- 1OncoRay—National Center for Radiation Research in Oncology, Faculty of Medicine and University Hospital Carl Gustav Carus, Technische Universität Dresden, Helmholtz-Zentrum Dresden—Rossendorf, Dresden, Germany
- 2Helmholtz-Zentrum Dresden—Rossendorf, Institute of Radiooncology—OncoRay, Dresden, Germany
- 3Zittau/Görlitz University of Applied Sciences, Faculty of Natural and Environmental Sciences, Zittau, Germany
- 4Department of Computer Science, Electrical Engineering and Mathematical Sciences, Western Norway University of Applied Sciences, Bergen, Norway
- 5German Cancer Consortium (DKTK), Partner Site Dresden, and German Cancer Research Center (DKFZ), Heidelberg, Germany
- 6Department of Radiotherapy and Radiation Oncology, Faculty of Medicine and University Hospital Carl Gustav Carus, Technische Universität Dresden, Dresden, Germany
Robust and fast in vivo treatment verification is expected to increase the clinical efficacy of proton therapy. The combined detection of prompt gamma rays and neutrons has recently been proposed for this purpose and shown to increase the monitoring accuracy. However, the potential of this technique is not fully exploited yet since the proton range reconstruction relies only on a simple landmark of the particle production distributions. Here, we apply machine learning based feature selection and multivariate modelling to improve the range reconstruction accuracy of the system in an exemplary lung cancer case in silico. We show that the mean reconstruction error of this technique is reduced by 30%–50% to a root mean squared error per spot of 0.4, 1.0, and 1.9 mm for pencil beam scanning spot intensities of 108, 107, and 106 initial protons, respectively. The best model performance is reached when combining distribution features of both gamma rays and neutrons. This confirms the advantage of hybrid gamma/neutron imaging over a single-particle approach in the presented setup and increases the potential of this system to be applied clinically for proton therapy treatment verification.
1 Introduction
Proton therapy is an emerging technology in the context of cancer radiotherapy that features an advantageous dose profile due to the so-called Bragg peak, a steep dose maximum at the end of the proton range whose position can be tailored to the tumour volume. Due to the dependence of the proton interaction cross sections on the traversed material, this proton range can only be accurately predicted during treatment planning if the exact patient anatomy during irradiation is known over the whole treatment course. In most cases, this knowledge is incomplete, since the patient anatomy may vary, e.g., due to tumour shrinkage, weight loss, digestion and breathing, because the patient setup may not be exactly reproducible, and due to limitations in inferring the material properties from the patient computed tomography (CT) images [1]. This induces uncertainties in the treatment planning, which can lead to sub-optimal tumour control and adverse side effects in healthy organs [2].
To counteract this, several strategies are being pursued, including repeated (cone-beam) CT imaging, surface tracking, and the use of fiducial markers. The advantage of secondary-radiation monitoring over these approaches is that it promises direct, in vivo feedback on the applied proton range in the patient without prolonging the treatment workflow and without inflicting additional radiation dose or surgical intervention on the patient [3]. First clinical prototypes measuring the distribution of prompt gamma rays emitted in the patient to monitor the correct treatment application are currently already undergoing clinical studies [4, 5]. Beyond this, it has recently been shown that the detection of fast neutrons in addition to gamma rays can provide complementary information and increase the monitoring accuracy by about 1 mm. This approach is referred to as NOVO (NeutrOn and gamma-ray imaging for real-time range VerificatiOn and image guidance in particle therapy) and has been shown feasible in a proposed prototype simulation study (cf. Figure 1; [6]).
