimized in detail in a digital auxiliary design software called 3-Matic, while preserving the integrity of the inner wall boundary of the esophageal model.
The esophageal model data extracted from Mimics were transferred to 3-Matic. The Smooth tool in the Fix function area was used for surface processing, and then the Local Smoothing tool in the Finish function area was used to paint and smooth the local unevenness, abnormally raised areas, and the areas with serious granularity of the model, resulting in a smooth esophagus model. To accurately capture the boundary lines of the inner and outer walls of the esophagus, the Calculate Polylines tool was used to generate the boundary polylines of the inner and outer walls of the esophagus from the smooth esophageal model.
To ensure the convergence of the FEM and avoid the problems of numerical instability and long calculation time during the simulation process, the complex boundary polylines were fitted into circles one by one using the Radius tool in the Measure function area. By placing the centers of all circles on the same axis without changing their relative positions in the axial direction, the extracted esophagus was simplified to a slightly narrower cylinders in the middle, with a length of 64 mm, an inner diameter of 22 mm at both ends, and 18 mm in the middle, and an outer diameter of 28 mm at both ends and in the middle. The wall thickness of the esophageal cylinder was 3 mm at both ends and 5 mm in the middle, which was consistent with the results and analysis of the images on the patient’s post-chemotherapy examination report.
The two ends of the simplified esophageal cylinder were extended 30 mm and 50 mm up and down the axis respectively as the esophageal non-chemotherapy area. As shown in Figure 2A, the non-chemotherapy zone was simplified to a uniform cylinder with an inner diameter of 22 mm and a wall thickness of 3 mm, in order to facilitate the simulation of stent implantation, anti-migration test and simulation. The simplified entire esophageal cylinder was modeled in SolidWorks software for subsequent 3D printing.
2.2 Stent design with different structural parameters
The biomechanical performance of the interaction between the stent and the esophagus after implantation depends on the structural parameters of the stent. The stent was designed based on four structural parameters by SolidWorks: 1) outer diameter; 2) wall thickness; 3) length; 4) presence of flares at both ends.
Based on the variation of the inner diameter of the middle section of the simplified cylindrical esophagus model, three different outer diameters of stents with a slightly narrower middle were designed (Figure 2B). For each outer diameter, the stents were designed different varying in wall thickness, length and presence of flares at both ends. The flare was designed as a bell mouth with a length of 4 mm and a slope of 0.5. To facilitate the subsequent training of the BP neural network, three more stents with different structural parameters were added to the orthogonal experimental design scheme. The structural parameters of the 12 different stents are shown in Table 1. The outer diameters of the stents are recorded on the diameter of the narrowest point in the center.
2.3 3D printed esophageal and stents
The esophagus material was TPE and the stent material was TPU/PCL blend.
TPE with shore hardness of 30A was purchased from Jie Jia Plastic Technology Co., Ltd. (Dongguan, China). TPU with a specific gravity of 1.14–1.18 g/cm3 and shore hardness of 60A was purchased from De Chuang Co., Ltd. (Dongguan, China). PCL 600C (Mn = 65,000) was purchased from Shenzhen eSUN Industrial Co., Ltd. (Shenzhen, China). All materials were commercially available and were not further purified.
Materials with 80%TPU/20%PCL component ratios were prepared using a co-rotating twin-screw extruder (Nanjing Hass Extrusion Equipment Co., Ltd. China, diameter of screw = 2 cm, length/diameter ratio = 40/1). The process and details of TPU/PCL blend preparation can be found in Ref. [45].
The esophagus model and the designed stents in SolidWorks were exported as STL format files, and were sliced in Creality Print (Version3.5.9.0) slicing software. Then the G5 screw extrusion 3D printer from Shenzhen Creality 3D Technology Co., Ltd. (Shenzhen, China) was used for 3D printing (Figure 3). The printing parameters for different materials are presented in Table 2.
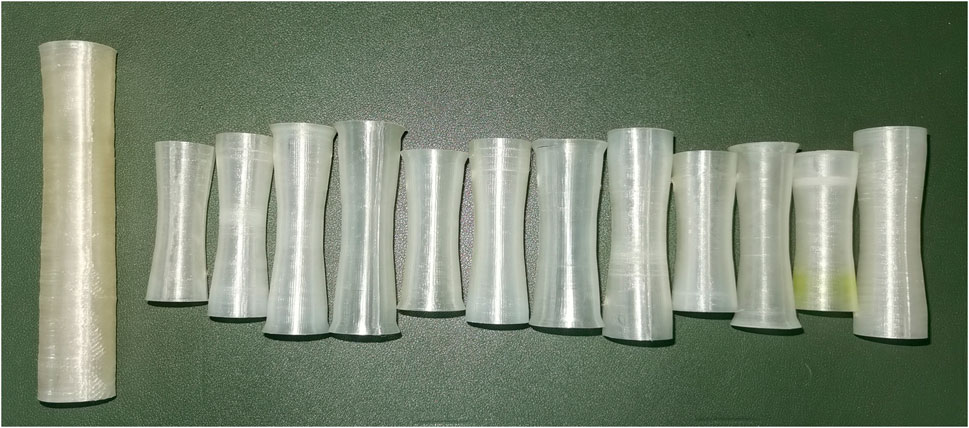
Figure 3. 3D printed esophageal model and designed stent (from left to right are the esophagus and No. 1-No. 12 stents).
2.4 Radial behavior and migration resistance of the stent
2.4.1 Radial force test
The radial performance of esophageal stents was evaluated using a universal testing machine (JJ UTM-1422, Jin Jian Testing Instrument Co., Ltd., Chengde, China). Three samples of each stent were tested. As shown in Figure 4A, the stents were placed between two circular platens and compressed at a rate of 10 mm/min until the stents reached 50% strain, and the force was recorded.
2.4.2 Migration resistance force test
The migration resistance of the esophageal stents in the 3D printed simplified esophageal model was tested. As shown in Figure 4B, the lower end of the esophageal model was clamped, and then the esophageal stent was implanted into the corresponding position of the chemotherapy area of the esophageal model. Holes were perforated at the upper end of the esophageal stent and tied to the universal tensile machine with a rope to ensure the same placement position each time. Use the universal testing machine to pull out the esophageal stent from the esophageal model at a constant speed, and set the end distance to 35 mm. The force-distance curve and maximum peak force during the uniform traction process of the stent along the esophageal axis were recorded.
