- 1Odontostomatologic and Specialized Clinical Sciences Department, Università Politecnica delle Marche, Ancona, Italy
- 2Neurosciences Imaging and Clinical Sciences Department, University of Chieti-Pescara, Chieti, Italy
- 3Medical Oral and Biotechnological Sciences Department, University of Chieti-Pescara, Chieti, Italy
Mechanical stimuli are regulators not only in cells but also of the extracellular matrix activity, with special reference to collagen bundles composition, amount and distribution. Synchrotron-based phase-contrast computed tomography was widely demonstrated to resolve collagen bundles in 3D in several body districts and in both pre-clinical and clinical contexts. In this perspective study we hypothesized, supporting the rationale with synchrotron imaging experimental examples, that deep learning semantic image segmentation can better identify and classify collagen bundles compared to common thresholding segmentation techniques. Indeed, with the support of neural networks and deep learning, it is possible to quantify structures in synchrotron phase-contrast images that were not distinguishable before. In particular, collagen bundles can be identified by their orientation and not only by their physical densities, as was made possible using conventional thresholding segmentation techniques. Indeed, localised changes in fiber orientation, curvature and strain may involve changes in regional strain transfer and mechanical function (e.g., tissue compliance), with consequent pathophysiological implications, including developmental of defects, fibrosis, inflammatory diseases, tumor growth and metastasis. Thus, the comprehension of these kinetics processes can foster and accelerate the discovery of therapeutic approaches for the maintaining or re-establishment of correct tissue tensions, as a key to successful and regulated tissues remodeling/repairing and wound healing.
1 Introduction
The collagen family consists of about 30 proteins, all structural molecules of great importance in the human body. The most abundant collagen is type I, which forms fibrillar networks that shape and strengthen tissues such as skin, tendons and bones. The three-dimensional structure and organization of these networks adapt to different tissue-specific functions. For example, collagen in weight-bearing tendons forms thick fibers (200 nm) that are aligned along the tendon to optimize force transmission and tendon strength. Conversely, collagen in the cornea forms interwoven sheets of thin (∼30 nm) fibers that provide strength combined with optical transparency. Moreover, collagen in interstitial tissue mainly forms isotropic networks, which provide mechanical strength combined with porosity to facilitate nutrient transport and cell migration [1].
Structure and mechanics of collagen determine not only the function of the tissue as a whole, but also the functions of the cells residing in the tissue. Collagen fibers provide cells with topographical, biochemical and mechanical signals, which regulate cell proliferation, differentiation, migration and apoptosis [2]. The mechanobiological interplay between cells and the surrounding collagen extracellular matrix is essential to guide physiological processes such as wound healing, but it can also trigger pathological processes [1]. Abnormal stiffening of interstitial collagen networks, for instance, promotes cell invasion, which contributes to fibrosis, cancer and metastasis [3].
The relation between collagen structure and its biomechanics has triggered a long history of research: in fact, it has been known for some time that collagen shows a non-linear elasticity due to a stiffening induced by deformation [4]. This mechanical design allows tissues such as skin and arteries to be soft at low strain but stiff at high strain, providing mechanical stability under large loads [5]. However, the complex architecture of collagenous tissues, which is structured on different dimensional scales, makes it difficult to identify the structural basis of the stiffening response following deformation. Tissues contain networks of bundles of fibrils, which in turn contain hundreds of molecules per cross section packed into an axially ordered lattice [6]: in fact, thanks to X-ray scattering studies, it has been known for decades that multiple mechanisms operating on different length scales contribute to the overall mechanical response at the tissue level [7]. In this context, using X-ray diffraction data, a model of the nanomechanics of a collagen microfibril that incorporates the full biochemical details of the amino acid sequence of constituting molecules and the nanoscale molecular arrangement was presented and experimentally validated. They found that collagen molecules alone are unable to provide the wide range of mechanical functionality required for the physiological function of collagenous tissues. Instead, a number of deformation mechanisms, due to the material’s hierarchical composition, are critical to the material’s ability to impart the key mechanical properties, i.e., the large extensibility, strain hardening, and toughness [6].
For a long time it was also believed that, during wound healing, tissue tension was attributed to forces produced by tissue-resident (myo-)fibroblasts alone; conversely, it was recently found in a wound healing model that the storage of tensile forces in the collagen bundles of the extracellular matrix has a significant, so-far neglected contribution to macroscopic tissue tension (Figure 1) [8]. Thus, in general, the rate of collagen deposition determines the amount of macroscopic contraction and tension of the regenerating tissues, which is important for restoring their function. Increased contraction, however, is associated with conditions such as fibrosis and cancer. Indeed, if the tissue begins to stiffen, these cells produce more collagen, disrupting this balance. This increases the stiffness of the organ, stimulating the fibroblasts to release even more collagen. However, while this explains how fibrosis progresses, it is less clear how the cycle begins [9].
