- 1Business School, University of Shanghai for Science and Technology, Shanghai, China
- 2Department of Printing and Packaging Engineering, Shanghai Publishing and Printing College, Shanghai, China
- 3Library, University of Shanghai for Science and Technology, Shanghai, China
- 4Shanghai Center for Research and Development of Cyberculture in Education (SCRDCE), Shanghai, China
Nowadays, Study of comments in MicroBlog online public opinion is of great significance for relevant departments in managing public opinion, due to the increasing influence of online public opinion on the Internet. This paper presents a method for studying the evolutionary characteristics of netizens’ comment focus in university online public opinion. This method is based on a three-stage framework called Topic-Temporal-Focus. Firstly, in the topic mining stage, the KTF-BTM model is proposed for topic recognition, which effectively improves the quality of analysis. Secondly, in the temporal segmentation stage, time periods are divided into 4-hour intervals, and the identified topics are paired with each comment text to generate a topic-temporal list. Finally, in the focus recognition stage, the content and evolution patterns of netizens’ comment focus within shorter time sequences are explored by analyzing the data characteristics of the topic-temporal list. Experimental results show that the proposed KTF-BTM model significantly enhances topic recognition quality for short texts. The Topic-Temporal-Focus framework overcomes the challenge of sparse comment text data within shorter time periods and effectively classifies topic evolution within limited time sequences. This research work serves as a valuable contribution towards understanding the evolutionary characteristics of netizens’ focal points in university online public opinion.
1 Introduction
Microblog hot search is an important indicator that reflects public attention and event popularity. However, in shorter time periods, the text data often becomes sparse due to the limitation of Microblog comment volume. Efficiently extracting valuable information from a large amount of high-dimensional, low-quality, and unlabeled unstructured data has become an important goal in current data mining research.
Probabilistic topic modeling is one of the crucial methods for addressing the aforementioned problem, and the Latent Dirichlet Allocation (LDA) model and its variants are among the most widely used probabilistic topic models. The LDA model, first proposed by Blei et al. in 2003 [1], categorizes topics by computing the topic-word and topic-document distribution probabilities of a corpus. It is suitable for analyzing long texts such as news and scientific literature. However, when applied to short-text analysis of online comments, this model treats each comment sentence as a sampling object and performs topic modeling through word co-occurrence. Due to the limited number of keywords in a single comment text, the issue of sparse feature words arises.
In 2014, Jianhua Yin et al. proposed the Dirichlet Multinomial Mixture Model (DMM), known as the GSDMM model [2]. The biggest difference between GSDMM and LDA lies in the assumption that each short text contains at most one topic, instead of multiple topics. Additionally, all words within a document share the same topic. This effectively alleviates the impact of sparse text features on modeling. Mazarura et al., by measuring the topic coherence and stability of the model, discovered that the performance of the GSDMM algorithm is superior to that of the LDA model in handling short texts [3].
Xiaohui Yan et al. introduced the Biterm Topic Model (BTM), a probabilistic topic model [4]. Unlike the LDA and DMM models, the BTM model considers all comment texts as a whole and models the entire corpus by extracting biterm word pairs. It calculates the topic distribution by analyzing the probability of two words belonging to the same topic in a biterm pair, which is determined by their co-occurrence frequency. This model extends the original single-word assumption in the LDA model to a word pair, thus partially alleviating the issue of sparse features in short texts. However, not all co-occurring word pairs demonstrate a strong topic relationship.
Traditional BTM models are based on the assumption of a “binomial distribution” between different words in the same document to construct the topic model. Under this assumption, each word is considered equally important. However, in such cases, irrelevant words and noise may have a negative impact on the model and lead to less accurate topic identification. Additionally, when faced with sparse text data in shorter time periods, BTM models may suffer from overfitting or underfitting issues, affecting the accuracy and reliability of topic mining.
Therefore, this article first proposes the KTF-BTM model, which combines the traditional BTM model with the TF-IDF algorithm weighted by part-of-speech, in order to improve the effectiveness of topic recognition. Based on this, a Topic-Temporal-Focus framework is constructed. In the topic extraction stage, the KTF-BTM model is utilized to enhance the accuracy of topic recognition. In the temporal segmentation stage, the identified topics are matched with the corresponding comment text to obtain a topic-temporal list. Finally, statistical analysis is conducted on the topic-temporal list to explore the evolving characteristics of comment focus, addressing the issue of data sparsity within a short time period.
Netizens possess independent thinking abilities. When participating in public opinion discussions, they selectively absorb and disseminate partial information about events based on personal stances, life experiences, interests, values, and other factors. Through the mechanism of “collaborative filtering,” the amplification effect highlights and magnifies this partial information, leading to the convergence of public opinion on certain viewpoints. Public opinion includes public sentiment information. Nowadays, when relevant authorities handle and respond to public opinion events, they need to consider the public sentiment information within the online public opinion sphere. They should communicate the handling results and address public concerns through platforms like MicroBlog. Changes in public opinion viewpoints have a certain influence on the direction of events. If the handling and response to public opinion diverge significantly from the expectations of netizens, it may potentially trigger secondary public opinion events.
For example, in 2021, an incident of clandestine filming in a university restroom gained attention on the MicroBlog Hot Search List, sparking extensive discussion. Dissatisfied with the punishment of “campus surveillance,” students at the university shifted their protests from online to offline, demanding the expulsion of the individuals involved. Compared to the restroom filming incident, incidents of sexual harassment by university teachers generate more topicality and impact for discussion. If the handling results greatly deviate from the expectations of netizens, it may incite online or even offline protests. Furthermore, if there are online mobilizations related to extended topics like the “Me Too” movement, it could potentially lead to more intense secondary public opinion events.
As more and more public opinion incidents in universities trend on MicroBlog, understanding the characteristics of comments in these incidents is crucial for public opinion management, managing related events, and imposing constraints. Within the same public opinion event, the comment focus will change over time. Comparing the differences in comment focus during different time periods helps identify newly emerged public opinion focuses, enabling timely grasp of key information and adjustment of response strategies.
The main contributions of this article are as follows.
1. Proposed the KTF-BTM model, which improves the effectiveness of identifying topics in short texts.
2. Proposed an extendable Topic-Temporal-Focus framework for monitoring the focus and evolution of public opinion in comments. Overcame the problem of sparse comment data within short time periods by dividing the comments into shorter intervals. Leveraged the KTF-BTM model to identify the focus of netizens and analyze the evolution of comment topics.
2 Related research
The evolution of online public opinion in universities refers to the dynamic development process of events that receive attention in the online space. It is the result of the mutual influence and interaction between netizens and their comments. The purpose of studying the evolution of online public opinion in universities is to understand the patterns of public opinion development, improve proactive and effective responses to online public opinion in universities, and enhance the efficiency of governing online public opinion in universities. Currently, there has been some progress in research on the evolution of online public opinion in universities, with many valuable research findings accumulated.