2.5 Finite element method of esophagus and stents
2.5.1 Modeling and material parameters of esophagus and stents
Modeling of the simplified esophagus and stents were completed in Abaqus, a finite element software for engineering simulation. A rigid cylinder with a diameter slightly larger than the maximum diameter of the esophagus and stent was built to assist in the contraction surface.
The esophagus was manufactured using TPE, a highly elastic material that had mechanical properties close to those of the human esophagus. The stent material was 80%TPU/20%PCL hyperelastic blend, so the Mooney-Rivlin hyperelastic model was used for finite element calculations.
The strain energy density function of the Mooney-Rivlin model is:
Where I1 and I2 are strain invariants, W is the strain energy density function, and c10, c01, c11, c20, c02, and D1 are the hyperelastic constants. The subscripts of the constants indicate different strain invariants. c10 denotes the linear term coefficient of the first strain invariant I1, c01 denotes the linear term coefficient of the second strain invariant I2, c11 denotes the cross-term coefficient of I1 and I2, c20 denotes the quadratic term coefficient of I1, and c02 denotes the quadratic term coefficient of I2.
The Mooney-Rivlin hyperelastic constants were automatically generated by uniaxial tensile tests (n = 5) in combination with Abaqus software to fit the stress-strain curves. The simulation curves of the experimental stress-strain curves fitted to the Mooney-Rivlin model can be found in ref. [46]. The hyperelastic constants of esophagus and 80%TPU/20%PCL hyperelastic materials are listed in Table 3 [47].
2.5.2 Assembly and interaction of esophageal-stent
The esophagus, stent, and auxiliary constriction surface were assembled as follows: As shown in Figure 5, the plane center point of the narrowest position of the simplified esophageal chemotherapy area as the coordinate origin. Then the stent was moved so that the center point of the plane at the narrowest position in the middle section of the stent and the axis of the cylinder coincided with the coordinate origin and the axis of the esophagus. Similarly, the auxiliary contraction surface was moved so that the center of mass and axis of the auxiliary surface coincide with the coordinate origin and the esophageal axis.
Simulation of stenting for esophageal treatment: A cylindrical coordinate system was established with the axis as the Z-axis and the radial direction of the stent as the T-axis. The esophagus was fixed in the Z-axis direction, and a uniform displacement was applied to the entire auxiliary contraction surface in the radial direction. After contraction, the auxiliary surface contacted the outer surface of the stent, and the stent was pressed into the simplified esophagus. Then the constraints between the auxiliary surface and the stent were released, allowing the stent to self-expand and interact with the inner wall of the esophageal model until equilibrium was reached.
Stent migration simulation in the esophagus: After the stent was implanted in the esophagus, the esophagus was kept fixed in the Z-axis direction, while a distance in the negative Z-axis direction was applied to the stent. The stent moved at a constant speed along the negative direction of the Z-axis in the esophagus. The friction coefficient between the outer surface of the stent and the surface of the inner wall of the esophagus was set to be 0.1, and the termination distance was 40 mm.
2.6 Artificial neural network design
2.6.1 Selection of input and output parameters
We established a simple single-output artificial neural network model to train the structural parameter combinations and performance metrics of existing esophageal stents, which could quickly predict the stent performance metrics of other structural parameter combinations. The most common BP neural network topology includes input layer, hidden layer, and output layer, as shown in Figure 6. The number of nodes in the input layer was set to 4, representing the four structural parameters of the designed stent: outer diameter, wall thickness, length and flare width. The number of nodes in the output layer was set to 1, which represented the performance index value of the designed stent.
The radial force of the stent determines the effect of supporting the esophageal treatment area, facilitating eating and assisting treatment after implantation. However, excessive radial force not only affects the patient’s comfort, but can also lead to bleeding and other complications. In contrast, the maximum equivalent force in the esophagus after stent implantation can largely reflect the patient’s comfort. Therefore, we used the stent radial force and the maximum equivalent stress of the esophagus after implantation as the performance indicators of the stent for training and prediction.
The characteristic parameters of the training set and test set of the BP neural network model are shown in Table 1, while the performance index values were obtained from the results in vitro test and FEM result. Common neural networks require 70% of the data as the training set and 30% of the data as the test set, and the amount of data will directly affect the prediction results. Therefore, all the data in the first 9 groups of the 12 sets of stents data were repeatedly trained as the training set, and the last 3 groups were used as the test set.
2.6.2 BP neural network modeling
The amount of data in this study was small and the calculation was simple, so the BP neural network model can be designed with a single hidden layer. The number of nodes in the hidden layer can be set according to the following empirical formula:
where n is the number of input nodes, m is the number of output nodes, and a is a constant value from 1 to 10.
Since the number of hidden layer nodes is the main determinant of the accuracy of the neural network model, we set a to 1–10 in turn and plugged it into the formula to get the number of hidden layer nodes for the function training, after completing the design of the training function and data normalization. In this way, we obtained the number of hidden layer nodes that minimized mean square error (MSE) of the training set, and set it as the optimal number of hidden layer nodes to update the training function parameters.
The trainlm of Levenberg-Marquardt (L-M) optimization algorithm was adopted as the training function. Considering that the different magnitudes of the input parameters would interfere with the accuracy and performance of the network structure when training the neural network, all data were normalized through the mapminmax function so that the values of all magnitudes converge between 0 and 1. The maximum number of training times of the network was set to 1,000, the learning rate was 0.01, and the minimum error of the training target was 1e-6. After completing the training and testing of the network, denormalization was performed through the mapminmax function to obtain the actual predicted value of the output index value. Finally, the plot function and the bar function were used respectively to complete the plotting of the actual value, predicted value and error of the output indicator value.
3 Results and discussion
3.1 Results of radial compression test and migration test
3.1.1 Radial force of the stents
Figure 7 shows the radial force-distance curves of stents with different structural parameters and a commercial stent. The radial force of No.4 stent was the smallest, 1.64 N. The radial force of No.6 stent was the largest, 18.07 N. The radial force of commercial stent was 8.28 N, which lay between No.3 stent and No.11 stent. This suggests that the 3D printed polymer stent could provide the same radial support force as the commercial esophageal stent by adjusting the structural parameters, thereby supporting the chemotherapy area to assist with feeding.
