- 1European XFEL, Schenefeld, Germany
- 2University of Southampton, Southampton, United Kingdom
The European XFEL is a megahertz repetition-rate facility producing extremely bright and coherent pulses of a few tens of femtoseconds duration. The amount of data generated in the context of user experiments can exceed hundreds of gigabits per second, resulting in tens of petabytes stored every year. These rates and volumes pose significant challenges both for facilities and users thereof. In fact, if unaddressed, extraction and interpretation of scientific content will be hindered, and investment and operational costs will quickly become unsustainable. In this article, we outline challenges and solutions in data reduction.
1 Introduction
Scientific ambition pushes the progress of modern X-ray light sources. As a result of the steady evolution in accelerator and detector technology, as well as increased levels of automation and improved quasi-real-time feedback, the amount of experimental data produced by photon sources is increasing at unprecedented rates [1, 2]. In particular, the continuous development and improvement of X-ray imaging detectors (see, e.g., [3], and references therein) is instrumental to enable the scientific exploitation of the exceptional brightness characteristic of fourth-generation light sources [4] and X-ray free electron lasers (XFELs) (see, e.g., [5, 6]). State-of-the-art pixelated X-ray detectors, custom-made or commercially available, can routinely collect hundreds to a few thousands images per second [3, 7–14]. Among modern facilities, the European XFEL is a MHz-repetition-rate X-ray free electron laser providing extremely bright, spatially coherent pulses, which are characterized by a temporal duration of tens of femtoseconds or less [15, 16]. The facility operates in a so-called “burst mode,” delivering 10 Hz trains of up to 2,700 X-ray pulses. The intra-pulse separation can be as low as 222 ns, equivalent to a repetition rate of 4.5 MHz.
The detector data rates at the European XFEL can exceed one hundred gigabits per second, resulting in the production of several petabytes of data for a single experiment with a typical duration of two to 6 days. The MHz-capable detectors at the European XFEL are the DEPFET Sensor with Signal Compression (DSSC) [12], the Adaptive Gain Integrating Pixel Detector (AGIPD) [10, 11], and the Large Pixel Detector (LPD) [8, 9], each of which has up to 1024 × 1024 pixels. When integrated into our infrastructure, the maximum data rates are 134 Gbit/s, 118 Gbit/s, and 86 Gbit/s, respectively. Additionally, digitizers’ data rates can approach several gigabits per second, and multiple of these devices may be employed during a single experiment. As a result, the volume of scientific data collected during user experiments has steadily increased since operation began in 2017. This is illustrated by Figure 1, where the total amount of raw data (its precise definition is given in Section 2.1)—about 100 PiB as of today—is shown as a function of time. Apart from a deceleration caused by a reduced number of experiments during the most acute phase of the COVID-19 pandemic, the rate of data collection is ever-growing. This can be explained by the asynchronous start of the seven scientific instruments, as highlighted in Figure 1, as well as the continuous enhancement in operational efficiency—from accelerator and instrument performances to data systems reliability, from procedure optimisation and automation to advances in sample delivery. While operational efficiency cannot increase indefinitely, future facility upgrades will inevitably result in higher data throughput. In the short term, for example, an AGIPD of 3.7 Mpx, with a theoretical data rate approaching half a terabit per second will be installed. In the medium term, upgrades of the accelerator will increase its duty factor, and in turn the number of X-ray pulses delivered each second [16, 17]. Additionally, the current scientific data policy1 defines that scientific data at European XFEL shall be curated for at least five years although striving for ten.
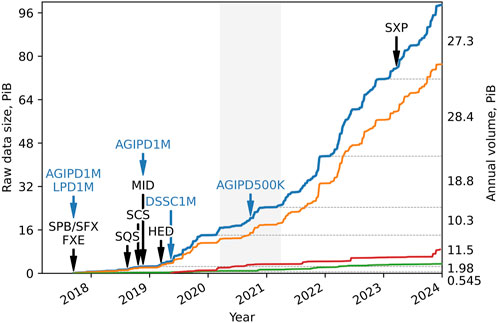
FIGURE 1. The blue line shows the total amount of data collected (raw data) in pebibytes (PiB) at European XFEL as a function of time since the beginning of operation. The orange, green and red lines show the subset of data produced by the AGIPD, LPD and DSSC detectors, respectively. The black arrows indicate the start of instrument operations, blue arrows show the installation of megahertz imaging detectors, the gray span corresponds to reduced operation due to the COVID-19 pandemic. The labels of imaging detectors are suffixed either by 1M or 500K, which indicates the approximate number of pixels.
Storage systems are expensive and limited in lifetime, they consume electric energy, increase CO2 emissions, and require dedicated personnel for their maintenance and operation. The resulting non-negligible economical and environmental footprint must be urgently addressed. This means that, altogether, a continuous expansion of the storage system is not sustainable.
While storage-related issues are the most evident, the enormous data rates and volumes pose other challenges, both from a technical and a scientific point of view. In fact, the complex solutions required to handle the enormous data rates often necessitate using leading-edge technology. This is expensive and requires deep expert knowledge to keep the systems stable. In operation, these systems may be prone to instabilities—like the degradation of their performances—which in turn, could potentially disrupt data acquisition and near real-time monitoring of the experiments. The data coming from the MHz-capable detectors is not trivial to interpret, and the European XFEL has been developing the so-called correction pipeline to transform it into physics content [18]. This pipeline is typically triggered automatically as soon as data is collected, and results in additional data transfer, processing, and storage requirements. As exemplified by the correction pipeline, the analysis of large amounts of data typically requires software that can exploit multiple computational nodes, and cope with latencies inherent to ingesting data at high rates. Accordingly, distilling scientific content can be considerably more challenging with a larger data volume, and can be potentially compromised if data is not pre-processed by specialized tools developed by experts. More complex analysis methods, in turn, increase latency, which is particularly detrimental when using analysis results to steer the running experiments. The additional complexity can even represent an insurmountable barrier for inexperienced users, which makes the facility less accessible to test new scientific methodologies and ideas.
The only solution to the aforementioned issues is to reduce the amount of data, while maximizing its scientific value. Generally, several reduction operations can be performed during processing and evaluation of collected data. These are either data selections—rarely the entirety of collected data is used—or data transformations, e.g., dimensionality reduction through integration along some variable.
While the previous discussion revolved around the use case of the European XFEL, the issues encountered are by no means specific to our facility. Large-scale high-energy particle physics facilities, for instance, have embedded their data reduction strategy as part of their original technical design decades ago (see, for example, Refs. [19–21]). Additionally, most of the modern X-ray photon sources are exploring and developing data reduction strategies [22], also owing to initiatives of policymakers such as European Union’s Horizon 2020 LEAPS-INNOV, and the results of individual research groups [23–30]. Topics explored vary from lossless and lossy compression [31] to artificial intelligence [25, 26, 32, 33], from dedicated hardware solutions to FAIR data [34].
Even though the benefits are clear to both facilities and users, several open questions and challenges remain from a technical, scientific, and social point of view. Overall, the risk of data reduction introducing bias in the results must be minimized, and the ratio of scientific content over collected data maximized. That is, only the data contributing to the answer of a specific scientific question should ideally be curated. Scientists must be given control of the reduction pipeline, including access to detailed validation metrics.
Reducing data is not avoidable anymore at the European XFEL. It is our duty to provide tools that enable users to perform data reduction, thereby maximizing the scientific outcome of the experiments and minimizing the pressure on our infrastructure. These tools need to be as transparent and automated as possible, and their output must be corroborated through extensive validation. We finally aim at providing extensive and reliable information to support users’ decisions during and after experiments.
This manuscript aims to serve as an entry-point for our users as it reports on developed solutions, future plans, as well as strategies for data reduction and curation at the European XFEL. It furthermore details challenges and opportunities intrinsic to data reduction. Further documentation and continuously updated information are, and will be, made available in Ref. [35]. In Section 2, we provide an overview of the data infrastructure of the European XFEL, and its upgrade to enable integration of data reduction techniques. In Section 3, we present and discuss selected data reduction workflows, and their applications to data reduction. In Section 4, the current state and future plans are discussed.