Some scholars have conducted research on the stage characteristics of online public opinion. Regarding the development stages of online public opinion in universities, different models have been proposed. Laihua Wang, Jinghong Xu, Yuexin Lan, and others propose a three-stage model: “generation, development, and decline.” Yi Liu, Jinsong Cao, Hui Tian, Fujian Fang, Gengyun Xie, Guodong Yuan, and others propose a four-stage model: “formation, diffusion, outbreak, and decline.” Mingyi Gu, Kefan Xie, and others propose a five-stage model: “dissemination, aggregation, sublimation, continuation, and termination.” Gang Li, Biao Li, and others propose a six-stage model: “latent, outbreak, spread, recurrence, relief, and long tail.” In general, despite different perspectives, the four-stage theory, five-stage theory, and six-stage theory can be considered as subdivisions and supplements to the three-stage theory. Based on previous research, they can be summarized into the following three stages:
Stage 1: Emergence phase - After a sudden incident in the university is reported or exposed, it attracts attention and discussion among netizens.
Stage 2: Diffusion phase - Attention and discussion continue to increase, and different opinions interact and merge, gradually forming and strengthening dominant views.
Stage 3: Decline phase - Attention and discussion gradually decrease, and public opinion gradually subsides.
Based on the above, some scholars have focused their research on the understanding of the evolution patterns and trend prediction of online public opinion. For example, researchers have deconstructed the evolution cycle of online public opinion in universities based on theoretical frameworks, and then used the E-Divisive algorithm to segment the evolving trends of public opinion. On this basis, they further predicted the evolving trends of online public opinion in universities [5]. Other studies have utilized LSTM models to predict the amount of information dissemination based on the rules of retweeting in campus Microblog public opinion [6]. Another study, by analyzing the polarization characteristics of Microblog user groups in campus public opinion events, has established quantitative indicators such as network density, team structure coefficient, betweenness centrality, closeness centrality, and contribution value to effectively analyze the current status and trends of Microblog user group polarization [7]. With regard to managing online public opinion, researchers have constructed a five-stage model for the evolution of self-media network public opinion and analyzed the laws and governance strategies for each stage of public opinion events [8]. Moreover, the use of social network analysis (SNA) has been explored to study individual node centrality through degree centrality, designing suitable guided algorithms for steering public opinion during the process of public opinion evolution [9]. The research mentioned above mainly explores the patterns of nodes.
Researchers are currently exploring more comprehensive research methods to investigate the changing patterns of public opinion and to uncover the developmental characteristics of events in online public discourse. For instance, studies have utilized the LDA model to identify topics within comments from online public opinion. These studies then analyze the temporal evolution of topic content and popularity during different stages of public opinion, including the latent period, outbreak period, and decline period [10]. Another approach involves dividing the public sentiment cycle into four stages, extracting topics for each stage using the LDA topic model, and examining the evolving trends of topics and sentiments across these stages [11]. Furthermore, researchers have also developed a public opinion analysis system [12].
Although research on the changing patterns of public opinion in online discourse is increasing, most existing work has primarily focused on topic identification and classification, overlooking the importance of data quality [13]. Short text modeling often encounters data quality issues such as data sparsity, high noise levels, and data imbalance [14–16]. The problem of data sparsity and high noise directly affects the accuracy of topic identification, posing a significant challenge. To address this issue, some studies have introduced dropout techniques to traditional probabilistic topic models, which randomly deactivate a portion of words to reduce the model’s reliance on specific words and improve its generalization ability [17].
One study proposed a novel hybrid model that combines the Dirichlet process and biterm representation. This model utilizes biterms to model word co-occurrence relationships in short texts and leverages the Dirichlet process to learn shared characteristics of topic distributions across the entire dataset. By doing so, it reduces the need for manual hyperparameter tuning, enhancing the model’s robustness and reliability [18]. Another study treats sentiment features as user-level information and addresses the issue of data sparsity in short text data by employing a topic modeling technique called “Biterm Topic Mixture Model.” The incorporation of user sentiment information improves the algorithm’s performance and enhances the accuracy of sentiment identification in short texts [19].
Furthermore, a study combines extended Latent Dirichlet Allocation (LDA) and Infinite Biterm Topic Model (IBTM) algorithms and employs a technique called “dynamic time windows” to handle streaming data. This approach enables effective processing of large-scale data [20]. Additionally, another study proposes a method called Social Media Data Cleaning Model (SMDCM) to address data quality issues in microblog data specifically related to effective short text topic modeling (STTM) [21].
We have noticed that an increasing number of scholars are focusing on addressing the issue of data sparsity in studying online public opinion, particularly emphasizing the ability of models to capture the changing patterns of public sentiment. Improved techniques have been developed to enhance the accuracy of long-term topic identification within time slices, such as three or four stages. However, due to the influence of data sparsity, there is limited research that divides the public opinion cycle into even smaller time slices to examine the changing characteristics of online discourse within shorter time periods.
As more and more university-related online public opinions trend on the MicroBlog hot search list, the “hot search crisis” in universities becomes increasingly severe. The focus of MicroBlog comments can undergo significant changes within a short period of time. Exploring the changing characteristics of comment topics within a short time cycle has become an urgent issue to address. Building upon the aforementioned research, if we can improve the accuracy of topic identification while shortening the time span for topic analysis, it would provide a good approach for monitoring online public opinion content within shorter monitoring periods. To address the low topic recognition performance of existing topic models, this paper proposes the KTF-BTM topic model to enhance topic identification. Generally, the duration of concentrated attention on university-related online public opinions on the MicroBlog hot search list is around 3–5 days. To solve the problem of monitoring public opinion content, this paper extracts online public opinion information in time periods, referring to the stage characteristics of university-related online public opinions and setting the information extraction time span to 4 h to monitor the dynamic changes in comment focus. Finally, from the perspective of system integration, a topic-temporal-focus framework is established to monitor the focus of public opinion comments and their evolution.
3 The KTF-BTM topic model with topic-temporal-focus framework
3.1 Proposed topic-temporal-focus framework
This section introduces the model framework for the evolution of comment focus in university-related online public opinion. We have constructed a Topic-Temporal-Focus framework that analyzes the focal topics of interest to netizens and their evolving patterns using Microblog data on university-related online public opinion. This framework can be used for monitoring Microblog data on university-related online public opinion and extracting general patterns and specific information, which will help analyze the focal points of netizens’ attention to relevant events and their feedback on governance strategies.
The framework consists of three stages, each with its own objectives, as shown in Figure 1. Stage 1 is the topic modeling process. In this stage, comment data is collected from Microblog, and the proposed KTF-BTM topic model is used for topic identification. Stage 2 categorizes the comments into 37 time intervals based on their posting times and assigns the identified topics to each comment text. In Stage 3, the extracted topics are merged into clusters of similar topics, and visualization tools are utilized to explore the content and changes in comment focus at each stage.
