- 1School of Nuclear Science and Technology, University of South China, Hengyang, China
- 2Department of Radiation Oncology, Hunan Cancer Hospital/the Affiliated Cancer Hospital of Xiangya School of Medicine, Central South University, Changsha, China
Backgroud and objectives: The implementation of patient-specific quality assurance (PSQA) has become a crucial aspect of the radiation therapy process. Machine learning models have demonstrated their potential as virtual QA tools, accurately predicting the gamma passing rate (GPR) of volumetric modulated arc therapy (VMAT)plans, thereby ensuring safe and efficient treatment for patients. However, there is limited multi-center research dedicated to predicting the GPR. In this study, a dosiomics-based machine learning approach was employed to construct a prediction model for classifying GPR in multiple radiotherapy institutions. Additionally, the model’s performance was compared by evaluating the impact of two distinct feature selection methods.
Methods: A retrospective data collection was conducted on 572 VMAT patients across three radiotherapy institutions. Utilizing a three-dimensional dose verification technique grounded in real-time measurements, γ analysis was conducted according to the criteria of 3%/2 mm and 2%/2 mm, employing a dose threshold of 10% along with absolute dose and global normalization mode. Dosiomics features were extracted from the dose files, and distinct subsets of features were selected as inputs for the model using the random forest (RF) and RF combined with SHapley Additive exPlanations (SHAP) methods. The data underwent training using the extreme gradient boosting (XGBoost) algorithm, and the model’s classification performance was assessed through F1-score and area under the curve (AUC) values.
Results: The model exhibited optimal performance under the 3%/2 mm criteria, utilizing a subset of 20 features and attaining an AUC value of 0.88 and an F1-score of 0.89. Similarly, under the 2%/2 mm criteria, the model demonstrated superior performance with a subset of 10 features, resulting in an AUC value of 0.91 and an F1-score of 0.89. The feature selection methods of RF and RF + SHAP have achieved good model performance by selecting as few features as possible.
Conclusion: Based on the multi-center PSQA results, it is possible to utilize dosiomics features extracted from dose files to construct a machine learning predictive model. This model demonstrates excellent discriminative abilities, thus promoting the progress of gamma passing rate prognostic models in clinical application and implementation. Furthermore, it holds potential in providing patients with secure and efficient personalized QA management, while also reducing the workload of medical physicists.
1 Introduction
The treatment of tumors has increasingly become a multidisciplinary collaboration. Radiation therapy, as an important method in tumor treatment, will continue to play a key role in treating various tumor diseases with technological innovation and development [1]. Volumetric modulated arc therapy (VMAT) is an emerging technique in intensity-modulated radiation therapy (IMRT). Compared to traditional IMRT, VMAT not only shortens treatment time but also significantly improves dose coverage in the target area and protection of normal tissues [2–4]. Due to the complexity of VMAT treatment, implementing patient-specific quality assurance (PSQA) before treatment is crucial. It ensures that the VMAT treatment plan is implemented as expected and verifies the accuracy of dose calculation and beam model in the treatment planning system (TPS) [5]. Currently, the standard workflow for PSQA of intensity-modulated radiation therapy plans relies on technology based on actual measurements of phantoms. It compares the dose calculation results in the TPS with measurements on phantoms to determine if the plan is suitable for treatment [6, 7]. Gamma analysis is commonly used to evaluate the difference between calculated and measured doses. It quantitatively assesses regions that pass or fail the criteria [8]. Performing PSQA based on phantom measurements involves several processes: dose calculation on the phantom using the treatment plan parameters to generate a PSQA plan, data transfer of the PSQA plan, positioning of verification equipment, beam delivery, and gamma analysis. These repetitive tasks not only increase the workload of medical physicists but may also delay the patient’s first treatment. Previous studies have shown a correlation between plan complexity metrics and gamma passing rate (GPR), which is expected to optimize the PSQA process [9, 10].
In recent years, artificial intelligence (AI) has shown great potential in the clinical workflow of radiation therapy, thanks to the rapid development of computer technology. This includes tasks such as image reconstruction, image registration, target delineation, automated planning, automatic QA, and treatment efficacy evaluation [11, 12]. Deep learning and machine learning models have the potential to become accurate and time-saving virtual QA tools, making the QA process more efficient and effective [13, 14]. Several studies have used plan complexity parameters to predict GPR in VMAT with good accuracy [15–17]. However, there is limited research on predicting and classifying GPR using multi-institutional data. Valdes et al. [18, 19] extracted 78 plan complexity metrics for each IMRT plan and developed a lasso regularized Poisson regression model to predict GPR. The error for all analyzed plans was less than 3% under the 3%/3 mm gamma criterion. They validated this approach using 139 IMRT measurement data from different institutions, accurately predicting GPR across multiple institutions and measurement techniques. Yang et al. [20] used 54 complexity metrics to validate GPR prediction and classification accuracy for different delivery devices, QA equipment, and treatment planning systems. The average absolute error and root mean square error in the multi-institutional validation were between 2.42%–4.60% and 2.83%–4.95%, respectively, under the 3%/2 mm criterion. The sensitivity and specificity were 90% and 70.1%, respectively. Independent end-to-end testing showed a deviation within 3% between predicted and measured results.