2 Methods
We define data reduction as the act of applying selection and transformation techniques to experimental data with the goal of maximizing the density of scientific content. Different quality criteria or filtering of particular event types can potentially be used to distinguish valuable and disposable data. An example of a quality criterion is the X-ray pulse energy being measured above a given threshold, while a possible event type includes the identification of photons scattered by a sample. Similarly, interesting detector regions can be identified, and data outside these regions can be ignored. Possible data transformations include dimensionality reduction, change of representation, compression, and additional data analysis methods. Dimensionality reduction may be achieved by discarding a portion of the parameter space, or by integrating data along certain variables. Common operations include binning, averaging of several data sets, or integration of images along, e.g., the azimuthal angle.
Different experimental techniques leverage various sets of physical observables, which are analysed according to the scientific goal of the experiment. European XFEL offers a wide spectrum of such techniques, hence requiring a flexible choice of the data reduction method. We refer to such data reduction methods as technique-specific. In contrast, operation-specific methods depend on particular experiment modalities, e.g., a specific detector configuration.
Examples of technique-specific data reduction can be found in serial femtosecond crystallography (SFX) [36, 37] and single particle imaging (SPI) [36, 38], where a significant fraction of the collected data does not capture a scattering event. The procedure of identifying whether or not the X-ray beam scattered off the sample is referred to as hit finding, and can be an important trigger for selecting data. Another example of a technique-specific data reduction method is found in small- and wide-angle X-ray scattering experiments [39], where rotation invariant scattering data can be azimuthally integrated and reduced to a one-dimensional curve. Likewise, in X-ray photon correlation spectroscopy (XPCS) [40, 41] and X-ray cross-correlation analysis (XCCA) [42], the 2D detector data may be reduced to intensity-intensity correlation functions.
Technique-specific methods are often more challenging, as they rely on a proper selection and configuration of the analysis pipeline. Facility users are often the most experienced with the latter task, driving the scientific analysis for a given experiment. Thus, we aim at offering full control over the reduction pipeline, alongside detailed metrics to continuously monitor the reduction outcome.
Finally, it is worth mentioning that data reduction can be implemented at different stages of the experiment, that is, during the data acquisition (online) or after data have been stored to disk (offline), implying different requirements and limitations. Decisions can either be automatic and irreversible, or manual and assisted through detailed event-based annotation.
2.1 Overview of the data infrastructure at the European XFEL
The European XFEL’s storage and computing systems [43] are separated into online and offline storage and processing infrastructure (see Figure 2). The online storage is a performant cache capable of ingesting scientific data produced during experiments. In order to be able to effectively steer and control experiments, the online computing cluster is used to process data streams provided during data acquisition, and with minimal latency. After collection, data identified as potentially interesting in the data management portal myMdC [44] is copied from the online storage to a second high-performance layer, implemented using the IBM Elastic Storage System building blocks and presented as a unified IBM Spectrum Scale (a.k.a. GPFS) filesystem [45]. Here, file-based processing is performed using the offline computing cluster Maxwell [46]. This storage system is used during the experiment up to a few months after it. The third layer is mass storage based on the middleware system dCache [47], which extends the capacity of the high-performance system. Both the high-performance and mass storage systems together are often referred to as offline storage. The last layer is the tape archive, which provides resources for long-term data preservation.
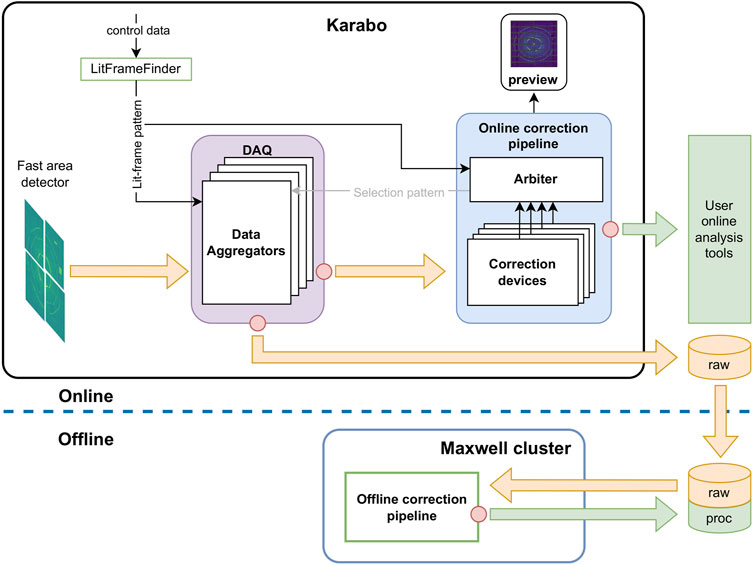
FIGURE 2. Simplified schematic of the European XFEL data acquisition and processing. Cylinders represent data storage elements. Processes above the dashed blue line are part of the online infrastructure. After the raw data is stored, it is copied to the offline Maxwell cluster, at which point the offline correction pipeline transforms raw data into corrected data, both of which are made available as part of the offline infrastructure. The online infrastructure is implemented in the Karabo framework, in which fast detector data is fed to the data acquisition system, that aggregates data from several sources. The online correction pipeline not only corrects data for online inspection: it also contains an arbiter, which decides which data to store, based on the configured data reduction methods. In this schematic, red circles represent data reduction points.
The supervisory control and data acquisition (SCADA) system Karabo [48, 49], which is developed in-house, plays a key role in data ingestion, and experiment and beamline control. Karabo implements an event-driven paradigm, built around a central message broker. Functionalities—either hardware integration or high-level procedures—can be easily added to the core system via plugins called devices. Devices can access any information in the distributed Karabo system, e.g., control parameters or detector readings, which can be readily used also for data reduction purposes.
The data acquisition (DAQ) system is also implemented in Karabo [50, 51]. It aggregates data from any selected device in the distributed Karabo system, including area detectors, and matches the data by event (train) index, before storing it on the disk. This data is termed raw data. In addition, the DAQ outputs and streams the data online for monitoring purposes. Data acquisition and recording are implemented in so-called data aggregators.
On the online cluster, the monitoring data stream from the DAQ is sent to the online correction pipeline [18]. The latter, also implemented in Karabo, transforms raw into usable data with latencies up to a few seconds. The correction pipeline can be extended through computational kernels—say, custom data analysis procedures—implemented as add-ons. For big area detectors, consisting of multiple sensor modules, processed data is aggregated and dispatched for further online processing.
After raw data stored on the online cluster is copied to the offline cluster, the offline correction pipeline produces a corrected copy of this data, which is stored as so-called corrected data. This typically doubles the volume of data collected from area detectors in the context of an experiment. However, the lifetime of processed data can be arbitrarily short, as it can be reproduced from the corresponding raw dataset at any point in time.
2.2 Data reduction points in the data system and associated risks
Data reduction can be applied at different points in the data system (see Figure 2), with different implications. In particular, the earlier and closer the point to the source of the data, the higher the impact on the system.
As previously introduced, the DAQ defines which raw data will be stored or transferred to the correction pipeline. Therefore, any reduction at the DAQ-level is irreversible and can hence only be applied when the associated risk is minimal. Furthermore, at this stage, it is difficult to include complex processing of detector data, due to the strict latency requirements, and the dependencies on the scientific methodology or detailed data analysis, which are difficult to automate. Therefore, decisions are only based on operating conditions that are readily available in the Karabo environment. Any reduction at the DAQ level maximizes the impact on the downstream data system. As of today, this point is used solely to filter detector frames not exposed to X-rays (see Section 3.1.1), but other reduction techniques will be implemented, including module or region of interest selection or gain bit suppression.
