- 1School of Environmental Science and Engineering, Liaoning Technical University, Fuxin, China
- 2School of Materials Science and Engineering, Liaoning Technical University, Fuxin, China
- 3Ordos Institute of Liaoning Technical University, Ordos, China
At the intersection of technological evolution and escalating computational demand, the role of optics is reemerging as a transformative force in the field of computing. This article examines the evolving landscape surrounding optical advantages in computing, focusing on current trends and prospects. Optical computing finds applications across various domains, such as parallel processing, high-speed signal processing, energy efficiency, quantum computing, machine learning, secure communication, and signal/image processing. This review synthesizes insights from scholarly articles, peer-reviewed journals, and academic papers to analyze the potential and challenges of leveraging optics for computational tasks. The literature review also critically examines the challenges of adopting optical computing solutions. The recommended multidimensional approach to overcoming adoption challenges involves holistically addressing integration challenges, manufacturing complexities, and infrastructure needs where collaboration will catapult optical computing into an era of computational power. Through a multidimensional exploration, this article provides a comprehensive understanding of the opportunities and challenges in harnessing optical advantages in computing, positioning optical computing as a revolutionary force with far-reaching consequences. Consequently, this review offers insight and guides researchers, industry professionals, and policymakers toward a computational future that maximizes the advantages of optical computing in specific and pivotal application areas, transcending existing boundaries.
1 Introduction
Optical computing is a cutting-edge technical advancement that has the potential to completely change the computer industry because of its exceptional benefits derived from the characteristics of light. Optical computing harnesses the unique properties of light for processing information, presenting a potential shift from the established dominance of electronic processors. Traditionally, electronic processors have been the backbone of computational systems, but the limitations they pose regarding processing speed, energy efficiency, and information-handling capabilities have sparked a growing interest in the potential advantages offered by optics in some applications. This literature review is an extensive analysis of peer-reviewed journals on the benefits and challenges associated with optical computing. The literature review focuses on different applications, including parallel processing for extensive data handling, fast signal processing, energy efficiency issues, quantum computing using optics, applications in machine learning, and secure optical communication through optical quantum key distribution. This literature review discusses various optical computing areas, including parallel processing and massive data handling, high-speed signal processing with energy efficiency considerations optics in data centers, quantum computing with optics optical neural networks for machine learning secure communication via optical quantum key distribution, and signal and image processing. In this technological era, understanding the state of knowledge regarding optical advantages in computing is imperative for researchers, engineers, and stakeholders.
1.1 Research objective
This review focuses on the existing optical computing knowledge. The primary research question guiding this exploration is to discern the transformative potential of optical advantages in computing applications and understand the challenges that impede their widespread adoption. Through a comprehensive analysis of scholarly articles, peer-reviewed journals, and academic papers, this review aims to offer insights by exploring the merits and limitations of optical computing. By synthesizing the current state of research, this paper aims to provide a nuanced perspective that informs future directions and advancements in the field.
1.2 Scope and limitations
The scope of this literature review spans a diverse array of applications, encompassing parallel processing for massive data handling, high-speed signal processing, energy efficiency considerations, quantum computing leveraging optics, machine learning applications, secure communication through optical quantum key distribution, and the role of optics in signal and image processing. While the breadth of coverage aims to offer a holistic understanding, it is essential to acknowledge the limitations inherent in the available literature. The dynamic nature of technology implies that newer developments may have occurred post-cutoff date, and specific niche applications may receive limited attention in the literature. Nevertheless, this review provides a comprehensive overview and analysis within the parameters of the available research landscape. This review focuses on eight crucial properties that present potential success of optics in computing, namely, parallel processing and massive data handling, high-speed signal processing, energy efficiency, optical interconnects in data centers, quantum computing, optical neural networks for machine learning, secure communication, and signal and image processing.
2 Parallel processing and massive data handling in optical computing
Time-multiplexed optical computing presents multiple advantages compared to conventional parallel processing designs that are particularly relevant regarding simultaneous data processing, high bandwidth, reduced latency, and scalability. For instance, according to [1], optics excel in parallelism, which enables various data streams to run and be processed simultaneously. As opposed to sequential processing used by electronic processors, optical computing systems presented by [2] allow performing the operations simultaneously to reduce time-consuming massive datasets [3, 4]. Additionally, the inherent properties of light enable high-bandwidth communication [5, 6]. It becomes feasible to efficiently transfer large volumes of data at higher speeds, given that signals with different wavelengths can be transported simultaneously. [7], [5], and [8] reveal that optics have the potential to achieve high bandwidth parallelism in data-intensive applications. Therefore, as argued by several researchers, the inherent parallelism and high speeds with which time-multiplexed optical computing transmits reveal its efficiency in processing that can provide answers to huge data sets at low latencies but great rates.
Parallel processing in optics also contributes to reduced latency. Since light travels much faster than electrical signals, optical systems can provide faster data access and processing than electronics [9, 10]. This property would be specifically advantageous to applications that need real-time decision-making and response. Furthermore, optical systems are inherently scalable [11, 12]. [13] explains that the necessary parallelism of visual computing enables scalability due to the ease with which new components can be added. A component can scale on demand without significantly increasing complexity when it fails. This scalability is critical for applications that deal with constantly growing datasets [14]. Accordingly, the significant latency reduction provided by optical parallel processing because of speed–of–light indicates that they can accelerate real-time decision-making and adjust to changing data demands.
However, optics also face some drawbacks that should be addressed for widespread adoption in parallel processing and massive data handling, including integration challenges, signal loss, and noise, as well as cost and complexity [15–17]. For instance, an integration problem of optic components with current electronic systems is far from simple because connecting two processors requires specific interfaces. [18] discuss the barriers to integration and note that this limitation can influence the reliability of parallel processing in optical systems operated over long ranges. Suitable signal gain and noise suppression procedures are essential for preventing perverted parallel data processing. Additionally, optical elements may be challenging to implement due to their cost and complexity. Optical computing devices require highly specialized materials and manufacturing processes, which make them more expensive than the traditional electronic components [2, 19].
Furthermore, the complexity of an optics system can cause problems related to management and maintenance [18]. Although optical technologies exhibit excellent capabilities for parallel computation, there remain a few challenges, like integration concerns that lead to signal fading out. However significant the benefits are, optical computing in parallel processing and extensive data handling demands a careful weighing of advantages against disadvantages; otherwise, effective integration into computation would be impaired. The landscape ahead for optic computing will largely depend on emerging technologies aimed at overcoming these challenges.
3 High-speed signal processing in optical computing
The speed advantages of optical computing include computational acceleration, low latency, fast computation rate, and improved data transfer, allowing the use of the fact that light travels significantly faster than electrical signal does. Another fundamental advantage of optical computing is the speed associated with light. In conventional electronic circuits, light travels much faster than electric signals. As [13] depicted, this velocity benefit allows optical systems to process signals quickly. This fundamental characteristic can greatly benefit tasks characterized by frequent data analysis and communication [13]. Moreover, the speed of light is high, hence less latency in communication. Optical signals travel long distances without much delay, thus making optical communication systems suited for Real-Time applications. This feature is especially critical in the telecommunications and network infrastructure sectors [13]. For instance, an experiment by [20] successfully reported using 256QAM-based constellation shaping with this pre-equalization calibration approach to test 800 Gbit/s per wavelength transmission (see Figure 1). In addition, optical computing may overperform electronic processors in some calculations. Parallelism in optics and the fast signal processing speeds translate to faster execution of algorithms. It is essential for applications that demand fast data processing, such as simulations and scientific computing [21].
