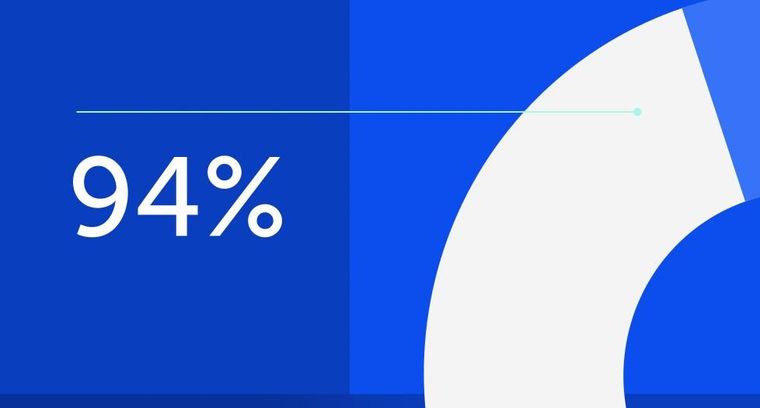
94% of researchers rate our articles as excellent or good
Learn more about the work of our research integrity team to safeguard the quality of each article we publish.
Find out more
EDITORIAL article
Front. Phys., 13 September 2023
Sec. Physical Acoustics and Ultrasonics
Volume 11 - 2023 | https://doi.org/10.3389/fphy.2023.1287956
This article is part of the Research TopicAdvances in Noise Reduction and Feature Extraction of Acoustic SignalView all 13 articles
Editorial on the Research Topic
Advances in noise reduction and feature extraction of acoustic signal
It is our pleasure to introduce the Research Topic on advances in noise reduction and feature extraction of acoustic signals in Frontiers in physics. Acoustic signal processing and its analysis is one of the hot topics of research in physics and has been studied by many engineers and scientists in various real-world fields, including underwater acoustics, architectural acoustics, engineering acoustics, physical acoustics, environmental acoustics, psychological acoustics, and so on. Noise reduction is the foundation of acoustic signal pre-processing in order to extract useful features from the acoustic signal, which is the linchpin for pattern recognition, target detection, tracking, and localization.
The real-world acoustic signals are usually non-linear and accompanied by intense background noise, and features extracted directly from these signals generally contain a large volume of useless as well as noisy information leading to ambiguous results. Therefore, the study of noise reduction methods of acoustic signals is the first step to effectively utilize this signal, including but not restricted to wavelet analysis, integrated empirical mode decomposition, variational mode decomposition, and consequential improvements. In addition, whether the extracted features contain sufficient useable information also determines the performance of the results of acoustic signal research. For weak signals present in many application areas, it is extremely difficult to precisely describe certain physical meanings of the signal utilizing specified features. In recent years, some researchers have used entropy to characterize the dynamics of the signal, but there are also issues such as missing scale and distance information as well as fractional order differential information. In a word, it is urgent to put forward more advanced features to solve the problem of missing information.
This Research Topic welcomed the research and review articles on advanced acoustic signal noise reduction and feature extraction in various fields. This Research Topic brought together a Research Topic of articles that addresses these challenges and/or showcase the latest real-world applications and enabling algorithmic advances in intelligent control and optimisation, as they pertain to system identification, intelligent control, and optimisation of dynamical systems.
The call for papers was launched in September 2022 and closed in April 2023. In total, 12 papers were finally selected for inclusion in the Research Topic.
Yi and Tian have utilised the butterfly optimization algorithm (BOA) to optimize the parameters of the variational mode decomposition. The proposed method BOA-VMD has also been incorporated with novel slope entropy for extracting the features from ship-radiated noise (SNR).
Zhufeng et al. presented advances in the mechanism of underwater target radiation noise generation and analyzed the research progress. Further, they applied machine learning in underwater target radiation applications from three perspectives: signal acquisition, feature extraction, and signal recognition. The authors elaborated the challenges of underwater target-radiated noise recognition technology against the backdrop of rapid computing science development.
Huang and Liu proposed a new conjugate gradient method for noise reduction in signal processing and image restoration. The superiority of this method lies in its employment of the ideas of accelerated conjugate gradient methods in conjunction with a new adaptive method for choosing the step size. The authors have demonstrated the effectiveness and superiority of the proposed method through numerical simulation.
Zhao et al. have used four types of multi-scale entropies, including multi-scale sample entropy (MSE), multi-scale fuzzy entropy (MFE), multi-scale permutation entropy (MPE), and multi-scale dispersion entropy (MDE) to extract more effective information from underwater acoustic signals.