The next reduction point is at the output of the online correction pipeline. Here, the data has been modified for the benefit of downstream online analysis tools, which receive filtered or pre-processed and simplified data. Owing to this, data reduction at this point can decrease feedback latency, thereby enhancing response times in experiment steering. Additionally, the load on the network and computing infrastructure decreases. Examples of add-ons implemented within the correction pipeline include a peak-finding algorithm (for SFX), a lit-pixel counter (mainly for SPI) and a per-detector-module estimator of average intensity. These cover typical imaging- and event-based experimental techniques. We foresee that our users will be able to fully exploit this reduction point by contributing additional data processing code in the future. Decision criteria at this stage are potentially much more complex than the ones at the DAQ-level, and might require some degree of parameter tuning either by experts or algorithms as the experiment progresses. The time budget for these optimizations, however, is extremely limited owing to the ephemeral nature of data streams. That is, decisions must be taken before the next data batch. Therefore, there is a certain risk of biasing the downstream analysis due to inaccurate data reduction, with consequences on experiment steering and, thus, experiment outcome. In the future, the filtering applied at this point will also be fed back into the DAQ, which can either annotate or reduce raw data before storage. The latter case is more risky, as data is irreversibly discarded. Furthermore, the tuned configuration parameters will be stored such that they can be later considered as part of offline correction, or retroactively by users.
Further downstream, the next data reduction point is at the end of the offline correction pipeline. In this case, only processed data is affected, while raw data remains unaffected. As the former can be fully reproduced (see Ref. [18]) from the latter, this is a minimal risk data reduction. However, similar to its online counterpart, reduction can bias analysis, thus affecting the quality of the extracted scientific content. At this point, further reduction decisions can be taken that will be applied to the processed data immediately, or can be used to either annotate or reduce stored raw data as well.
Finally, data reduction methods can be applied to offline raw or processed data retroactively by users of the facility or sophisticated algorithms. The reduced data sets can be produced (i) by a tool provided by the European XFEL, (ii) by one of the said tools taking into account decisions derived from user input (e.g., list of hits for SFX or SPI experiments), or (iii) by user tools (perhaps to be integrated into our data system for the benefit of a larger community), provided the data format is compatible with the EuXFEL’s .3
To facilitate the reduction of existing data and ensure its compatibility with the facility’s data format, the exdf-tools package [52] has been developed. This is implemented via small plugins which allow for the usage of several data reduction operations, such as removing a train or pulse for specific sources and keys. All such operations may be collected and applied while rewriting the input data into new files, and serve as a detailed record of how the data was modified.
3 Results
Below, selected examples of data reduction methods implemented at the European XFEL are introduced, and their impact is discussed. First, operation-specific methods are presented. As is evident from Figure 1, to date, AGIPD detectors have produced the majority of data. Therefore, developing methods specific to this detector has been of the highest priority. In the second part of this section, technique-specific methods are discussed.
3.1 Operation-specific methods
Below, we describe the operation-specific methods currently implemented at European XFEL. As mentioned previously, operation-specific methods are technique-independent and related to instrument operation itself. As ideally, no analysis is required to decide on the data, these methods are robust, low risk, and the feedback latency is compatible with online requirements. All methods except for the module selection are fully automated.
3.1.1 Lit frames selection
Fast area detectors collect data frames in batch mode upon triggering at 10 Hz. Within such a batch, called a train, X-ray pulses can be delivered in arbitrary patterns, according to experimental conditions and requirements. For instance, the intra-train repetition rate can be lowered so as to allow the sample delivery system to replenish the interaction region before the next pulse. In other cases, a complex pulse pattern can be used to probe particular sample dynamics. As a result, some detector images might be recorded in absence of X-rays, and therefore are called dark frames. Megahertz imaging detectors at European XFEL were designed to implement veto mechanisms to reuse memory cells and avoid recording dark frames.
However, given the complexity of current detectors, vetoing might potentially affect data quality or complicate operation. This is particularly true for the AGIPD, which requires an individual set of calibration constants for different veto patterns. Covering all possibilities in calibration is infeasible, and thus, the AGIPD is usually operated with a fixed veto pattern, rather than one that acts on the dynamic changes in the X-ray pulse pattern. To mitigate this, we have implemented a Karabo device which aggregates relevant accelerator and AGIPD settings and annotates collected data accordingly. This information can be used to select data at any reduction point. This is a low risk method, and its reduction factor is the ratio of selected and collected frames.
The selection of lit-frames is routinely applied as part of the offline correction pipeline to the corrected data at the MID [53] and SPB/SFX [54] scientific instruments. Furthermore, this method has been applied at the DAQ reduction point, so far for testing proposes. Owing to the application of this method, in 2023 the storage of 0.65 PiB of raw data and 1.7 PiB of processed data has been avoided (see Table 1). In the latter case, the corresponding raw data can also be retroactively reduced.
3.1.2 Gain data suppression or compression
X-ray detectors at the European XFEL use different mechanisms to increase the dynamic range of the detected signal so as to extend their ability to acquire a trustworthy and physically meaningful signal. Among them, the AGIPD uses an adaptive gain method: it stores two 16-bit integers for every pixel, one for the signal amplitude and one encoding the gain stage, which identifies the amplification factor. Under certain illumination conditions, achieved typically during XPCS or SPI experiments, a single gain stage is used. Furthermore, for some experimental techniques, pinning the gain stage is desirable so as to achieve a simpler detector response. In such cases, the gain information could be substituted without risk by a fixed value for the entire data acquisition period.
The reduction factor corresponding to the suppression of the gain data is two. The method is currently available for retroactive reduction. To avoid any modification of the data format, we exploit a HDF5 [55] feature which allows to keep the original raw data dimensions without allocating storage for the gain information. An implementation compatible with the online correction pipeline is also being developed.
In 2023, 1.2 PiB of data has been collected with fixed (medium) gain at the SPB/SFX scientific instrument, and 3.1 PiB with special settings useful to amplify low-intensity signal at the MID instrument. Retroactive suppression of the gain data therefore will allow to release 2.2 PiB of storage (see Table 1).
Complementary to this strategy, we are evaluating the replacement of a 16-bit gain signal with a unique integer representing the gain stage. Ideally such a step would not represent a loss of data. However, the impact of noise in the original gain signal may lead to a data quality loss, especially when close to a gain transition. Therefore we are currently evaluating that impact and establishing contingencies. For AGIPD, the original 16-bit values are converted into three possible values. Accordingly, the expected benefit from a lossless compression of the gain information, even when using a standard algorithm such as Deflate [56], is sizeable.
3.1.3 Train selection
In some cases, it may be meaningful to select a subset of the trains to record. This is particularly relevant to the HED scientific instrument [57], where a shutter wheel is used to mechanically filter X-rays and illuminate the sample only with a specific pulse train. An incorrect selection poses a large risk of losing the relevant data. Thus, the DAQ stores several adjacent trains in addition to the selected one. This allows for validation of the train selection reduction method, and minimizes the risk of data loss in case of an incorrect setting. The information on selected trains is available in Karabo, which controls the wheel, and can be readily exploited by the offline correction pipeline upon validation, or retroactively. In the future, we aim to incorporate this reduction method at the DAQ level.
The reduction factor equals the ratio of DAQ-acquired and selected trains, and, depending on the DAQ settings and the applied procedure, it can be of the order of hundreds. In 2023, 0.6 PiB of data, including disposable trains, has been collected at the HED scientific instrument. The train selection method has been applied to these data at the offline correction pipeline stage, reducing it by a factor of 19 (see Table 1). We plan to retrospectively reduce the corresponding raw data as well.
3.1.4 Module and region-of-interest selection
For certain experiments, the relevant signal is confined to a well-defined region-of-interest (ROI) on the detector. Most European XFEL X-ray imaging detectors are modular, and therefore, only a few modules may intersect with the ROI. For technical reasons, the data from each module is saved in a different file. Hence, it is straightforward to select only files corresponding to the relevant modules to obtain a significant reduction of offline data.
Bragg coherent diffraction imaging (BCDI) is one of the experimental techniques that can benefit from this reduction method. Since 2022, five BCDI experiments were performed at the MID scientific instrument, and for half of them only one detector module (out of sixteen) contained data of interest. By retroactively removing data corresponding to the other modules, the initial volume can be reduced from 2.3 PiB to 0.46 PiB, that is a reduction factor of five (see Table 1).