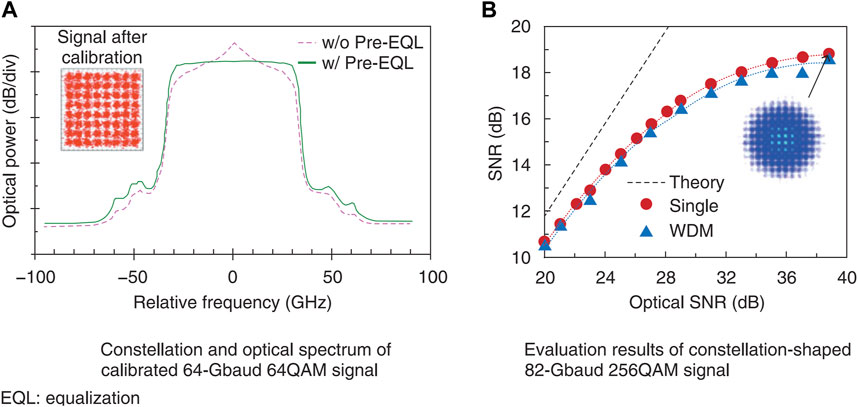
FIGURE 1. Results of experiments using constellation shaping and calibration technologies [20]. (A) Constellation and optical spectrum of calibrated 64-Gbaud 64QAM signal. (B) Evaluation results of constellation-shaped 82-Gbaud 256QAM signal EQL, equalization.
Additionally, the data transfer rate increases due to a faster optical system. [22] discussed that data center optical interconnects eliminate bottlenecks and improve a system’s overall performance through faster communication between components. Not only computational acceleration and short latency but also high data rates are achieved with the optical computing advance driven by light speed, which increases its applicability for applications requiring responses in real-time processing to be efficient. The possible quicker algorithm running and better system performance make optical computing an attractive option for tasks that demand rapid data analysis and high-speed operations.
Issues related to the design of complex components, sensitivity towards the environment, and fewer material options must be considered in successfully applying high-speed optical systems. Complexity often arises in formulating elements that capitalize on all benefits of high-speed signal processing using optics. Controlling optical signals with precision and sustaining coherence leads to complex designs and sometimes even involves. It can become an issue while implementing high-speed optical systems in practice [23]. In addition, optical signals are weather condition-based as they react to temperature and humidity changes. These differences can alter light’s nature, resulting in signal degradation or loss. Environmental effects should be detected and eliminated to ensure reliable high-speed signal processing in optical systems [13]. Finally, there is a lack of sufficient materials that can be used as high-speed optical components. This limitation may also hamper the evolution of devices that can fully realize speed opportunities in optical computing. Further research should be continued to discover and create the materials capable of satisfying these demands to meet the ultrahigh-speed signal processing needs.
Multiple strategies can be implemented to address the environmental sensitivity of high-speed optical components to temperature and humidity changes, thus mitigating their impact on signal integrity and system reliability. For instance, using proper packaging techniques helps protect optical components from environmental factors. Sealing optical components in hermetic packages or using protective coatings can shield them from temperature and humidity fluctuations, thereby preserving signal integrity [24, 25]. For instance, hydrophobic and anti-reflective coatings can prevent moisture absorption and minimize signal degradation caused by environmental factors [26]. Incorporating temperature and humidity sensors within the packaging can also enable real-time monitoring and adjustment of environmental conditions. Besides, deploying and monitoring basic environmental control systems, such as air conditioning, humidity control units, and thermal management solutions, within optical computing facilities can regulate temperature and humidity levels to maintain optimal conditions for high-speed optical components. Optimizing high-speed optical component designs and implementing rigorous testing and quality assurance protocols can improve their resilience to environmental sensitivity. By implementing these strategies, the environmental sensitivity of high-speed optical components to temperature and humidity changes can be effectively mitigated, thereby enhancing signal integrity and system reliability in optical computing systems.
4 Energy efficiency in optical computing
The energy efficiency of optical computing offers multiple advantages due to low power consumption during operation and reduced heat generation. Optical computing has an advantage over conventional electronic circuits regarding power requirements. In this regard, [27] observed in their analysis that light transmits information better and faster than electric signals, particularly when spaces are long distances apart. This feature is handy in applications where energy efficiency has a significant impact. Also, optical systems for data are less heat-producing than electronic processors. This feature is helpful in applications where heat dissipation is essential, such as in data centers. Reduced heat generation can help improve energy efficiency by reducing or eliminating complex cooling systems [13]. Heat dissipation and decreased power consumption make optical computations an innovative concept for minimal energy production applications.
However, challenges related to energy consumption during component manufacturing and integration with existing infrastructure must be carefully addressed to realize the overall energy-efficient potential of optical computing fully. Though the operation of optical systems provides low energy consumption, manufacturing their components can be energy-consuming. However, unique materials and precise manufacturing processes involving a more extensive energy footprint during production are frequently used. Based on [28], balancing the overall energy equation requires considering operational and manufacturing energetic demands. In addition, integrating optical components with standing electronic infrastructure cannot be easy. Seamless compatibility necessitates more components and interfaces, which may contribute to increased total energy consumption. It is necessary to overcome integration challenges to achieve the full energy-saving potential of optical computing [29].
Simplifying the integration process of optical components with electronic systems is essential in facilitating practical deployment and addressing challenges such as interface compatibility and reliability over long ranges. For instance, establishing standardized interfaces between optical and electronic components promotes compatibility and interoperability. Adopting common communication protocols and connector standards streamlines the integration process, allowing seamless connectivity between different components [30–32]. Besides, interdisciplinary collaboration between optical engineers and electronic system designers is essential to tackle integration challenges holistically. Such collaboration allows leveraging diverse expertise to develop integrated solutions that meet optical and electronic requirements, ensuring compatibility and reliability over long ranges. Reliability engineering principles also play an important role in enhancing the robustness and longevity of integrated systems. Rigorous reliability testing, including accelerated aging tests and environmental stress screening, identifies potential failure modes and ensures the reliability of integrated systems under diverse operating conditions [33]. Moreover, flexible deployment options, including centralized and distributed architectures, accommodate various application scenarios and installation environments, optimizing performance and scalability.
Offering comprehensive training and educational resources to end-users and system integrators would foster effective deployment and operation of integrated optical-electronic systems. Hands-on training programs and detailed documentation would empower personnel with the knowledge and skills to effectively deploy, maintain, and troubleshoot integrated systems. Thus, integrating optical components with electronic systems can be simplified by implementing these strategies, resulting in more practical and reliable deployment of optical systems across diverse applications and industries. Indeed, the pursuit of sustainable computing solutions, considering both operational and manufacturing aspects, remains a key focus in advancing the energy efficiency of optical computing technologies.
