- 1Graduate School of Creative Science and Engineering, Waseda University, Tokyo, Japan
- 2School of Commerce, Waseda University, Tokyo, Japan
- 3University of Pittsburgh, Pittsburgh, PA, United States
Despite the recent complex intertwining of firms in fierce intellectual property disputes, the formation mechanisms of patent infringement litigation relationships between firms have been little studied from a network science perspective. We construct an inter-firm patent litigation network using longitudinal data on patent infringement litigation and the firms involved in the US, and examine its structural characteristics and the mechanisms of tie formation through network motif analysis, linking it to existing knowledge on negative ties. The results reveal the significant influence of mechanisms such as homophily, popularity, and activity on network formation, with reciprocity emerging as a pivotal factor. The absence of triadic closure is also observed. This distinct structural pattern is consistent across different technological fields and throughout the 20-year period. Furthermore, our analysis delves into the rapid countersuit strategies common within this network and provides valuable insights into patent litigation strategies between firms.
1 Introduction
In the recent surge of innovation research, patents are often regarded as the embodiment of innovation. The detailed documentation and public accessibility of patent data, including information about the innovators, the inherently elusive timing of innovation, its relationship to existing technologies, and its impact on subsequent technologies, make it highly favorable for data science. Network science approaches have also been applied to analyze, for instance, the time-series evolution of citation relationships between patents and patent co-ownership relationships between organizations [1–4], providing valuable insights for the field of innovation research.
However, network science has thus far paid little attention to understanding the competitive relationships between firms concerning intellectual property (IP). Patents are not just a record of inventions but a tool for competitive and defensive strategies that enable firms (as well as other types of organizations and individuals) to assert the value of their intellectual property and establish a competitive advantage by restraining others (e.g., [5]). In the modern innovation economy, the competition for IP is becoming increasingly fierce, and the demand to understand its dynamics has grown significantly. Among other strategic elements, patent litigation is of great importance for firms due to its lengthy disputes, substantial costs, and enormous risks for both plaintiffs and defendants [6, 7, 8]. The network science approach to understanding inter-firm patent litigation relationships is of high significance.
Patent litigation is the legal action taken by a plaintiff, which can be a firm, another organization, or an individual, to sue a defendant for infringing on the scope of a patent they hold, with the explicit strategic intention of inhibiting the activities of others and defending themselves [9]. Over the years, extensive research has been conducted on patent litigation and settlement mechanisms. A key focus of those studies was to identify the characteristics of patents likely to lead to litigation. Research indicates that, for instance, the higher the value of a patent, the greater the likelihood of infringement by other firms, hence increasing the probability of litigation [10, 11]. Additionally, differences in litigation strategies across technological fields have been explored. Notably, two highly innovative industries, the medical field (including pharmaceuticals and medical biology) and the computer field (IT-related technologies and other electronics), have frequently been compared. Literature suggests that in the medical field, strategic stakes are likely to be more important because each invention is challenging to imitate, and products often depend on a single invention. In the computer field, on the other hand, since multiple inventions are combined in end-products, and many similar products are on the market, firms are more likely to infringe on each other’s patents, leading to countersuits [5, 12–14]. Another main focus of existing studies has been on the characteristics of plaintiff and defendant firms. For example, large firms are deemed more ‘attractive’ as litigation targets because of the large sums they are capable of paying when they lose (or settle) a case (known as the deep pocket theory - [15–17]). Nevertheless, targeting large firms naturally entails the risk of countersuits, as they also have the capacity and strength to fight lawsuits. Indeed, countersuits are common, often resulting in repeated, fierce patent wars between the world’s largest firms (e.g., the famous case of Apple and Samsung–see, e.g., [18]).
Despite the accumulation of detailed knowledge on patents and firms involved in litigation, very few studies have focused on the inter-firm relationship structure. The limited existing studies that have done so base their findings on the unilateral, dyadic relationship between plaintiff and defendant firms (e.g., the impact of technological distance between the two firms on the likelihood of litigation occurrence) [19, 20]. In contrast, we introduce a network science perspective to investigate inter-firm relationships beyond dyads–the mechanisms by which one firm forms a litigious relationship with several firms, and whether the litigious relationship between two firms is influenced by third parties. In other words, we are interested in the competitive dynamics in networks of firms connected by adversarial relationships, rather than in the causes of each patent litigation case itself.
Inter-firm adversarial patent litigious relationships can be regarded as a type of ‘negative ties’ in social network science. The research on networks consisting of negative ties has become increasingly important in recent years. In particular, the mechanisms of formation of negative ties are of great interest, and various real-world data analyses and modeling have examined whether the formation mechanisms of positive ties–closure, reciprocity, homophily, popularity, and activity–also function as mechanisms for the formation of negative ties [21–25]. Examining whether these mechanisms are also at work in inter-firm patent litigation relationships would reveal how this relational structure shares common or different features with other networks of negative ties.
This paper constructs a patent litigation network among firms using the longitudinal data of patent infringement lawsuits that have arisen among firms in the US. The method of collecting the relevant data is described in detail in Sections 2.1, 2.2. Section 2.3 summarizes the possible mechanisms for negative tie formations that are examined in this paper. In Section 3.1, we identify the structural features of this network. We then identify the local relationship structures that are the building blocks of this network, i.e., the triadic network motifs (Section 3.2). Network motifs are statistically overrepresented subgraphs that reflect the local topology of a graph [26]. Triadic motifs are the smallest structural patterns that are essential for understanding the dynamics of complex networks, as they provide insights into the underlying mechanisms shaping network behavior. (In the following text, for simplicity, we use the term ‘motifs’ to refer to all network patterns of limited small size, not just statistically overrepresented patterns, as other studies have done (e.g., [27,28]).) Furthermore, we examine whether the pattern of occurrence of the motifs differs between technological fields (specifically, computer and medicine) and whether it has changed over time (Section 3.3).
The results provide us the following insights. The inter-firm patent litigation network is not merely a collection of isolated dyadic relationships, but exhibits a structure in which many firms are connected. It has two distinct clusters (= communities of the network) that are loosely connected. These two clusters are formed by litigation relationships in the computer-related field and the medical field, respectively. This suggests that the homophily mechanism is at work (as expected), whereby firms in the same technological field are more likely to be connected to each other. We also observe the working of the mechanisms of popularity and activity–indeed, some firms attract many incoming edges (i.e., being sued by many others) while some others have many outgoing edges (i.e., initiating many litigation cases). The edges bridging between different technological fields are not found to be due to firms ‘attacking’ indiscriminately many firms irrespective of their fields. The motif analysis reveals that triadic closure is largely absent in this network, while inter-litigious relationships (where firm A litigates against B and B litigates against A) occur significantly frequently, meaning that reciprocity is indeed one of the key mechanisms underlying tie formation in this network. Reciprocal interactions occur over a very short period, implying that litigation and countersuits tend to be short-term conflicts rather than expressions of long-term rivalry. The distribution of the occurrences of triadic motifs has remained unchanged over the past 20 years, with no significant differences by technological field. The mechanism of local tie formation thus seems universal, regardless of field or era.