The multicenter data employed in the GPR prediction model confers greater representativeness, thus enhancing its applicability and reliability. Furthermore, radiomics features encompass semi-quantitative and/or quantitative characteristics extracted from radiographic images. When integrated with AI, they hold the potential to facilitate the practical implementation of precision medicine in radiation therapy [21]. Dosiomics features, on the other hand, refer to radiomics features extracted based on dose distribution. However, the applicability of utilizing dosiomics features to construct predictive models for GPR classification across multiple institutions remains uncertain.
In this study, we utilized dosiomics features based on dose files as inputs to construct machine learning classification models for predicting VMAT PSQA results. The data used in the study was collected from three radiation therapy institutions. To account for the high-dimensional nature of dosiomics features, we employed two different feature selection methods and compared their impact on the performance of the models.
2 Materials and methods
2.1 Data collection
This study retrospectively collected data from 572 VMAT patients from three different radiation therapy institutions (Institution 1: Hunan Cancer Hospital, Institution 2:Yueyang Central Hospital, Institution 3: Changde First People’s Hospital). Among them, there were 174 cases of head and neck tumor plans, 141 cases of chest tumor plans, 24 cases of abdominal tumor plans, 223 cases of pelvic tumor plans, and 10 cases of other plans. The specific distribution is as follows: 213 VMAT plans from institution 1 underwent dose validation using Monaco (Elekta, Sweden) and Eclipse (Varian, United States) Treatment Planning Systems (TPS) on the ArcCHECK (Sun Nuclear, United States) platform, subsequently executed on the Axesse (Elekta, Sweden) and Trilogy (Varian, United States) linear accelerators. Likewise, institution 2’s 200 VMAT plans were dose validated on the Compass (IBA, Belgium) system, employing Monaco TPS, and delivered on the Infinity (Elekta, Sweden) linear accelerators. Institution 3’s 159 VMAT plans underwent dose validation using Eclipse TPS on the ArcCHECK device, and were administered on the Trilogy linear accelerators. The dose calculation grid resolution in the Eclipse and Monaco TPS was set to 3.0 mm, the Monaco TPS was a Monte Carlo algorithm, and the dose uncertainty was set to 1%. Regular checks and calibrations were conducted on the linear accelerators and verification devices during the measurement period to ensure their good performance. Please refer to Table 1, 2 for detailed distribution of the research data.
According to the recommendations of the American Association of Physicists in Medicine (AAPM) Task Group 218 report [22], gamma analysis was performed in the modes of absolute dose, global normalization, and 10% dose threshold. The mean ± standard deviation of the GPR data measured in this study, under the 3%/2 mm and 2%/2 mm criteria, were 96.72% ± 2.10% and 92.43% ± 4.49%, respectively. To construct the GPR classification prediction model, a tolerance threshold was introduced to classify the measurement results. In this study, the 99% confidence level of the average measured GPR value was used as the tolerance threshold [23]. When the measured GPR exceeded this tolerance threshold, the result was labeled as “pass” and denoted as “1"; otherwise, the result was labeled as “failure” and denoted as “0". Figure 1 illustrates the workflow for establishing the GPR classification prediction model.
2.2 Feature extraction
In this study, the region for extracting dosiomics features was determined by importing the RT dose files of each VMAT plan using 3D Slicer 5.0.2. This region encompassed the range covered by the isodose line, specifically 10% of the maximum dose. A Gaussian smoothing filter with a standard deviation of two pixels was used for each image in determining the feature extraction range to reduce image noise. All the images were resampled using B-spline interpolation algorithm to standardise the computation of features and resampled Pixel Spacing was set to 1 × 1 × 1 mm3. To eliminate the effect of different grey scale ranges and to ensure better comparability, discretisation was performed using a fixed bin width of 25 HU. The feature extraction process employed the radiomics library in Python 3.7, encompassing various image types such as original images (Original), wavelet-transformed images (Wavelet), and Gaussian-filtered images (LoG). A total of 1,130 features were extracted, which can be categorized into seven different types: shape features (2D/3D), first-order features, gray level cooccurrence matrix features (GLCM), gray level size zone matrix features (GLSZM), gray level run length matrix features (GLRLM), neighboring gray tone difference matrix features (NGTDM), and gray level dependence matrix features (GLDM), as presented in Table 3.
2.3 Dataset partitioning and processing
The entire dataset is randomly divided, with 90% of the data (514 plans) used as the training dataset, and the remaining 58 plans reserved solely for model performance evaluation. Given the inherent imbalance in the data, a stratified sampling technique was employed during the dataset partitioning process to ensure that the proportions of different data classes in the training and testing sets remained consistent with the original data. The data was then standardized using Eq. 1.