5 Optical interconnects in data centers
The advantages of optical interconnect in data centers include wide bandwidth, ultra-low latency, and reduced signal attenuation, thereby enhancing communication performance and overall system efficiency. Optical interconnect has high-bandwidth solutions and low latency advantages, rendering them perfect for data centers. [34] also say optical communication technology has a great potential to increase the data transfer rates within and between DCs. It also reduces latency, thus improving the performance of a system in general [34]. For instance, [35] review the performance corresponding to the three intra-DC situations studied: uncompensated SMF propagation at 120, 560, and 960 m. The findings are compiled in Figure 2, which displays the BER evolution against the received optical power for each scenario where successful post-FEC communication is achieved. Additionally, a table summarizing the optimal sensitivity results for all combinations, FEC codes, and equalization strategies that were taken into consideration is included. Optical signals suffer significantly lesser attenuation over long-haul transmissions when compared to electrical waveforms. This characteristic of optical interconnects helps to preserve signal integrity, providing accurate communication between different elements in a data center. Reduced signal degradation helps to maintain high-speed data transfer [36]. In this sense, the benefits of optical interconnect in terms of high bandwidth capacity, low latency levels, and minimal signal attenuation contribute to increased communication efficiency and overall system performance level that makes use of such a technology helpful in improving data centers.
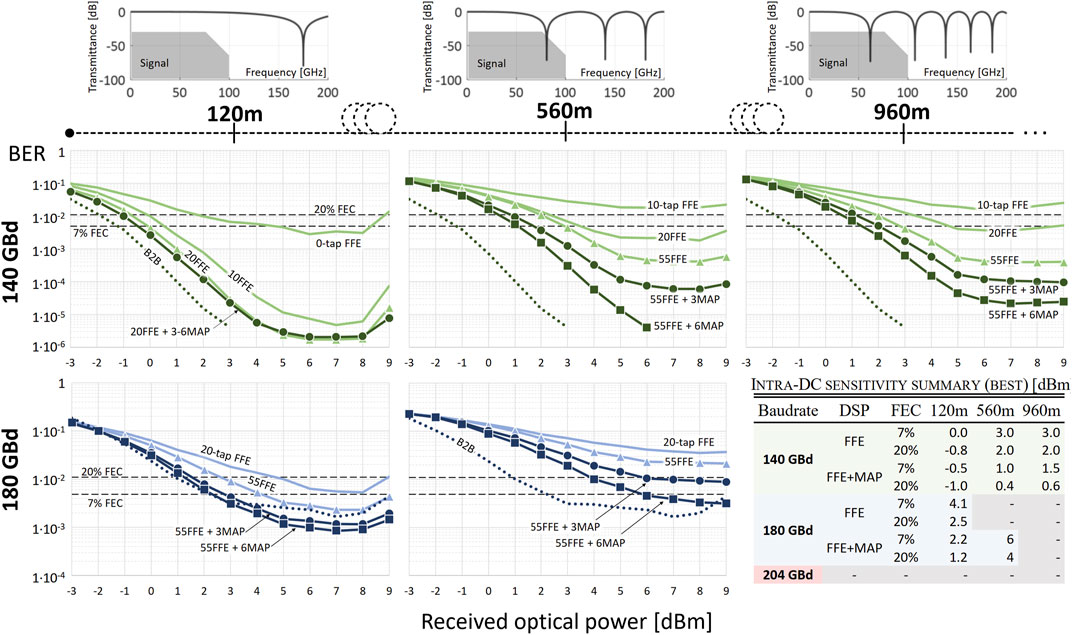
FIGURE 2. Bit-error rate (BER) vs. received optical power into the photodiode for all combinations of intra-DC transmission distances [35].
However, the cost, complexity, and compatibility challenges must be thoroughly addressed for implementation. Optical interconnects are expensive and can be complicated. Optical communication in data centers necessitates specialized equipment and infrastructure. The initial configuration costs and the complexities involved with maintenance may be hurdles for organizations that want to implement optical interconnects [27]. In addition, incorporating optical interconnects alongside installed electronic infrastructure in data centers is not easy. Achieving seamless compatibility between optical and electronic components requires additional interfaces and may necessitate modifications to the existing infrastructure. Overcoming compatibility challenges would enable the successful deployment of optical interconnects in data centers. However, utilizing optical interconnects for data center deployment is subject to a few constraints related to their compatibility [37]. As data centers evolve to meet increasing demands for higher performance and efficiency, exploring and resolving these challenges is crucial for realizing the full potential of optical interconnects in transforming data center architectures. Such limitations are, however, being addressed by continuous research and advances in optical communication technologies that will make the application of optical interconnects a real possibility for modern data centers [38–40].
Various cost-effective approaches can be adopted to make optical interconnect technology more accessible for organizations despite its cost, complexity, and specialized infrastructure requirements. For instance, just as in other systems, standardization and modularization efforts can promote interoperability, lower development costs, and facilitate mass production of optical components [41, 42]. Modular designs allow flexible integration into existing infrastructure, minimizing the need for specialized equipment and custom solutions. The innovative world continuously embraces open-source development models that encourage collaboration and innovation within the optical interconnect community, reducing costs and fostering a broader ecosystem of compatible hardware and software solutions [43]. Likewise, using commercial off-the-shelf (COTS) components would offer affordability, standardization, and availability due to economies of scale, reducing reliance on custom-designed solutions and minimizing development time. Integrating COTS components also reduces development time and minimizes reliance on proprietary technologies [44]. Moreover, designing optical interconnect solutions that seamlessly integrate with existing electronic infrastructure can reduce deployment costs and complexity as hybrid approaches would allow organizations to maximize their current investments while gradually transitioning to some optical technologies. Furthermore, collaborative research initiatives involving academia, industry, and government agencies can help pool resources and expertise, fostering knowledge exchange and cost-sharing arrangements to tackle common challenges in developing optical interconnect solutions.
These cost-effective approaches can help organizations overcome barriers to optical interconnect adoption and harness its benefits for improved computing performance and scalability. Scalable deployment models enable organizations to start small and expand gradually, mitigating financial risks and ensuring alignment with organizational objectives. Pilot projects and phased rollouts allow testing, optimization, and cost control before full-scale deployment, facilitating a smooth transition to optical technologies. Ultimately, embracing these strategies can enable organizations to unlock the potential of optical interconnect technology to drive innovation, efficiency, and competitiveness in computing systems.
6 Quantum computing with optics
Significant benefits are associated with quantum computing using optics, including quantum entanglement for information processing and parallelism in quantum operations. For instance, optical systems play a crucial role in some quantum computing techniques based on quantum entanglement principles. [45] and [46] outline the implementation of optical elements, including photons that create entangled states and allow quantum gates necessary for operation with quantum information. In addition, optical elements in quantum computing make parallel quantum computations possible. Techniques such as superposition illustrate quantum parallelism, which allows multiple inputs to be processed simultaneously [47]. It can substantially accelerate specific quantum algorithms; see the discussion of quantum optical computing [48, 49]. These qualities are auspicious properties that can solve some computational problems better than classical computing.