At the time of writing, this method can be employed by manually selecting relevant modules using the DAQ interface. In the near future, a graphical user interface will be available to configure the DAQ and perform validation by monitoring the signal on the entire detector.
The reduction factor equals the ratio of the total number of detector modules to the number of selected modules. In the future, the possibility of storing defined regions of interest within modules will also be exploited.
3.2 Technique-specific methods
Technique-specific methods require processing of collected data, which typically involves fine tuning of certain analysis parameters so as to ensure accurate results. As such, associated risks of discarding meaningful data are generally higher compared to operation-specific methods, and present challenges for automation. Furthermore, appropriate pre-processing of the data—e.g., handling of detector artifacts such as pixels with erroneous readings (perhaps damaged), or accurately mapping data according to the physical detector layout—as well as extensive validation are required. For several experimental techniques, the scientific community has developed specialized methods and software tools, which can provide feedback on data content and quality. These may need to be integrated into the European XFEL computing and control environments to fully leverage data reduction opportunities through automation and implementation of fast feedback loops.
3.2.1 Effective compression by decreasing entropy: conversion to integer photon counts and down-sampling of collected intensities
The effectiveness of compression methods increases with a lower Shannon entropy of collected data [58]. To reduce entropy, we have evaluated the application of physics-motivated techniques to compress detected intensities. The risk associated to these is particularly low if analysis techniques rely on a sizable ensemble of individual measurements.
In some cases, it may be advantageous to convert measured intensities into the absolute number of photons and represent them as integers. This applies to experiments where the scattering signal is rather weak, such as SPI and XPCS, or the strong signal is localized and the background is sparse and weak, such as BCDI. Through this quantization procedure the entropy may be significantly reduced and detector images become extremely compressible with lossless methods. We use the Deflate algorithm as implemented in the HDF5 library, making access to data transparent to users.
In particular, at the MID scientific instrument the combination of selection of lit-frames, conversion to integer photon counts and compression is routinely applied at the offline correction reduction point. Owing to this, 5.7 PiB of processed data were not stored in 2023, corresponding to an overall reduction factor of approximately 42 (see Table 1). Figure 3 illustrates the effect of applying the above-mentioned reduction chain to a Bragg XPCS experiment. Here the overall reduction factor was 97. In the figure, the reduced size of AGIPD corrected data is shown as a function of its original size. Each point refers to the data set of an individual measurement, a so-called run. Points are colored depending on the number of X-ray pulses utilized for the measurment, and each dashed gray line, shown to guide the eye, is an isoline indicating the same reduction factor.
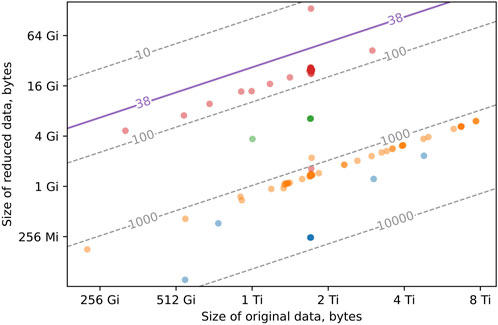
FIGURE 3. Reduced size of AGIPD corrected data versus its original size. Lit-frame selection, conversion to integer photon counts and compression are applied during offline correction. Each dot corresponds to one data set. Its color identifies a different number of X-ray pulses utilized: blue – 1 pulse, orange – 10 pulses, green – 50 pulses, red – 200 pulses. The dashed gray isolines refer to different reduction factors and are shown to guide the eye. The purple line corresponds to the average effect of conversion to integer photon counts followed by compression.
The position of each point is given by the combined effect of selecting lit-frames, converting intensities to an integer number of photons and, finally, compressing this data. The average reduction factor due to conversion to integer photon counts followed by compression, shown as the purple line, corresponds to 38. This value depends on illumination conditions, which, in this experiment, are similar for most data sets. Additionally, the lit-frames selection contributes, with a reduction factor equal to the ratio between total number of collected frames to X-ray pulses, varying between 1.76 and 352.
For experiment techniques exploiting a large intensity range, such as SFX, rounding to a given number of significant bits reduces the distribution of pixel values [24], and Shannon entropy accordingly. This also makes images more compressible with lossless methods. The quality of the final result depends on the rounding settings, which have to be balanced with respect to the potential for desired reduction.
This method is typically reliable upon validation, which can be based, for example, on comparing subsets of results, and its reliability increases with the number of repetitions of a certain measurement. The risk of using such methods is mitigated by applying them only to the corrected data.
Both methods discussed are available for offline usage. Conversion to integer photon counts and subsequent compression is integrated into the offline correction pipeline and used in operations.
3.2.2 Azimuthal integration of rotation invariant data: small- and wide-angle scattering
The first processing step of rotation invariant data is typically the azimuthal integration of detector frames. The transformation of two-dimensional images into one-dimensional radial profiles is an operation which scales with the square-root of the number of pixels, and thus yields a reduction factor of about 1,000 for megapixel images.
Proof-of-principle automatic azimuthal integration after detector data correction has already been employed at European XFEL. The automated pipeline processes frames in batch mode and can exploit the pyFAI library [59]. We are further improving it by enabling parallel data reads from disk, and signal integration in parallel on GPUs. The pipeline output can replace processed data containing corresponding two-dimensional images. Furthermore, we are developing an azimuthal integrator add-on for the online correction pipeline.
Current research in validation includes a reliable and automatic correction for potential displacements of the X-ray beam during data collection. In fact, several experimental techniques require a precise estimation of the X-ray axis. Its erroneous assessment degrades the quality of azimuthally integrated data, for example, as the integration axis does not coincide with the X-ray axis, which is a symmetry one.
3.2.3 Hit finding: serial femtosecond crystallography and single particle imaging
For experimental techniques like SFX and SPI, the X-ray beam interacts with the sample with a certain probability, known as the hit rate. In fact, “hits” and “non-hits” are defined as detector frames either containing signal scattered from the sample, or only background photons. The number of hits compared to the total amount of delivered pulses, the hit rate, is typically rather modest, of the order of 0.1%–10%, depending on the sample and the injection method. As a result, a considerable amount of detector images have to be acquired during the experiment for successful data analysis, and the potential for data reduction by discarding all non-hits is significant.
The first step of the SFX data analysis pipeline consists of the identification of Bragg peaks in a detector frame. If the number of peaks exceeds a user-defined threshold, that frame is considered to be a hit. If the next step of the analysis pipeline, indexing, is considered as well, the reduction factor can be potentially increased further at the cost of higher complexity. Different software tools provided by the scientific community implement such complete analysis pipelines [60, 61] (a description can be found, e.g., in [62] and references therein). Among these, we have integrated the CrystFEL suite [60] into the European XFEL infrastructure. We provide the latter through the EXtra-Xwiz tool [62, 63], so as to abstract certain complications specific to our data structure and computing environment.
When processing SPI data, the number of pixels on a detector frame characterized by a signal intensity above a certain threshold is initially evaluated. Hits satisfy the condition that the number of such lit pixels exceeds another threshold. Also in this case, data analysis tools provided by scientific communities exist [64].
Strategies for validation of the hit finding output include the (graphical) provision of key indicators. These can be statistical views – such as mean, variance, or detected outliers – of retained and discarded data, or more sophisticated feedback calculated at different stages of the data analysis pipelines. For example, a pseudo-powder diffraction pattern can be calculated from SFX data as the sum of extracted Bragg peaks, and relates to the crystalline structure of the sample. Similarly, cell parameters or quality metrics can be extracted at the indexing step.