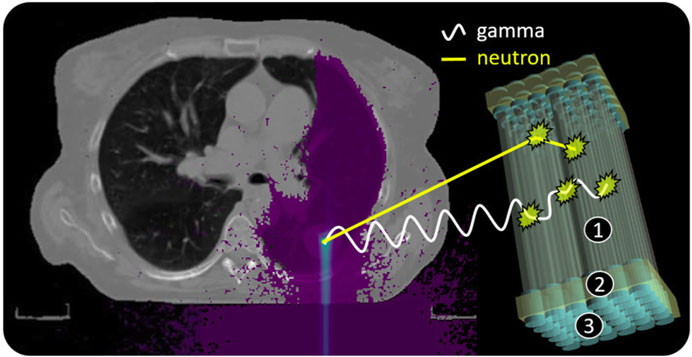
FIGURE 1. Schematic view of the simulation setup. Proton pencil beams (blue) were directed at a lung tumour in a patient CT. Emitted gamma rays (white) and neutrons (yellow) were scored in the detector, consisting of scintillators (1), a support structure (2) and photodetectors (3). Their distribution of origin was then reconstructed from scatter kinematics used to construct cones that describe possible incidence directions of gamma rays and neutrons, and back projection of these cones. Figure adapted from [6], licensed under CC BY 4.0
However, the minimum detectable range deviation with this hybrid multi-particle approach is still higher than 5 mm for clinical low-intensity spots [6]. One of the main fields of improvement here appears to be the range reconstruction method, which is currently based only on the arithmetic mean of the spatial neutron and gamma-ray emission distributions. Yet, anatomical deviations lead to changes in the emission distribution not covered by the arithmetic mean, such as broadenings and intensity changes (cf. Figure 2).
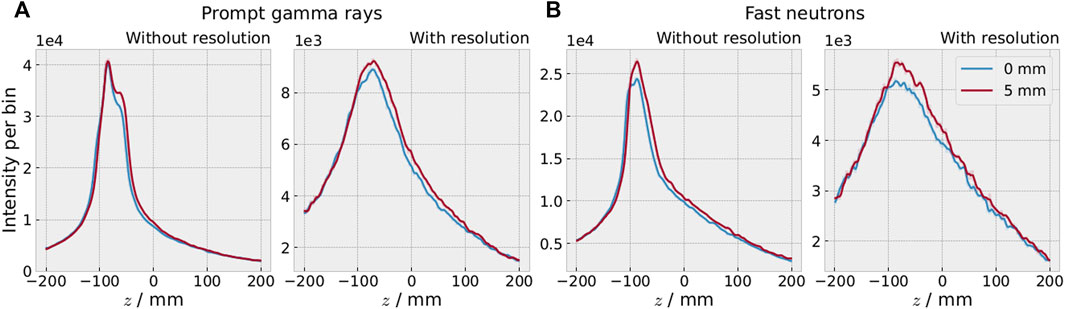
FIGURE 2. Input gamma-ray (A) and neutron (B) emission distributions along the beam axis z, simulated without and with incorporating a realistic detector resolution, for 109 initial protons. A 5 mm range shift (red) leads to a shape change of the distribution, including a broadening and intensity increase. Distributions with incorporated detector resolution were used for this study. The histogram bin width is 2 mm. Note that the displayed intensity range varies between the subfigures.
Therefore, the aim of this work is to apply an improved reconstruction method in order to increase the proton range reconstruction precision of the NOVO treatment verification method. The presented approach is based on a standardised distribution feature assortment, out of which strongly predictive parameters are selected by machine learning and combined to multivariate regression models. The method is introduced in the following section, validated and compared against the current method in Section 3, and the results and implications are conclusively discussed in Section 4.
2 Material and methods
2.1 Setup and secondary particle production distributions
Simulated secondary particle distributions described in detail by Meric et al. [6] were used as input data for this study. The setup and distribution reconstruction are shortly summarized in the following.
The simulations were performed using the toolkit GATE v. 8.2 [7] and a publically available lung cancer patient CT [8] (see Figure 1). The introduced hybrid neutron/gamma detector was a scintillator allowing for position-resolved time and energy determination and gamma/neutron discrimination by pulse-shape discrimination. The detector is referred to as NOVCoDA (NOVO Compact Detector Array), has a volume of 30 cm3 × 20 cm3 × 20 cm3, and consists of optically segmented organic glass bars with dual-ended silicon photomultiplier light readout. A pencil beam scanning (PBS) treatment plan spot was simulated by a posterior proton beam of 85 MeV with a width of 4.7 mm (FWHM) and 2.5 mrad divergence directed centrally at the tumor.