3.2 KTF-BTM topic model
The traditional BTM (Biterm Topic Model) assumes that every word in the text is important and can effectively explain the topics [4]. Additionally, it considers word pairs with higher frequencies as more important. However, this assumption leads to the BTM model excessively relying on high-frequency words, overlooking the significant role of medium and low-frequency words in topic interpretation. It also neglects the role of words themselves within sentences.
For example, consider the sentence"pushing the hot search to the top”. After preprocessing and word segmentation, this sentence is divided into “push”, “hot search”, and “to the top”. BTM’s biterm sampling, we obtain the results: “push-hot search”, “push-to the top”, “hot search-push”, “hot search-to the top”, “to the top-push”, and “to the top-hot search”. In this sentence, all six word pairs are sampled once, suggesting equal importance without distinguishing which pair is more important. However, in reality, the pairs “hot search-to the top” and “to the top-hot search” have a better semantic contribution to topic identification. By increasing the distribution probability of these word pairs with higher semantic contribution into the probability model, we can enhance the identification performance of the topic model.
This paper further explores two questions.
1. How can we effectively identify keywords with higher semantic contribution?
2. How can we enhance the distribution probability of these keyword pairs?
To address these questions, this study proposes improvements to the Gibbs sampling method based on the BTM model. Firstly, it introduces a part-of-speech weighting value K and utilizes the TF-IDF algorithm combined with part-of-speech weighting to identify the semantic contribution of keywords. Then, based on Donohue’s law of high-low word frequency boundary, it determines the number T of keywords. During the Gibbs sampling process, selected keyword pairs undergo extended sampling to enhance their role in topic identification. The framework of the KTF-BTM topic model is illustrated in Figure 2.
According to the KTF-BTM model diagram, the document generation process of the KTF-BTM model is as follows:
1. For each topic z.
(a) draw a topic-specific word distribution φz ∼ Dir (β+λ).
2. Draw a topic distribution θ ∼ Dir (α+λ) for the whole collection.
3. For each biterm w in the biterm set |B|+Q.
(a) draw a topic assignment z ∼ Multi(θ).
(b) draw two words: wa, wb ∼ Mulit (φz).
3.3 Determination of part-of-speech weighting value K
By linguistic knowledge, it is known that comment short texts are usually composed of words of different parts of speech. Generally speaking, in the process of topic identification, nouns and verbs carry the most significant information. Adjectives and adverbs contain rich semantic information and have stronger document representation capabilities. Quantifiers, particles, prepositions, and other parts of speech carry less semantic information and often appear frequently in documents, causing serious interference to topic identification. To eliminate the interference of low-information words, we can use part-of-speech tagging to weight the words, giving higher weights to words with richer semantic information. This allows for the extraction of more reasonable keywords for text representation. Based on experience, the specific definition is as follows:
3.4 Determination of the number of expanded keywords
The Term Frequency-inverse Document Frequency (TF-IDF) weighting method [22] is used to assess the importance of a word in a text. Its main idea is that if a word appears frequently in one document but rarely in other documents, it is considered an important keyword suitable for classification. TF-IDF is calculated by combining the term frequency and inverse document frequency, i.e., TF multiplied by IDF. Term frequency (TF) refers to the frequency of a given word appearing in a document, and it is calculated using the formula shown in Eq. 2:
Where
Where
In this study, we use TF-IDF to obtain the TF-IDF value ωa for all words. By applying the part-of-speech weight K, ωa is further weighted to describe the importance of words in the entire corpus. The number of keywords is determined based on Donohue’s law of high-frequency and low-frequency word boundaries [23]:
Where T represents the number of high-frequency words, and
4 Algorithm
During the initialization of Gibbs sampling, the decision to expand word pairs and increase the sampling count is based on the relationship between the weighted TF-IDF value ωa of word wa and the weighted TF-IDF value ωt of the given T-th keyword. If ωa>ωt, the word pair (wa,wb) will have its sampling count increased byωa *λ times.
If the condition is not met, normal sampling will take place.
During Gibbs sampling, the update of the topic during each sampling iteration is performed differently based on the relationship between ωa and the given semantic distance value ωt. If ωa > ωt, then executing Eq. 6:
Otherwise, executing Eq. 7:
Once Gibbs sampling is done, the counts
Finally, set the parameters and perform Gibbs sampling, updating the topic for each word pair. Repeat this process until Gibbs sampling converges.
After topic extraction, we obtain the probability distribution of topics for each Microblog post. The topic with the highest probability is considered as the corresponding topic for the comment text. As shown in Eq. 10:
Where
5 Experimental and analysis
5.1 Data collection and preprocessing
This article was written to develop a web crawler code based on actual data requirements. The code searches for relevant links using keywords such as “S University responds to alleged harassment incident” and “S University suspends classes for professor suspected of molesting students”. It collects the primary comments and their timestamps under the original MicroBlog posts within the timeframe of 6 December 2019, 20:00, to 12 December 2019, 23:59. A total of 18,658 comments were collected, and after data cleaning, 15,396 valid comments were obtained.
5.2 Evaluation metrics
The commonly used criteria for evaluating the effectiveness of topic identification are Topic Coherence (TC) and Jensen-Shannon Divergence (JS).
Topic Coherence is used to assess the performance of topic modeling. A method for calculating topic coherence was proposed in Ref. [24]. Its basic assumption is that words with similar meanings appear in similar contexts. If most or all words are closely related, the topic is considered coherent. This method measures the quality of the target based on word co-occurrence. The calculation formula is as shown in Eq. 11:
Where
Kullback-Leibler divergence (KL), can measure the difference between two separate probability distributions within the same event. A smaller KL distance indicates a higher similarity. In this paper, the KL distance is used to quantify the difference in topic-word distributions after being processed by different models. Under this condition, a larger KL distance implies a higher quality of obtained topics. The formula for KL distance is as shown in Eq. 12:
Where p and q represent the topic-word distributions of different topics, and xi represents the number of topic-word distributions.
Due to the asymmetry of traditional KL distance, it cannot fully represent the relationship between two topic-word distributions. The JS (Jensen-Shannon divergence) distance, which is based on KL distance, is symmetric and ranges from [0, 1]. A JS divergence closer to 1 indicates higher quality of obtained topics, and it can be used to measure the distribution differences between topics and words. The formula for calculating JS distance is as shown in Eq. 13:
Here,
5.3 Parameter settings
The KTF-BTM model proposed in this paper is influenced by the number of expansion sampling iterations λ during Gibbs sampling. In order to achieve better topic identification results, it is necessary to determine the optimal value of λ. By setting different values of λ, the coefficient corresponding to the maximum Topic Coherence (TC) value is selected as the number of expanded sampling iterations. In the experiment, the initial number of word pair expansion λ is set to 2, λ is gradually increased with a step size of 2, The selected λ values are 2, 4, 6, 8, 10, 12, 14, 16, 18, and 20. The Gibbs sampling parameters are set as α = 50, ß = 0.01, and the number of topics k is set to 5, 10, 15, and 20. The KTF-BTM model was applied to the corpus for topic identification, and the TC values are calculated by averaging the results of three experiments. Some experimental results are shown in Figure 3.