By analysis of range and analysis of variance (ANOVA) on the maximum radial force obtained from the stents. We found that all the four structural parameters studied in the tests had significant influence (statistically significant, p < 0.01) and main effect on the stent radial force. Among them, wall thickness was the structural parameter with the greatest impact, which could greatly improve the stiffness of the stent. This was followed by flare and length in that order, while the outer diameter had the least effect. The optimum level of structural parameters for the maximum radial force were: 21 mm for the outer diameter, 0.16 mm for the wall thickness and 74 mm for the length without flare.
3.1.2 Migration resistance force of the stents
Since the FEM often has some differences with the actual situation, we performed in vitro tests to verify the accuracy of the model by comparing the test results with the finite element analysis results. The force-distance curves of the stent migration test and simulation are shown in Figure 8. From the figure, it could be seen that the force-distance curve shows a rapid upward trend in the initial stage. Then the upward trend gradually slowed down, became relatively flat, and finally decreased after passing the peak point. No. 1 stent had the lowest peak resistance to migration of 3.00 N, while No. 12 stent had the highest peak resistance to migration of 20.07 N. Increasing the diameter, wall thickness, length, as well as designing the flare of the stent can improve migration resistance. Among them, the outer diameter had the most significant effect on the migration resistance. Improving the migration resistance can reduce the probability of migration or even fall of the stent.
3.2 Finite element simulation of stent implantation and self-expansion
The stress distribution of the esophageal wall after the stent with different structural parameters is implanted into the esophagus is shown in Figure 9. The smallest maximum equivalent force on the esophageal wall after implantation was caused by stent 1 with 12.04 kPa. The largest maximum equivalent force caused was caused by stent 7 with 103.2 kPa. It could be seen that the maximum equivalent stress on the esophageal wall caused by the esophageal stent occurs at both ends of the stent, because the diameter of the stent is largest at both ends of the stent, which stretches the esophagus and causes greater deformation of the esophagus. This is most obvious in stents with flares at both ends. The stent without flares exerted a greater equivalent force on the esophageal wall at 10 mm from the origin because the rate of change of the stent diameter became greater here, resulting in a concentration of stress in the esophageal wall.
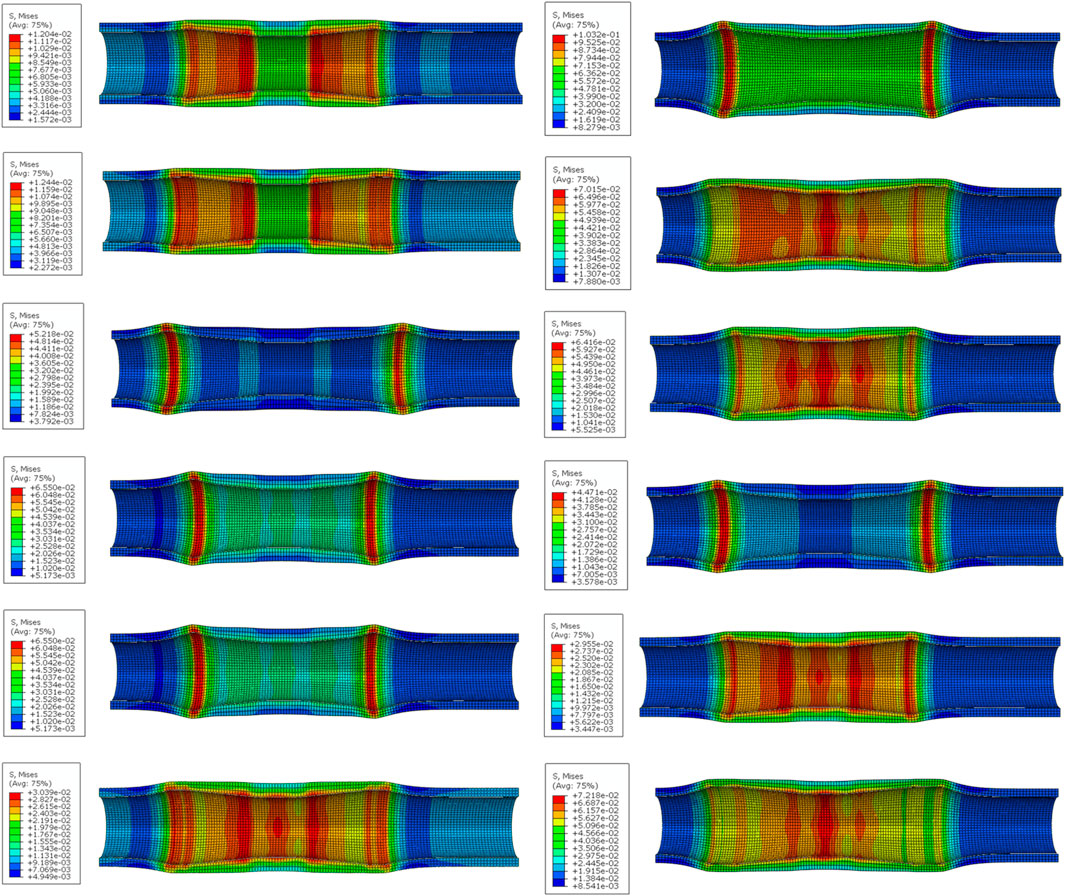
Figure 9. The stress distribution of the esophageal wall after stenting. (From top to bottom on the left are No.1 stent to No. 6 stent, and on the right are No. 7 stent to No.12 stent).
By performing range and ANOVA on the simulated maximum equivalent stress of the esophagus, it could be found that the outer diameter and dilation had a significant impact on the maximum equivalent stress (statistically significant, p < 0.01), and there was a main effect. Flare was the structural parameter that had the greatest impact, causing convex deformation of the esophageal wall and stress concentration at the contact point with the esophagus. Then followed the order of diameter, length and wall thickness. When the equivalent stress of the esophagus wall was minimum, the optimal levels of structural parameters were: outer diameter 19 mm, wall thickness 0.16 mm, length 64 mm, and without flare.
3.3 Finite element simulation of stent migration
The process of stent migration was investigated by applying displacement to the completed implanted stent in the esophagus. Comparing the force-distance curves of the migration test and the simulation in Figure 9, it was easy to find that the trends of the migration resistance of the stents with different structural parameters obtained by FEM were similar to those of the test results, and the peaks of the two were very close to each other. The peaks of the anti-migration force approximately occurred at the displacement point where the end of the stent passes through the narrowest area in the middle of the esophagus. Although the force-distance curves have errors due to the differences in the boundary conditions and load settings of the finite element simulation and the real test, this does not affect our analysis of the anti-migration ability of the stents through FEM.