At the time of writing, hit finders for SFX and SPI experiments are implemented as add-ons in the online correction pipeline, with reduction decisions taken at the arbiter (cfr. Figure 2). These have been tested in production, and satisfy the stringent latency requirements of online analysis. Furthermore, implementations for the offline correction pipeline are in progress, and information on hits produced by diverse tools can be used to retroactively reduce data. Certain SPI experiments have been already identified for retroactive data reduction, corresponding to a raw data volume of 5.5 PiB and with hit rates ranging between 0.1% and 13.5% (see Table 1). Therefore, in this case the average reduction factor is roughly 19 or lower, depending on the need for non-hits which can be used for background estimation. Corrected data can be reduced with the same ratio or better, if conversion to integer photon counts and compression are further applied.
While in this section we present examples of binary classification, concepts introduced here can be extended to more complicated use cases and generalized as data clustering.
3.2.4 Physics reconstruction: reaction microscopy
Reaction microscopy (REMI) [65], also called cold target recoil ion momentum spectroscopy (COLTRIMS), is a momentum imaging technique employed at the SQS scientific instrument [66]. Up to two delay line detectors placed on opposite sides at the end of time-of-flight spectrometers are used to record the kinetic energy and momentum of electrons and ions in coincidence, potentially allowing for a full reconstruction of the scattering process in the molecular frame of reference. On the detector side, the raw data consists of digitized voltage levels acquired at gigahertz sample rates across multiple channels reaching rates up to 4 Gbit/s. From a scientific perspective, only the correlated pairs of position and time for each particle impact are relevant. These have a much lower bandwidth on the order of a few Mbit/s.
An automated reconstruction process from raw data to detector hits is available via the facility offline correction pipeline and allows for efficient data reduction by a factor of approximately 1,000. Validation is provided in the form of reports, which contain statistics and document signal correlations to allow for rapid assessments of reconstruction quality.
3.2.5 Correlation functions: X-ray photon correlation spectroscopy
X-ray photon correlation spectroscopy (XPCS) [40, 41] is a technique used to measure the dynamics of a sample on various time scales. Most XPCS experiments at European XFEL measure dynamics on a timescale of microseconds. Central to the data analysis for XPCS is the calculation of two-time correlation functions (TTCFs). For microsecond XPCS, calculating the TTCF’s requires correlating regions of the detector across pulses within a single train.
A library to streamline and optimize the analysis of XPCS data [67] is being developed at the European XFEL. This approach reduces the data that users need to deal with from (on average) 1 TB of detector data to approximately 1 GB of TTCF data, that is a factor of 1,000.
4 Discussion
Although the data reduction activities at the European XFEL are still at their infancy, several methods and strategies have been identified and integrated as part of the data acquisition and analysis systems. Consequently, an initial portfolio of tools has been made available to users of the facility. By routinely applying low-risk methods to processed data, we have already avoided the storage of approximately 7 PiB of data in 2023, thereby reducing the expected volume of processed data to about 70%. Furthermore, the same tools can be applied retroactively with minimal risk, potentially making approximately 17 PiB of additional storage available. An overview of applying the discussed reduction methods to selected AGIPD data is shown in Table 1.
Risks have been assessed for each considered method to ensure minimal impact on the scientific activities, as reiterated throughout this paper (see in particular, Section 2.2). Data reduction is intrinsically associated to the risk of compromising scientific throughput, as a consequence of discarding valuable and non-redundant information, or of applying inaccurate transformations, for example, due to unreliable parameters. Additionally, an incorrect application of data reduction methods to the online data stream would lead to degraded online analysis feedback. Consequently, the experiment steering quality and the beamtime efficiency are compromised. At the other end of the spectrum, if raw data are erroneously reduced, scientific content is potentially irremediably destroyed.
To mitigate risks, and in addition to extensive user support, we aim to provide our users with information which is as complete and reliable as possible. This includes the production of extensive quality and validation metrics, transparent and comprehensive documentation of the reduction workflow (including any parameter involved), powerful interfaces, as well as various statistics on data usage, which will support user decisions. To be effective, validation metrics must be interpretable, and offer feedback on the effect of any parameter involved. Validation is particularly critical when technique-specific methods are used, as they rely on data assessment and might require tuning. At the time of writing, we offer an initial set of metrics for certain reduction techniques, both in the form of online feedback, and offline reports. These include, for example, time-averaged online views of retained and discarded data, or monitoring of the signal on the entire detector when only a region of interest is selected.
Additionally, we systematically organize workshops, in which presentations and tutorials are shown to aid users even before they access the facility. Invaluable user feedback is also obtained at such meetings, and helps us adapt to the user needs and address their concerns. This integrates with the extensive documentation and training material that will be made available.
A complementary strategy to reduce risks relies on the development of sophisticated algorithms to decide on reduction methods. Such algorithms work with a clear optimization strategy and with several interpretable metrics embedded in them, to allow for monitoring and control. Although this leads to a more abstract decision process, the availability of meaningful validation systems empowers users to disengage such methods or reconfigure them as required. An example of such an automated process under development, is an application based on mathematical modeling of the parameter optimization procedure for SFX data analysis [68]. This method empowers users by providing high-quality information on collected data, aiding them in steering the experiment, and preventing accordingly the acquisition of low-quality data. Another procedure under development includes the clustering of data as it arrives in the data stream, which allows users to rapidly assess similarities in the data collected, discover patterns and establish low-quality data.
In addition to the technical and scientific aspects of data reduction, another essential enabling step is a corresponding legal framework, which establishes a contract between all parties and defines their responsibilities in this process. The scientific data policy of the European XFEL has undergone a major upgrade to provision this. The upgrade process involved all the stakeholders inside the facility, including groups responsible for data management and analysis, legal specialists, instrument scientists, as well as external advisory committees, and users of the European XFEL. The latter have either been approached individually, or through dedicated events in the context of European XFEL user meetings.
This inclusive process has contributed to assess and address the sociological aspect intrinsic in the paradigm of data reduction. This is overall a new paradigm in photon science, and as such concerns might originate both from (i) the risk of scientific data loss, and (ii) the burden associated to selection of viable data reduction methods, the decision process itself, as well as further analysis downstream of the reduced data sets. Mitigation strategies for the former have already been discussed: we are convinced that, in addition to involving users early on in the process, these strategies will increase users’ confidence when applying reduction methods. For the latter, we aim at providing simple interfaces to aid in the decision process, as well as software allowing users to transparently access any kind of data produced at the facility. Furthermore, to support and advise on reduction opportunities as well as to train on available methods and tools we provide, we will take advantage of internal experts as contacts. Overall, users will be assisted in data reduction activities with the provision of tools, information and expertise.
Another measure to increase user involvement in the data reduction process has been the establishment of a data management plan. Such a data management plan, required by the updated scientific data policy3 for each user proposal, would contain a detailed overview of the reduction solutions applicable to the data collected, formalize requirements and document decisions. This procedure establishes a clear bidirectional communication pathway between the users and the facility from proposal acceptance onward, with the aim of increasing users’ trust in the data reduction processes.
To summarize, our aim is to empower users to extract valuable scientific content from collected data. Data reduction is in the users’ benefit: for instance, it allows them to achieve a faster turnaround when analysing the experiment’s result and to simplify their analysis methodology. Importantly, users shall be responsible for selecting methods and reduction points, balancing risks and benefits, or the retroactive reduction of collected data, such that within a defined amount of time (six months at the time of writing) the size of their data is within the constraints defined by the facility (up-to-date information is available in Ref. [69]).
5 Conclusion
Reducing collected data to its scientific content brings significant advantages to users, the environment, and facilities. For users, the scientific outcome of the experiment is potentially improved due to better decision making, as well as simpler and more effective data analysis. The environment benefits from a decreased energy footprint in processing and storing the data, and in turn facilities profit from the reduced initial investment and operation costs, therefore improving operational sustainability.
To support this effort, we have developed an initial portfolio of data reduction methods. A few of these have been deployed and are already routinely used in operation at the European XFEL. We show that, by applying these, we avoided storage of about one third of the expected volume of processed data in 2023. Additionally, we have started developing technique-specific methods, some of which have been already employed for online data analysis and reduction. In parallel, the data system has been upgraded to include reduction points, and technique-specific data reduction methods have been investigated. To further develop and validate effective solutions for the latter, the considerable domain knowledge of our users is required.