Secondary particles generated in the patient were tracked and the phase-space information of those interacting with the detector volume were scored. Double elastic scatter events on hydrogen-1 nuclei were evaluated for neutrons and triple scatter events were evaluated for prompt gamma-rays. Background due to wrong event sequences was included, whereas random coincidences were not modelled. The applied energy cuts were 100 keV for incident neutrons and 10 keV for incident prompt gamma-rays. The time resolution, position resolution, energy resolution and segmentation effects of a realistic detector were accounted for by smearing out the phase-space distributions accordingly prior to reconstruction (see [6]; Figure 2). Event cones were then kinematically reconstructed from the time-of-flight between detector elements (only for incident neutrons), the deposited energy and the positions of interaction. Finally, the distribution of the particle origin in the patient was estimated by a simple back projection algorithm and projected onto the beam axis.
In order to evaluate the ability of the system to quantify range deviations, this process was repeated 21 times with range-shifted versions of the patient [6]. For this, range deviations of up to ±5 mm were introduced in steps of 0.5 mm in the CT by adding and removing surface tissue, respectively. For each case, the simulation was performed with 109 initial protons. Realistic spot intensities (106 to 108 protons per spot) were achieved by sub-sampling [9] of the projected distributions (see Figure 3).
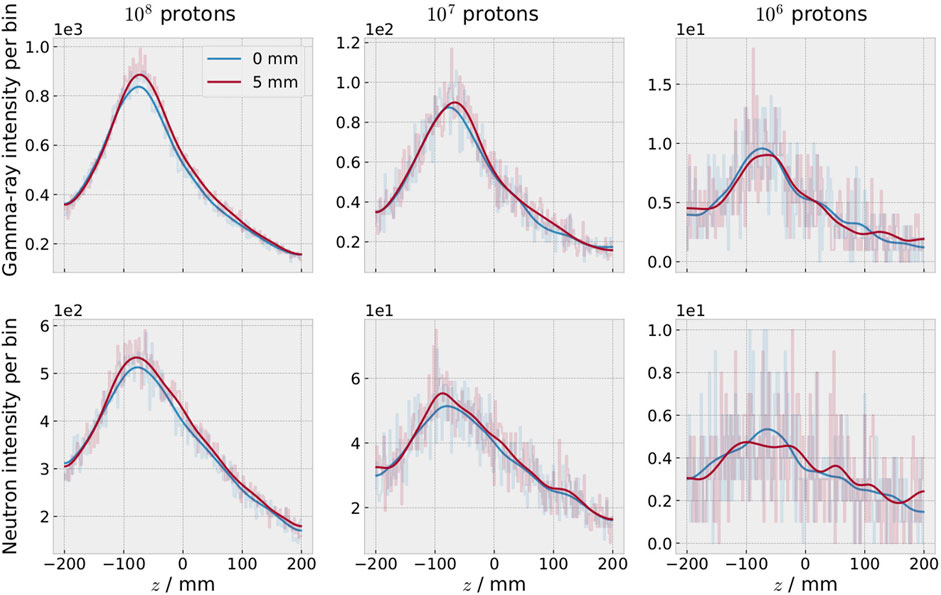
FIGURE 3. Resampled gamma-ray (top) and neutron (bottom) emission distributions along the beam axis z for clinically realistic spot-wise initial proton numbers between 106 (right) and 108 (left), with (red) and without (blue) a 5 mm range shift. For better visibility, the data was smoothed with a Gaussian filter (σ = 8 bins) for this graph. The unsmoothed data was used for analysis and is underlaid semi-transparently. The histogram bin width is 2 mm. Low-intensity spots (with 106 to 107 protons) are most common in realistic clinical treatment plans, but exhibit the lowest signal-to-noise ratio. Note that the displayed intensity range varies between the subfigures.
2.2 Range reconstruction by multivariate modelling
To estimate the range deviation ΔR from the projected distributions p(z), previous work [6] used the arithmetic mean of the distribution as a range landmark RL = ∑(p(z) ⋅ z)/∑p(z) and considered the difference between the RL of two distributions to be approximately equal to the introduced range deviation between those two (ΔR ≈ ΔRL). The performance of this RL was compared for each particle species individually and for the summed distribution of both particle species.
In this work, we aimed to replace this simplistic range deviation estimation by a multiple linear regression model in order to improve the estimation accuracy. As apparent from Figures 2, 3, the introduction of range deviations does not only shift the arithmetic mean (RL) of the distribution, but leads to changes in the distribution shape. Additionally, the distributions are subject to statistical noise, especially for low spot intensities (see Figure 3), which may affect the arithmetic mean more strongly than more robust measures such as distribution percentiles. Therefore, a set of 28 distribution parameters was tested in this work to estimate the range deviation more precisely, including higher statistical momenta, robust histogram features, percentiles, interquantile ranges, and the integral number of counts.