When the maximum equivalent stress is exerted on the esophageal wall during dynamic migration of blended stents with different structural parameters, the stress distribution of the esophageal wall is shown in Figure 10. Obviously, the dynamic stress on the esophageal wall caused by dynamic migration of the stent was higher than the static stress at the completion of implantation. This is due to the fact that in order to make the stent fit better into the esophagus to improve patient comfort, the esophageal stent was designed as a cylindrical model with a slightly narrower middle like the simplified esophagus model. Even the rate of change of the stent’s outer diameter was basically the same as that of the esophagus’s inner diameter. Therefore, when migration of the stent occurs to the point where its end passes through the narrowest region in the middle of the esophagus, the deformation of the narrowest region is the greatest, and the dynamic maximum equivalent stress caused by the stent to the esophagus is also the largest during the migration process. Therefore, when increasing the stent migration resistance, it is important to avoid excessive migration resistance that may cause secondary damage to the mucosa of the esophageal inner wall during stent migration [48].
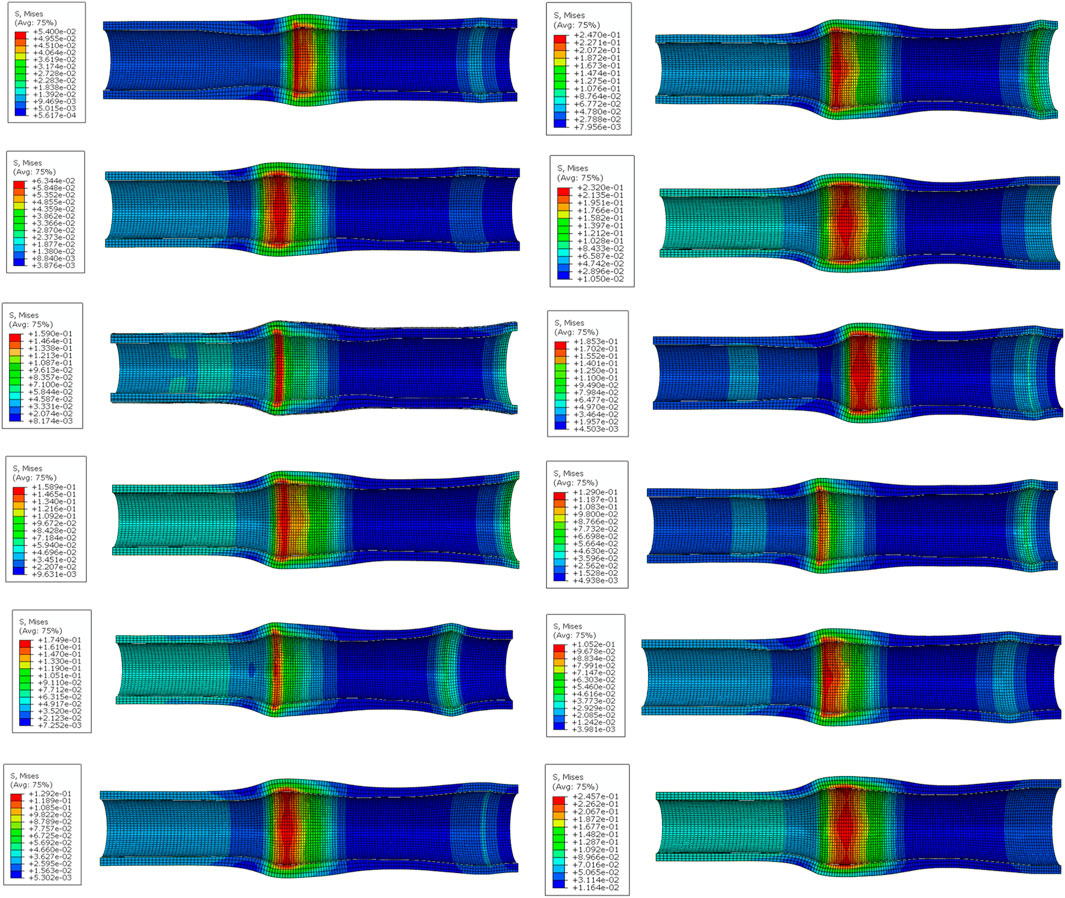
Figure 10. The stress distribution of the esophageal wall when the dynamic migration of the stent produces the maximum equivalent stress on the esophageal wall. (From top to bottom on the left are No. 1 stent to No. 6 stent, and on the right are No. 7 stent to No. 12 stent).
3.4 Comparative analysis of neural network model predictions
3.4.1 Stent radial force prediction
When cyclically training and predicting the radial force of the stent through the BP neural network, the optimal number of hidden layer nodes was 9, and the corresponding MSE was 0.00019. The standard BP neural network was constructed with 9 hidden layer nodes, and the trend of MSE during the training process is shown in Figure 11A. As can be seen from the figure, the best verification performance occurred in the 6th round with MSE of 0.0004. The performance of the BP neural network at this point is shown in Figure 11B. It is evident that the constructed BP neural network model fitted the test data excellently, and the correlation coefficients R were all above 0.998. By analyzing the true and predicted values of the radial force of the last three groups of stents listed in Table 4, we found that the error between them was less than 10%, and the overall prediction accuracy was as high as 97.5%, which indicated that the BP neural network predicted the radial force of stents very well.