Where
2.4 Feature selection
Feature selection is a crucial step in building machine learning prediction models based on dosiomics due to the high dimensionality of dosiomics features. It helps address challenges associated with high-dimensional data, such as reducing training time and improving model interpretability and predictive performance [24]. Random Forest (RF) is an extraordinary ensemble technique that combines multiple decision trees, wherein each tree relies on the values of independently sampled random vectors. It is worth noting that all trees within the forest share the same distribution [25]. RF can be used as a feature selection method by calculating the importance of each feature in the dataset and sorting them in descending order. In addition to RF, this study incorporates the use of SHAP (SHapley Additive exPlanations) values for feature selection. SHAP values assign importance to features based on their contributions to the model’s output. A feature selection algorithm based on SHAP values can yield good results [26]. RF + SHAP is defined as a feature selection method for RF algorithms combined with SHAP. The process begins by inputting the training dataset into the RF model. Then, the SHAP values for each feature in the samples are calculated to measure their importance. Finally, the features are sorted in descending order based on their SHAP values [27]. The SHAP value of feature i was defined as Eq. 2.
Where N denotes the feature sets of the original data and S represents any feature subset in N.
2.5 Model training and evaluation
In this study, the data training was conducted using the extreme gradient boosting (XGBoost) algorithm. XGBoost is an expandable tree boosting system that utilizes the entire dataset for each decision tree generation. It takes into account the residuals between the prediction results of the previous decision tree model and the actual results during the generation of subsequent decision trees. XGBoost demonstrates high precision and effectively mitigates overfitting while supporting parallelization [28]. The performance of the binary classification model was evaluated using the F1-score, receiver operating characteristic (ROC) curve, and the area under the ROC curve (AUC). The ROC curve is a graphical representation that plots the false positive rate on the x-axis and the true positive rate on the y-axis, at different threshold values. The F1-score is defined as in Eqs. 3–5:
TP, FP, TN and FN represent the number of positive samples predicted positive, number of negative samples predicted positive, number of negative samples predicted negative, and number of positive samples predicted negative, respectively. In assessing the model’s performance, greater values of AUC and F1-score are indicative of better performance. All modeling and analysis procedures were executed using Python 3.7.
3 Results
3.1 The results of feature selection
Feature selection was conducted separately using the RF and RF + SHAP methods on the training set to derive distinct subsets of features. Table 4 showcases the top ten significant feature names based on the 3%/2 mm criterion. Among the features chosen by RF, there were five GLCM features, two GLSZM features, two GLRLM features, and one GLDM feature. Conversely, RF + SHAP recognized three GLCM features, two GLSZM features, three GLRLM features, and two GLDM features as the top ten important features. Additionally, Table 5 displays the top ten vital feature names under the 2%/2 mm criterion. RF selection yielded seven GLCM features, one GLSZM feature, and two GLRLM features, whereas RF + SHAP selected six GLCM features, three GLRLM features, and one GLDM feature. It is evident that both methods consistently identified texture features as the top ten important features under different criteria.
3.2 Evaluation of classification performance
The ROC curves and F1-score under different γ criteria for the test set are depicted in Figure 2 and Table 6 respectively. Under the 3%/2 mm criterion, the AUC values and F1-score of the prediction models built using the feature subsets selected by RF ranged from 0.82 to 0.88 and 0.85 to 0.89, respectively. The best performance was achieved when the feature subset size was 20 (AUC = 0.88, F1-score = 0.89). For the feature subsets selected by RF + SHAP, the AUC values and F1-score ranged from 0.78 to 0.86 and 0.84 to 0.92, respectively. The best performance was also observed when the feature subset size was 20 (AUC = 0.86, F1-score = 0.92), which was similar to the best model based on RF feature selection. Under the 2%/2 mm criterion, the AUC values and F1-score of the prediction models built using the feature subsets selected by RF ranged from 0.80 to 0.91 and 0.81 to 0.89, respectively. The best performance was achieved when the feature subset size was 10 (AUC = 0.91, F1-score = 0.89). For the feature subsets selected by RF + SHAP, the AUC values and F1-score ranged from 0.78 to 0.86 and 0.80 to 0.86, respectively. The best performance was observed when the feature subset size was 40 (AUC = 0.86, F1-score = 0.85), slightly lower than the best model based on RF feature selection. Utilize GridSearchCV on the training set to fine-tune hyperparameter values for all models. The hyperparameter values acquired for the optimal model using various criteria are presented in Table 7.