Data reduction activities are a clear priority of European XFEL. Their development involves a multitude of actors, inside and outside the facility, which exemplifies the need for the diverse expertise intrinsic to data reduction.
Our strategy to maximize the impact of reduction activities is founded on increasing this synergy between facility experts and users. The deep understanding of infrastructure, software practices, detection systems, and methodologies that facility staff can provide needs to be paired with the knowledge of the scientific domain users bring in. Facility-side we aim at offering information, interpretable metrics, efficient interfaces and expertise that support our users in making effective decisions on the data reduction strategy for their experiments.
To conclude, in this paper we report on our vision for data reduction at the European XFEL, as well as selected preliminary results. We are convinced that the collaboration and co-design of reduction tools with our users will simultaneously ensure excellent scientific results and a sustainable operation. On this note, our early experience with users that volunteered to apply reduction methods at the European XFEL resulted in critical feedback that is contributing to shape ideas and develop tools. The systematic implementation of streamlined data reduction methods as part of the data acquisition, analysis and storage can result in a paradigm shift in photon science concerning data handling and processing.
Data availability statement
The original contributions presented in the study are included in the article, further inquiries can be directed to the corresponding author.
Author contributions
ES: Conceptualization, Formal Analysis, Investigation, Methodology, Software, Project administration, Validation, Visualization, Writing–original draft, Writing–review and editing. PS: Conceptualization, Formal Analysis, Investigation, Methodology, Software, Validation, Visualization, Writing–original draft, Writing–review and editing. JaM: Formal Analysis, Investigation, Methodology, Writing–review and editing. DH: Methodology, Software, Writing–review and editing. DB: Methodology, Software, Writing–review and editing. JoM: Conceptualization, Formal Analysis, Investigation, Validation, Writing–review and editing. KA: Software, Writing–review and editing. RB: Resources, Writing–review and editing. IB: Validation, Writing–review and editing. JB: Validation, Writing–review and editing. UB: Validation, Writing–review and editing. CC: Software, Writing–review and editing. FD’A: Validation, Writing–review and editing. SE: Software, Writing–review and editing. HFa: Conceptualization, Funding acquisition, Project administration, Supervision, Visualization, Writing–review and editing. DFdL: Conceptualization, Visualization, Writing–original draft, Writing–review and editing. LM: Software, Writing–review and editing. HFi: Investigation, Writing–review and editing. GF: Software, Writing–review and editing. PG: Resources, Writing–review and editing. GG: Software, Writing–review and editing. JK: Validation, Writing–review and editing. AM: Resources, Writing–review and editing. TM: Software, Writing–review and editing. MiS: Validation, Writing–review and editing. MaS: Validation, Writing–review and editing. AS: Software, Writing–review and editing. JS–D: Validation, Writing–review and editing. MT: Validation, Writing–review and editing. OT: Formal Analysis, Investigation, Software, Writing–original draft, Writing–review and editing. JW: Formal Analysis, Investigation, Software, Writing–original draft, Writing–review and editing. SA: Conceptualization, Funding acquisition, Supervision, Writing–review and editing. SH: Conceptualization, Software, Writing–original draft, Writing–review and editing. KW: Conceptualization, Project administration, Supervision, Writing–original draft, Writing–review and editing. LG: Conceptualization, Funding acquisition, Project administration, Supervision, Visualization, Writing–original draft, Writing–review and editing.
Funding
The author(s) declare financial support was received for the research, authorship, and/or publication of this article. Data reduction activities at the European XFEL have been partially funded through the European Union’s Horizon 2020 research and innovation programme under grant agreement no. 101004728.
Acknowledgments
The authors truly acknowledge the users of the European XFEL which are bravely assisting us in validating and pushing forward data reduction activities. We acknowledge the participants in the Horizon 2020 LEAPS-INNOV WP7, for the constructive discussions over the last few years. We acknowledge countless colleagues at the European XFEL and DESY that have contributed in different ways to this project, and in particular the participants of the working group on scientific data policy and data reduction. We particularly acknowledge the members of the European XFEL Detector Advisory Committee for the continuous support in this activity, and the members of the Data Analysis group at the European XFEL for the endless assistance and feedback. We are indebted to Thomas Tschentscher for the steady encouragement, insightful discussions and critical reading of this manuscript.
Conflict of interest
The authors declare that the research was conducted in the absence of any commercial or financial relationships that could be construed as a potential conflict of interest.
Publisher’s note
All claims expressed in this article are solely those of the authors and do not necessarily represent those of their affiliated organizations, or those of the publisher, the editors and the reviewers. Any product that may be evaluated in this article, or claim that may be made by its manufacturer, is not guaranteed or endorsed by the publisher.
Footnotes
1Scientific Data Policy of the European X-Ray Free-Electron Laser Facility GmbH (Version 1). European XFEL, Schenefeld, Germany (2017). doi:10.22003/XFEL.EU-TR-2017-003.
2Scientific Data Policy of the European X-Ray Free-Electron Laser Facility GmbH (Version 2). European XFEL, Schenefeld, Germany (2025). doi:10.22003/XFEL.EU-TR-2025-001.
References
1. Wang C, Steiner U, Sepe A. Synchrotron big data science. Small (2018) 14:1802291. doi:10.1002/smll.201802291
2. Götz A, le Gall E, Konrad U, Kourousias G, Knodel O, Matalgah S, et al. LEAPS data strategy. The Eur Phys J Plus (2023) 138:617. doi:10.1140/epjp/s13360-023-04189-6
3. Hatsui T, Graafsma H. X-ray imaging detectors for synchrotron and XFEL sources. IUCrJ (2015) 2:371–83. doi:10.1107/S205225251500010X
4. Chapman HN. Fourth-generation light sources. IUCrJ (2023) 10:246–7. doi:10.1107/S2052252523003585
5. Pellegrini C. The history of X-ray free-electron lasers. The Eur Phys J H (2012) 37:659–708. doi:10.1140/epjh/e2012-20064-5
6. Chapman HN. X-ray free-electron lasers for the structure and dynamics of macromolecules. Annu Rev Biochem (2019) 88:35–58. PMID: 30601681. doi:10.1146/annurev-biochem-013118-110744
7. Blaj G, Caragiulo P, Carini G, Dragone A, Haller G, Hart P, et al. Future of ePix detectors for high repetition rate FELs. AIP Conf Proc (2016) 1741:040012. doi:10.1063/1.4952884
8. Hart M, Angelsen C, Burge S, Coughlan J, Halsall R, Koch A, et al. Development of the LPD, a high dynamic range pixel detector for the European XFEL. In: 2012 IEEE nuclear science symposium and medical imaging conference record (NSS/MIC) (2012). p. 534–7. doi:10.1109/NSSMIC.2012.6551165
9. Veale M, Adkin P, Booker P, Coughlan J, French M, Hart M, et al. Characterisation of the high dynamic range Large Pixel Detector (LPD) and its use at X-ray free electron laser sources. J Instrumentation (2017) 12:P12003. doi:10.1088/1748-0221/12/12/P12003
10. Allahgholi A, Becker J, Bianco L, Bradford R, Delfs A, Dinapoli R, et al. The adaptive gain integrating pixel detector. J Instrumentation (2016) 11:C02066. doi:10.1088/1748-0221/11/02/C02066
11. Allahgholi A, Becker J, Delfs A, Dinapoli R, Goettlicher P, Greiffenberg D, et al. The adaptive gain integrating pixel detector at the European XFEL. J Synchrotron Radiat (2019) 26:74–82. doi:10.1107/S1600577518016077
12. Porro M, Andricek L, Aschauer S, Castoldi A, Donato M, Engelke J, et al. The MiniSDD-Based 1-Mpixel Camera of the DSSC Project for the European XFEL. IEEE Trans Nucl Sci (2021) 68:1334–50. doi:10.1109/TNS.2021.3076602
13. Johnson I, Bergamaschi A, Billich H, Cartier S, Dinapoli R, Greiffenberg D, et al. Eiger: a single-photon counting x-ray detector. J Instrumentation (2014) 9:C05032. doi:10.1088/1748-0221/9/05/C05032
14. Hatsui T. CITIUS: a 17400 frames/s x-ray imaging detector. Tenth Intl. Workshop on Pixel Detectors for Particles and Imaging (2022). https://indico.cern.ch/event/829863/contributions/4479490/ (Accessed January 23, 2024).