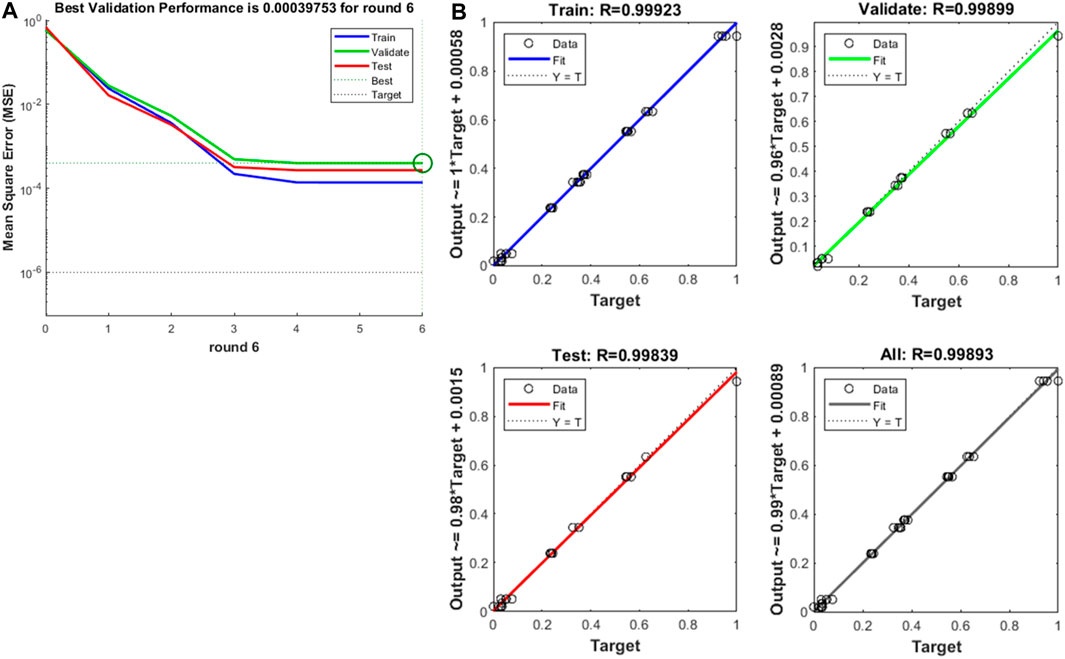
Figure 11. (A) Training mean squared error curve, (B) The curve fitting of BP neural network on training and predicting the radial force.
3.4.2 Prediction of maximum equivalent stress of esophagus
Similarly, the optimal number of hidden layer nodes was 6 and the corresponding MSE was 1.75e-05 when the maximum equivalent force of esophagus after stent implantation was trained and predicted cyclically by BP neural network. The standard BP neural network was constructed with 6 hidden layer nodes, and the trend of MSE was obtained during the training process as shown in Figure 12A. The figure shows that the best validation performance occurred in round 5 with MSE of 2.60e-05, at which time the performance of the BP neural network is shown in Figure 12B. It is also clear that the established BP neural network model fitted the finite element simulation data excellently, with correlation coefficients R above 0.999. Table 5 lists the true and predicted values of the maximal equivalent stress of the esophagus after stent implantation in the last three groups, and we could find that the errors between them are all less than 10%, and the overall prediction accuracy was as high as 99.91%, showing that the BP neural network also predicted the maximum equivalent stress of the esophagus very well.
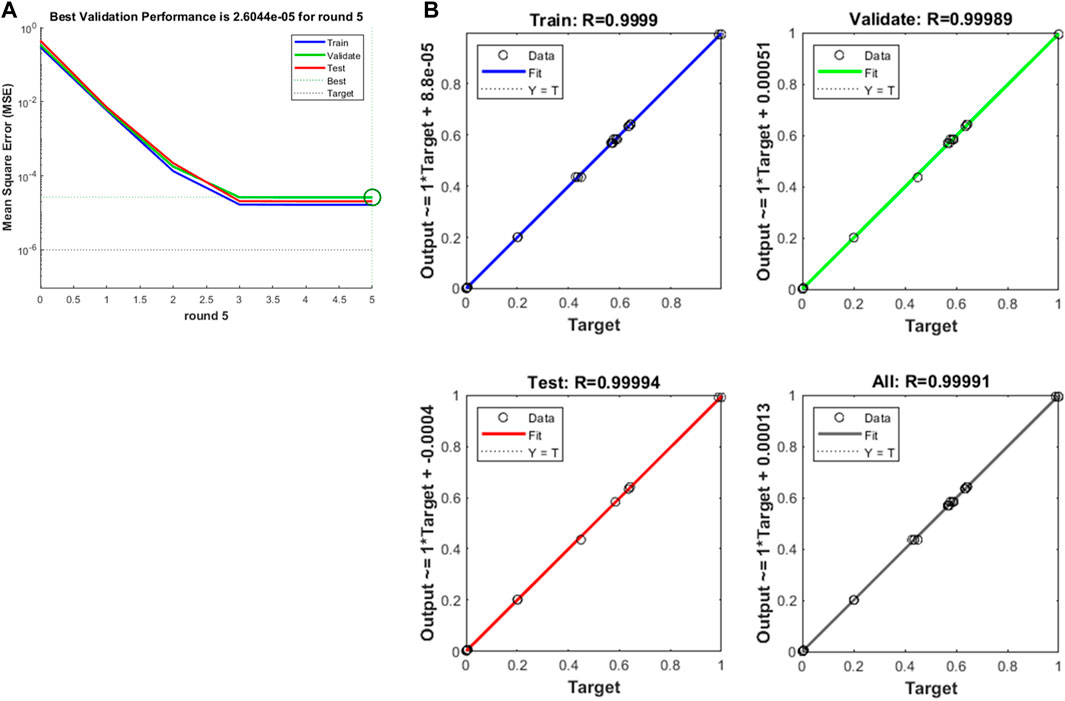
Figure 12. (A) Training mean squared error curve, (B) The curve fitting of BP neural network on training and predicting the maximum equivalent force of esophagus after stenting.
3.5 Discussion
From the overall results of the in vitro tests and FEM, it can be seen that among the 12 designed stents, No. 6 stent has the best mechanical properties. Its radial force is the largest at 18.07 N, which can effectively stretch the middle section of the esophagus that is still somewhat stenotic after treatment and prevent the occurrence of secondary narrow. The maximum equivalent stress on the inner wall of the esophageal stent after its implantation is only 30.39 kPa, which improves patient comfort to a large extent. However, in the clinic, it is necessary to select the appropriate stent according to the complex actual situation and needs of the patient.
In the current study, we obtained a simplified esophageal reconstruction model of the patient by processing the patient’s CT scan images using Mimics software and 3-Matic software. Based on this, esophageal stents with different structural parameters were designed. Using a twin-screw extruder, we melt blended two polymers with different flexibility, TPU and PCL, in a ratio of 8:2 for 3D printing of flexible esophageal stents.
In this study, quasi-static finite element simulations of the stent implantation process were performed using Abaqus software to obtain the biomechanical interactions between the stent and the esophagus after stent implantation, which is important for assessing patient comfort and the likelihood of bleeding from esophageal injury after stent implantation. On this basis, finite element simulations of dynamic migration occurring after completion of stent implantation were performed to assess the effectiveness of stent-assisted therapy and the possibility of complications, such as stent displacement, after stent implantation. Therefore, FEM plays a vital role in the design of esophageal stent and can provide a reference for optimizing the structural parameters of the esophagus.