Liang et al. introduced enhanced cross-spectrum processing to improve the computational efficiency of time-frequency analysis (TFA) for passive source localization and train-bearing fault diagnosis. Through results, it has been shown that an improvement up to 85% can be achieved without a noticeable impact on the accuracy of parameter estimates.
Qu et al. have shown the effect of parameter mismatch on source localization in cases involving environments and seabed uncertainties. To address these issues, the authors have developed the simplified seabed model for focalization using two geoacoustic parameters viz., the amplitude F and phase of reflection
Li et al. proposed a method based on a single hydrophone that can jointly identify the mode order and estimate the propagation range in an unknown marine environment. The method uses Bayesian theory as the main methodology and applied to broadband pulse sound sources in shallow seas with long-range propagation. The dispersion curves extracted from the data and those calculated by the dispersion formula are the input signal and the replica of the methods, respectively. Accurate identification of the normal mode order and estimation of the propagation range can be achieved by establishing the joint cost function.
Zhang et al. proposed the robust underwater multi-target direction-of-arrival (DOA) tracking method to address the issues of typical multi-target problems under unknown underwater environments with missing detection, false alarms, and uncertain measurement noise.
Ji presented an exhaustive review of the research progress of dispersion entropy (DE) in the feature extraction of ship radiated noise (SRN). He first described the DE and its improved algorithms. Then the traditional and DE-based SRN feature extraction methods are summarized, and the application of DE in SRN feature extraction methods is concluded from two aspects: methods that apply DE algorithms only and methods that combine DE with mode decomposition algorithms.
Jiang et al. suggested that most researchers have ignored the relationship between one entropy with another. Inspired by this point, they proposed the synergistic relationship between bubble entropy (BE) and permutation entropy (PE). Through experimentation, it has been demonstrated that the synergistic complementarity between BE and PE increases the recognition rate of sea state signals by 10.5% and the recognition rate of bearing signals reaches 99.5%.
Zhou and Wang proposed the triple feature extraction and classification method based on multi-scale dispersion entropy (MDE) and multi-scale permutation entropy (MPE) to extract the features from the rolling bearing and used them in K nearest neighbour classification algorithm to determine whether there is a fault in the bearing and the type of the fault.
Yu et al. proposed an ambient-noise-assisted multivariate empirical mode decomposition (ANA- MEMD) method for adaptively suppressing noise in low signal-to-noise (S/N) microseismic data encountered during data processing.
GV: Writing–original draft, Writing–review and editing. RK: Methodology, Supervision, Visualization, Writing–original draft, Writing–review and editing.
We hope that this Research Topic will inspire readers to explore the possibilities in noise reduction and feature extraction of acoustic signals and to contribute to the ongoing efforts to create a more sustainable and equitable future. We would like to thank all the authors and reviewers who have contributed to the Research Topic. We would also like to express our gratitude to the Editor-in-Chief Prof. Alex Hansen and the Frontiers editorial team. Without their support, this Research Topic would not have been successful.
The authors declare that they have no known competing financial interests or personal relationships that could have appeared to influence the work reported in this paper.
All claims expressed in this article are solely those of the authors and do not necessarily represent those of their affiliated organizations, or those of the publisher, the editors and the reviewers. Any product that may be evaluated in this article, or claim that may be made by its manufacturer, is not guaranteed or endorsed by the publisher.
Keywords: acoustic signal, entropy, wavelet analysis, emprical mode decomposition, variational mode decomoposition (VMD)
Citation: Vashishtha G and Kumar R (2023) Editorial: Advances in noise reduction and feature extraction of acoustic signal. Front. Phys. 11:1287956. doi: 10.3389/fphy.2023.1287956
Received: 03 September 2023; Accepted: 06 September 2023;
Published: 13 September 2023.
Edited and reviewed by:
Michele Meo, University of Southampton, United KingdomCopyright © 2023 Vashishtha and Kumar. This is an open-access article distributed under the terms of the Creative Commons Attribution License (CC BY). The use, distribution or reproduction in other forums is permitted, provided the original author(s) and the copyright owner(s) are credited and that the original publication in this journal is cited, in accordance with accepted academic practice. No use, distribution or reproduction is permitted which does not comply with these terms.
*Correspondence: Govind Vashishtha, Z292aW5keXVkaXZhc2hpc2h0aGFAZ21haWwuY29t
†ORCID: Govind Vashishtha, orcid.org/0000-0002-5160-9647
Disclaimer: All claims expressed in this article are solely those of the authors and do not necessarily represent those of their affiliated organizations, or those of the publisher, the editors and the reviewers. Any product that may be evaluated in this article or claim that may be made by its manufacturer is not guaranteed or endorsed by the publisher.
Research integrity at Frontiers
Learn more about the work of our research integrity team to safeguard the quality of each article we publish.