The method is described in detail in [10]. First, relevant distribution features were calculated from both the fast neutron and the prompt gamma-ray distributions based on the Image Biomarker Standardisation Initiative parameter set [11]. This parameter set contained measures of the distribution width, such as the standard deviation, interquartile ranges, coefficients of variation and mean absolute deviation, measures of position, such as the arithmetic mean and percentiles, and further parameters such as the area under the curve. The most relevant parameters were then selected by forward feature selection and the Least Absolute Shrinkage and Selection Operator (LASSO) [12]. In short, the forward selection method iteratively adds parameters based on the p-value of linear regression, while the LASSO method reduces the number of parameters by an additional penalty term in the cost function. The feature selection was performed once for each particle species individually and once for a merged parameter set containing the parameters of both particle distributions. Based on this, multivariate linear models were generated using the most predictive features as regressors to predict the applied range deviation from the reconstructed distributions.
For each investigated spot intensity, the models were trained on 40 individually re-sampled projected distributions and validated on further 20 individually re-sampled projected distributions. The root mean squared error (RMSE) between estimated and actual range deviation was used as a measure to evaluate the model performance in comparison to the previously used method based solely on RL.
3 Results
3.1 Predictive distribution parameters
The two studied feature selection methods (LASSO and forward selection) showed a high agreement in the selected parameters and attainable RMSE. The selected parameters are discussed in the following.
For neutrons, the distribution parameter “area under the curve,” i.e., the integral number of detected events per spot, was identified as the single most predictive feature by both selection algorithms for all spot intensities. Here, the model performance did not further increase when adding more parameters to the model.
For gamma rays, the area under the curve was also most often chosen by the feature selection. Here, a clear improvement of accuracy was observed for multivariate models over univariate ones. The forward selection algorithm took less parameters to converge to the final accuracy than LASSO. It converged at three to four parameters per model, without further relevant improvement when adding more parameters. This result is consistent with previous results for the prompt gamma-ray timing system [10]. Next to the area under the curve, the median and the mean absolute deviation of the distributions were chosen as predictive features.
The hybrid models combining information of the neutron and gamma-ray distributions all relied on the area under the curve of the neutron distribution as most predictive parameter, independent of the spot intensity and feature selection algorithm. The area under the curve of the gamma-ray distribution was identified as the second most important feature, confirming that the combined information of neutrons and gamma rays is advantageous. Further often-selected parameters were the median and the coefficient of variation of the prompt gamma-ray distributions. The forward-selected models converged with slightly less parameters than LASSO, again reaching their optimal reconstruction accuracy at three to four parameters.
3.2 Model performance and comparison
The distribution features selected by the presented method clearly improved the range reconstruction accuracy, as compared to the previously used RL (see Figure 4). For medium-intensity spots (107 initial protons), the RMSE decreased from 2.8to 1.3 mm, from 1.9 to 1.6 mm, and from 2.0 to 1.3 mm, for neutrons, gamma rays, and hybrid models, respectively, when taking into account only the feature identified as most predictive. When combining the three most predictive features, the RMSE further decreased to 1.2 mm for gamma rays only and 1.0 mm for hybrid models, and stayed at 1.3 mm for neutrons only. This corresponds to a decrease by 37%–54% relative to the previously used method for all distributions.