However, the simulation in this article used a simplified patient esophageal model, which ignored the weight of the stent itself, the complex environment in the esophagus, and the impact of the patient’s eating and esophageal physiological swallowing on the stent. It also does not consider the possible changes in the esophageal characteristics due to chemotherapy [49]. Follow-up studies should explore the impact of patient feeding on the stent and the fatigue of the stent under cyclic swallowing stress [50].
The esophagus model used in the test was 3D printed by TPE, which can quickly obtain an esophagus model with physical properties close to those of the real human esophagus, but it cannot achieve a complete simulation of the real esophagus. In the study, we extracted and simplified the esophageal model as an unstratified homogeneous structure, which was uniformly printed with TPE of the same properties. The entire esophageal model was given the same material properties during finite element analysis. However, based on the histology, the real esophagus consists of five layers from inside to outside are: mucosa, the submucosa, the virtual interfacial layer, the circumferential inner and the longitudinal outer muscle layer [51]. There may be differences in properties between the different histologic layers. Moreover, the tests were conducted in vitro, ignoring the influence of the in vivo environment on the stent, which may not be able to directly and accurately reflect the effect of stent implantation on the human body. Therefore, it is necessary to consider animal tests or even clinical tests in future studies to further evaluate the performance of stents.
The constructed BP neural network can be trained according to the performance index values derived from existing experiments or simulations, and can rapidly and well predict the radial force of other stents and the maximum equivalent force of the esophagus after stent implantation, so as to provide a reference for the design of structural parameters of stents. But the amount of data in the training set was not very large. In the future, the accuracy of prediction can be further improved by increasing the amount of data in the training set and optimizing the BP neural network by using genetic algorithm according to the influence of structural parameters on the performance index.
Using 3D printing technology, we can rapidly design and manufacture customized esophageal stents based on patients’ CT and therapeutic needs, which can improve patient comfort and assist therapeutic effects.
4 Conclusion
In this study, the esophagus was inversely modeled based on CT images of the patient’s esophagus, and stents with different structural parameters were designed. TPU/PCL blend and TPE were selected as materials to 3D print the stent and simplified esophageal model. The results indicated that the 3D printed esophageal stent designed based on the CT of the patient’s esophagus exhibits good radial properties and anti-migration ability. Compared with 3D esophageal stents with fixed dimensions, it is able to ensure the required mechanical properties while generating less isotropic force on the esophageal wall, which can reduce the patient’s discomfort. We can adjust the mechanical properties of the stent and its effect on the esophagus by adjusting the structural parameters of the stent. In addition, a BP neural network was established in this paper to predict the radial force of the stent and the maximum equivalent stress of the esophagus after implantation. The results showed that the mechanical properties of the stent can be quickly and relatively accurately obtained by finite element simulation and BP neural network prediction, and fed back into the design of the stent, providing scientific guidance to further improve the comfort and clinical selection of the stent. Of course, further studies are needed regarding the fatigue performance of the stent to cope with physiological swallowing of the esophagus after implantation, more accurate finite element simulation, and optimization of the BP neural network.
Data availability statement
The original contributions presented in the study are included in the article/supplementary material, further inquiries can be directed to the corresponding author.
Author contributions
GlW: Formal Analysis, Methodology, Validation, Writing–review and editing. GhW: Conceptualization, Investigation, Methodology, Software, Writing–original draft, Writing–review and editing. SH: Conceptualization, Investigation, Methodology, Resources, Writing–review and editing. QZ: Formal Analysis, Investigation, Supervision, Writing–original draft. SZ: Data curation, Formal Analysis, Investigation, Writing–original draft. JF: Formal Analysis, Investigation, Supervision, Writing–original draft. BZ: Conceptualization, Resources, Validation, Writing–review and editing. PY: Conceptualization, Methodology, Project administration, Validation, Writing–review and editing.
Funding
The author(s) declare that financial support was received for the research, authorship, and/or publication of this article. This work was financially supported by Nanning Technology and Innovation Special Program (20204122) and Research grant for 100 Talents of Guangxi Plan.
Conflict of interest
Authors QZ and JF were employed by Guangxi Nanning Ruifeng Medical Devices Co., Ltd.
The remaining authors declare that the research was conducted in the absence of any commercial or financial relationships that could be construed as a potential conflict of interest.
Publisher’s note
All claims expressed in this article are solely those of the authors and do not necessarily represent those of their affiliated organizations, or those of the publisher, the editors and the reviewers. Any product that may be evaluated in this article, or claim that may be made by its manufacturer, is not guaranteed or endorsed by the publisher.