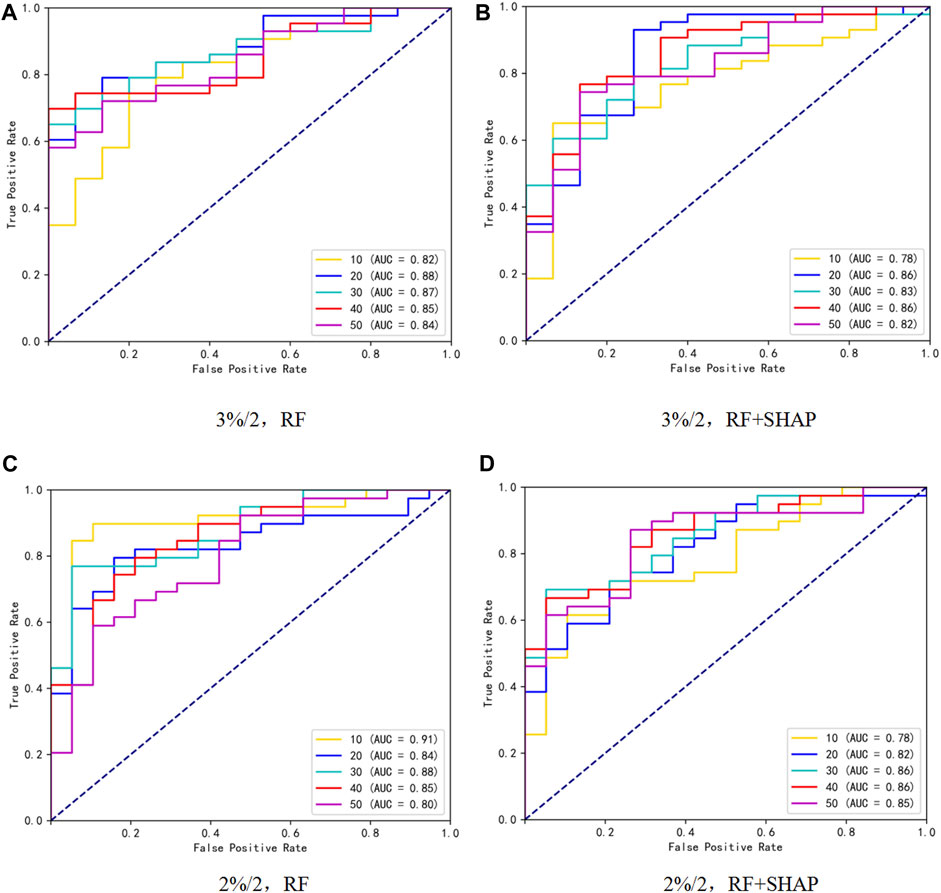
Figure 2. ROC curves under different γ criteria and subset size of 10,20,30,40,50, where (A, B) represent feature selection using RF and RF + SHAP methods, respectively, under the 3%/2 criterion, and (C, D) represent feature selection using RF and RF + SHAP methods, respectively, under the 2%/2 criterion.
3.3 Assessment of feature importance in model outputs
SHAP values explain the output of a predictive model by assigning a specific importance value to each feature [29]. Figures 3, 4 illustrate the importance ranking of input features based on SHAP values for the best model obtained through RF feature selection on the test set. Under the 3%/2 mm criterion, there are a total of 20 input features, comprising 9 GLCM features, 4 GLSZM features, 4 GLRLM features, and 3 GLDM features. The highest-ranked feature, Feature15, corresponds to log-sigma-3-0-mm-3D_gldm_HighGrayLevelEmphasis, closely followed by wavelet-LHL_glszm_SmallAreaLowGrayLevelEmphasis. Under the 2%/2 mm criterion, there are 10 input features, consisting of 7 GLCM features, 1 GLSZM feature, and 2 GLRLM features. The top-ranked feature, Feature6, corresponds to wavelet-LLH_glszm_LargeAreaHighGrayLevelEmphasis, closely followed by log-sigma-3-0-mm-3D_glrlm_RunEntropy.
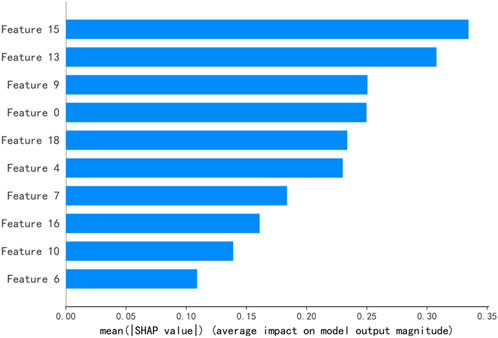
Figure 3. The top ten ranked features of the best predictive model under the conditions of 3%/2 mm. Note:Feature15:log-sigma-3-0-mm-3D_gldm_HighGrayLevelEmphasis, Feature13:wavelet-LHL_glszm_SmallAreaLowGrayLevelEmphasis, Feature9:log-sigma-3-0-mm-3D_glrlm_RunEntropy, Feature0:wavelet-HHL_glcm_Correlation, Feature18:wavelet-LLL_glcm_Imc2,Feature4:log-sigma-2-0-mm-3D_glcm_MaximumProbability, Feature7:wavelet-LHL_glszm_SmallAreaHighGrayLevelEmphasis, Feature16:wavelet-LHH_glrlm_GrayLevelNonUniformityNormalized, Feature10:log-sigma-3-0-mm-3D_glszm_GrayLevelNonUniformity, Feature6:wavelet-HHL_gldm_DependenceVariance.