From Figure 3, it can be observed that when the number of topics is 5, there is not much difference in the TC values for different expansion sampling iterations λ. As the number of topics gradually increases, the TC values for different λ values show clear regular patterns. When λ is set to 2, the topic coherence is relatively low. As λ continues to increase, the TC values first increase and then decrease, indicating the effectiveness of keyword expansion in improving the BTM model. When λ is set to 8, the topic coherence is highest. Due to the limited number of keywords in short text comments, further increasing the λ value will lead to diluted sampling word pairs and a gradual decrease in topic coherence. Therefore, in the subsequent model comparison experiments, the optimal value for the expansion sampling iteration λ is determined to be 8.
5.4 Topic quality
First, a few words are randomly selected from the corpus as topic words. The model is then used to process the corpus and find the top 3 most relevant words to each topic word. Cosine similarity is used to measure the similarity between the topic words and the related words, where a cosine similarity value closer to 1 indicates a higher degree of correlation between the words. The experimental results are shown in Table 1, which demonstrate that the word vectors trained by our model align with the expected outcomes.
To verify the correlation between the extracted topics and related words from short text comments by the KTF-BTM model, a comparative experiment is conducted with the BTM model, LDA model, DMM model, and our model. The experimental results are shown in Table 2. In Table 2, there are 20 keywords under each topic, and 6 keywords are randomly selected based on topic relevance. It can be observed that the LDA model has a lower descriptive ability for topic-related words and performs poorly. On the other hand, our model extracts related words that describe the topics well, enhancing the correlation between words.
Quantitative evaluation refers to the use of quantitative metrics to assess the performance of topic identification models. In this paper, topic coherence and JS, divergence were used as two quantitative evaluation metrics. In the comparative experiment, the parameter settings were α = 50/k, β = 0.01, and λ = 5. With these parameters, topics were extracted with quantities of 5, 10, 15, 20, 25, 30, 40, and 50 for experimentation, and corresponding topic coherence (TC) and JS, divergence values were obtained.
Topic coherence is primarily used to evaluate the effectiveness of topic clustering. A higher TC value indicates better topic clustering. From Figure 4, it can be observed that there is little difference between the BTM model and the KTF-BTM model when the number of topics is 5 or 10. As the number of topics gradually increases, the difference in TC values between the models becomes more significant. The clustering effectiveness of the KTF-BTM model gradually surpasses that of the BTM model. The TC values of the LDA model consistently remain lower than those of the BTM and KTF-BTM models. When the number of topics is 5, 10, 15, or 20, the TC values of the DMM model are comparable to the other models, with a small decreasing trend. However, when the number of topics exceeds 20, the TC values decrease more significantly. The DMM model consistently has the lowest TC values among all the models, which contradicts the results of [3].
Topic diversity reflects the differences between topics. In this paper, JS distance is used to assess topic diversity. A higher JS value indicates better experimental results. From Figure 5, it can be observed that due to data sparsity, the LDA model has the lowest JS value. When the number of topics is less than 20, the DMM model has a lower JS value than the BTM model. However, when the number of topics exceeds 20, the DMM model has a higher JS value than the BTM model. This suggests that the JS value of the DMM model is heavily influenced by the number of topics. Compared to the BTM model, our model incorporates part-of-speech semantic weighting and keyword expansion, leading to further improvements in JS values. The JS values of our model consistently remain high, indicating greater differences between the topics discovered by our model.
5.5 Comparative analysis of topic models
Through comparative experiments between the proposed KTF-BTM model and the BTM, LDA, and DMM models, it was found that both the KTF-BTM and BTM models demonstrated superior performance compared to the LDA and DMM models in terms of experimental effectiveness. This indicates that the BTM model, along with its enhanced version that utilizes bilingual term sampling across the entire corpus, is more suitable for short text topic analysis when compared to the LDA and DMM models that rely on word co-occurrence analysis at the document level.
Furthermore, the KTF-BTM model delivered promising results in terms of topic quality, coherence (TC value), and diversity (JS value). These findings signify the substantial advantages of the KTF-BTM model in dealing with short text topic analysis. Therefore, building upon the success of the KTF-BTM model, this study will continue exploring short text topic modeling in the subsequent analysis.
5.6 Dividing time into slices
On 6 December 2019, the “S School Teacher Harassment of Female Students Incident” made it to the trending topics list on Microblog, sparking continuous attention and discussions. The incident was exposed on 6 December 2019, at 20:00 and gradually subsided by 12 December 2019. It exhibited a multi-peaked long-tailed characteristic, which does not conform to the classical lifecycle theory. In this study, we divided the timeline based on the development stages of the incident.
According to historical news reports, it can be noted that on the evening of December 6 at 20:00, after being exposed by a Microblog influencer, the incident quickly gained attention and sparked inquiries from the public and media. The “S School Teacher Harassment of Female Students Incident” started trending on Microblog. On December 7 at 13:40, a Microblog media outlet published a follow-up post regarding the handling of the incident, causing another round of public discussion. On December 9 at 21:47, the school issued an announcement on Microblog, stating that the implicated teacher had been dismissed, had their associate professor position revoked, and had their teaching qualification revoked, pending approval from higher authorities. This announcement once again drew attention to the incident. By December 12, public opinion gradually subsided. Based on significant time points in the development of the incident and considering the timing of Microblog media reports and user engagement, this study divided the data into three major phases: the initial escalation phase, the secondary escalation phase, and the subsiding phase, corresponding to the time points of 19:59 on December 6, 11:59 on December 7, and 23:59 on December 9, respectively.
In the digital age, the resolution of sudden public crises requires the establishment of a rapid response mechanism. On one hand, it is important to acquire critical information promptly and accurately assess the nature of the crisis. On the other hand, timely and proactive disclosure of information is necessary to safeguard the public’s right to be informed [25]. Based on the characteristics of public opinion outbreaks in the current converged media environment, the People’s Daily Public Opinion Data Center has proposed the “Golden Four Hours” principle, which emphasizes the need to clarify the facts, coordinate efforts, and complete information disclosure within 4 hours. [26], [27], [28], [29], and others have also emphasized the importance of adhering to the “Four Hour” principle in the process of handling public opinion. Therefore, this study takes 6 December 2019, as the starting point and divides the data into 37 small public opinion cycles based on 4-h intervals. This approach allows us to identify the focal issues that capture public attention within the first “Four Hours” and also capture the evolving details of public opinion in subsequent developments. Overall, analyzing the major public opinion cycles helps reveal the overall characteristics of the evolution of public opinion focus, while analyzing the minor public opinion cycles helps uncover the detailed characteristics of this evolution.