References
1. Santucci C, Mignozzi S, Malvezzi M, Collatuzzo G, Levi F, La Vecchia C, et al. Global trends in esophageal cancer mortality with predictions to 2025, and in incidence by histotype. Cancer Epidemiol (2023) 87:102486. doi:10.1016/j.canep.2023.102486
2. Collaboration GBOD, Abate D, Abbasi N, Abbastabar H, Abd-Allah F, Abdel-Rahman O, et al. Global, regional, and national cancer incidence, mortality, years of life lost, years lived with disability, and disability-adjusted life-years for 29 cancer groups, 1990 to 2017: a systematic analysis for the global burden of disease study. Jama Oncol (2019) 5:1749–68. doi:10.1001/jamaoncol.2019.2996
3. Goense L, van Rossum P, Kandioler D, Ruurda JP, Goh KL, Luyer MD, et al. Stage-directed individualized therapy in esophageal cancer. Ann N Y Acad Sci (2016) 1381:50–65. doi:10.1111/nyas.13113
4. Qi L, He W, Yang J, Gao Y, Chen J. Endoscopic balloon dilation and submucosal injection of triamcinolone acetonide in the treatment of esophageal stricture: a single-center retrospective study. Exp Ther Med (2018) 16:5248–52. doi:10.3892/etm.2018.6858
5. Yamasaki T, Tomita T, Takimoto M, Ohda Y, Oshima T, Fukui H, et al. Esophageal stricture after endoscopic submucosal dissection treated successfully by temporary stent placement. Clin J Gastroenterol (2016) 9:337–40. doi:10.1007/s12328-016-0685-0
6. Zhang Y, Zhang BZ, Wang YD, Zhang JJ, Wu YF, Xiao TY, et al. Advances in the prevention and treatment of esophageal stricture after endoscopic submucosal dissection of early esophageal cancer. J Transl Int Med (2020) 8:135–45. doi:10.2478/jtim-2020-0022
7. Wlodarczyk JR, Kuzdzal J. Stenting in palliation of unresectable esophageal cancer. World J Surg (2018) 42:3988–96. doi:10.1007/s00268-018-4722-7
8. Bi YH, Ren JZ, Chen HM, Bai LL, Han XW, Wu G. Combined airway and esophageal stents implantation for malignant tracheobronchial and esophageal disease a strobe-compliant article. Medicine (Baltimore) (2019) 98:e14169. doi:10.1097/MD.0000000000014169
9. Wen J, Yang YS, Liu QS, Yang J, Wang SF, Wang XD, et al. Preventing stricture formation by covered esophageal stent placement after endoscopic submucosal dissection for early esophageal cancer. Dig Dis Sci (2014) 59:658–63. doi:10.1007/s10620-013-2958-5
10. Kang Y. A review of self-expanding esophageal stents for the palliation therapy of inoperable esophageal malignancies. Biomed Res Int (2019) 2019:1–11. doi:10.1155/2019/9265017
11. Kim KY, Tsauo J, Song HY, Kim PH, Park JH. Self-expandable metallic stent placement for the palliation of esophageal cancer. J Korean Med Sci (2017) 32:1062–71. doi:10.3346/jkms.2017.32.7.1062
12. Herskovic AM, Rivard M, Rothstein RI, Hussein Z, Saunders J, Corcoran K, et al. Radiation esophageal stent. J Clin Oncol (2017) 35:e15514. doi:10.1200/JCO.2017.35.15_suppl.e15514
13. Singla V, Arora A, Khare S, Kumar A, Sharma P, Bansal N, et al. A novel technique to prevent migration of esophageal stent. Endoscopy (2020) 52:1040–1. doi:10.1055/a-1149-1084
14. Verschuur EM, Repici A, Kuipers EJ, Steyerberg EW, Siersema PD. New design esophageal stents for the palliation of dysphagia from esophageal or gastric cardia cancer: a randomized trial. Am J Gastroenterol (2008) 103:304–12. doi:10.1111/j.1572-0241.2007.01542.x
15. Oh YS, Kochman ML, Ahmad NA, Ginsberg GG. Clinical outcomes after self-expanding plastic stent placement for refractory benign esophageal strictures. Dig Dis Sci (2010) 55:1344–8. doi:10.1007/s10620-010-1134-4
16. Wang ZZ, Yang Y. Application of 3d printing in implantable medical devices. Biomed Res Int (2021) 2021:1–13. doi:10.1155/2021/6653967
17. Kalyan B, Kumar L. 3d printing: applications in tissue engineering, medical devices, and drug delivery. Aaps Pharmscitech (2022) 23:92. doi:10.1208/s12249-022-02242-8
18. Farzin A, Miri AK, Sharifi F, Faramarzi N, Jaberi A, Mostafavi A, et al. 3d-printed sugar-based stents facilitating vascular anastomosis. Adv Healthc Mater (2018) 7:e1800702. doi:10.1002/adhm.201800702
19. de Oliveira MF, Da Silva L, Catori DM, Lorevice M, Galvao K, Millás A, et al. Photocurable nitric oxide-releasing copolyester for the 3d printing of bioresorbable vascular stents. Macromol Biosci (2023) 23:e2200448. doi:10.1002/mabi.202200448
20. Ha DH, Chae S, Lee JY, Kim JY, Yoon J, Sen T, et al. Therapeutic effect of decellularized extracellular matrix-based hydrogel for radiation esophagitis by 3d printed esophageal stent. Biomaterials (2021) 266:120477. doi:10.1016/j.biomaterials.2020.120477
21. Lin M, Firoozi N, Tsai CT, Wallace MB, Kang Y. 3d-printed flexible polymer stents for potential applications in inoperable esophageal malignancies. Acta Biomater (2019) 83:119–29. doi:10.1016/j.actbio.2018.10.035
22. Wysocki MA, Doyle S. Enhancing biomedical data validity with standardized segmentation finite element analysis. Sci Rep (2022) 12:9860. doi:10.1038/s41598-022-13961-0
23. Mousavi SR, Khalaji I, Naini AS, Raahemifar K, Samani A. Statistical finite element method for real-time tissue mechanics analysis. Comput Methods Biomech Biomed Engin (2012) 15:595–608. doi:10.1080/10255842.2010.550889
24. Alkentar R, Máté F, Mankovits T. Investigation of the performance of ti6al4v lattice structures designed for biomedical implants using the finite element method. Materials (Basel) (2022) 15:6335. doi:10.3390/ma15186335
25. Ciklacandir S, Mihcin S, Isler Y. Detailed investigation of three-dimensional modeling and printing technologies from medical images to analyze femoral head fractures using finite element analysis. Ing Rech Biomed (2022) 43:604–13. doi:10.1016/j.irbm.2022.04.005
26. Liu X, Liu H, Wang D, Wang EP, Liu WJ, Yao KF, et al. Metallic glass-strengthened thermoplastic elastomer composites. Physica E Low Dimens Syst Nanostruct (2017) 90:37–41. doi:10.1016/j.physe.2017.02.020
27. Huang YC, Zheng Y, Sarkar A, Xu YM, Stefik M, Benicewicz BC. Matrix-free polymer nanocomposite thermoplastic elastomers. Macromolecules (2017) 50:4742–53. doi:10.1021/acs.macromol.7b00873