The discipline has problems, including quantum decoherence and designing building optical components. The decoherence is found in quantum systems, including those using optics. The delicate quantum states are disturbed by environmental factors and interactions with external systems, which results in errors during computations. Quantum decoherence is one of the challenges in quantum optical computing, as [50] and [51] described. The complexity and developmental difficulty of quantum optical assemblies are also alike because the strict oversight of quantum states and coherence requires highly developed technologies. Scalability and ease of use are also undermined by the challenges associated with quantum optical elements, especially in systems that become very large or host dense nodes [52].
Quantum optics research is still concerned with these constraints and the development of working and scalable quantum computing solutions. Advancements in technology to mitigate quantum decoherence effects and ensure the stability of quantum states for practical applications are crucial for the progress of quantum optical computing. One of the major approaches involves developing efficient error correction codes specifically tailored for quantum computing systems [53]. Quantum error correction codes, such as the surface code, encode quantum information redundantly to detect and correct errors caused by decoherence, thereby enhancing the stability of quantum computations [54, 55]. Complementing error correction, specialized hardware implementations for quantum error correction circuits and techniques, such as syndrome measurement and feedback control, can actively monitor and correct errors in real-time, bolstering the resilience of quantum states against decoherence.
Moreover, exploring strategies to avoid or minimize the occurrence of quantum errors can complement error correction approaches. Techniques such as dynamical decoupling and the quantum Zeno effect aim to prolong the coherence time of quantum states by actively suppressing decoherence processes through controlled interactions with the environment [56, 57]. Additionally, implementing environmental shielding measures to isolate quantum computing systems from external noise sources, such as cryogenic cooling systems in ultra-low temperature environments, can stabilize quantum states by reducing thermal fluctuations and environmental disturbances [58]. Studies are also investigating topological quantum computing approaches, which rely on robust qubits with intrinsic protection against local errors, offering a promising avenue to mitigate decoherence effects [55, 59]. These technological advancements collectively enhance the stability and performance of quantum computing hardware, advancing the realization of practical quantum optical computing systems. That said, quantum optical computing is a promising option to fundamentally alter the type of computation performed, resolving current intractable classical computer problems.
7 Optical neural networks for machine learning
Optical neural networks for machine learning exhibit merits such as parallel matrix-vector multiplications and the potential for high-speed training. Optical neural networks have demonstrated the capability to perform parallel matrix-vector multiplications, a critical operation in many machine learning algorithms [60–62]. It is based on the parallel processing of light by optical systems, which can expedite these operations, significantly speeding up training and inferencing stages for neural networks [34]. The parallelism inherent in optical processing can contribute to the high-speed training of neural networks [63, 64]. Optical neural networks can process multiple data points simultaneously, reducing the time required for training large-scale models [13]. These advantages can have a direct influence on the neural network operations performance.
Nevertheless, challenges related to the complexity of optical implementations and the limited flexibility in network architecture should be addressed to enable the successful integration of optical neural networks into the broader landscape of machine learning [14, 65]. Sub-optimal precision and performance might involve resolving issues associated with device development that need optimization at a system level [66]. However, even in adjusting and reconfiguring optical networks to do different jobs or changing the demands–these constraints might not be easily surmounted. Successfully overcoming challenges will ensure that optical neural networks find diverse implementations in machine learning [34, 61]. Therefore, continuous research is necessary to improve optical neural network designs to increase flexibility and maximize performance in various machine learning tasks.
8 Secure communication with optical quantum key distribution
Advantages of secure communication using optical quantum key distribution include unbreakable encryption via quantum entanglement, detection of eavesdropping attempts, globally available networks for secured communications, and information-theoretic security. Quantum key distribution (QKD) uses quantum entanglement to build secure communication channels. According to [67] and [68], the unique characteristics of entangled photons allow for producing keys that, as a rule, can be cryptographically broken with encryption but provide high communication security levels. Also, QKD systems can notice the intruders’ efforts. Quantum mechanics principles mean that the quantum information interception changes it by unveiling the states, an alert about a possible eavesdropper. This function increases the security of light quantum communication [68, 69]. Moreover, optical QKD allows for establishing safe communication chains stretching over long distances. This function is vital to creating an internationally secure communication network. Quantum communication investigates the feasibility of creating global networks based on secure communications to work with optical QKD [70, 71]. Finally, optical QKD is information-theoretically secure communication based on physical laws. It significantly differs from classical cryptographic approaches based on computational complexity assumptions. Optical QKD has the information-theoretic security feature as one of its fundamental benefits that guarantees secrecy in transmitted data.
However, practical technical obstacles, low transmission rates, and infrastructure requirements should be addressed to make optical quantum communication more prevalent. It may be hard to implement optical QKD systems in real-life settings. Energy issues connected to quantum information transmission over long distances, state channel stability, and optical-channel decay losses should be addressed so the world can adopt secure communication networks [72, 73]. Additionally, the transmission rates of current optical QKD systems are much lower than those in traditional communication approaches [74]. However, there are essential advances in transmission rates, while current technology may not be good enough for applications that have to go sky-high [69, 75]. In addition, setting up secure communication with optical QKD might involve a unique infrastructure. Implementing quantum repeaters and other elements to increase the reach capability would require significant financial resources [76].
Nonetheless, multiple improvements can be made to increase the transmission rates of current optical QKD systems for practical, real-world applications. For instance, increasing the quantum efficiency of QKD systems by adopting advanced single-photon detectors with heightened sensitivity is a promising avenue [77, 78]. This approach enhances the signal-to-noise ratio, facilitating faster key generation rates while upholding security protocols. Furthermore, parallelization techniques can be instrumental in elevating transmission rates by simultaneously producing multiple quantum keys [79, 80]. Implementing multiplexing schemes like time-bin encoding or wavelength division multiplexing enables the transmission of multiple quantum signals over a single optical channel, effectively amplifying the critical generation rate [81, 82].
Furthermore, overcoming infrastructural and financial barriers is pivotal for the widespread adoption of secure optical QKD systems. Standardization efforts in QKD protocols and hardware interfaces promote interoperability and integration with existing communication networks, mitigating compatibility issues and reducing implementation costs [83–85]. Moreover, scalable QKD solutions offer a cost-effective deployment strategy, allowing for flexible adaptation in large-scale networks and smaller-scale applications [86–88]. Collaboration between public and private sectors can also be crucial, stimulating investment in QKD infrastructure and technology development. Through public-private partnerships, funding, resources, and expertise can be pooled to accelerate the deployment of secure optical QKD systems, fostering widespread adoption and paving the way for secure communication networks in various real-world applications.