We further analyze the most characteristic tie formation mechanism in this network, reciprocity, and when it occurs in inter-firm triadic relationships (Section 3.4). As mentioned earlier, litigation entails significant costs and firms seek to minimize their involvement in litigation with multiple parties. Consequently, they carefully select the parties with whom they maintain a close contentious relationship, which can be termed a mutual litigation relationship. We investigate which focal firms are inclined to adopt a countersuit strategy when sued by others, and which of the opponent firms they opt to countersue. In essence, we delve into whether the tie formation mechanisms (i.e., homophily, popularity, and activity) also contribute to fostering reciprocal relationships.
Finally, Section 4 provides a summary of the findings derived from these analyses.
2 Materials and methods
2.1 Scope of analysis and data collection
2.1.1 Scope of analysis
Since the focus of this paper is the dynamics of patent litigation relationships between firms, we analyze the structure of a network with firms as nodes and litigation relationships between firms as directed edges. Prior to constructing a network suitable for this scope of analysis, we clarify the definitions and conditions regarding the firms and the litigation relationships to be analyzed.
• Firms (= nodes in the network): In this paper, a “firm” refers to an organization registered as a legal entity, whereby all subsidiary firms associated with the parent entity are treated as a single firm. It is not limited to those whose registered place of incorporation is in the US; rather, it encompasses firms involved in litigation cases filed in the US, whether as plaintiffs or defendants.
• Litigation relationships (network edges): Although there are various types of patent litigation cases other than infringement (e.g., false marking, licensing, inventorship, etc.), our analysis focuses solely on infringement cases where both the plaintiff and defendant are firms, as defined in (1). We limit our scope to cases filed in the US, where the majority of patent litigation occurs, due to differences in litigation systems and laws across countries. Declaratory judgment cases, where the alleged infringer is the nominal plaintiff seeking a declaratory judgment of non-infringement, invalidity, or unenforceability, are also excluded from the analysis. Additionally, litigation cases in the International Trade Commission (ITC) are excluded from our analysis.
• Countersuits: In this paper, we refer to the scenario where firm A files an infringement lawsuit against firm B, and subsequently, B files an infringement lawsuit against A, as a ‘countersuit’ for convenience. These two lawsuits are considered independent and are represented in our network as a reciprocal relationship between the two nodes. Responses that a firm may take to a lawsuit filed against it (such as counterclaims, responses to declaratory judgment, etc.) are not considered as countersuits in this paper and are excluded from the analysis.
2.1.2 Data collection
We utilized data from the patent database Orbis Intellectual Property (hereafter Orbis IP), provided by Bureau van Dijk, one of the world’s largest providers of financial information, along with its corporate database Orbis. Orbis IP encompasses patent litigation data from across the globe, while Orbis includes data from organizations registered as legal entities worldwide. One major advantage of using these databases is their integration, allowing for reliable identification of the firms involved in litigation. Additionally, Orbis provides detailed firm data, facilitating the identification of firm name changes, mergers and acquisitions histories, and parent-subsidiary relationships between firms.
The data collection proceeded according to the following procedure. Initially, data on approximately 190,000 patent litigation cases spanning from 2000 to 2021 were extracted from Orbis IP. From this pool, only cases that met the specified criteria were retained, namely, infringement cases in the US excluding declaratory judgements (cases at the ITC were not included in this dataset), and those for which corporate information of both the plaintiff and defendant was available in the Orbis database. The detailed information provided by Orbis was then used to merge the firm information, identifying that the firms were the same, even if they had undergone name changes, mergers or acquisitions. When a firm is a subsidiary of a parent firm, we unified them under the name of the parent firm, as conflicts between firms are considered to occur at the parent firm level as well.
Consequently, the total number of litigated cases available for analysis (i.e., the number of network edges) amounted to 32,164, with a combined total of 13,831 plaintiff and defendant companies (i.e., the number of network nodes).
For each of these litigation cases, information was obtained on the filing date, the publication number of litigated patents (note that several patents may be associated with a single litigation case), the name of the plaintiff firm and the name of the defendant firm. Regarding each litigated patent, data on the International Patent Classification (IPC), the number of backward citations (i.e., prior patents cited by the litigated patent), and the number of forward citations (i.e., subsequent patents citing the litigated patent) was collected. For the firms involved, information on the list of owned patents, the number of forward and backward citations of those owned patents, and the number of times they self-cited the litigated patents was obtained. All of this information was available in Orbis IP and Orbis.
2.1.3 Verification of data reliability
The Stanford Patent Litigation Database (hereafter referred to as the Stanford Data) [29] is a publicly available dataset that provides information about all US patent infringement cases from 2000 to 2021. We confirmed that all the cases included in the Stanford Data (76,406 with declaratory judgement cases and 71,172 without them) are also included in the data we extracted from Orbis IP. Within the Stanford Data, asserters for each case are categorized into 13 different types (e.g., individual, failed startup, product company, IP and subsidiary of product company), with ‘product companies’ being the most frequent asserters, accounting for 35,756 cases.
In our analysis, the definition of a ‘firm’ does not necessarily align with the categorization of ‘product companies’ within the Stanford Data (see Section 2.1.1). Additionally, we only focus on cases where the defendant is also a firm, which are not identifiable by the Stanford Data. Consequently, directly extracting cases with product companies listed as asserters from the Stanford Data was not feasible. However, the number of lawsuits included in our analysis (32,164) closely matches the count of such cases (35,756), indicating a reasonable coverage of our data. Furthermore, the reliability and legitimacy of our data were further supported by rigorous validation procedures and the reputable nature of the Orbis IP database.
Also, as mentioned in Section 2.1.1, declaratory judgment (DJ) cases are not included in our analysis. This exclusion is due to the distinct strategic approaches in different types of litigation; specifically, a firm’s DJ strategy differs from its infringement litigation strategy (e.g., [30]). In DJ cases, the alleged infringer initiates the case to seek a declaratory judgment of patent non-infringement or invalidity, thus positioning themselves as the plaintiff rather than the defendant. Including DJ cases in the network would require different data preprocessing (such as reversing the direction of connections) to accurately represent these different initiating mechanisms, which is advisable to avoid from a network analysis perspective. To ensure that excluding DJ cases did not skew our results, we conducted a preliminary investigation of DJ case edges. We identified 1,096 DJ cases, representing only 3% of all litigation cases. The majority of these cases’ edges overlapped with existing edges in the network, with only seven unique new edges found. This demonstrates that the exclusion of DJ cases does not significantly affect the outcomes of our analysis.
2.2 Relevant background knowledge and data pre-processing
2.2.1 Litigated patents, NPEs, and PAEs
A patent-in-suit, which serves as the initial basis for a lawsuit, is a patent owned by the plaintiff, the party bringing the lawsuit. The plaintiff firm initiates legal action, alleging that the defendant firm has infringed upon the rights granted by a patent it owns. In other words, the plaintiff is necessarily restricted to firms that own patents, while the defendant firm could theoretically be one that does not own any patents. A firm without a single patent cannot counter-sue. In essence, the inter-firm patent litigation network we construct in this paper includes firms that can only have incoming edges but no outgoing edges.