28. Mars WV, Ellul MD. Fatigue characterization of a thermoplastic elastomer. Rubber Chem Technol (2017) 90:367–80. doi:10.5254/rct.17.83780
29. Weerasekera N, Ajjarapu K, Sudan K, Sumanasekera G, Kate K, Bhatia B. Barocaloric properties of thermoplastic elastomers. Front Energ Res (2022) 10. doi:10.3389/fenrg.2022.887006
30. Wu YY, Liu CR, Lapiere M, Ciatti JL, Yang DS, Berkovich J, et al. Thermoplastic elastomers for wireless, skin-interfaced electronic, and microfluidic devices. Adv Mater Technol (2023) 8. doi:10.1002/admt.202300732
31. Wu GZ, Li SM, Hu JY, Dong MC, Dong K, Hou XL, et al. A capacitive sensor using resin thermoplastic elastomer and carbon fibers for monitoring pressure distribution. Pigm Resin Technol (2021) 50:437–43. doi:10.1108/PRT-10-2019-0098
32. Fischenich KM, Lewis JT, Bailey TS, Donahue T. Mechanical viability of a thermoplastic elastomer hydrogel as a soft tissue replacement material. J Mech Behav Biomed Mater (2018) 79:341–7. doi:10.1016/j.jmbbm.2018.01.010
33. Esmaeili S, Shahali M, Kordjamshidi A, Torkpoor Z, Namdari F, Saber-Samandari S, et al. An artificial blood vessel fabricated by 3d printing for pharmaceutical application. Nanomed J (2019) 6:183–94. doi:10.22038/nmj.2019.06.00005
34. Jiang H, Cui Y, Ma K, Gong M, Chang D, Wang T. Experimental reconstruction of cervical esophageal defect with artificial esophagus made of polyurethane in a dog model. Dis Esophagus (2016) 29:62–9. doi:10.1111/dote.12258
35. Wen XL, Sun ST, Wu PY. Dynamic wrinkling of a hydrogel-elastomer hybrid microtube enables blood vessel-like hydraulic pressure sensing and flow regulation. Mater Horiz (2020) 7:2150–7. doi:10.1039/d0mh00089b
36. RodríguezParada L, de la Rosa S, Mayuet PF. Influence of 3d-printed tpu properties for the design of elastic products. Polymers (Basel) (2021) 13:2519. doi:10.3390/polym13152519
37. Rigotti D, Dorigato A, Pegoretti A. 3d printable thermoplastic polyurethane blends with thermal energy storage/release capabilities. Mater Today Commun (2018) 15:228–35. doi:10.1016/j.mtcomm.2018.03.009
38. Lee H, Eom RI, Lee Y. Evaluation of the mechanical properties of porous thermoplastic polyurethane obtained by 3d printing for protective gear. Adv Mater Sci Eng (2019) 2019:1–10. doi:10.1155/2019/5838361
39. Tardajos MG, Cama G, Dash M, Misseeuw L, Gheysens T, Gorzelanny C, et al. Chitosan functionalized poly-ε-caprolactone electrospun fibers and 3d printed scaffolds as antibacterial materials for tissue engineering applications. Carbohydr Polym (2018) 191:127–35. doi:10.1016/j.carbpol.2018.02.060
40. Lin YH, Chiu YC, Shen YF, Wu Y, Shie MY. Bioactive calcium silicate/poly-ε-caprolactone composite scaffolds 3d printed under mild conditions for bone tissue engineering. J Mater Sci Mater Med (2018) 29:11. doi:10.1007/s10856-017-6020-6
41. Pinto LA, Backes EH, Harb SV, Pinto GM, Da Cunha D, Andrade R, et al. Shape memory thermoplastic polyurethane/polycaprolactone blend and composite with hydroxyapatite for biomedical application. J Mater Res (2023) 39:90–106. doi:10.1557/s43578-023-01172-w
42. Yang ST, Luo LN, Tan BH. Research on sports performance prediction based on bp neural network. Mob Inf Syst (2021) 2021:1–8. doi:10.1155/2021/5578871
43. Wang ZH, Chen QQ, Wang ZY, Xiong J. The investigation into the failure criteria of concrete based on the bp neural network. Eng Fract Mech (2022) 275:108835. doi:10.1016/j.engfracmech.2022.108835
44. Sun HL. Prediction of building energy consumption based on bp neural network. Wirel Commun Mob Comput (2022) 2022:1–10. doi:10.1155/2022/7876013
45. Yu P, Huang S, Yang Z, Liu T, Qilin Z, Feng J, et al. Biomechanical properties of a customizable tpu/pcl blended esophageal stent fabricated by 3d printing. Mater Today Commun (2023) 34:105196. doi:10.1016/j.mtcomm.2022.105196
46. Wu G, Huang S, Liu T, Yang Z, Wu Y, Wei G, et al. Numerical study of the biomechanical behavior of a 3d printed polymer esophageal stent in the esophagus by bp neural network algorithm. Comp Model Eng Sci (2024) 138:2709–25. doi:10.32604/cmes.2023.031399
47. Mozafari H, Dong PF, Zhao SJ, Bi YH, Han XW, Gu LX. Migration resistance of esophageal stents: the role of stent design. Comput Biol Med (2018) 100:43–9. doi:10.1016/j.compbiomed.2018.06.031
48. Garbey M, Salmon R, Fikfak V, Clerc CO. Esophageal stent migration: testing few hypothesis with a simplified mathematical model. Comput Biol Med (2016) 79:259–65. doi:10.1016/j.compbiomed.2016.10.024
49. Conklin JL. Evaluation of esophageal motor function with high-resolution manometry. J Neurogastroenterol Motil (2013) 19:281–94. doi:10.5056/jnm.2013.19.3.281
50. Li Y, Li J, Mao M, Yin H, Ni X, Pan C. Investigation on the design and application of hydraulic loading fatigue test device for non-vascular stent. Exp Tech (2023). doi:10.1007/s40799-023-00685-7
Keywords: 3D printing, esophageal stent, CT, biomechanics, TPU/PCL, reverse modeling, artificial neural network
Citation: Wu G, Wei G, Huang S, Zhang Q, Zeng S, Feng J, Zeng B and Yu P (2024) Study on the biomechanical properties of 3D printed blended esophageal stents with different structural parameters based on patient CT. Front. Phys. 12:1390321. doi: 10.3389/fphy.2024.1390321
Received: 23 February 2024; Accepted: 15 March 2024;
Published: 27 March 2024.
Edited by:
Pei Li, Xi’an Jiaotong University, ChinaReviewed by:
Jian Deng, Nanjing University of Aeronautics and Astronautics, ChinaChunlei Li, South China University of Technology, China
Copyright © 2024 Wu, Wei, Huang, Zhang, Zeng, Feng, Zeng and Yu. This is an open-access article distributed under the terms of the Creative Commons Attribution License (CC BY). The use, distribution or reproduction in other forums is permitted, provided the original author(s) and the copyright owner(s) are credited and that the original publication in this journal is cited, in accordance with accepted academic practice. No use, distribution or reproduction is permitted which does not comply with these terms.
*Correspondence: Guihong Wei, 2110302085@st.gxu.edu.cn