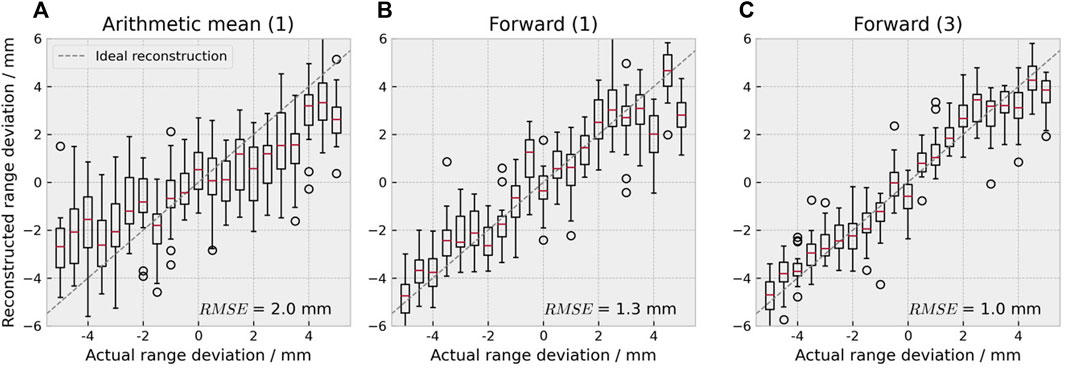
FIGURE 4. Boxplot of reconstructed and actual range deviation on the validation dataset for 107 protons per spot using the combined data of both neutrons and gamma rays. The presented models are univariate models based on the previously used range landmark [arithmetic mean of the sum distribution [6]; (A)], the distribution feature identified by forward selection as the most predictive [neutron count, (B)], and a multivariate model based on the three most predictive features [neutron count, gamma median, gamma count; (C)]. The number of parameters used per model is given in parenthesis in the subfigure title. The new method clearly improved the range reconstruction accuracy.
For all studied spot intensities (106 to 108 initial protons), the uncertainty of the range reconstruction RMSE was reduced by 30%–50% by the new method (see Figure 5). For the lowest (106) and highest (108) intensity spots, an RMSE of 1.9 and 0.4 mm was reached, respectively.
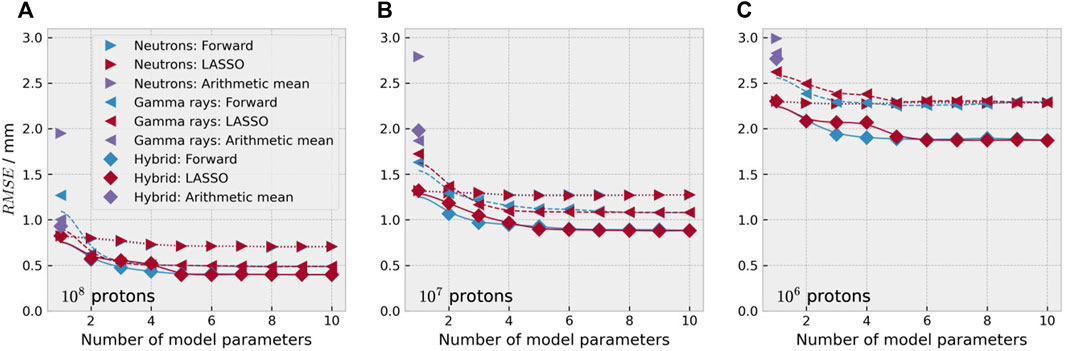
FIGURE 5. RMSE between estimated and actual range deviation for 108 (A) 107 (B) and 106 (C) protons per spot, as a function of the number of parameters included in the model. The previously used range landmark (purple) is outperformed by the new method (blue, red) for all spot intensities. Results are displayed for neutron data only (right arrow marker), gamma-ray data only (left arrow marker) and combined data of both particles (diamond marker). The discrete data points of the models are underlayed with a smoothed line for improved visibility (neutrons—dotted, gamma rays—dashed, combined models—solid).
For low and medium-intensity spots, the multivariate models using the neutron signal alone were the least predictive of the range deviation, which confirms previous work [6]. The multivariate models based on the gamma-ray signal alone performed better, possibly due to a higher number of collected events per spot. The highest accuracy could be reached by hybrid models combining features of the gamma-ray and neutron distributions, which underlines the advantage of a detector system capable to evaluate both particle types.
4 Discussion
The aim of this study was to improve the range reconstruction accuracy of the hybrid neutron/gamma-ray treatment verification method NOVO by use of multivariate modelling. This aim was achieved by applying forward and LASSO variable selection on a standardised histogram feature assortment and successive multivariate regression. By combining distribution features of both particle species, the newly developed models decreased the mean prediction error by 30%–50% to 0.4, 1.0, and 1.9 mm for pencil beam scanning spot intensities of 108, 107, and 106 protons per spot, respectively (without spot accumulation).