9 Optical computing in signal and image processing
The advantages of optical computing for signal/image processing include parallelism for fast treatment, control implementation via spatial light modulators in real-time applications, and proficient Fourier transformation tasks involving image processing from optical computing’s parallel architecture. According to [89], optical systems are much more efficient than sequential electrical approaches in image processing because they can process several pixels simultaneously. First, real-time signal and image processing are offered by SLMs in optical computing. Allowing dynamic and adaptive processing enables SLMs to regulate light amplitude, phase, or polarization. This feature can benefit from Applications requiring quick modifications or real-time feedback [90]. Fourier transform operations benefit significantly from optical computing, which is essential for signal and image processing tasks. Fourier transform computations are made parallel in optics via parallelism, which speeds up methods for image reconstruction and pattern recognition [64, 91]. Signal and image treatment techniques may benefit significantly from optical computing features, including rapid Fourier transformation, parallel image processing, and real-time capabilities offered by spatial light modulators.
However, challenges regarding the complexity of optics-based setups and adaptability constraints must be addressed to successfully incorporate optical computing into various signal and image processing applications. Creating intricate optical computing systems for signal and image processing may be necessary. While maintaining long-term stability is one concern, further refinement of the optical components’ design and alignment may require specific requirements. For practical and widespread usage in signal and image processing applications, it is imperative to address the complexity of optical setups [91, 92]. Furthermore, specialized optical systems designed for specific signal or image processing operations could be less versatile and adaptable than electrical processors with software installed. An optical system’s adaptability for applications requiring frequent adjustments or shifting dynamic processing requirements may be limited if incorporating functional improvements requires physical setup changes [93]. However, recent research looks at novel designs that may capitalize on these different advantages while reducing the complexity of setups and increasing flexibility.
10 Conclusion
Examining optical gains for computing from various angles reveals a bright horizon with unique advantages and disadvantages. Based on the properties of light, optical computing has unique benefits in specific applications and obstacles that need careful consideration.
10.1 Parallel processing and massive data handling
Optical computing has become a potent option because its parallel processing capacity allows it to handle enormous databases effectively. Parallelism’s capacity for simultaneous processing offers unmatched speed in data analysis and computational tasks. However, challenges in integration with existing electronic systems should be addressed fully to unlock its potential.
10.2 High-speed signal processing
Optical computing may operate at high speed, which opens a world of possibilities for real-time applications because light propagates. Among the advantages are better data throughput rates, reduced latency, and the possibility of faster calculations. Nonetheless, the intricacy of the implementation and design of high-speed optical systems poses challenges that require innovative solutions.
10.3 Energy efficiency
Optical computing is known for its exceptional energy efficiency, especially during operation when it consumes less power and produces less heat. The entire energy calculation cannot ignore the challenges of manufacturing and integration, which in turn need an integrated approach to ensure overall sustainability in the broader computing context.
10.4 Optical interconnects in data centers
Data centers, which provide high capacity and low latency, are prime examples of the benefits of optical interconnects. These advantages facilitate improved system performance and communication. Optic interconnects must, however, be readily integrated into current data center infrastructures, posing significant obstacles in terms of cost and complexity.
10.5 Quantum computing
Quantum computing employs optics and brings new characteristics like invulnerable encryption from quantum entanglement and parallelization of many computer tasks. Although the benefits mentioned above are significant, this discipline is challenged by quantum decoherence and the intricate construction of optical components. The future of quantum computing will depend on how these problems are resolved.
10.6 Optical neural networks for machine learning
Optical neural networks present potential benefits of high-speed training of neural networks and parallel matrix-vector multiplications. However, the complications of optical deployments and restrictions regarding network structure adaptability are challenges. Future developments should focus on simplifying designs and achieving an increase in adaptability to a variety of machine-learning applications.
10.7 Secure communication
Optical quantum key distribution provides unprecedented security via the entanglement principle and eavesdropping detection. Although these properties have put optical quantum communication at the center of secure communications, implementation challenges and limited transmission rates require further investigation and technological improvement.
10.8 Optical computing in signal and image processing
Parallelization, real-time processing, and Fourier transform operations are some of the strengths of optical computing in signal and image processing. However, the complexity of optical setups and poor adaptability must also be addressed. Future developments could also include optimizing optical systems for greater practicality in applications outside of signal and image processing.
11 Recommendation
The future of harnessing the merits of optics in computing needs a multidimensional approach. Addressing integration challenges, manufacturing complexities, and infrastructure requirements across various domains is crucial. Researchers, engineers, and industry stakeholders should collaborate to propel optical computing from theoretical promise to practical implementation. Besides, progressive developments in material science, innovative component designs, and standardized integration protocols will play important roles in improving the world of optical computing. Interdisciplinary collaboration between quantum physicists, computer scientists, and engineers will also be vital for unlocking the full potential of quantum computing with optics. As technologies evolve, smooth integration of optical and electronic systems may become possible, enabling an age in which sensitivities offered by optics are deployed for a broad range of computing uses. The continuous search for efficiency, security, and adaptability will lead the way in the evolution of optical computing, lighting our future with a computational paradigm that harnesses light speed and accuracy.
Author contributions
CK: Investigation, Resources, Writing–original draft, Writing–review and editing. YL: Investigation, Resources, Supervision, Writing–review and editing. JT: Writing–review and editing, Validation, Investigation, Resources, Funding acquisition.
Funding
The author(s) declare financial support was received for the research, authorship, and/or publication of this article. Applied Basic Research Program of Liaoning Province (2022JH2/101300123). University-local government scientific and technical cooperation cultivation project of Ordos Institute-LNTU, (YJY-XD-2023-011). Scientific Research General Program of Department of Education in Liaoning Province (JYTMS20230810).
Acknowledgments
The authors would like to acknowledge and thank postgraduate students and academics at Liaoning Technical University, who assisted during the editing process of the article. The authors thank the editor and reviewers for their valuable time in reviewing the manuscript.
Conflict of interest
The authors declare that the research was conducted in the absence of any commercial or financial relationships that could be construed as a potential conflict of interest.
Publisher’s note
All claims expressed in this article are solely those of the authors and do not necessarily represent those of their affiliated organizations, or those of the publisher, the editors and the reviewers. Any product that may be evaluated in this article, or claim that may be made by its manufacturer, is not guaranteed or endorsed by the publisher.