Conversely, there are also firms that have outgoing edges but do not have incoming edges. Known as non-practicing entities (NPEs), these firms do not develop or sell products/services, thus they face no risk of infringing on others’ patents. NPEs include, for example, firms that invent but do not develop or market products based on their inventions (e.g., universities and research institutions), those that do not invent but profit through patent licensing, and those commonly referred to as ‘patent trolls’ that seek profits by suing other firms using patents obtained from third parties [31]. According to the Stanford Data, 12 of the 13 types of asserters (excluding product companies) are defined as NPEs. Three of these types are specifically categorized as patent assertion entities (PAEs), which are commonly referred to as ‘patent trolls’ [29]. In our analysis, the presence of PAEs, known for aggressively suing other firms without the risk of being countersued, may affect the network structure. Although the broader impact of PAEs on the U.S. patent system is beyond the scope of this paper, it is crucial to assess whether their presence affects the results of our analysis. Using the Stanford data, we identified 570 PAEs in our dataset.
2.2.2 Technological fields of litigated patents
Each patent is associated with one or more classification codes representing its field of technology. Several classification systems are in use worldwide, varying based on jurisdiction and intended application. In this study, the specifics of technological fields are beyond the scope of our analysis. We employ technological field information primarily for comparing patent litigation strategies in the computer and medicine fields, the two largest and most innovative industries. Thus, we use the International Patent Classification (IPC) codes, which is a consistent classification used throughout the analyzed period.
The correspondence between IPC codes and technology fields is not clearly defined. In this context, we examine the class level, which is the second hierarchical level of classification (for further information on the classification, refer to [32]). In our dataset, the most prevalent IPC class code was G06 (Computing; Calculating; Counting) (10,203 litigation cases), followed by H04 (Electric communication technique) (9,035 cases), and then A61 (Medical or veterinary science; Hygiene) (7,654 cases). Note that G06 and H04 had a substantial number of co-occurrences, totaling 5,095 cases. Consequently, we consider these two classes as indicative of the computer-related field, while A61 represents the field of medicine.
Additionally, there were 740 cases where both the computer field classes (i.e., G06 or H04) and the medicine field class (A61) co-occurred, indicating the existence of lawsuits spanning both these fields.
2.3 Mechanisms for negative tie formations
The mechanisms by which negative ties are formed between actors (nodes) in social networks differ from those by which positive ties are formed (e.g., [33]), and various real data analyses and models have examined these differences. The fundamental tie-forming mechanisms include closure, reciprocity, homophily, popularity, and activity. Whether each of these mechanisms drives negative relationships depends to a large extent on the type and context of the relationship, but some are thought to function or not universally regardless of these factors [21–25].
Below, each mechanism is briefly described, and existing findings on their presence/absence in negative networks are summarized, primarily based on the validation and discussion by [25].
• Triadic closure is the tendency for an edge to form between two nodes already connected to one node. The existing literature generally agrees that negative tie triadic closure is very weak or completely absent.
• Reciprocity refers to the tendency between two nodes to form an edge in the opposite direction when there is an edge from one to the other. Some studies suggest that reciprocity exists in negative tie networks, while others argue that it is entirely absent.
• Homophily refers to the tendency for nodes to form edges with other nodes that share similar attribute values. Existing literature has found homophily to be weaker or absent in negative networks.
• Popularity (also well known as preferential attachment) is the tendency for nodes receiving incoming edges to attract additional incoming edges. This phenomenon has been widely reported in both positive and negative networks.
• Activity refers to the tendency for nodes sending outgoing edges to continue sending more outgoing edges. Although literature on activity in negative networks is sparse, some studies have reported the presence of this mechanism as well.
The presence of these mechanisms in forming patent litigation relationships between firms is investigated in Section 3.
3 Analysis and results
3.1 Characteristics of the inter-firm patent litigation network
An inter-firm patent litigation network was constructed with 13,831 firms as nodes and 32,164 litigation relationships as directed edges from the plaintiff (the owner of the patent in suit) to the defendant. Multiple edges were formed when multiple litigations occurred between the same plaintiff/defendant pair.
The network consisted of the largest connected component, which contained approximately 60% of the nodes (8,038 firms) and 92% of the edges (29,624 litigations), along with several small components. The largest connected component is composed of two loosely connected clusters: a larger cluster formed by lawsuits in the computer-related field (G06 and H04) and a smaller cluster formed by lawsuits in the medicine-related field (A61) (see Figure 1A). This suggests that the majority of lawsuits occur within the same technological field, with the homophily mechanism contributing to the formation of litigation relationships. However, there are also instances where lawsuits span across different fields, indicating that homophily is not the predominant driving force in all cases.
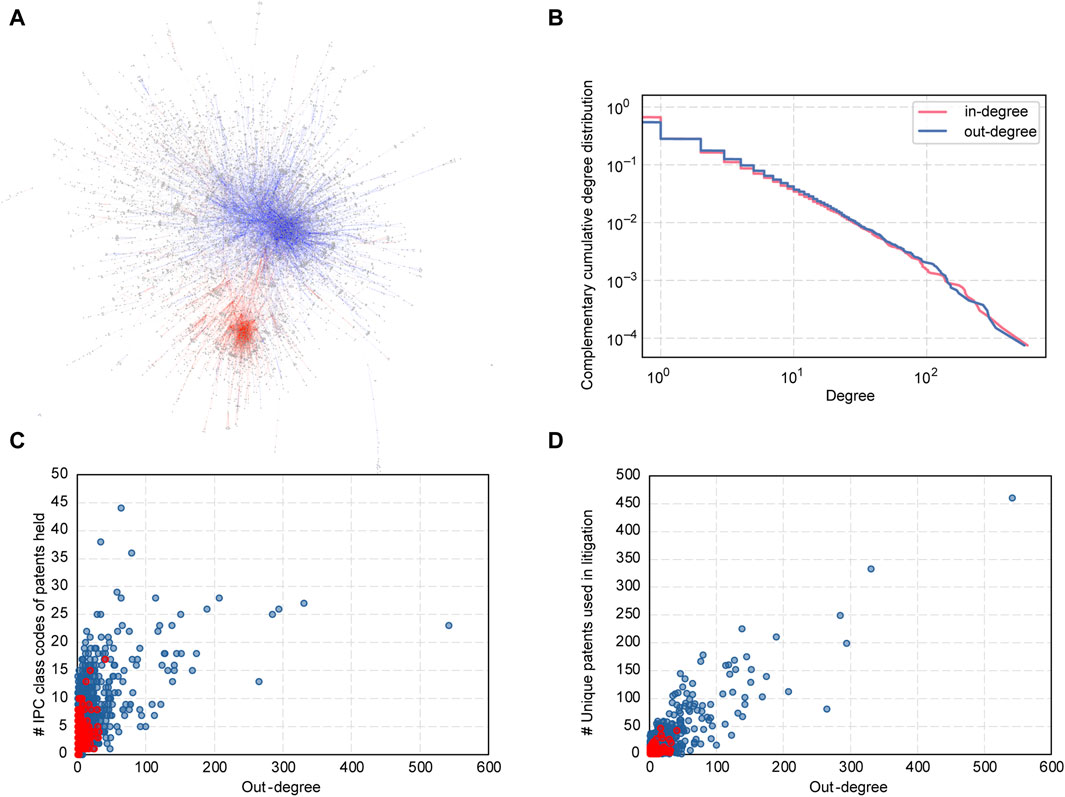
Figure 1. The inter-firm patent litigation network. (A) Structure of the largest connected component, visualized by applying a force-directed layout algorithm. Blue edges represent G06 or H01 (litigations in the computer field) and red edges represent A61 (those in the medicine field). (B) Complementary cumulative in- and out-degree distributions, with multiple edges between two edges being each counted. (C) Scatterplot illustrating the out-degrees of plaintiff firms (red: PAEs, blue: other firms) against the total number of unique IPC class codes associated with their patents. (D) Scatterplot depicting the out-degrees of plaintiff firms against the total number of unique patents utilized in litigation. Red data points denote PAEs, while blue data points denote other firms.