Building upon this, the KTF-BTM approach is employed to model the entire corpus and generate topics that are mapped to specific time periods. This enables a comparison of the changing characteristics of the focal points of public attention regarding the event during different public opinion cycles. The start and end dates of the public opinion cycles, as well as the number of Microblog posts during each time slice, are illustrated in Table 3.
5.7 Topic identification
In this study, we employed the KTF-BTM method to model the corpus. Based on the previous experiments, it was observed that setting different numbers of topics for the model led to varying TC (Topic Coherence) and JS (Jensen-Shannon Divergence) values. Thus, the number of topics directly affects the results and quality of topic mining. To determine the optimal number of topics, we used perplexity [30]. We calculated the perplexity values for different numbers of topics ranging from 5 to 90. The perplexity calculation results are illustrated in Figure 6.
As shown in Figure 6, perplexity exhibits an inverse relationship with the number of topics. When the number of topics is set to 45, the perplexity reaches a nadir. Furthermore, as the number of topics continues to increase, the rate of perplexity reduction slows down gradually, implying that there will be no significant improvement in topic identification and it may even lead to overfitting. Considering these factors collectively, this study selects M = 45 as the optimal number of topics.
5.8 The benefits of integrating the topic-temporal-focus framework in KTF-BTM
In order to verify the effectiveness of the Topic-Temporal-Focus framework in handling sparse data, this study selected the KTF-BTM model combined with the Topic-Temporal-Focus framework, as well as the individual KTF-BTM, BTM, LDA, and DMM models, to model the corpus within the 37 time slices. The perplexity was then used to determine the number of topics within each time slice. The obtained results were compared with the results from the KTF-BTM model integrated with the Topic-Temporal-Focus framework, shown in Figure 7.
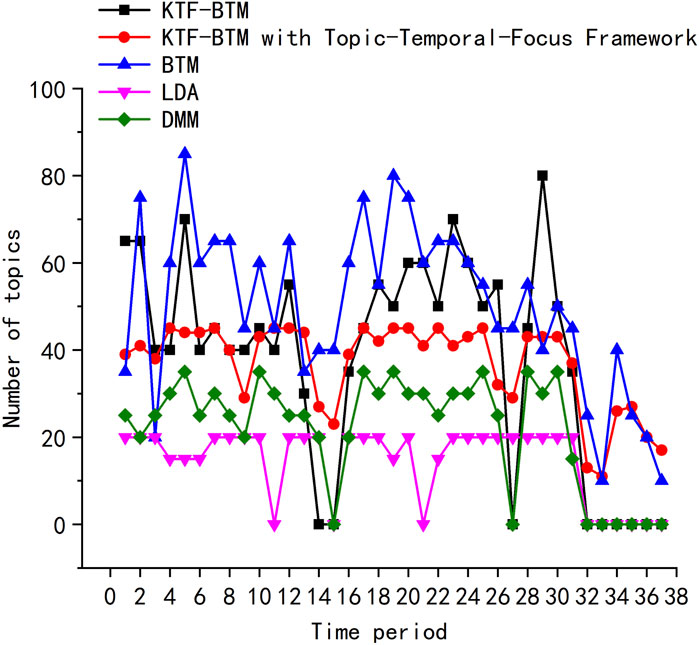
FIGURE 7. Comparison of the number of topics across KTF-BTM, BTM, LDA, DMM and KTF-BTM with Topic-Temporal-Focus framework.
In a broader context, dividing the corpus into 37 time slices leads to a significant reduction in the corpus size. When performing topic analysis on each time slices using the KTF-BTM and BTM models, a large number of noisy and redundant topics are generated, as depicted in Figure 7. The number of topics identified by the KTF-BTM and BTM models can even reach up to 90. Conversely, when conducting topic analysis on each time slices using the LDA and DMM models, the sparsity of features results in an insufficient number of topics and the loss of many fine-grained topics. Moreover, regardless of the chosen model, none of them can address the issue of potential topic loss caused by data sparsity. This is evident in the missing topic problem observed in time slices 32-37 for the KTF-BTM, LDA, and DMM models.
In conclusion, the advantages of the KTF-BTM model with the Topic-Temporal-Tocus framework are as follows:
1. It enables topic modeling of the entire corpus, thereby enhancing the quality of the generated topics.
2. By assigning each comment to the topic with the highest probability, it successfully addresses the problem of topic loss due to extreme data sparsity. Moreover, it captures the nuanced changes in the focus of comments within smaller time intervals.
5.9 Topic evolution analysis
As the categorization of topics involves subjective human labeling, this study employed the method of initial labeling by coders to extract 45 topic categories. Due to the limited interpretability of short text topic modeling results, we first divided the original comment texts into 45 categories based on the results of topic classification. Next, the original data was assigned to seven doctoral students specializing in management science and engineering public opinion management. Each coder was tasked with conducting preliminary labeling on the corresponding text data, extracting and identifying the relevant topics based on their understanding and perception. To deal with the labels provided by the seven coders, sections with overlapping semantics were further generalized, and areas of disagreement were discussed to eventually merge and select labels that were comprehensive and unambiguous. This process led to the definition of the 45 topic categories.
After topic extraction, the probability distribution of topics for each Microblog post was obtained. The topic with the highest probability was regarded as the corresponding topic for the comment text. The number of comments for each topic during three major public opinion cycles was then calculated, and a stacked chart depicting the evolution of topic popularity was generated as shown in Figure 8.
Based on Figure 9, the 8 comment focal points can be divided into two categories. The first category includes high-intensity topics such as discussing the event (attribution, process, details), discussing the teacher (identity, behavior, income, evaluation, condemnation, and insults), discussing the student (behavior, evaluation), and focusing on the disposition result. The second category consists of low-intensity sensitive topics, including related events, consulting authorities, maintaining attention, and discussing institutions.
According to Figure 8, it can be clearly seen that in the first stage, the topic with the highest level of engagement among all topics is “criticizing the actions of the involved teacher” (T28), accounting for 8.6%. This is followed by “expressing anger and emphasizing zero tolerance for such incidents” (T32), accounting for 5.9%, and “evaluating the behavior of the female student” (T14), accounting for 5.1%. Furthermore, netizens in the first stage showed a significantly higher level of interest in these three topics compared to the second and third stages. This is mainly because in the initial stage, when the incident was just exposed, netizens tend to focus on more straightforward topics such as who was involved and what happened, and engage in discussions around them. As the incident continued to unfold, netizens started to think and discuss more deeply, resulting in a gradual decrease in interest in these straightforward topics and a shift towards other topics.