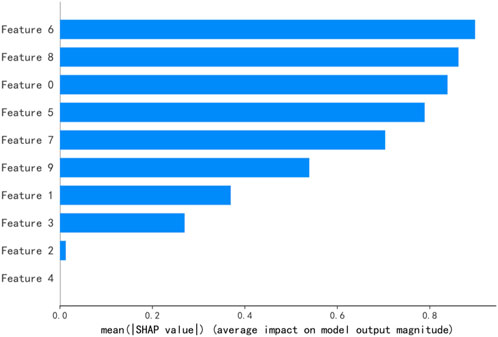
Figure 4. The top ten ranked features of the best predictive model under the conditions of 2%/2 mm. Note:Feature6:wavelet-LLH_glszm_LargeAreaHighGrayLevelEmphasis, Feature8:log-sigma-3-0-mm-3D_glrlm_RunEntropy, Feature0:wavelet-HHL_glcm_Correlation, Feature5:wavelet-HHL_glcm_MCC,Feature7:wavelet-HHL_glrlm_RunLengthNonUniformityNormalized, Feature9:wavelet-HHL_glcm_MaximumProbability, Feature1:wavelet-HHL_glcm_Contrast, Feature3:wavelet-HHL_glcm_ClusterTendency, Feature2:wavelet-HHL_glcm_DifferenceAverage, Feature4:wavelet-HHL_glcm_Idm.
4 Discussion
The implementation of individualized QA process for VMAT patients prior to treatment is a vital component of the clinical radiotherapy workflow. Developing a GPR classification prediction model can optimize the radiotherapy process, minimize the repetitive workload of medical physicists, and enable them to assess the plan’s “pass” or “failure” in advance without actual measurements. In case of a potential risk of “failure,” plan parameters can be adjusted for re-optimization. Multi-center studies are crucial for the application of prediction models in clinical decision-making as they enhance the reliability and robustness of the models. Multicenter studies help improve the reproducibility and applicability of predictive models. Two studies have successfully constructed GPR prediction models using plan modulation complexity indices as inputs, achieving excellent prediction accuracy. Furthermore, they demonstrated the feasibility of cross-validation across different delivery devices, QA devices, and TPS systems [19, 20]. Lambri et al [30] showed that single-centre GPR prediction model may not be directly applicable to other centres, and that the establishment of a public multicentre PSQA measurement database could provide benchmarking for the prediction model and help to advance the clinical implementation of PSQA outcome prediction models. In this study, a GPR classification prediction model was established using dosiomics features from VMAT plans in three radiotherapy institutions. These institutions encompassed three distinct combinations of devices (Trilogy + Eclipse + Arccheck, Infinity + Monaco + Compass, Axesse + Monaco + Arccheck). The results indicated that the optimal prediction model, based on the 3%/2 mm criterion, yielded an AUC value of 0.88 and an F1-score of 0.89. Similarly, the best model according to the 2%/2 mm criterion achieved an AUC value of 0.91 and an F1-score of 0.89. The model demonstrated favorable classification performance across various γ criteria.
The purpose of feature selection is to use as few features as possible to obtain better model performance. In order to compare the advantages and disadvantages of the two feature selection methods, the same number of feature subsets are used as model input. In this study, the maximum number of features was set to 50, and the number of features selected in order of feature importance was 10, 20, 30, 40, and 50. Under the 3%/2 mm standard, both the RF and RF + SHAP methods performed best when the number of feature subsets was 20, and the AUC values were 0.88 and 0.86 respectively. Under the 2%/2 mm standard, the RF method showed the best model performance with 10 feature subsets (AUC = 0.91), while the RF + SHAP method showed the best performance with 40 feature subsets (AUC = 0.86). Under the same γ criterion, the best model using RF + SHAP method in this study is superior to the results of the classification model based on dosimetry features by Hirashima et al [31], which shows that the use of RF + SHAP feature selection method to construct GPR classification prediction model has a certain degree of feasibility. Liu et al. [32] compared feature selection using SHAP values with feature selection using Fscore, Anova-F and MI, and confirmed the feasibility and superiority of SHAP-based feature selection in the classification diagnosis of Parkinson’s disease. This study also showed that superior performing algorithms combined with SHAP values build models that perform better. In this work, a preliminary comparison of two RF-based feature selection methods in GPR classification prediction was made, although the RF + SHAP feature selection method achieved good classification results, it did not show an absolute advantage in the test set compared to the RF feature selection method. According to the results of Liu et al. [32], SHAP value combined with other algorithms (gcForest and LightGBM) may make the model perform better, which requires in-depth analysis and discussion in the next steps.
Dosiomics features, derived from dose files, serve as quantifiable characteristics of dose distribution. Lizar et al. [33] have convincingly demonstrated the rationale of utilizing radiomics features for assessing PSQA results, with a particular emphasis on first-order and texture features as the most crucial ones. In our study, despite employing different feature selection methods on the training set under two distinct γ criteria, the top ten selected features consistently gravitated towards GLCM, GLSZM, GLRLM, and GLDM, underscoring the pivotal role of these four categories of texture features in the GPR prediction model. Notably, the input features of the optimal prediction model under both 3%/2mm and 2%/2 mm criteria also fell within these four texture feature categories, validating the robust performance of these texture features identified from the training set on the test set. These texture features are quantitative features of the 3D dose distribution and reflect the complexity of the treatment plan dose distribution. For PSQA results, it has been shown that texture features computed from fluence maps show a large correlation with plan deliverability and can be used as an indicator to assess the degree of modulation of a VMAT plan or may even have better performance than the traditional VMAT modulation index [34, 35]. Hirashima et al. [31] have further highlighted the significance of dosiomics features extracted from 3D dose distribution in predicting GPR values for individual plans, where texture features encompassing GLCM, GLDM, and GLRLM have exhibited substantial influence on GPR value prediction. Our findings unequivocally establish the significance of GLSZM as an additional influential factor, alongside GLCM, GLRLM, and GLDM, in the GPR classification prediction model.