The in- and out-degree distributions of this network (see Figure 1B) indicate that most firms are involved in only one or a few lawsuits, while a small number of firms are involved in many. This suggests that both popularity and activity are key mechanisms underlying the formation of litigation relationships. One possible explanation for the presence of a few firms with high out-degrees and the large connected component is that PAEs in particular may have many outgoing edges and connect many firms across different fields. However, as shown in Figure 1C, the out-degrees of PAEs are not particularly high in our sample, nor do they hold a significant number of unique IPC class codes. Furthermore, Figure 1D reveals a clear positive correlation between the number of lawsuits filed by a firm (i.e., out-degree) and the total number of unique patents used in these lawsuits. This trend is consistent for PAEs, which are not characterized by a higher number of litigations or a repetitive use of a limited number of patents compared to other firms.
This aspect of our findings is worth commenting upon, given the importance of PAEs in the literature on IP litigation. The possible negative impact of PAEs on innovation is a major concern for relevant practitioners and scholars, and the number of lawsuits filed by PAEs and the monetary awards to PAEs are reported to be growing (while there are also new indications that PAEs are not actually pervasive [34]). On the other hand, these existing investigations did not examine the positioning of PAEs in the litigation network (e.g., whether certain PAEs have a significantly high out-degree). Further pursuing the identity of PAEs requires detailed data to assess whether some PAE litigants are single-purpose vehicles affiliated with larger practicing entities, an intriguing possibility beyond the scope of this study. Nevertheless, these results suggest that a network analysis approach may offer us a new understanding of PAEs and that, at the very least, they did not appear to have a significant impact on the structural characteristics of the network as a whole.
3.2 Identifying triadic motifs
The adversarial relationships between firms, as expressed in litigation, are not transient phenomena. Therefore, we employed triadic motif identification (utilizing the tool provided by [35]) on the cumulative network, which included all litigation relationships, irrespective of the timing of the litigation events. More specifically, we calculated the frequency of occurrence of k-size node-induced motifs with k = 3 in the directed graph and their statistical significance. The statistical significance of each motif occurrence was determined using the z-score, calculated as follows:
Here,
Figure 2 displays the z-score corresponding to each of the 13 directed triadic motifs, along with the potential time evolution paths among these motifs. These results reveal the following findings. Firstly, instances where a firm unilaterally sues multiple firms (Motif-0) or is unilaterally targeted by several firms (Motif-1) are notably rare. Chain relationships such as Motif-2 (involving different parties suing and being sued) are also significantly infrequent. That is, when these two-edge open triads occur, it appears that the relationship among the three firms often evolves into triads with three or four edges.
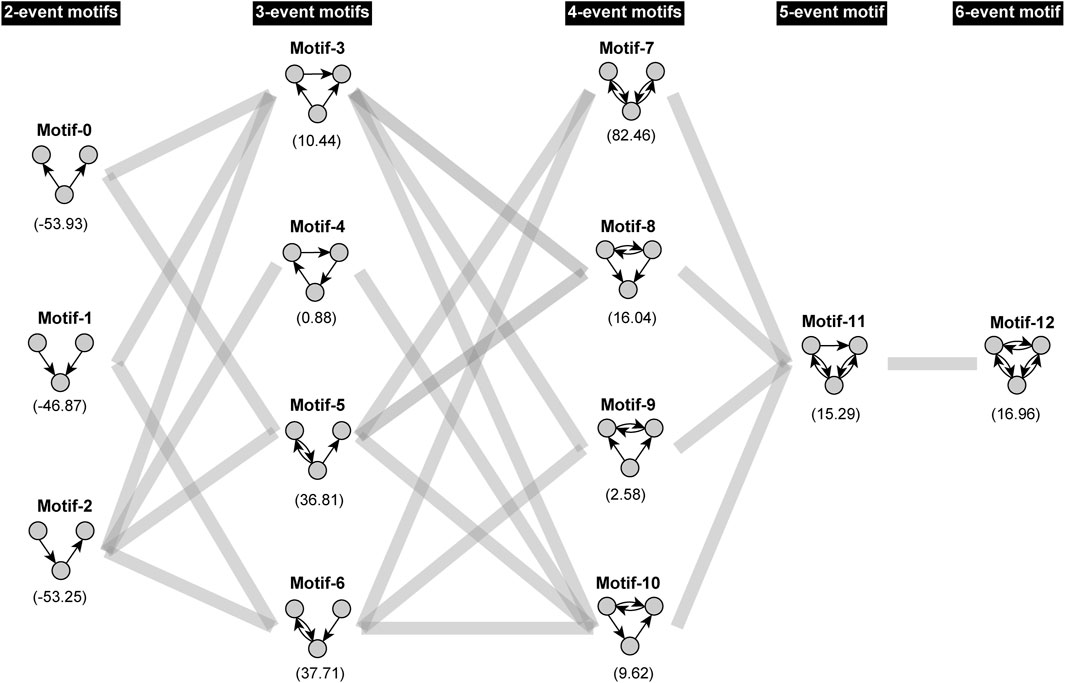
Figure 2. Triadic (3-node) network motifs. The number in brackets below the diagram for each motif represents the z-score for that motif. The semi-transparent lines between motifs indicate possible transitions between them. For instance, Motif-0 is a 2-event motif, but could transition to Motif-3 or Motif-5 when the third event (=litigation) occurs.
Motif-0 can transition into either Motif-3 or Motif-5, but Motif-5 is significantly more common. Similarly, Motif-1 can transition into either Motif-3 or Motif-6, but Motif-6 is significantly more common. It can thus be inferred that when one firm sues multiple firms (Motif-0) or is sued by multiple firms (Motif-1), the relationship often develops into a situation where the firm countersues one of the attacking firms or is countersued by one of the litigants. Reciprocal, or mutually litigious, relationships are highly probable. This distinctive characteristic of litigation relationships sets them apart from other types of negative relationships reported in existing studies [24, 25].
Compared to Motif-5 and Motif-6, Motif-3 and Motif-4 (closed triads) are notably less prevalent, suggesting that triadic closure is very seldom observed in this network. This finding aligns with discussions in existing literature regarding negative ties. Specifically, cyclical triads (Motif-4) are entirely absent, which is to be expected given that cyclical relationships are not feasible in the context of litigation relationships between firms.