References
1. Hofmann O, Stollenwerk J, Loosen P. Design of multi-beam optics for high throughput parallel processing. J Laser Appl (2020) 32(1):012005. doi:10.2351/1.5125778
2. Kazanskiy NL, Butt MA, Khonina SN. Optical computing: status and perspectives. Nanomaterials (2022) 12(13):2171. doi:10.3390/nano12132171
3. Feldmann J, Youngblood N, Karpov M, Gehring H, Li X, Stappers M, et al. Parallel convolutional processing using an integrated photonic tensor core. Nature (2021) 589(7840):52–8. doi:10.1038/s41586-020-03070-1
4. Schadt EE, Linderman MD, Sorenson J, Lee L, Nolan GP. Computational solutions to large-scale data management and analysis. Nat Rev Genet (2010) 11(9):647–57. doi:10.1038/nrg2857
5. Biberman A, Bergman K. Optical interconnection networks for high-performance computing systems. Rep Prog Phys (2012) 75(4):046402. doi:10.1088/0034-4885/75/4/046402
6. Chang J, Sitzmann V, Dun X, Heidrich W, Wetzstein G. Hybrid optical-electronic convolutional neural networks with optimized diffractive optics for image classification. Scientific Rep (2018) 8(1):12324. doi:10.1038/s41598-018-30619-y
7. Wang T, Arrathoon R. Limits of optical and electrical fan-out versus power and fan-out versus bandwidth. Spinger (1990). p. 133–49. doi:10.1117/12.21307
8. Schares L, Lee BG, Checconi F, Budd R, Rylyakov A, Dupuis N, et al. A throughput-optimized optical network for data-intensive computing. IEEE Micro (2014) 34(5):52–63. doi:10.1109/MM.2014.77
9. Duncan TS, Voas JK, Eager RJ, Newey SC, Wynia JL. Low-latency adaptive optics system processing electronics. In: Adaptive optical system technologies II. Proceedings of the SPIE. SPIE (2003). doi:10.1117/12.458955
10. McMahon PL. The physics of optical computing. Nat Rev Phys (2023) 5(12):717–34. doi:10.1038/s42254-023-00645-5
11. Tkach RW. Scaling optical communications for the next decade and beyond. Bell Labs Tech J (2010) 14(4):3–9. doi:10.1002/bltj.20400
12. True Merrill J, Volin C, Landgren D, Amini JM, Wright K, Charles Doret S, et al. Demonstration of integrated microscale optics in surface-electrode ion traps. New J Phys (2011) 13(10):103005. doi:10.1088/1367-2630/13/10/103005
13. Rudolph T. Why I am optimistic about the silicon-photonic route to quantum computing. APL Photon (2017) 2(3):4976737. doi:10.1063/1.4976737
14. Colburn S, Chu Y, Shilzerman E, Majumdar A. Optical frontend for a convolutional neural network. Appl Opt (2019) 58(12):3179. doi:10.1364/AO.58.003179
15. Agrell E, Karlsson M, Chraplyvy AR, Richardson DJ, Krummrich PM, Winzer P, et al. Roadmap of optical communications. J Opt (2016) 18(6):063002. doi:10.1088/2040-8978/18/6/063002
16. Miller DAB. Rationale and challenges for optical interconnects to electronic chips. Proc IEEE (2000) 88(6):728–49. doi:10.1109/5.867687
17. Werner S, Navaridas J, Luján M. A survey on optical network-on-chip architectures. ACM Comput Surv (2018) 50(6):1–37. doi:10.1145/3131346
18. Gaeta AL, Lipson M, Kippenberg TJ. Photonic-chip-based frequency combs. Nat Photon (2019) 13(3):158–69. doi:10.1038/s41566-019-0358-x
19. Butt MA. Integrated optics: platforms and fabrication methods. Encyclopedia (2023) 3(3):824–38. doi:10.3390/encyclopedia3030059
20. Kobayashi T, Hamaoka F, Nakamura M, Yamazaki H, Nagatani M, Miyamoto Y. Ultrahigh-speed optical communications technology combining digital signal processing and circuit technology. NTT Tech Rev (2019) 17(5):12–9. doi:10.53829/ntr201905fa2
21. Li H-YS, Qiao Y, Psaltis D. Optical network for real-time face recognition. Appl Opt (1993) 32(26):5026. doi:10.1364/AO.32.005026
22. Marin-Palomo P, Kemal JN, Karpov M, Kordts A, Pfeifle J, Pfeiffer MHP, et al. Microresonator-based solitons for massively parallel coherent optical communications. Nature (2017) 546(7657):274–9. doi:10.1038/nature22387
23. Hamerly R, Bernstein L, Sludds A, Soljačić M, Englund D. Large-scale optical neural networks based on photoelectric multiplication. Phys Rev X (2019) 9(2):021032. doi:10.1103/PhysRevX.9.021032
24. Lundén H, Murphy L, Määttänen A, Kumpulainen T. Hermetic package for optical devices using room temperature welding technology and transparent lid, for space applications. Int Symp Microelectronics (2015) 2015(1):000598–602. doi:10.4071/isom-2015-THA31
25. Madduri S, Sammakia BG, Infantolino B, Chaparala S. A review of non-hermetic optoelectronic packaging. In: 2008 11th Intersociety Conference on Thermal and Thermomechanical Phenomena in Electronic Systems; 28-31 May 2008; Orlando, FL, USA. IEEE (2008). p. 913–9. doi:10.1109/ITHERM.2008.4544364
26. Boentoro TW, Szyszka B. Protective coatings for optical surfaces. In: Optical thin films and coatings. Elsevier (2013). p. 540–63. doi:10.1533/9780857097316.4.540
27. Duprez H, Sivre E, Anthore A, Aassime A, Cavanna A, Ouerghi A, et al. Macroscopic electron quantum coherence in a solid-state circuit. Phys Rev X (2019) 9(2):021030. doi:10.1103/PhysRevX.9.021030
28. Safavi-Naeini AH, Van Thourhout D, Baets R, Van Laer R. Controlling phonons and photons at the wavelength scale: integrated photonics meets integrated phononics. Optica (2019) 6(2):213. doi:10.1364/OPTICA.6.000213
29. Minzioni P, Lacava C, Tanabe T, Dong J, Hu X, Csaba G, et al. Roadmap on all-optical processing. J Opt (2019) 21(6):063001. doi:10.1088/2040-8986/ab0e66
30. Ying Z, Dhar S, Zhao Z, Feng C, Mital R, Chung C-J, et al. Electro-optic ripple-carry adder in integrated silicon photonics for optical computing. IEEE J Selected Top Quan Elect (2018) 24(6):1–10. doi:10.1109/JSTQE.2018.2836955
31. Demkov AA, Bajaj C, Ekerdt JG, Palmstrøm CJ, Ben Yoo SJ. Materials for emergent silicon-integrated optical computing. J Appl Phys (2021) 130(7):070907. doi:10.1063/5.0056441
32. Ying Z, Feng C, Zhao Z, Soref R, Pan D, Chen RT. Integrated multi-operand electro-optic logic gates for optical computing. Appl Phys Lett (2019) 115(17). doi:10.1063/1.5126517
33. Suhir E. The future of microelectronics and photonics, and the role of mechanical, materials and reliability engineering. In: Proceedings of the International Conference on Materials in Microelectronics; 10-10 October 1997; Singapore. IEEE (2000).