Based on clinical practice, the GPR of VMAT patient plans rarely falls below the tolerance limits recommended by AAPM TG 218 [22]. As a result, the GPR data itself suffers from an imbalance issue. The setting of “pass” and “fail” tolerance limits for the GPR classification prediction model can significantly impact its performance. Previous studies have encountered severe data imbalance due to the challenge of collecting a sufficient number of low GPR plans for model training within a single radiation therapy institution [36, 37]. In this study, a total of 572 VMAT plans from three radiation therapy institutions were collected. To address the data imbalance issue, the classification tolerance limits were set based on the mean GPR. This approach helps improve the accuracy of GPR prediction. Specifically, for the 3%/2mm and 2%/2 mm γ criteria, the classification tolerance limits were set at 95.7% and 91.5%, respectively. Among the plans, approximately 26.4% (151 plans) were labeled as “fail” under the 3%/2 mm criterion, and approximately 32.7% (187 plans) were labeled as “fail” under the 2%/2 mm criterion. This distribution can be considered as a mild imbalance in the dataset [38]. Additionally, during the random partitioning of the dataset, stratified sampling techniques were employed to ensure that the proportions of different data classes in the training and test sets remained consistent with the overall dataset.
This study has several limitations. Firstly, it only utilized dosiomics features as inputs for multi-center GPR prediction. In future work, it is necessary to consider additional features such as plan complexity indices, MLC speed and acceleration. Moreover, it is crucial to explore methods for extracting a concise set of stable features from these combinations. By doing so, a prediction model with high robustness and generalizability can be constructed for clinical decision-making. These stable and significant features are expected to serve as valuable references for medical physicists in plan design. Secondly, the dataset used in this study encompasses multiple disease sites. Previous research has demonstrated that different disease sites can impact the classification performance of prediction models. Therefore, future multi-center studies and clinical validations should focus on specific treatment sites to enhance the model’s performance. Additionally, the relationship between dose-based dosiomics features and “failed” plans is complex. Currently, there is a lack of direct and accurate troubleshooting methods if a treatment plan fails dose validation.
5 Conclusion
Regarding the multi-center PSQA results, it is possible to construct a machine learning prediction model using dose-based dosiomics features. This model can exhibit good classification performance, which would facilitate the clinical application and implementation of GPR prediction models. This, in turn, has the potential to provide patients with safe and efficient personalized QA management while reducing the workload for medical physicists.
Data availability statement
The raw data supporting the conclusion of this article will be made available by the authors, without undue reservation.
Author contributions
QN: Writing–original draft, Writing–review and editing, Conceptualization. LC: Writing–review and editing, Conceptualization. JT: Data curation, Resources, Writing–review and editing. JP: Data curation, Resources, Writing–review and editing. LL: Data curation, Resources, Writing–review and editing. JZ: Data curation, Resources, Writing–review and editing. XY: Writing–review and editing, Conceptualization.
Funding
The author(s) declare that financial support was received for the research, authorship, and/or publication of this article. The study was supported by the Hunan Provincial Natural Science Foundation of China (project no: 2023JJ30373), the Science and Technology Innovation Program of Hunan Province (project no: 2021SK51116), and the Key Research and Development Project of Climbing Scientific Research Plan of Hunan Cancer Hospital (project no: YF2021006).
Conflict of interest
The authors declare that the research was conducted in the absence of any commercial or financial relationships that could be construed as a potential conflict of interest.
Publisher’s note
All claims expressed in this article are solely those of the authors and do not necessarily represent those of their affiliated organizations, or those of the publisher, the editors and the reviewers. Any product that may be evaluated in this article, or claim that may be made by its manufacturer, is not guaranteed or endorsed by the publisher.