The numbers of years and the frequencies of evolution of 2-event motifs into 3-event motifs are depicted in Figure 3. Motif-3 can be formed through three developmental pathways originating from Motifs-0, 1, and 2, respectively, with all three pathways showing similar frequencies over the 20-year period. Approximately 60% of the transformations occurred within 10 years, indicating a gradual formation of Motif-3. This indicates that the development of 2-event open triads (Motifs-0, 1, and 2) into closed transitive triads (Motif-3) may not be driven by any competitive dynamics between firms and could potentially occur by chance.
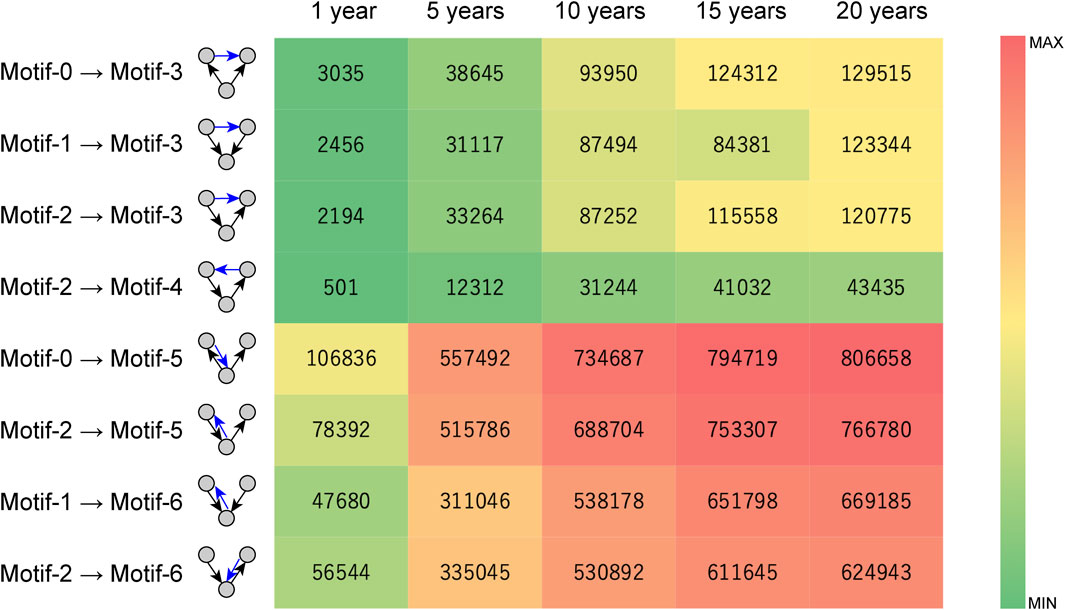
Figure 3. Heatmap summarizing the frequency of formation of each 2-event motif (indicated by the presence of two black edges in each corresponding diagram) and its subsequent transformation into the specified 3-event motif (represented by the blue edge in the diagram), over varying time intervals (y [years], where y = 1, 5, 10, 15, 20).
On the other hand, Motif-5 has two developmental pathways, originating from Motif-0 or 1, and Motif-6 has two pathways, originating from Motif-1 or 2. All four pathways showed similar frequencies over the 20-year period, akin to Motif-3. In contrast to Motif-3, however, around 60% of Motifs-5 and 6 formed within 5 years, and this percentage reached 80% to more than 90% within 10 years. This suggests that the strategy of countersuit when a firm faces litigation is more likely to be implemented within a relatively short time frame, typically within a few years.
Shifting the focus to 4-event motifs that inevitably include at least one countersuit (see Figure 2 again), Motif-7, an open triad with two countersuits, exhibits a z-score greater than 82, signifying its significantly higher frequency of occurrence when contrasted with other 4-event closed triad motifs. This motif evolves from Motif-5 or 6, and has a significantly larger z-score than Motifs-8 to10, which can also stem from the same Motif-5 or 6. Therefore, it suggests that the mechanism of triadic closure is much weaker than the mechanism of reciprocity in this network.
The observation also implies that a substantial number of the three-firm relationships persist in the Motif-7 form, rather than evolving into a closed triad (Motif-11).
Consistently, these results demonstrate that litigation is primarily driven by a reciprocal adversarial relationship. The mechanism by which a litigation relationship with one party triggers litigation with another is not in operation, while the mechanism by which litigation induces countersuits is notably robust.
Here, to facilitate understanding of these results and their practical implications, we provide a concrete illustration using the example of a smartphone patent litigation network diagram (see [36]), which has recently garnered significant attention. While network diagrams such as this example [36] offer an intuitive understanding of the complexity of inter-firm competitive relationships, they do not unravel that complexity. In contrast, our analysis yields several key findings. Firstly, even if three firms have established a closed triangular relationship, it is highly likely that these relationships arose independently of each other. The fact that firm A is in a litigation relationship with firms B and C does not influence the relationship between firms B and C (i.e., the absence of the triadic closure mechanism). Moreover, although many cases are observed where firms unilaterally sue multiple firms or are sued by multiple firms, it is very unlikely that these relationships will remain static. There is a high probability of countersuits, typically within 10 years (i.e., the dominance of the reciprocity mechanism). Thus, our analysis may be useful in identifying firms with varying levels of distinction and relationships regardless of their presence in the industry, as well as in foreseeing their potential future evolution.
In the next section (Section 3.3), we continue our investigation to determine if the mechanisms behind litigation relationship formation vary across different technology sectors and over time. Furthermore, in Section 3.4, we delve into the dominant formation mechanisms of reciprocal relationships. For instance, in the smartphone industry, firms like Apple and Samsung, despite being sued by multiple firms (i.e., high popularity), do not always countersue all their opponents. We explore which types of firm pairs are more likely to develop reciprocal relationships, where countersuits occur.
3.3 Comparison between the two fields and temporal changes in motif significance
This section investigates whether the mechanisms for constructing litigation relationships differed across technological fields and whether they changed over the years.
Figure 4A displays the z-scores of the 13 directed triadic motifs calculated in the entire inter-firm patent litigation network, as well as in the computer (G06 and H04) and medicine (A61) fields subnetworks. From this figure, it is evident that the occurrence pattern of the motifs is nearly identical in the computer and medicine fields. As indicated in the figure caption, comparing absolute z-score values between different networks is not feasible. Nevertheless, in all the networks (overall, G06, H04, and A61), the trends remained consistent: Motifs-0, 1, and 2 were significantly less frequent in comparison to the other motifs, while Motifs05, 6, and 7 were more frequent.