Overall, throughout the entire public opinion cycle, netizens paid more attention to the occurrence, development, and handling outcomes of the incident, as well as the identities and actions of the individuals involved. For example, they focused on the handling outcomes, demanding the dismissal of the involved teacher and holding them accountable legally (T15). They emphasized continued attention to the handling outcomes and used strategies like trending searches to keep the topic relevant (T33). There were instances where extreme language was used to insult the involved teacher (T40). Criticism was also directed at the teacher’s moral integrity and personal attacks were launched (T43). Additionally, netizens showed interest in similar past incidents and made comparisons (T25).
In order to further summarize and categorize the distribution of comment focal points, this article classifies the 45 topics into 8 major comment focal points. The composition of each comment focal point is shown in Table 4. The definitions of comment focal points and examples of typical comment texts are provided in Table 5.
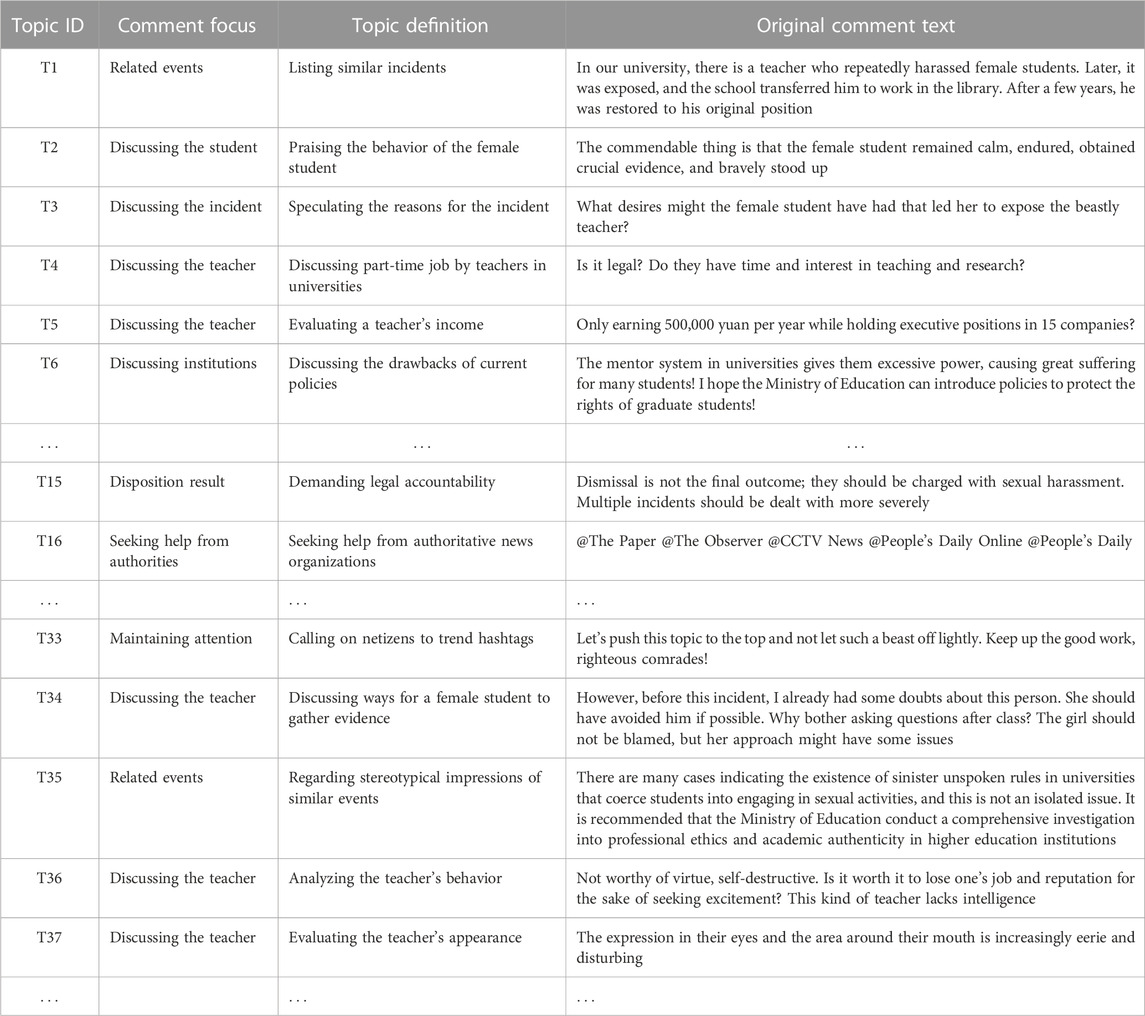
TABLE 4. Definition of topics contained in comment focus and examples of typical comment text (part).
Count the number of comments for each comment focal point within the three major public opinion cycles and create a stacked graph to visually illustrate the changing trends in topic popularity over time, as demonstrated in Figure 9.
The discussion surrounding the implicated teacher maintained the highest level of intensity throughout. In the second stage, the discussion saw an increase in intensity after the exposure of the teacher’s societal position. By the third stage, the intensity gradually declined, potentially due to a shift in focus to other topics following sustained discussions about the implicated teacher in the initial stages, thereby diluting the intensity of this particular topic. Regarding discussions about the outcome, there was a gradual increase from the first stage to the third stage. The initial stages primarily centered around speculations and expectations, while in the third stage, following the official announcement of the outcome, more netizens followed the media’s agenda, engaging in discussions and evaluations of the outcome, thereby intensifying this topic. It is notable that the topics of maintaining attention and consulting authorities peaked in the first stage. This was likely driven by concerns among some netizens during the initial exposure of the incident, who feared unfair treatment or suppression and thus sought to maintain topic intensity and expand its reach through maintaining attention and consulting authorities. In the second stage, as higher-level governing bodies responded to the incident, the intensity of these discussions gradually decreased. However, in the third stage, after the governing bodies officially announced the outcome, some netizens expressed their desire for legal consequences against the individuals involved by actively maintaining attention, resulting in an increased intensity of this topic.
When we divide Microblog comment texts into the three aforementioned public opinion cycles, we can observe the evolving characteristics of various topics from a macro perspective. The evolving characteristics of high-intensity topics are particularly noticeable, while the features of low-intensity but sensitive topics such as related events, consulting authorities, maintaining attention, and discussing institutions are not prominent. In practical public opinion monitoring, these low-intensity sensitive topics carry potential risks, necessitating real-time monitoring of changes in related comments to make appropriate public opinion management decisions at the right time.
Therefore, it is necessary to divide Microblog comment texts into shorter time periods and analyze the patterns of low-intensity sensitive topics in the evolution of public opinion. In Table 5, we have grouped the Microblog comment texts into 37 smaller public opinion cycles with a time interval of 4 h. Next, we will explore the changing characteristics of low-intensity sensitive topics (related events, consulting authorities, maintaining attention, discussing institutions) in the S School teacher harassing female students incident through line graphs.