34. Van der Sande G, Brunner D, Soriano MC. Advances in photonic reservoir computing. Nanophotonics (2017) 6(3):561–76. doi:10.1515/nanoph-2016-0132
35. Estaran JM, Mardoyan H, Jorge F, Ozolins O, Udalcovs A, Konczykowska A, et al. 140/180/204-Gbaud OOK transceiver for inter- and intra-data center connectivity. J Lightwave Techn (2019) 37(1):178–87. doi:10.1109/JLT.2018.2876732
36. Singh H, Mittal N, Miglani R, Singh H, Gaba GS, Hedabou M. Design and analysis of high-speed free space optical (FSO) communication system for supporting fifth generation (5G) data services in diverse geographical locations of India. IEEE Photon J (2021) 13(5):1–12. doi:10.1109/JPHOT.2021.3113650
37. Shen Y, Harris NC, Skirlo S, Prabhu M, Baehr-Jones T, Hochberg M, et al. Deep learning with coherent nanophotonic circuits. Nat Photon (2017) 11(7):441–6. doi:10.1038/nphoton.2017.93
38. Chen J, Gong Y, Fiorani M, Aleksic S. Optical interconnects at the top of the rack for energy-efficient data centers. IEEE Commun Mag (2015) 53(8):140–8. doi:10.1109/MCOM.2015.7180521
39. Ben Yoo SJ. Prospects and challenges of photonic switching in data centers and computing systems. J Lightwave Techn (2022) 40(8):2214–43. doi:10.1109/JLT.2021.3136570
40. Thraskias CA, Lallas EN, Neumann N, Schares L, Offrein BJ, Henker R, et al. Survey of photonic and plasmonic interconnect technologies for intra-datacenter and high-performance computing communications. IEEE Commun Surv Tutorials (2018) 20(4):2758–83. doi:10.1109/COMST.2018.2839672
41. Brutti A, De Sabbata P, Frascella A, Gessa N, Ianniello R, Novelli C, et al. Smart city platform specification: a modular approach to achieve interoperability in smart cities. In: The internet of things for smart urban ecosystems. Spinger (2019). p. 25–50. doi:10.1007/978-3-319-96550-5_2
42. Gepp M, Foehr M, Vollmar J, Schertl A, Schaeffler T. System integration in modularization and standardization programs. In: 2015 Annual IEEE Systems Conference (SysCon) Proceedings; 13-16 April 2015; Vancouver, BC, Canada. IEEE (2015). p. 847–52. doi:10.1109/SYSCON.2015.7116856
43. Chesbrough H. The future of open innovation. Research-Technology Manag (2017) 60(1):35–8. doi:10.1080/08956308.2017.1255054
45. Ho R, Mai KW, Horowitz MA. The future of wires. Proc IEEE (2001) 89(4):490–504. doi:10.1109/5.920580
46. Weaver MJ, Duivestein P, Bernasconi AC, Scharmer S, Lemang M, Thiel TCV, et al. An integrated microwave-to-optics interface for scalable quantum computing. Nat Nanotechnology (2023) 19:166–72. doi:10.1038/s41565-023-01515-y
47. Ramezani SB, Sommers A, Manchukonda HK, Rahimi S, Amirlatifi A. Machine learning algorithms in quantum computing: a survey. In: 2020 International Joint Conference on Neural Networks (IJCNN); 19-24 July 2020; Glasgow, UK. IEEE (2020). p. 1–8. doi:10.1109/IJCNN48605.2020.9207714
48. Kia B, Lindner JF, Ditto WL. Nonlinear dynamics as an engine of computation. Phil Trans R Soc A: Math Phys Eng Sci (2017) 375(2088):20160222. doi:10.1098/rsta.2016.0222
49. Guidry MA, Lukin DM, Yang KY, Trivedi R, Vučković J. Quantum optics of soliton microcombs. Nat Photon (2022) 16(1):52–8. doi:10.1038/s41566-021-00901-z
50. Fisher AJ. Quantum computing in the solid state: the challenge of decoherence. Phil Trans R Soc Lond Ser A: Math Phys Eng Sci (2003) 361(1808):1441–50. doi:10.1098/rsta.2003.1213
51. Fabre C, Treps N. Modes and states in quantum optics. Rev Mod Phys (2020) 92(3):035005. doi:10.1103/RevModPhys.92.035005
52. Zhang X, Kwon K, Henriksson J, Luo J, Wu MC. A large-scale microelectromechanical-systems-based silicon photonics LiDAR. Nature (2022) 603(7900):253–8. doi:10.1038/s41586-022-04415-8
54. Holmes A, Jokar MR, Pasandi G, Ding Y, Pedram M, Chong FT. NISQ+: boosting quantum computing power by approximating quantum error correction. In: 2020 ACM/IEEE 47th annual international symposium on computer architecture (ISCA). ACM (2020). p. 556–69. doi:10.1109/ISCA45697.2020.00053
55. Sihotang HT, Siringoringo R, Riandari F, Song JL, Sim LC. Development of stable qubits and error correction in quantum computer architecture for superconducting quantum processors. J Comp Sci Res (Jocosir) (2023) 1(4):104–9.
56. Harrington PM, Mueller EJ, Murch KW. Engineered dissipation for quantum information science. Nat Rev Phys (2022) 4(10):660–71. doi:10.1038/s42254-022-00494-8
57. Liu G-Q, Po HC, Du J, Liu R-B, Pan X-Y. Noise-resilient quantum evolution steered by dynamical decoupling. Nat Commun (2013) 4(1):2254. doi:10.1038/ncomms3254
58. Spivey RF, Inlek IV, Jia Z, Crain S, Sun K, Kim J, et al. High-stability cryogenic system for quantum computing with compact packaged ion traps. IEEE Trans Quan Eng (2022) 3:1–11. doi:10.1109/TQE.2021.3125926
59. Koh JM, Tai T, Phee YH, Ng WE, Lee CH. Stabilizing multiple topological fermions on a quantum computer. Npj Quan Inf (2022) 8(1):16. doi:10.1038/s41534-022-00527-1
60. Zhang H, Gu M, Jiang XD, Thompson J, Cai H, Paesani S, et al. An optical neural chip for implementing complex-valued neural network. Nat Commun (2021) 12(1):457. doi:10.1038/s41467-020-20719-7
61. Sui X, Wu Q, Liu J, Chen Q, Gu G. A review of optical neural networks. IEEE Access (2020) 8:70773–83. doi:10.1109/ACCESS.2020.2987333
62. Wang T, Ma S-Y, Wright LG, Onodera T, Richard BC, McMahon PL. An optical neural network using less than 1 photon per multiplication. Nat Commun (2022) 13(1):123. doi:10.1038/s41467-021-27774-8
63. Liu J, Wu Q, Sui X, Chen Q, Gu G, Wang L, et al. Research progress in optical neural networks: theory, applications and developments. PhotoniX (2021) 2(1):5. doi:10.1186/s43074-021-00026-0
64. Miscuglio M, Hu Z, Li S, George JK, Capanna R, Dalir H, et al. Massively parallel amplitude-only Fourier neural network. Optica (2020) 7(12):1812. doi:10.1364/OPTICA.408659
65. Marom DM, Colbourne PD, D’Errico A, Fontaine NK, Ikuma Y, Proietti R, et al. Survey of photonic switching architectures and technologies in support of spatially and spectrally flexible optical networking [invited]. J Opt Commun Networking (2017) 9(1):1. doi:10.1364/JOCN.9.000001
66. McArdle N, Naruse M, Toyoda H, Kobayashi Y, Ishikawa M. Reconfigurable optical interconnections for parallel computing. Proc IEEE (2000) 88(6):829–37. doi:10.1109/5.867696
67. Yuan Z-S, Bao X-H, Lu C-Y, Zhang J, Peng C-Z, Pan J-W. Entangled photons and quantum communication. Phys Rep (2010) 497(1):1–40. doi:10.1016/j.physrep.2010.07.004