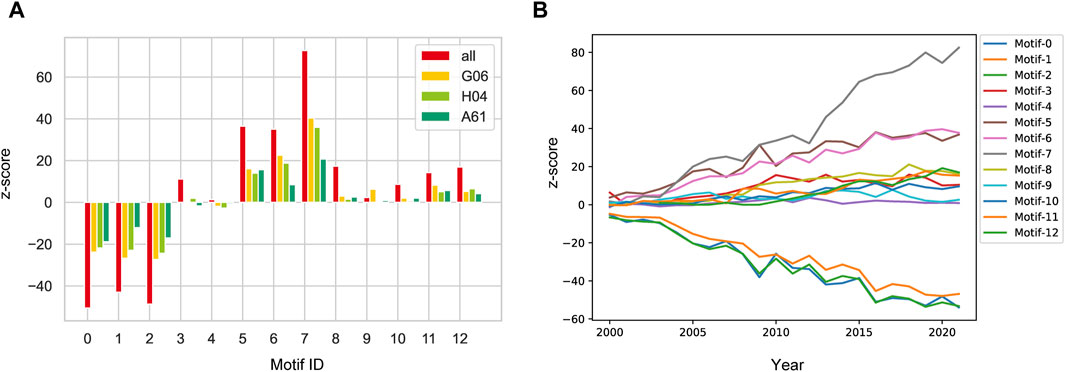
Figure 4. z-score of each motif. (A) Differences in z-scores of Motifs-0 to 12 were compared between the computer (G06 and H04) and medical (A61) fields. “all” (the red bars) show the same values as the z-scores shown in Figure 2. For each of G06, H04 and A61, the lawsuit with that IPC classification were extracted and the motif significance was calculated for the network formed by those edges. Although absolute values of z-scores should not be compared between networks of different sizes, z-scores of the 13 motifs in the same network can be compared. (B) Time-series variation of the z-scores of Motifs-0 to 12. The values for a given year are the result of applying motif identification to a cumulative network consisting of lawsuits that occurred from 2000 to that year. Note that it is inappropriate to compare absolute values of z-scores between different years, as the size of the network is different in each year. However, it is reasonable to compare z-scores between motifs in a given year.
Figure 4B shows the results of the pattern of occurrence of motifs over time. Litigation has increased over the years, and the network size varies annually. However, the graph reveals that the rank order of the significance of motif occurrence remained consistent throughout the 20-year period, while the differences in absolute values increased from year to year. In other words, over the years, motifs that appeared less frequently than expected became significantly less frequent, and motifs that appeared more frequently became significantly more frequent.
These results suggest that, at least during the period analyzed, there were no substantial changes in the compositional mechanisms of the litigious relationships, and no clear differences were observed between fields.
3.4 Mechanism of countersuits
In this section, we perform a logistic regression analysis to examine the dynamics of reciprocity. We specifically focus on the factors that prompt firms to initiate countersuits in response to being sued by multiple other firms. Most importantly, the analysis seeks to determine whether the tie formation mechanisms–popularity, activity, homophily–identified in Section 3.1 are also pivotal in the development of reciprocal relationships.
Let A be the focal firm and assume that firm A is sued by multiple firms Bi (i = 1, … , n and n ≥ 2), each at time ti (illustrated in Figure 5). The dependent variable in the analysis is whether or not firm A sued firm Bi back at any given time at time tc (>ti) (=1, if it did). Table 1 provides a summary of the independent variables employed in the analysis, along with descriptions of the rationale and hypotheses guiding their selection. These variables encompass measures of both firms’ popularity (IN_DEG) and activity (OUT_DEG). In addition, BtoA_CNT asseses whether frequent unilateral litigations provoke reciprocal actions. Here, we also introduced TECH_PROX, which quantifies the extent of technological homophily, determined by the degree of overlap in unique IPC codes associated with patents in the respective portfolios of the involved firms. While established metrics exist for evaluating technological proximity between two firms [37], they may yield inaccurate results when portfolios exhibit substantial variance in size. Our devised simple variable offers a nuanced perspective, focusing on the degree of competition between portfolios rather than their overall similarity.
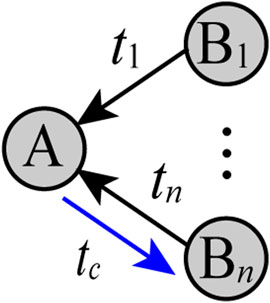
Figure 5. The setting for examining the mechanism of countersuits. The focal firm A was litigated by firm Bi (where i = 1, … , n and n
Additional variables include PORT_SIZE and MAX_FC, which are unrelated to the network structure. For the variable MAX_FC, we utilized forward citation data, representing the number of citations for each patent. This metric is commonly employed in research as a surrogate indicator of patent value [38–40]. Nevertheless, caution is advised, as several studies emphasize the need to consider differences across fields and the time interval between a patent’s filing date and its citation [41,42]. In this study, we simplify our approach by using the maximum number of forward citations among all patents held by a firm. We do not account for the filing dates of patents; instead, we rely solely on the number of citations each patent received by the end of 2021. We acknowledge that this metric may not provide a precise measure of a firm’s possession of high-value patents. However, it has been used in prior studies to discuss patent value [11], making it a readily available variable for our analysis.
The results of the logistic regression analysis, presented in Table 2, unveil several noteworthy findings. Firstly, the degree of technological competition (homophily) between the firms significantly contributes to the emergence of reciprocal relationships, aligning with the broader network characteristics identified in Section 3.1. Moreover, it appears that a smaller portfolio size and lower outdegree (i.e., activity) of the plaintiff firm correlate with a heightened likelihood of the defendant firm initiating countersuits. In other words, firms are less inclined to countersue when the opponent has a larger portfolio or has a history of filing numerous lawsuits. On the contrary, firms tend to respond with countersuits when they face repeated attacks (BtoA_CNT).
Additionally, defendant firms with higher out-degree (i.e., greater activity) are more inclined towards adopting a countersuit strategy, a finding consistent with expectations. Furthermore, firms with lower in-degree (i.e., lesser popularity) demonstrate a greater propensity for employing countersuits. This suggests that the decision to adopt a countersuit strategy is not primarily driven by a ‘learning effect’ from other firms’ strategies, where being frequently targeted prompts the adoption of similar strategies. Instead, the individualistic factor of having the resources to counterattack, especially when not frequently targeted by other firms, is more influential.
Moreover, our analysis indicates that the status of the opponent as a PAE, i.e., an opponent who is immune be sued on the basis of patent infringement, does not exert a significant effect. Similarly, the variable associated with the number of patent citations was found to be insignificant, implying that whether or not the firm or its opponent holds a patent with high value does not impact countersuits.
In summary, our findings suggest that mechanisms related to homophily, activity, and popularity play pivotal roles in the evolution of unilateral inter-firm litigation relationships into reciprocal ones.
4 Discussion
4.1 Findings and implications
We constructed an inter-firm patent litigation network based on real data and investigated the characteristics of the relationship structure and its formation mechanisms. Despite the dyadic nature of litigation relationships, our analysis revealed that more than 8,000 firms across various technological fields were directly or indirectly connected by litigation relationships. The network exhibited two distinct clusters, one primarily comprising litigation in the computer field and the other in the medical field. The formation of this network structure was influenced by several factors, including the tendency for litigation to occur between firms in the same technological field (homophily), the presence of firms initiating multiple lawsuits (activity), and the presence of firms facing multiple lawsuits (popularity).