As shown in Figure 10, in terms of topic popularity, “Maintaining attention” has the highest level of heat among these four topic categories. This may be because some netizens are concerned that the event will not receive fair treatment, so they deliberately boost its popularity to maintain high exposure. Essentially, this can be regarded as an act of safeguarding rights in the face of injustice. In terms of evolutionary characteristics, the topic of “Maintaining attention” shows the earliest and fastest increase in heat. The fluctuations in topic popularity throughout the entire public opinion cycle may be related to the sleeping habits of netizens, as seen in the third time period (12-7 4:00-12-7 7:59), where the topic heat decreases, possibly because it coincides with netizens’ sleeping hours. On the other hand, it may also be related to the confrontational mentality of netizens. When they feel that the event is being forcefully suppressed or not properly addressed, they resort to boosting its search rankings as a way of resisting and safeguarding their rights. For example, during the early stages of the first and second phases, netizens engaged in activities to boost the topic’s popularity in response to attempts to suppress it.
Overall, the changing trends of these four low-intensity sensitive topics are generally similar throughout the entire public opinion cycle. The main difference lies in the first stage of public exposure (initial fermentation period, corresponding to time periods 1–4). The topic of “maintaining attention” experiences an explosive increase in heat right from the beginning, while the topic of “consulting authorities” remains relatively low in terms of overall heat. From the perspective of the topic itself, it experiences the highest level of heat at the initial stage of the event. It is also an act of netizens exercising their rights in the face of injustice, although fewer netizens chose this approach in this particular incident. The topics of “discussing institutions” and “related events” follow the overall trend of heat in the event. These two types of topics are submerged amidst the high-intensity events, making them less noticeable but still carrying the risk of generating secondary public opinion. Therefore, they deserve continuous attention in public opinion monitoring.
6 Conclusion and outlook
The purpose of this article is to explore the evolution patterns of netizens’ comment focus in the online public opinion of universities. Firstly, in order to improve the effectiveness of short text topic identification in Microblog comments, this article combines the traditional BTM model and proposes a KTF-BTM method for short text topic identification based on the fusion of BTM and TF-IDF. Building upon the BTM model, the Gibbs sampling method is improved by introducing a part-of-speech weighting value K. By incorporating the part-of-speech weighted TF-IDF algorithm, the semantic contribution of keywords is identified. Furthermore, based on Donohue’s law of high and low word frequency decomposition, the number of key words T is determined. During the Gibbs sampling process, the selected keyword pairs are expanded for sampling to enhance their role in topic identification. Experimental results indicate that, considering topic quality, topic coherence, and topic diversity, the KTF-BTM model outperforms the traditional BTM, LDA, and DMM models, demonstrating the effective improvement of the KTF-BTM model in accurately identifying topics in Microblog comments.
Furthermore, this article uses the case of a teacher harassing female students at S University to construct a topic-temporal-focus framework. Combined with the general time patterns of netizens using social media, the comment texts are divided into 37 small public opinion cycles with a time interval of 4 h, in order to explore the content and evolution patterns of netizens’ focus within shorter time sequences. The experimental results show that the KTF-BTM model, combined with the topic-temporal-focus framework, overcomes the problem of sparse comment data within shorter time periods and achieves topic classification within short time sequences. This is helpful for further exploring the content and evolution patterns of comment focus within shorter time sequences. By utilizing the topic-temporal-focus framework, this article identifies four high-intensity topics and four low-intensity sensitive topics in the case of a teacher harassing female students at S University. The high-intensity topics include discussions about the incident (causation, process, details), discussions about the individual involved (identity, behavior, income, evaluation, condemnation), discussions about the students (behavior, evaluation), and attention to the handling results. The low-intensity sensitive topics include raising related incidents, seeking help from authorities, generating hype, and discussing institutional issues. High-intensity topics are issues of general concern among netizens, often involving discussions about the occurrence, development, and outcome of the incident, as well as evaluations or expressions of emotions regarding the actions of the individuals involved. Low-intensity sensitive topics have a relatively lower proportion of intensity, and when there are greater risks, such as behaviors aimed at generating hype, there is already a clear manifestation of resistance consciousness and behavior, posing a risk of group polarization. In traditional topic identification processes, such topics may be overlooked due to sparse data and low topic intensity. The method proposed in this article can further monitor the evolving characteristics of low-intensity sensitive topics.
Based on the above research, we can draw the following conclusions:
The main factors influencing the evolution of comment focus and topics include the nature and stage of the event, the involved parties, official handling (including stages, methods, response speed, response content, etc.), media involvement, and online user interactions. These factors also reflect the focus of netizens’ attention and their emotional expressions. When the comment focus is centered around the event and the involved parties, the comments tend to be more expression of emotions. When the comment focus is related to higher-level authorities, there is a possibility of provocative expressions. Therefore, in future studies, the author will further analyze the emotions associated with comment focus, using the PAD emotional model to examine the emotional characteristics of different types of comment focus, and further analyze the changes in emotions during the evolution of comment focus.
By analyzing the evolution of comment focus and topics, it can help public opinion management departments gain a clear understanding of the content that netizens are focused on within the “golden 4 hours,” as well as the interactive changes between event trends, media involvement, official handling, and public opinion focus during the evolution of public opinion. This allows for the selection of appropriate topics and content based on public concerns, and targeted information dissemination and communication. By timely and accurately adjusting propaganda strategies, responding to public concerns, and guiding public opinion based on public sentiment and attitudes, effective public opinion management can be achieved.
Data availability statement
The raw data supporting the conclusion of this article will be made available by the authors, without undue reservation.
Author contributions
YZ provided this topic and wrote the manuscript. J-QL, R-DL, and H-TD guided, discussed, and modified the manuscript. All authors contributed to the article and approved the submitted version.
Funding
This work was supported by the ‘Chenguang Program’ supported by Shanghai Education Development Foundation and Shanghai Municipal Education Commission (No. 20CGB07), Shanghai Municipal Youth Teacher Training Support Program (ZZSPPC21002), the National Natural Science Foundation of China (Grant No. 71901144), China Youth and Children Research Association (Grant No. 2023B18), the Foundation of USST (Grant No. 22SLCX-ZD-005).
Conflict of interest
The authors declare that the research was conducted in the absence of any commercial or financial relationships that could be construed as a potential conflict of interest.
Publisher’s note
All claims expressed in this article are solely those of the authors and do not necessarily represent those of their affiliated organizations, or those of the publisher, the editors and the reviewers. Any product that may be evaluated in this article, or claim that may be made by its manufacturer, is not guaranteed or endorsed by the publisher.
References
1. Blei DM, Ng AY, Jordan MI. Latent dirichlet allocation. J Machine Learn Res (2003) 3:993–1022. doi:10.1162/jmlr.2003.3.4-5.993
2. Yin J, Wang J. A dirichlet multinomial mixture model-based approach for short text clustering. In: Proceedings of the 20th ACM SIGKDD international conference on Knowledge discovery and data mining (2014). p. 233–42.