15. Decking W, Abeghyan S, Abramian P, Abramsky A, Aguirre A, Albrecht C, et al. A MHz-repetition-rate hard X-ray free-electron laser driven by a superconducting linear accelerator. Nat Photon (2020) 14:391–7. doi:10.1038/s41566-020-0607-z
16. Tschentscher T. Investigating ultrafast structural dynamics using high repetition rate x-ray FEL radiation at European XFEL. Eur Phys J Plus (2023) 138:274. doi:10.1140/epjp/s13360-023-03809-5
17. Sekutowicz J, Ayvazyan V, Barlak M, Branlard J, Cichalewski W, Grabowski W, et al. Research and development towards duty factor upgrade of the European X-Ray Free Electron Laser linac. Phys Rev ST Accel Beams (2015) 18:050701. doi:10.1103/PhysRevSTAB.18.050701
18. Schmidt P, Ahmed K, Danilevski C, Hammer D, Rosca R, Kluyver T, et al. Turning European XFEL raw data into user data. Front Phys (2024) 11. doi:10.3389/fphy.2023.1321524
20. Jenni P, Nessi M, Nordberg M, Smith K. ATLAS high-level trigger, data-acquisition and controls: technical Design Report. in Tech. rep. Geneva: CERN (2003).
21. Bayatyan GL, Grigorian N, Khachatrian VG, Margarian AT, Sirunyan AM, Stepanian S, et al. CMS TriDAS project: technical design report. In: The trigger systems. Tech. Rep., 1. CERN (2000).
22. Thayer JB, Carini G, Kroeger W, O’Grady C, Perazzo A, Shankar M, et al. Building a data system for LCLS-II. In: 2017 IEEE nuclear science symposium and medical imaging conference (NSS/MIC) (2017). p. 1–4. doi:10.1109/NSSMIC.2017.8533033
23. Hadian-Jazi M, Sadri A, Barty A, Yefanov O, Galchenkova M, Oberthuer D, et al. Data reduction for serial crystallography using a robust peak finder. J Appl Crystallogr (2021) 54:1360–78. doi:10.1107/S1600576721007317
24. Galchenkova M, Tolstikova A, Yefanov O, Chapman H. Data reduction in protein crystallography. Acta Crystallogr Section A (2022) 78:e266. doi:10.1107/S2053273322094517
25. Nawaz S, Rahmani V, Pennicard D, Setty SPR, Klaudel B, Graafsma H. Explainable machine learning for diffraction patterns. J Appl Crystallogr (2023) 56:1494–504. doi:10.1107/S1600576723007446
26. Rahmani V, Nawaz S, Pennicard D, Setty SPR, Graafsma H. Data reduction for X-ray serial crystallography using machine learning. J Appl Crystallogr (2023) 56:200–13. doi:10.1107/S1600576722011748
27. Kieffer J, Petitdemange S, Vincent T. Real-time diffraction computed tomography data reduction. J Synchrotron Radiat (2018) 25:612–7. doi:10.1107/S1600577518000607
28. Kieffer J, Brennich M, Florial JB, Oscarsson M, De Maria Antolinos A, Tully M, et al. New data analysis for BioSAXS at the ESRF. J Synchrotron Radiat (2022) 29:1318–28. doi:10.1107/S1600577522007238
29. Kieffer J, Coquelle N, Santoni G, Basu S, Debionne S, Homs A, et al. Real-time pre-processing of serial crystallography. Acta Crystallogr Section A (2022) 78:e263. doi:10.1107/S2053273322094530
30. Zhang Q, Dufresne EM, Nakaye Y, Jemian PR, Sakumura T, Sakuma Y, et al. 20μs-resolved high-throughput X-ray photon correlation spectroscopy on a 500k pixel detector enabled by data-management workflow. J Synchrotron Radiat (2021) 28:259–65. doi:10.1107/S1600577520014319
31. Zhao K, Di S, Lian X, Li S, Tao D, Bessac J, et al. SDRbench: scientific data reduction benchmark for lossy compressors. In: 2020 IEEE international conference on big data (big data). Los Alamitos, CA, USA: IEEE Computer Society (2020). p. 2716–24. doi:10.1109/BigData50022.2020.9378449
32. Wang C, Florin E, Chang HY, Thayer J, Yoon CH. SpeckleNN: a unified embedding for real-time speckle pattern classification in X-ray single-particle imaging with limited labeled examples. IUCrJ (2023) 10:568–78. doi:10.1107/S2052252523006115
33. Sun Y, Brockhauser S, Hegedűs P, Plückthun C, Gelisio L, Ferreira de Lima DE. Application of self-supervised approaches to the classification of X-ray diffraction spectra during phase transitions. Scientific Rep (2023) 13:9370. doi:10.1038/s41598-023-36456-y
34. Wilkinson MD, Dumontier M, Aalbersberg IJ, Appleton G, Axton M, Baak A, et al. The FAIR Guiding Principles for scientific data management and stewardship. Scientific Data (2016) 3:160018. doi:10.1038/sdata.2016.18
35. European XFEL. User documentation for data reduction at European XFEL (2024). Available at: https://rtd.xfel.eu/docs/data-reduction-user-documentation/en/latest/ (Accessed January 23, 2024).
36. Neutze R, Wouts R, van der Spoel D, Weckert E, Hajdu J. Potential for biomolecular imaging with femtosecond X-ray pulses. Nature (2000) 406:752–7. doi:10.1038/35021099
37. Chapman HN, Fromme P, Barty A, White TA, Kirian RA, Aquila A, et al. Femtosecond X-ray protein nanocrystallography. Nature (2011) 470:73–7. doi:10.1038/nature09750
38. Bogan MJ, Benner WH, Boutet S, Rohner U, Frank M, Barty A, et al. Single particle X-ray diffractive imaging. Nano Lett (2008) 8:310–6. doi:10.1021/nl072728k
39. Graewert MA, Svergun DI. Impact and progress in small and wide angle X-ray scattering (SAXS and WAXS). Curr Opin Struct Biol (2013) 23:748–54. doi:10.1016/j.sbi.2013.06.007
40. Lehmkühler F, Dallari F, Jain A, Sikorski M, Möller J, Frenzel L, et al. Emergence of anomalous dynamics in soft matter probed at the European XFEL. Proc Natl Acad Sci (2020) 117:24110–6. doi:10.1073/pnas.2003337117
41. Reiser M, Girelli A, Ragulskaya A, Das S, Berkowicz S, Bin M, et al. Resolving molecular diffusion and aggregation of antibody proteins with megahertz X-ray free-electron laser pulses. Nat Commun (2022) 13:5528. doi:10.1038/s41467-022-33154-7
42. Altarelli M, Kurta RP, Vartanyants IA. X-ray cross-correlation analysis and local symmetries of disordered systems: general theory. Phys Rev B (2010) 82:104207. doi:10.1103/PhysRevB.82.104207
43. Malka J, Aplin S, Boukhelef D, Dietrich S, Filippakopoulos K, Gasthuber M, et al. Data management infrastructure for European XFEL. In: Proceedings of ICALEPCS2023; Geneva, Switzerland: JACoW Publishing (2024). p. 952–957. doi:10.18429/JACoW-ICALEPCS2023-WE1BCO02
44. European XFEL. Metadata catalogue (2023). Available at: https://in.xfel.eu/metadata (Accessed January 23, 2024).
45. Schmuck F, Haskin R. GPFS: a shared-disk file system for large computing clusters. In: Proceedings of the 1st USENIX conference on file and storage technologies (USA: USENIX association) (2002). FAST ’02, 19–es.
46. Deutsches Elektronen-Synchrotron. Maxwell cluster (2023). https://confluence.desy.de/display/MXW/Maxwell+Cluster (Accessed January 23, 2024).