The identification of directed triadic motifs and the analysis of their frequency over time yielded the following findings: (1) litigation tends to be more mutual than unilateral, indicating that reciprocal relationships are prevalent in the patent litigation network; (2) litigation relationships are primarily dyadic disputes between two firms and are unlikely to develop into triadic relationships involving a third firm; (3) reciprocal litigation relationships tend to occur in a short period of time, suggesting that countersuits are a prompt and reactive strategy; and (4) these characteristics are commonly observed in both the medical and computer fields, and have been consistent throughout the past 20 years. Our further investigation of the mechanism of mutual relationships (countersuits) revealed that (1) firms tend to respond to repeated attacks with countersuits; (2) a high technological homophily between firms correlates with a higher likelihood of countersuits; (3) they are less likely to countersue if the opponent is belligerent (i.e., high activity) and has a strong patent portfolio; and (4) they are more inclined to countersue when the opponent has been targeted by many others (i.e., high popularity). In addition, the characteristics of the countersuing firms were identified as having litigated many other firms (i.e., high activity) and not being litigated by many others (i.e., low popularity).
Our approach enables the network participants and observers to benefit from the whole-of-network perspective as compared to the more limited dyadic and ego-network perspectives. This wide-angle and dynamic view of litigation networks may provide early warning of emerging “hot spots” in IP conflicts or help identify influential nodes who may not be the biggest names in the industry. As discussed in Section 3.2, the mere visualization of inter-firm litigation relationships (e.g., [36]) only offers an intuitive comprehension of their complexity, but does not fully elucidate it. Our study provides insight into the structural characteristics of the network (e.g., which patterns are significantly more or less common), the mechanisms by which they are formed, and their potential future evolution. In addition, our analysis also both supports some of the network properties that are familiar from other realms of network theory and highlights interesting overall patterns (such as the finding that NPEs appear not to play as critical a role as anecdotal coverage of NPEs might suggest). This may serve as a useful guidepost for the next steps in patent litigation research.
Moreover, as the adversarial relationships between firms in the IP field have been thus far insufficiently studied in the context of negative ties [43], the findings of this study (e.g., identification of the (non-)existence of the formation mechanisms) will also contribute to the development of research on negative networks.
4.2 Limitations and future work
We acknowledge several limitations of this study. One limitation concerns the data used. Although the Orbis IP data is widely recognized for its high degree of integration and comprehensiveness, it may not capture all information, such as the relationships between parent firms and their affiliates. This is particularly relevant as PAEs often go to great lengths to mask ownership of single-purpose entities used for litigation, which may affect the characteristics of these entities identified in our analysis. However, PAEs are not the focus of our study, and this limitation is inherent to any dataset. To our knowledge, there is no alternative data source more suitable for the analysis conducted in this paper. We anticipate that future developments in data availability will enable more comprehensive analysis, in which we believe our approach will remain valid.
PAEs’ insignificance in the litigation network may also be related to other factors, such as the characteristics of their patent stocks. Further detailed investigation requires capturing patent values, which is another limitation of this study. This study does not fully consider the value of each patent and its impact on related fields and markets. The number of forward citations of a patent is typically used as a quick measure to estimate its value, and this approach was adopted in this paper. However, more sophisticated measures have been proposed to quantify patent value [44], suggesting the need for further exploration of how patent value influences litigation strategies.
Additionally, this study does not explore the consequence of litigation. As mentioned earlier in this paper, the mechanisms of litigation settlement are of great interest to both practitioners and academics (e.g., [45]). Nevertheless, the impact of networks beyond the dyadic relationship between two firms on settlement decisions has not been fully addressed. This is a complex area that could benefit from further detailed investigation.
From a network science perspective, it would be valuable to simultaneously investigate the mechanisms of the formation and disappearance (or subsidence) of negative ties. The interactions between negative and positive ties are also important issues to explore. Research on both negative and positive edges within the same node set has not been extensively pursued [25]. Positive (albeit strategic) relationships can also arise between firms involved in IP disputes, such as strategic alliances, patent co-ownership, and acquisitions, warranting investigation of these dynamics for both academic and practical purposes.
Additionally, a more detailed analysis of when statistically underrepresented local structures (such as cycles or unilateral attacks) occur in reality would be valuable from a managerial perspective. This ties back to the identification of firms that are not major players but may be influential. From a technical standpoint, constructing and operating temporal network null models [46] that account for the dominance of dyadic relationships warrants future work.
Data availability statement
The data analyzed in this study is subject to the following licenses/restrictions: The data supporting this study are partially sourced from publicly available data and partially obtained through commercial third-party providers. Requests to access these datasets should be directed to Stanford NPE Litigation Database (https://npe.law.stanford.edu); Orbis Intellectual Property (https://www.bvdinfo.com/en-gb/ourproducts/data/international/orbis-intellectual-property).
Author contributions
TK: Writing–original draft, Writing–review and editing, Conceptualization, Data curation, Formal Analysis, Funding acquisition, Investigation, Methodology, Project administration, Resources, Supervision, Validation, Visualization. YM: Data curation, Formal Analysis, Validation, Visualization, Writing–review and editing. JY: Conceptualization, Data curation, Project administration, Resources, Writing–review and editing. RM: Conceptualization, Investigation, Writing–review and editing.
Funding
The author(s) declare financial support was received for the research, authorship, and/or publication of this article. TK acknowledges the support by JSPS KAKENHI Grant (Grants-in-Aid for Scientific Research) No. 22H00884.
Conflict of interest
The authors declare that the research was conducted in the absence of any commercial or financial relationships that could be construed as a potential conflict of interest.
Publisher’s note
All claims expressed in this article are solely those of the authors and do not necessarily represent those of their affiliated organizations, or those of the publisher, the editors and the reviewers. Any product that may be evaluated in this article, or claim that may be made by its manufacturer, is not guaranteed or endorsed by the publisher.
References
1. Verspagen B. Mapping technological trajectories as patent citation networks: a study on the history of fuel cell research. Adv Compl Syst (2007) 10:93–115. doi:10.1142/s0219525907000945
2. Funk RJ, Owen-Smith J. A dynamic network measure of technological change. Manag Sci (2017) 63:791–817. doi:10.1287/mnsc.2015.2366
3. Acemoglu D, Akcigit U, Kerr WR. Innovation network. Proc Natl Acad Sci USA (2016) 113:11483–8. doi:10.1073/pnas.1613559113
4. Fleming L, Mingo S, Chen D. Collaborative brokerage, generative creativity, and creative success. Admin Sci Q (2007) 52:443–75. doi:10.2189/asqu.52.3.443
5. Cohen W, Nelson R, Walsh J Protecting their intellectual assets: appropriability conditions and why U.S. manufacturing firms patent (or not). Cambridge, MA: National Bureau of Economic Research (2000).