68. Shannon K, Towe E, Tonguz OK. On the use of quantum entanglement in secure communications: a survey (2020). arXiv.
69. Stallings W. Data and computer communications. 8th ed. Upper Saddle River, New Jersey: Pearson Prentice Hall (2007).
70. Deal WR, Leong K, Yoshida W, Zamora A, Mei XB. InP HEMT integrated circuits operating above 1,000 GHz. IEEE Int Electron Devices Meet (Iedm) (2016) 29:11–29. doi:10.1109/IEDM.2016.7838502
71. Saade A, Caltagirone F, Carron I, Daudet L, Dremeau A, Gigan S, et al. Random projections through multiple optical scattering: approximating Kernels at the speed of light. In: 2016 IEEE International Conference on Acoustics, Speech and Signal Processing (ICASSP); 20-25 March 2016; Shanghai, China. IEEE (2016). p. 6215–9. doi:10.1109/ICASSP.2016.7472872
72. Miller DAB. Attojoule optoelectronics for low-energy information processing and communications. J Lightwave Techn (2017) 35(3):346–96. doi:10.1109/JLT.2017.2647779
73. Spitz O, Herdt A, Wu J, Maisons G, Carras M, Wong C-W, et al. Private communication with quantum cascade laser photonic chaos. Nat Commun (2021) 12(1):3327. doi:10.1038/s41467-021-23527-9
74. Mehic M, Niemiec M, Rass S, Ma J, Peev M, Aguado A, et al. Quantum key distribution. ACM Comput Surv (2021) 53(5):1–41. doi:10.1145/3402192
75. Chi Y, Huang J, Zhang Z, Mao J, Zhou Z, Chen X, et al. A programmable qudit-based quantum processor. Nat Commun (2022) 13(1):1166. doi:10.1038/s41467-022-28767-x
76. Sharma P, Agrawal A, Bhatia V, Prakash S, Mishra AK. Quantum key distribution secured optical networks: a survey. IEEE Open J Commun Soc (2021) 2:2049–83. doi:10.1109/OJCOMS.2021.3106659
77. Ceccarelli F, Acconcia G, Gulinatti A, Ghioni M, Rech I, Osellame R. Recent advances and future perspectives of single-photon avalanche diodes for quantum photonics applications. Adv Quan Tech (2021) 4(2):102. doi:10.1002/qute.202000102
78. Zhang C-X, Wu 吴 D, Cui 崔 P-W, Ma J-C, Wang 王, 玥 Y, et al. Research progress in quantum key distribution. Chin Phys B (2023) 32(12):124207. doi:10.1088/1674-1056/acfd16
79. Terhaar R, Rödiger J, Häußler M, Wahl M, Gehring H, Wolff MA, et al. Ultrafast quantum key distribution using fully parallelized quantum channels. Opt Express (2023) 31(2):2675. doi:10.1364/OE.469053
80. Wu X, Zhang B, Jin D. Parallel simulation of quantum key distribution networks. In: Proceedings of the 2020 ACM SIGSIM conference on principles of advanced discrete simulation. ACM (2020). p. 187–96. doi:10.1145/3384441.3395988
81. Kim J-H, Chae J-W, Jeong Y-C, Kim Y-H. Quantum communication with time-bin entanglement over a wavelength-multiplexed fiber network. APL Photon (2022) 7(1):73040. doi:10.1063/5.0073040
82. Fitzke E, Bialowons L, Dolejsky T, Tippmann M, Nikiforov O, Walther T, et al. Scalable network for simultaneous pairwise quantum key distribution via entanglement-based time-bin coding. PRX Quan (2022) 3(2):020341. doi:10.1103/PRXQuantum.3.020341
83. Länger T, Lenhart G. Standardization of quantum key distribution and the ETSI standardization initiative ISG-QKD. New J Phys (2009) 11(5):055051. doi:10.1088/1367-2630/11/5/055051
84. Cao Y, Zhao Y, Wang Q, Zhang J, Ng SX, Hanzo L. The evolution of quantum key distribution networks: on the road to the Qinternet. IEEE Commun Surv Tutorials (2022) 24(2):839–94. doi:10.1109/COMST.2022.3144219
85. Stanley M, Gui Y, Unnikrishnan D, Hall SRG, Fatadin I. Recent progress in quantum key distribution network deployments and standards. J Phys Conf Ser (2022) 2416(1):012001. doi:10.1088/1742-6596/2416/1/012001
86. Geihs M, Nikiforov O, Demirel D, Sauer A, Butin D, Gunther F, et al. The status of quantum-key-distribution-based long-term secure internet communication. IEEE Trans Sust Comput (2021) 6(1):19–29. doi:10.1109/TSUSC.2019.2913948
87. Tysowski PK, Ling X, Lütkenhaus N, Mosca M. The engineering of a scalable multi-site communications system utilizing quantum key distribution (QKD). Quan Sci Techn (2017). doi:10.1088/2058-9565/aa9a5d
88. Guo M, Cao Y, Zhu J, Zhou X, Zhang C, Yu X, et al. Cost-effective QKD protocol upgrading for metropolitan quantum optical networks. J Opt Commun Networking (2023) 15(9):700. doi:10.1364/JOCN.496154
89. Zhang Y, Ríos C, Shalaginov MY, Li M, Majumdar A, Gu T, et al. Myths and truths about optical phase change materials: a perspective. Appl Phys Lett (2021) 118(21):54114. doi:10.1063/5.0054114
90. Matoba O, Nomura T, Perez-Cabre E, Millan MS, Javidi B. Optical techniques for information security. Proc IEEE (2009) 97(6):1128–48. doi:10.1109/JPROC.2009.2018367
91. Goodman J. Introduction to fourier optics. 3rd ed. Greenwood Village, CO, United States: Roberts and Company Publishers (2005).
92. De Mel I, Klymenko OV, Short M. Balancing accuracy and complexity in optimisation models of distributed energy systems and microgrids with optimal power flow: a review. Sustainable Energ Tech Assessments (2022) 52:102066. doi:10.1016/j.seta.2022.102066
Keywords: optical computing, parallel processing, high-speed signal processing, quantum computing, machine learning, secure communication, signal and image processing, energy efficiency
Citation: Kibebe CG, Liu Y and Tang J (2024) Harnessing optical advantages in computing: a review of current and future trends. Front. Phys. 12:1379051. doi: 10.3389/fphy.2024.1379051
Received: 30 January 2024; Accepted: 29 February 2024;
Published: 15 March 2024.
Edited by:
Sushank Chaudhary, Chulalongkorn University, ThailandReviewed by:
Abhishek Sharma, Guru Nanak Dev University, IndiaMeet Kumari, Chandigarh University, India
Copyright © 2024 Kibebe, Liu and Tang. This is an open-access article distributed under the terms of the Creative Commons Attribution License (CC BY). The use, distribution or reproduction in other forums is permitted, provided the original author(s) and the copyright owner(s) are credited and that the original publication in this journal is cited, in accordance with accepted academic practice. No use, distribution or reproduction is permitted which does not comply with these terms.
*Correspondence: Yue Liu, yueliu000@163.com; Jiaxi Tang, tangjiaxi1986@163.com