Supplementary material
The Supplementary Material for this article can be found online at: https://www.frontiersin.org/articles/10.3389/fphy.2024.1387608/full#supplementary-material
References
1. Chandra RA, Keane FK, Voncken FEM, Thomas CR. Contemporary radiotherapy: present and future. Lancet (2021) 98(10295):171–84. doi:10.1016/S0140-6736(21)00233-6
2. Davidson MT, Blake SJ, Batchelar DL, Cheung P, Mah K. Assessing the role of volumetric modulated arc therapy (VMAT) relative to IMRT and helical tomotherapy in the management of localized, locally advanced, and post-operative prostate cancer. Int J Radiat Oncol Biol Phys (2011) 80(5):1550–8. doi:10.1016/j.ijrobp.2010.10.024
3. Nguyen K, Cummings D, Lanza VC, Morris K, Wang C, Sutton J, et al. A dosimetric comparative study: volumetric modulated arc therapy vs intensity-modulated radiation therapy in the treatment of nasal cavity carcinomas. Med Dosim (2013) 38(3):225–32. doi:10.1016/j.meddos.2013.01.006
4. Teoh M, Clark CH, Wood K, Whitaker S, Nisbet A. Volumetric modulated arc therapy: a review of current literature and clinical use in practice. Br J Radiol (2011) 84(1007):967–96. doi:10.1259/bjr/22373346
5. Wall PDH, Hirata E, Morin O, Valdes G, Witztum A. Prospective clinical validation of virtual patient-specific quality assurance of volumetric modulated arc therapy radiation therapy plans. Int J Radiat Oncol Biol Phys (2022) 113(5):1091–102. doi:10.1016/j.ijrobp.2022.04.040
6. Siochi RA, Molineu A, Orton CG. Point/Counterpoint. Patient-specific QA for IMRT should be performed using software rather than hardware methods. Med Phys (2013) 40(7):070601. doi:10.1118/1.4794929
7. Ezzell GA, Burmeister JW, Dogan N, LoSasso TJ, Mechalakos JG, Mihailidis D, et al. IMRT commissioning: multiple institution planning and dosimetry comparisons, a report from AAPM Task Group 119. Med Phys (2009) 36(11):5359–73. doi:10.1118/1.3238104
8. Low DA, Harms WB, Mutic S, Purdy JA. A technique for the quantitative evaluation of dose distributions. Med Phys (1988) 25(5):656–61. doi:10.1118/1.598248
9. Masi L, Doro R, Favuzza V, Cipressi S, Livi L. Impact of plan parameters on the dosimetric accuracy of volumetric modulated arc therapy. Med Phys (2013) 40(7):071718. doi:10.1118/1.4810969
10. Chiavassa S, Bessieres I, Edouard M, Mathot M, Moignier A. Complexity metrics for IMRT and VMAT plans: a review of current literature and applications. Br J Radiol (2019) 92(1102):20190270. doi:10.1259/bjr.20190270
11. Deig CR, Kanwar A, Thompson RF. Artificial intelligence in radiation Oncology. Hematol Oncol Clin North Am (2019) 33(6):1095–104. doi:10.1016/j.hoc.2019.08.003
12. Vandewinckele L, Claessens M, Dinkla A, Brouwer C, Crijns W, Verellen D, et al. Overview of artificial intelligence-based applications in radiotherapy: recommendations for implementation and quality assurance. Radiother Oncol (2020) 153:55–66. doi:10.1016/j.radonc.2020.09.008
13. Chan MF, Witztum A, Valdes G. Integration of AI and machine learning in radiotherapy QA. Front Artif Intell (2020) 3:577620. doi:10.3389/frai.2020.577620
14. Osman AFI, Maalej NM. Applications of machine and deep learning to patient-specific IMRT/VMAT quality assurance. J Appl Clin Med Phys (2021) 22(9):20–36. doi:10.1002/acm2.13375
15. Ono T, Hirashima H, Iramina H, Mukumoto N, Miyabe Y, Nakamura M, et al. Prediction of dosimetric accuracy for VMAT plans using plan complexity parameters via machine learning. Med Phys (2019) 46(9):3823–32. doi:10.1002/mp.13669
16. Wall PDH, Fontenot JD. Application and comparison of machine learning models for predicting quality assurance outcomes in radiation therapy treatment planning. Inform Med Unlocked (2020) 18:100292. doi:10.1016/j.imu.2020.100292
17. Salari E, Shuai Xu K, Sperling NN, Parsai EI. Using machine learning to predict gamma passing rate in volumetric-modulated arc therapy treatment plans. J Appl Clin Med Phys (2023) 24(2):e13824. doi:10.1002/acm2.13824
18. Valdes G, Scheuermann R, Hung CY, Olszanski A, Bellerive M, Solberg TD. A mathematical framework for virtual IMRT QA using machine learning. Med Phys (2016) 43(7):4323–34. doi:10.1118/1.4953835
19. Valdes G, Chan MF, Lim SB, Scheuermann R, Deasy JO, Solberg TD. IMRT QA using machine learning: a multi-institutional validation. J Appl Clin Med Phys (2017) 18(5):279–84. doi:10.1002/acm2.12161
20. Yang R, Yang X, Wang L, Li D, Guo Y, Li Y, et al. Commissioning and clinical implementation of an Autoencoder based Classification-Regression model for VMAT patient-specific QA in a multi-institution scenario. Radiother Oncol (2021) 161:230–40. doi:10.1016/j.radonc.2021.06.024
21. Arimura H, Soufi M, Kamezawa H, Ninomiya K, Yamada M. Radiomics with artificial intelligence for precision medicine in radiation therapy. J Radiat Res (2019) 60(1):150–7. doi:10.1093/jrr/rry077