3. Jocelyn M, de Wall A. A comparison of the performance of latent dirichlet allocation and the dirichalet multinomial mixture model on short-text. In: Proc of 2016 Pattern Recognition Association of South Africa and Robotics and Mechatronics International Conference(PRASA-RobMech) (2016). p. 1–6.
4. Yan X, Guo J, Lan Y, Cheng X. A biterm topic model for short texts. In: Proceedings of the 22nd International Conference on WWW (2013).
5. He S. Precise prediction modeling of university social network public opinion evolution trend. In: Proceedings of SPIE, 12453 (2022). p. 1245318.
6. Lv Z. Prediction of the forwarding volume of campus microblog public opinion emergencies using neural network. Mobile Inf Syst (2022) 2022:1–8. doi:10.1155/2022/3064266
7. Wang M, Lei G. Research on the construction of quantitative index of microblog group polarization in university public opinion events. J Engineering-Joe (2022) 2022:285–94. doi:10.1049/tje2.12113
8. Zhou H, Li X. Quantitative research on the evolution stages of we-media network public opinion based on a logistic equation. Tehnicki Vjesnik-Technical Gaz (2021) 28:983–93. doi:10.17559/TV-20210316155352
9. Cui X, Hu Y, Ding X-F., Wu Y, Wu R-J. Study on the mechanism of guiding internet public opinion based on point centrality in SNA. J Sichuan Univ (2011) 43:104–8. (in Chinese).
10. Liu Y, Zhang H, Xu H, Wei P. Research on evolutionary topic map of internet public opinion with multi-dimensional feature fusion. J China Soc Scientific Tech Inf (2019) 38:798–806. doi:10.3772/j.issn.1000-0135.2019.08.004
11. Kaixi T, Lixun X, Lifa L. Research on the evolution path of public opinion in environmental emergencies. E3S Web of Conferences (2021) 257:03059. doi:10.1051/e3sconf/202125703059
12. He W, Fang Y, Malekian R, Li Z. Time series analysis of online public opinions in colleges and universities and its sustainability. Sustainability (2019) 11:3546. doi:10.3390/su11133546
13. Vaisman A, Arolfo F. Analyzing the quality of twitter data streams. Inf Syst Front (2020) 24:349–69. doi:10.1007/s10796-020-10072-x
14. Cai L, Zhu Y. The challenges of data quality and data quality assessment in the big data era. Data Sci J (2015) 14:2–3. doi:10.5334/dsj-2015-002
15. Arolfo F, Rodriguez KC, Vaisman A. Analyzing the quality of twitter data streams. Inf Syst Front (2022) 24:349–69. doi:10.1007/s10796-020-10072-x
16. Qureshi MA, Asif M, Hassan MF, Abid A, Kamal A, Safdar S, et al. Sentiment analysis of reviews in natural language: Roman Urdu as a case study. Ieee Access (2022) 10:24945–54. doi:10.1109/access.2022.3150172
17. Cuong H, Van-Dang I, Linh Ngo V, Khoat T. Eliminating overfitting of probabilistic topic models on short and noisy text: The role of dropout. Int J Approximate Reasoning (2019) 112:85–104. doi:10.1016/j.ijar.2019.05.010
18. Chen J, Gong Z, Liu W. A Dirichlet process biterm-based mixture model for short text stream clustering. Appl Intelligence (2020) 50:1609–19. doi:10.1007/s10489-019-01606-1
19. Nimala K, Jebakumar R. A robust user sentiment biterm topic mixture model based on user aggregation strategy to avoid data sparsity for short text. J Med Syst (2019) 43:93. doi:10.1007/s10916-019-1225-5
20. Zhu L, Xu H, Xu Y, Xiao Y, Li J, Deng J, et al. A joint model of extended LDA and IBTM over streaming Chinese short texts. Intell Data Anal (2019) 23:681–99. doi:10.3233/ida-183836
21. Murshed BAH, Abawajy J, Mallappa S, Saif MAN, Al-Ghuribi SM, Ghanem FA. Enhancing big social media data quality for use in short-text topic modeling. Ieee Access (2022) 10:105328–51. doi:10.1109/access.2022.3211396
22. Salton G, Buckley C. Term-weighting approaches in automatic text retrieval. Inf Process Manag (1988) 24:513–23. doi:10.1016/0306-4573(88)90021-0
23. Donohue JC. Understanding scientific literature—a bibliometric approach. Cambridge: The MIT Press (1973).
24. Mimno DM, Wallach HM, Talley EM, Leenders M, Mccallum A. Optimizing semantic coherence in topic models. In: Proceedings of the 2011 Conference on Empirical Methods in Natural Language Processing, EMNLP (2011). p. 27–31. John McIntyre Conference Centre, Edinburgh, UK, A meeting of SIGDAT, a Special Interest Group of the ACL.
25. Wang L, Li C. The influencing factors and mechanisms of sudden public crisis resolution in the era of the internet: A qualitative comparative analysis based on 40 cases. Mod Commun (2021) 43:81–6. (in Chinese).
26. Cai Y, Wu P, Wang J, Zhang J. The research on negative emotion cognitive decision-making process of netizens of micro-blog based on ACT-R theoretical model. Inf Sci (2018) 36:135–40. (in Chinese).
28. Li W, Jian Y. Judgment on the decline of network public opinion in emergent public events:multiple case study based on RBF neural network. Inf Documentation Serv (2022) 43:48–57. (in Chinese).
29. Zou H, Liu H. Evolution and response of online public opinion in campus bullying:A case study based on the "zhongguancun second primary school bullying incident. Res Educ Develop (2018) 38:42–8. (in Chinese).
Keywords: topic modeling, BTM, university public opinion, social media, microblog
Citation: Zhang Y, Lian J-Q, Li R-D and Duan H-T (2023) Research on the evolution of netizens’ comment focus in university online public opinion: KTF-BTM topic model with topic-temporal-focus framework. Front. Phys. 11:1251386. doi: 10.3389/fphy.2023.1251386
Received: 01 July 2023; Accepted: 15 August 2023;
Published: 25 August 2023.
Edited by:
Hui-Jia Li, Beijing University of Posts and Telecommunications (BUPT), ChinaReviewed by:
Haicheng Tao, Nanjing University of Finance and Economics, ChinaJinlong Ma, Hebei University of Science and Technology, China
Copyright © 2023 Zhang, Lian, Li and Duan. This is an open-access article distributed under the terms of the Creative Commons Attribution License (CC BY). The use, distribution or reproduction in other forums is permitted, provided the original author(s) and the copyright owner(s) are credited and that the original publication in this journal is cited, in accordance with accepted academic practice. No use, distribution or reproduction is permitted which does not comply with these terms.
*Correspondence: Hong-Tao Duan, aHRkdWFuQHNqdHUuZWR1LmNu