It was seen that independent of the spot intensity, the best range estimation accuracy was reached when combining the neutron and gamma-ray data. This confirms the advantage of hybrid gamma-ray/neutron imaging over a single-particle approach in the presented setup. Using this hybrid model, about three distribution parameters were sufficient for an accurate estimate of the range deviation on the validation dataset, which is in agreement with previous findings for gamma rays [10] and suggests that the model complexity is not too high, i.e., that overfitting and a high model variance have been avoided. The good agreement in the parameters selected by the two tested methods (LASSO and forward selection) indicates that the found parameters are likely independent of the selection method and therefore reliably predictive.
When considering only one particle type, the model accuracy was degraded, but still better from gamma ray-only than for neutron-only data. The reasons for the neutron data being less predictive may be three-fold: (a) the neutron yield is lower than the gamma-ray yield at the considered proton energy of 85 MeV [13], (b) the angular neutron emission distribution is forward-peaked whereas the detector was placed at a lateral orientation to the beam, and (c) the back projection reconstruction is more difficult for lower energy neutrons that are more likely to scatter in the patient before reaching the detector [6]. All of these factors lead to a lower signal-to-noise ratio in the neutron distributions than in the gamma-ray distributions, as evident in Figure 3. It is possible that for higher proton beam energies, the neutron signal may become more predictive, since the neutron yield increases more steeply with increasing beam energy than the gamma-ray yield and dominates at proton beam energies above 130 MeV [13]. The lower signal-to-noise ratio in the neutron distributions may also be the reason why other parameters of these distributions than the area under the curve were not found to be predictive of the proton range, especially the previously used arithmetic mean.
The modelling method used in this work has previously been shown capable of improving the range estimation accuracy of data acquired with the prompt gamma-ray timing system with a similar number of parameters per model [10]. In this work, it was found that the method is directly transferable to the NOVO system without the need for system-specific adjustments, making it a possible method of interest also for other treatment verification systems.
In this study, we focussed on establishing the method on a single patient dataset and beam energy and angle. This enables future studies to investigate more generalised models covering different patient anatomies and beam parameters to advance the clinical translation of the NOVO system. It appears likely that these models will consist of different distribution parameters, depending on the proton beam energy range and the tumour entity. Following these simulation studies, the next necessary step will be extending these investigations to in vivo measured experimental data. First experiments to facilitate this with a NOVCODA prototype are currently under preparation.
The two most advanced alternative range verification systems, prompt gamma-ray imaging and spectroscopy, have been reported to currently reach a spot-wise range shift detection accuracy of about 2 mm [4, 14] for high-intensity spots. The accuracy of the NOVO system was reduced to below 1 mm in this work, which is a promising result. However, one has to note that the accuracy of these two systems has been reported from clinical experimental data, whereas this study relied on simulation data only. Limitations of the simulation include the exclusion of random coincidences and detector effects such as gain instabilities and possible limitations in the detector throughput. Therefore, experimental data with the NOVCoDA system is necessary to enable a direct comparison and to present conclusive evidence for its suitability as a clinically viable range monitoring system.
In conclusion, this study confirms that elaborate statistical modelling is a valuable tool to enhance different types of particle treatment verification systems and increases the potential of hybrid neutron/gamma imaging for clinical application.
Data availability statement
The data analyzed in this study is subject to the following licenses/restrictions: The original contributions presented in the study are included in the article, further inquiries can be directed to the corresponding author. Requests to access these datasets should be directed to SS, sonja.schellhammer@hszg.de.
Author contributions
SS: Conceptualization, Data curation, Formal Analysis, Investigation, Methodology, Project administration, Software, Validation, Visualization, Writing–original draft, Writing–review and editing. IM: Conceptualization, Data curation, Funding acquisition, Project administration, Writing–review and editing. SL: Methodology, Validation, Writing–review and editing. TK: Conceptualization, Project administration, Resources, Writing–review and editing.
Funding
The authors declare financial support was received for the research, authorship, and/or publication of this article. The authors acknowledge financial support by the Research Council of Norway under the NANO2021 program (Grant no. 301459).
Conflict of interest
The authors declare that the research was conducted in the absence of any commercial or financial relationships that could be construed as a potential conflict of interest.
Publisher’s note
All claims expressed in this article are solely those of the authors and do not necessarily represent those of their affiliated organizations, or those of the publisher, the editors and the reviewers. Any product that may be evaluated in this article, or claim that may be made by its manufacturer, is not guaranteed or endorsed by the publisher.