47. Ernst M, Fuhrmann P, Gasthuber M, Mkrtchyan T, Waldman C. dCache, a distributed storage data caching system. In: Proceedings of computing in high energy physics. Beijing (China): Science Press (2001). Available from China Nuclear Information Centre.
48. Hauf S, Heisen B, Aplin S, Beg M, Bergemann M, Bondar V, et al. The Karabo distributed control system. J Synchrotron Radiat (2019) 26:1448–61. doi:10.1107/S1600577519006696
49. Göries D, Ehsan W, Flucke G, Annakkappala N, Bondar V, Costa R, et al. The Karabo SCADA system at the European XFEL. Synchrotron Radiation News (2023). 36, 40–46. doi:10.1080/08940886.2023.2277650
50. Esenov S, Wrona K, Youngman C. Technical design report: European XFEL DAQ and DM computing – 2009 public version. Schenefeld, Germany: Tech. Rep. XFEL.EU TR-2009-001, European XFEL (2009). doi:10.3204/XFEL.EU/TR-2009-001
51. Boukhelef D, Szuba J, Wrona K, Youngman C. Software development for high speed data recording and processing. In: Proceedings of ICALEPCS2013; Geneva, Switzerland: JACoW Publishing (2014). p. 665–668.
52. European XFEL. EXDF-tools: tools to work with EXDF HDF5 files (2023). https://git.xfel.eu/dataAnalysis/exdf-tools (Accessed January 23, 2024).
53. Madsen A, Hallmann J, Ansaldi G, Roth T, Lu W, Kim C, et al. Materials Imaging and Dynamics (MID) instrument at the European X-ray Free-Electron Laser Facility. J Synchrotron Radiat (2021) 28:637–49. doi:10.1107/S1600577521001302
54. Mancuso AP, Aquila A, Batchelor L, Bean RJ, Bielecki J, Borchers G, et al. The Single Particles, Clusters and Biomolecules and Serial Femtosecond Crystallograph instrument of the European XFEL: initial installation. J Synchrotron Radiat (2019) 26:660–76. doi:10.1107/S1600577519003308
56. Deutsch LP. DEFLATE compressed data format specification version 1.3 (1996). doi:10.17487/RFC1951
57. Zastrau U, Appel K, Baehtz C, Baehr O, Batchelor L, Berghäuser A, et al. The High Energy Density Scientific Instrument at the European XFEL. J Synchrotron Radiat (2021) 28:1393–416. doi:10.1107/S1600577521007335
58. Shannon CE. A mathematical theory of communication. Bell Syst Tech J (1948) 27:379–423. doi:10.1002/j.1538-7305.1948.tb01338.x
59. Kieffer J, Valls V, Blanc N, Hennig C. New tools for calibrating diffraction setups. J Synchrotron Radiat (2020) 27:558–66. doi:10.1107/S1600577520000776
60. White T, Kirian R, Martin A, Aquila A, Nass K, Barty A, et al. CrystFEL: a software suite for snapshot serial crystallography. J Appl Cryst (2012) 45:335–41. doi:10.1107/S0021889812002312
61. Brewster AS, Waterman DG, Parkhurst JM, Gildea RJ, Young ID, O’Riordan LJ, et al. Improving signal strength in serial crystallography with DIALS geometry refinement. Acta Crystallogr Section D (2018) 74:877–94. doi:10.1107/S2059798318009191
62. Turkot O, Dall’Antonia F, Bean RJ, E J, Fangohr H, Ferreira de Lima DE, et al. Extra-xwiz: a tool to streamline serial femtosecond crystallography workflows at European XFEL. Crystals (2023) 13:1533. doi:10.3390/cryst13111533
63. Turkot O, Dall’Antonia F, Bean RJ, E J, Fangohr H, Ferreira de Lima DE, et al. Towards automated analysis of serial crystallography data at the European XFEL. In: T Tschentscher, L Patthey, K Tiedtke, and M Zangrando, editors. X-ray free-electron lasers: advances in source development and instrumentation VI, 12581. Bellingham, WA: International Society for Optics and Photonics (2023). p. 125810M. doi:10.1117/12.2669569
64. Barty A, Kirian RA, Maia FRNC, Hantke M, Yoon CH, White TA, et al. Cheetah: software for high-throughput reduction and analysis of serial femtosecond X-ray diffraction data. J Appl Crystallogr (2014) 47:1118–31. doi:10.1107/S1600576714007626
65. Ullrich J, Moshammer R, Dorn A, Dörner R, Schmidt LPH, Schmidt-Böcking H. Recoil-ion and electron momentum spectroscopy: reaction-microscopes. Rep Prog Phys (2003) 66:1463–545. doi:10.1088/0034-4885/66/9/203
66. Boll R, Schäfer JM, Richard B, Fehre K, Kastirke G, Jurek Z, et al. X-ray multiphoton-induced coulomb explosion images complex single molecules. Nat Phys (2022) 18:423–8. doi:10.1038/s41567-022-01507-0
67. Dallari F, Reiser M, Lokteva I, Jain A, Möller J, Scholz M, et al. Analysis strategies for MHz XPCS at the European XFEL. Appl Sci (2021) 11:8037. doi:10.3390/app11178037
68. Ferreira de Lima D, Davtyan A, Turkot O, Yefanov O, White T, Galchenkova M, et al. Automatic online data analysis optimization: application to serial femtosecond crystallography. In: preparation (2024).
69. European XFEL. Quality of data services (2025). Available at: https://www.xfel.eu/sites/sites_custom/site_xfel/content/e51499/e141242/e141245/xfel_file234455/Quality_of_data_services_01.2024_draft_eng.pdf (Accessed January 23, 2024).
Keywords: data reduction, photon science, X-ray free electron laser, data management, high-repetition rate, big data
Citation: Sobolev E, Schmidt P, Malka J, Hammer D, Boukhelef D, Möller J, Ahmed K, Bean R, Bermúdez Macías IJ, Bielecki J, Bösenberg U, Carinan C, Dall’Antonia F, Esenov S, Fangohr H, Ferreira de Lima DE, Ferreira Maia LG, Firoozi H, Flucke G, Gessler P, Giovanetti G, Koliyadu J, Madsen A, Michelat T, Schuh M, Sikorski M, Silenzi A, Sztuk-Dambietz J, Turcato M, Turkot O, Wrigley J, Aplin S, Hauf S, Wrona K and Gelisio L (2024) Data reduction activities at European XFEL: early results. Front. Phys. 12:1331329. doi: 10.3389/fphy.2024.1331329
Received: 31 October 2023; Accepted: 06 February 2024;
Published: 27 February 2024.
Edited by:
Iain Sedgwick, Rutherford Appleton Laboratory, United KingdomReviewed by:
Emmanuel Farhi, Soleil Synchrotron, FranceAndras Wirth, Diamond Light Source, United Kingdom
Copyright © 2024 Sobolev, Schmidt, Malka, Hammer, Boukhelef, Möller, Ahmed, Bean, Bermúdez Macías, Bielecki, Bösenberg, Carinan, Dall’Antonia, Esenov, Fangohr, Ferreira de Lima, Ferreira Maia, Firoozi, Flucke, Gessler, Giovanetti, Koliyadu, Madsen, Michelat, Schuh, Sikorski, Silenzi, Sztuk-Dambietz, Turcato, Turkot, Wrigley, Aplin, Hauf, Wrona and Gelisio. This is an open-access article distributed under the terms of the Creative Commons Attribution License (CC BY). The use, distribution or reproduction in other forums is permitted, provided the original author(s) and the copyright owner(s) are credited and that the original publication in this journal is cited, in accordance with accepted academic practice. No use, distribution or reproduction is permitted which does not comply with these terms.
*Correspondence: Egor Sobolev, ZWdvci5zb2JvbGV2QHhmZWwuZXU=
†Present address: Hans Fangohr, Max Planck Institute for the Structure and Dynamics of Matter, Hamburg, Germany