6. Bhagat S, Bizjak J, Coles JL. The shareholder wealth implications of corporate lawsuits. Financ Manage (1998) 27(4):5–27. doi:10.2307/3666410
7. Raghu TS, Woo W, Mohan SB, Rao HR. Market reaction to patent infringement litigations in the information technology industry. Inf Syst Front. (2008) 10:61–75. doi:10.1007/s10796-007-9036-5
8. Yang D. Patent litigation strategy and its effects on the firm. Int J Manag Rev (2019) 21:427–446. doi:10.1111/ijmr.12202
9. Awate KS, Makhija M. A Trojan horse inside the gates? Knowledge spillovers during patent litigation. Acad Manage J (2022) 65(5):1747–69. doi:10.5465/amj.2018.1181
10. Cooter RD, Rubinfeld DL. Economic analysis of legal disputes and their resolution. J Econ Lit (1989) 27(3):1067–97.
11. Lanjouw JO, Schankerman M. Characteristics of patent litigation: a window on competition. RAND J Econ (2001) 32(1):129–51. doi:10.2307/2696401
12. Somaya D. Strategic determinants of decisions not to settle patent litigation. Strateg Manag J (2003) 24(1):17–38. doi:10.1002/smj.281
13. Somaya D. Firm strategies and trends in patent litigation in the United States. Intellect Property Entrepreneurship (2004) 15:103–47. doi:10.1016/S1048-4736(04)01504-8
14. James SD, Leiblein MJ, Lu S. How firms capture value from their innovations. J Manag (2013) 39(5):1123–55. doi:10.1177/0149206313488211
15. Chen CV. Of trolls, davids, goliaths, and kings: narratives and evidence in the litigation of high-tech patents. N C L Rev (2008) 87:1571–616.
18. Kastrenakes J Apple and Samsung settle seven-year-long patent fight over copying the iPhone. The Verge (2018). Available from: https://www.theverge.com/2018/6/27/17510908/apple-samsung-settle-patent-battle-over-copying-iphone (Accessed June 4, 2024).
21. Lusher D, Robins G. Chapter 3: formation of social network structure. In: Exponential random graph models for social networks: theory, method and applications. New York, NY: Cambridge University Press (2013).
22. Lusher D, Robins G. Chapter 5: example exponential random graph model analysis. In: Exponential random graph models for social networks: theory, method and applications. New York, NY: Cambridge University Press (2013).
23. Papachristos AV, Hureau DM, Braga AA. The corner and the crew: the influence of geography and social networks on gang violence. Am Sociol Rev (2013) 78(3):417–47. doi:10.1177/0003122413486800
24. Boda Z, Néray B. Inter-ethnic friendship and negative ties in secondary school. Soc Netw (2015) 43:57–72. doi:10.1016/j.socnet.2015.03.004
25. Harrigan N, Yap J. Avoidance in negative ties: inhibiting closure, reciprocity, and homophily. Soc Netw (2017) 48:126–41. doi:10.1016/j.socnet.2016.07.003
26. Milo R, Shen-Orr S, Itzkovitz S, Kashtan N, Chklovskii D, Alon U. Network motifs: simple building blocks of complex networks. Science (2002) 298:824–7. doi:10.1126/science.298.5594.824
27. Paranjape A, Benson AR, Leskovec J. Motifs in temporal networks. In: ACM Conference on Web Search and Data Mining. Association for Computing Machinery (ACM) Digital Library (2017). p. 601–10. doi:10.1145/3018661.3018731
28. Liu P, Masuda N, Kito T, Sarıyüce AE. Temporal motifs in patent opposition and collaboration networks. Sci Rep (2022) 12:1917. doi:10.1038/s41598-022-05217-8
29. Miller SP. Who’s suing us? Decoding patent plaintiffs since 2000 with the Stanford NPE litigation dataset. Stan Tech L Rev (2018) 21:235.
30. Rudy BC, Black SL. Attack of defend? The role of institutional context on patent litigation strategies. J Manag (2018) 44(3):1226–49. doi:10.1177/0149206315605168
31. Cohen L, Gurun UG, Kominers SD. Patent trolls: evidence from targeted firms. Manag Sci (2019) 65(12):5461–86. doi:10.1287/mnsc.2018.3147
32. IPC International patent classification (IPC) (2023). Available from: https://www.wipo.int/classifications/ipc/en/ (Accessed June 4, 2024).
33. Everett MG, Borgatti SP. Networks containing negative ties. Soc Networks (2014) 38:111–20. doi:10.1016/j.socnet.2014.03.005
34. The 2024 Marcum Patent Litigation Study The 2024 marcum patent litigation study (2024). Available from: https://info.marcumllp.com/2024-marcum-patent-litigation-study (Accessed June 4, 2024).
35. Peixoto TP The graph-tool python library. Figshare (2014). Available at: https://graph-tool.skewed.de/static/doc/faq.html.
36. Kewalramani L. Patent wars and Frand licensing. IIPRD website (2019). Available from: https://www.iiprd.com/patent-wars-and-frand-licensing/ (Accessed June 4, 2024).
37. Jaffe AB. Characterizing the “technological position” of firms, with application to quantifying technological opportunity and research spillovers. Res Pol (1989) 18(2):87–97. doi:10.1016/0048-7333(89)90007-3
38. Argyres N, Silverman B. R&D, organization structure, and the development of corporate technological knowledge. Strateg Manag J (2004) 25(8 – 9):929–58. doi:10.1002/smj.387
39. Rosenkopf L, Almeida P. Overcoming local search through alliances and mobility. Manag Sci (2003) 49(6):751–66. doi:10.1287/mnsc.49.6.751.16026
40. Nair SS, Mathew M, Nag D. Dynamics between patent latent variables and patent price. Technovation (2011) 31(12):648–54. doi:10.1016/j.technovation.2011.07.002
41. Falk N, Train K. Patent valuation with forecasts of forward citations. J Bus Valuat Econ Loss Anal (2016) 12(1):101–21. doi:10.1515/jbvela-2016-0002
42. Suh JH. Exploring the effect of structural patent indicators in forward patent citation networks on patent price from firm market value. Technol Anal Strateg Manag (2015) 27(5):485–502. doi:10.1080/09537325.2015.1011613
43. Kito T, Moriya N, Yamanoi J. Inter-organisational patent opposition network: how companies form adversarial relationships. Jpn Econ Rev (2021) 72:145–66. doi:10.1007/s42973-020-00057-5
44. Grimaldi M, Cricelli L. Indexes of patent value: a systematic literature review and classification. Knowl Manag Res Pract (2020) 18(2):214–233. doi:10.1080/14778238.2019.1638737
45. Allison JR, Lemley MA, Schwartz DL. Our divided patent system. U Chi L Rev (2015) 82(3):1073–154.
Keywords: patent litigation, patent analysis, inter-firm network, triadic motifs, competitive dynamics, adversarial relationships, negative ties
Citation: Kito T, Murata Y, Yamanoi J and Madhavan R (2024) Inter-firm patent litigation networks: a study of network motif analysis. Front. Phys. 12:1331286. doi: 10.3389/fphy.2024.1331286
Received: 31 October 2023; Accepted: 24 May 2024;
Published: 24 June 2024.
Edited by:
Rossana Mastrandrea, IMT School for Advanced Studies Lucca, ItalyReviewed by:
Ted Sichelman, University of San Diego, United StatesDror Y. Kenett, Johns Hopkins University, United States
Copyright © 2024 Kito, Murata, Yamanoi and Madhavan. This is an open-access article distributed under the terms of the Creative Commons Attribution License (CC BY). The use, distribution or reproduction in other forums is permitted, provided the original author(s) and the copyright owner(s) are credited and that the original publication in this journal is cited, in accordance with accepted academic practice. No use, distribution or reproduction is permitted which does not comply with these terms.
*Correspondence: Tomomi Kito, kito@aoni.waseda.jp