References
1. Lomax AJ. Intensity modulated proton therapy and its sensitivity to treatment uncertainties 2: the potential effects of inter-fraction and inter-field motions. Phys Med Biol (2008) 53:1043–56. doi:10.1088/0031-9155/53/4/015
2. Engelsman M, Bert C. Precision and uncertainties in proton therapy for moving targets. In: H Paganetti, editor. Proton therapy physics. United, States: CRC Press, Taylor & Francis Group (2011). doi:10.1201/b11448-15
3. Pausch G, Müller C, Berthold J, Enghardt W, Küchler M, Römer KE, et al. Effect of strong load variations on gain and timing of CeBr3 scintillation detectors used for range monitoring in proton radiotherapy. In: Paper presented at the IEEE Nuclear Science Symposium and Medical Imaging Conference; November 10-17, 2018; Sydney, Australia (2018).
4. Hueso-González F, Rabe M, Ruggieri TA, Bortfeld T, Verburg JM. A full-scale clinical prototype for proton range verification using prompt gamma-ray spectroscopy. Phys Med Biol (2018) 63:185019. doi:10.1088/1361-6560/aad513
5. Berthold J, Khamfongkhruea C, Petzoldt J, Thiele J, Hölscher T, Wohlfahrt P, et al. First-in-human validation of CT-based proton range prediction using prompt gamma imaging in prostate cancer treatments. Int J Radiat Oncology*Biology*Physics (2021) 111:1033–43. doi:10.1016/j.ijrobp.2021.06.036
6. Meric I, Alagoz E, Hysing L, Kögler T, Lathouwers D, Lionheart W, et al. A hybrid multi-particle approach to range assessment-based treatment verification in particle therapy. Sci Rep (2023) 13:6709. doi:10.1038/s41598-023-33777-w
7. Jan S, Benoit D, Becheva E, Carlier T, Cassol F, Descourt P, et al. GATE V6: a major enhancement of the GATE simulation platform enabling modelling of CT and radiotherapy. Phys Med Biol (2011) 56:881–901. doi:10.1088/0031-9155/56/4/001
8. Jinzhong Y, Greg S, Harini V, Wouter VE, Andre D, Tim L, et al. Data from Lung CT segmentation challenge (2017).
9. Efron B. Bootstrap methods: another look at the jackknife. Ann Stat (1979) 7:1–26. doi:10.1214/aos/1176344552
10. Schellhammer SM, Wiedkamp J, Löck S, Kögler T. Multivariate statistical modelling to improve particle treatment verification: implications for prompt gamma-ray timing. Front Phys (2023) 10. doi:10.3389/fphy.2023.1295157
11. Zwanenburg A, Leger S, Vallieres M, Löck S. Image biomarker standardisation initiative reference manual (2019). arXiv. doi:10.48550/arXiv.1612.07003
12. Kuhn M, Johnson K. Applied predictive modeling. New York: Springer (2013). doi:10.1007/978-1-4614-6849-3Applied predictive modeling
13. Smeets J, Roellinghoff F, Prieels D, Stichelbaut F, Benilov A, Busca P, et al. Prompt gamma imaging with a slit camera for real-time range control in proton therapy. Phys Med Biol (2012) 57:3371–405. doi:10.1088/0031-9155/57/11/3371
Keywords: proton therapy, treatment verification, prompt gamma ray, fast neutron, machine learning, multivariate modelling
Citation: Schellhammer SM, Meric I, Löck S and Kögler T (2023) Hybrid treatment verification based on prompt gamma rays and fast neutrons: multivariate modelling for proton range determination. Front. Phys. 11:1295157. doi: 10.3389/fphy.2023.1295157
Received: 15 September 2023; Accepted: 16 November 2023;
Published: 12 December 2023.
Edited by:
Aleksandra Wrońska, Jagiellonian University, PolandCopyright © 2023 Schellhammer, Meric, Löck and Kögler. This is an open-access article distributed under the terms of the Creative Commons Attribution License (CC BY). The use, distribution or reproduction in other forums is permitted, provided the original author(s) and the copyright owner(s) are credited and that the original publication in this journal is cited, in accordance with accepted academic practice. No use, distribution or reproduction is permitted which does not comply with these terms.
*Correspondence: Sonja M. Schellhammer, sonja.schellhammer@hszg.de; Toni Kögler, toni.koegler@oncoray.de