22. Miften M, Olch A, Mihailidis D, Moran J, Pawlicki T, Molineu A, et al. Tolerance limits and methodologies for IMRT measurement-based verification QA: Recommendations of AAPM Task Group No. 218. Med Phys (2018) 45(4):e53–e83. doi:10.1002/mp.12810
23. Kusunoki T, Hatanaka S, Hariu M, Kusano Y, Yoshida D, Katoh H, et al. Evaluation of prediction and classification performances in different machine learning models for patient-specific quality assurance of head-and-neck VMAT plans. Med Phys (2022) 49(1):727–41. doi:10.1002/mp.15393
24. Chandrashekar G, Sahin F. A survey on feature selection methods. Comput Electr Eng (2014) 40(1):16–28. doi:10.1016/j.compeleceng.2013.11.024
26. Marcílio WE, Eler DM. From explanations to feature selection: assessing shap values as feature selection mechanism//2020. In: 33rd SIBGRAPI conference on graphics, patterns and images (SIBGRAPI). Ieee (2020). p. 340–7. doi:10.1109/SIBGRAPI51738.2020.00053
27. Nohara Y, Matsumoto K, Soejima H, Nakashima N. Explanation of machine learning models using shapley additive explanation and application for real data in hospital. Comput Meth Prog Bio (2022) 214:106584. doi:10.1016/j.cmpb.2021.106584
28. Chen T, Guestrin C. Xgboost: a scalable tree boosting system. Proc 22nd acm sigkdd Int Conf knowledge Discov Data mining (2016) 785–94. doi:10.1145/2939672.2939785
29. Lundberg SM, Lee SI. A unified approach to interpreting model predictions. Proc 31st Int Conf Neural Inf Process Syst. 2017: 4768–77. doi:10.5555/3295222.3295230
30. Lambri N, Hernandez V, Sáez J, Pelizzoli M, Parabicoli S, Tomatis S, et al. Multicentric evaluation of a machine learning model to streamline the radiotherapy patient specific quality assurance process. Phys Med (2023) 110:102593. doi:10.1016/j.ejmp.2023.102593
31. Hirashima H, Ono T, Nakamura M, Miyabe Y, Mukumoto N, Iramina H, et al. Improvement of prediction and classification performance for gamma passing rate by using plan complexity and dosiomics features. Radiother Oncol (2020) 153:250–7. doi:10.1016/j.radonc.2020.07.031
32. Liu Y, Liu Z, Luo X, Zhao H. Diagnosis of Parkinson’s disease based on SHAP value feature selection. Biocybern Biomed Eng (2022) (2022) 42(3):856–69. doi:10.1016/j.bbe.2022.06.007
33. Lizar JC, Yaly CC, Colello Bruno A, Viani GA, Pavoni JF. Patient-specific IMRT QA verification using machine learning and gamma radiomics. Phys Med (2021) 82:100–8. doi:10.1016/j.ejmp.2021.01.071
34. Park SY, Kim IH, Ye SJ, Carlson J, Park JM. Texture analysis on the fluence map to evaluate the degree of modulation for volumetric modulated arc therapy. Med Phys (2014) 41(11):111718. doi:10.1118/1.4897388
35. Park JM, Kim JI, Park SY. Prediction of VMAT delivery accuracy with textural features calculated from fluence maps. Radiat Onco (2019) 14(1):235. doi:10.1186/s13014-019-1441-7
36. Thongsawad S, Srisatit S, Fuangrod T. Predicting gamma evaluation results of patient-specific head and neck volumetric-modulated arc therapy quality assurance based on multileaf collimator patterns and fluence map features: a feasibility study. J Appl Clin Med Phys (2022) 23(7):e13622. doi:10.1002/acm2.13622
37. Li J, Wang L, Zhang X, Liu L, Li J, Chan MF, et al. Machine learning for patient-specific quality assurance of VMAT: prediction and classification accuracy. Int J Radiat Oncol Biol Phys (2019) 105(4):893–902. doi:10.1016/j.ijrobp.2019.07.049
38. Feng H, Wang H, Xu L, Ren Y, Ni Q, Yang Z, et al. Prediction of radiation-induced acute skin toxicity in breast cancer patients using data encapsulation screening and dose-gradient-based multi-region radiomics technique: a multicenter study. Front Oncol (2022) 12:1017435. doi:10.3389/fonc.2022.1017435
Keywords: machine learning, volumetric modulated arc therapy, dosiomics, gamma passing rate, multi-center study
Citation: Ni Q, Chen L, Tan J, Pang J, Luo L, Zhu J and Yang X (2024) Predicting the PSQA results of volumetric modulated arc therapy based on dosiomics features: a multi-center study. Front. Phys. 12:1387608. doi: 10.3389/fphy.2024.1387608
Received: 18 February 2024; Accepted: 08 May 2024;
Published: 21 May 2024.
Edited by:
Ruijie Yang, Peking University Third Hospital, ChinaReviewed by:
Wei Wei, Hubei Cancer Hospital, ChinaXiadong Li, Hangzhou Cancer Center, China
Fada Guan, Yale University, United States
Copyright © 2024 Ni, Chen, Tan, Pang, Luo, Zhu and Yang. This is an open-access article distributed under the terms of the Creative Commons Attribution License (CC BY). The use, distribution or reproduction in other forums is permitted, provided the original author(s) and the copyright owner(s) are credited and that the original publication in this journal is cited, in accordance with accepted academic practice. No use, distribution or reproduction is permitted which does not comply with these terms.
*Correspondence: Xiaohua Yang, xiaohua1963@usc.edu.cn; Luqiao Chen, m19186599706@163.com