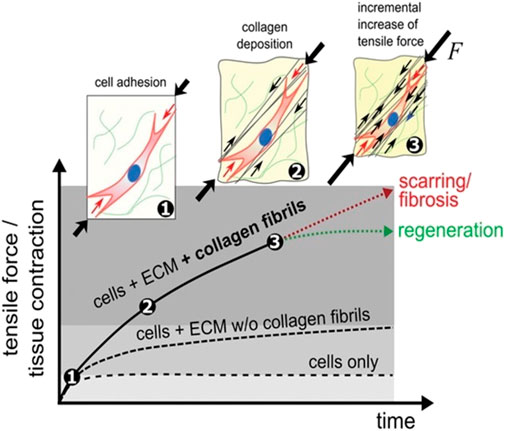
FIGURE 1. Tissue formation and tensioning process. After initial cell diffusion and adhesion to a scaffold (1), cells progressively deposit tense collagen fibrils (black arrows, 2) leading to a gradual increase in total force resulting in macroscopic contraction (3). The amount of macroscopic force exceeds the sum of individual cell forces or contributions from non-fibrillar ECM networks, demonstrating that fibrillar collagen forces strongly contribute to tissue contraction during wound healing ([8]; CC-BY-4.0).
2 Contribution of synchrotron-based phase-contrast imaging
The study of structural morphology of collagen fibers seems to be crucial to better understand the biological events associated with wound healing, fibrosis, tumorigenesis and metastasis, and patients’ stratification. Only an advanced three-dimensional (3D) characterization of the collagenous tissue could improve our knowledge of its structure.
In this framework, high-resolution synchrotron-based X-ray tomographic microscopy was shown to be a useful tool in analyzing topology and morphometry of structures in 3D collagen matrices for more than 10 years [10,11].
In particular, X-ray phase-contrast imaging (XRPCI) represents a powerful method to study the microarchitecture of collagenous tissues. Indeed, while the conventional absorption-based contrast originates from attenuation mismatches between different tissues inside a sample, the XRPCI contrast is due to the phase-shift δ of the refractive index n = 1 − δ + iβ, representing the interaction of X-ray photons with the tissues. The δ shift, in non-mineralized biological tissues like collagenous tissues, can be up to three orders of magnitude larger than the attenuation complex value β, allowing to achieve a reliable 3D imaging, with increased contrast, for the analysis of several organs/tissues.
In the last 25 years, different approaches for phase-based X-ray imaging methods have been explored and are nowadays widely applied. The most diffused phase sensitive methods are propagation-based imaging [12–14], analyzer-based imaging [15,16], edge illumination [17–19], Talbot (or Grating) X-ray interferometry [20–22]. The propagation-based imaging is the simplest one, as no optical elements are needed in the beam and there is no constraint for beam monochromaticity.
In this context, in the last 10 years, the study of collagenous tissues by synchrotron radiation-based high-resolution phase-contrast tomography (SR-PhC-microCT) spread rapidly. In particular, SR-PhC-microCT was successful in detecting, with high spatial resolution, the 3D structural organization of the extracellular matrix (ECM) within bioscaffolds, supporting the understanding on how the presence of cells modified the construct arrangement [23,24]). Moreover, SR-PhC-microCT successfully imaged collagenous tissues in osteons [25,26], intervertebral discs [27], dermal tissues [28], cartilages [29], vessels [30], meniscus tissue [31], tendons [32,33], liver [34,35], cardiac endomyocardium [36], uterine myometrium and leiomyomas [37,38], vocal folds [39] and neck of dental implants [40], demonstrating the capability of this method to discriminate healthy and pathologic tissues.
3 The perspective of introducing deep learning-based image analysis
There are limitations associated with the use of synchrotron-based phase contrast imaging for the unraveling of the biomechanical properties of collagenous tissues pathologies. For example, limited synchrotron availability hampers the time it takes to produce more replicas; synchrotron structures can be accessed on the basis of a peer review application and are not as widely available as other imaging techniques. Second, experiments are usually limited to the capabilities of the beamline, i.e., the samples studied must fit the field of view and resolution of the beamline. For a synchrotron (parallel) beam, the field of view is ultimately limited by the size of the beam; therefore, a trade-off between field of view and resolution must be reached. Moreover, further studies are needed to establish parameters that can be used as reliable quantitative measures of image structures to describe tissue quality and/or function. With reference to this last point, many studies underline the need to quantitatively determine the complexity of the shape of the collagenous tissues; in particular, it is essential to quantitatively evaluate the preferential directions of the collagenous bundles and their connectivity degree [1,28,40].
To overcome this last limitation, i.e., the difficulty of quantifying the direction and connectivity of collagen bundles in all tissue contexts, an interesting perspective is offered by artificial intelligence (AI) and in particular by deep learning (DL) algorithms. Very recently, we demonstratively showed that AI could be applied directly on high-resolution images acquired by synchrotron-based phase contrast tomography to automatically segment the collagen bundles of the connective tissue surrounding dental implants [41]. Artificial neural networks have been able to distinguish the inner portions of the soft tissue not only based on the grey levels of the synchrotron image, as conventional thresholding methods do, but also based on the orientation of the collagen bundles themselves. In this way, it was possible to quantitatively distinguish longitudinal and transverse peri-implant collagen bundles, with evidence of the timing and methods of formation of the connective tissue around the implant during the wound healing process (Figure 2).
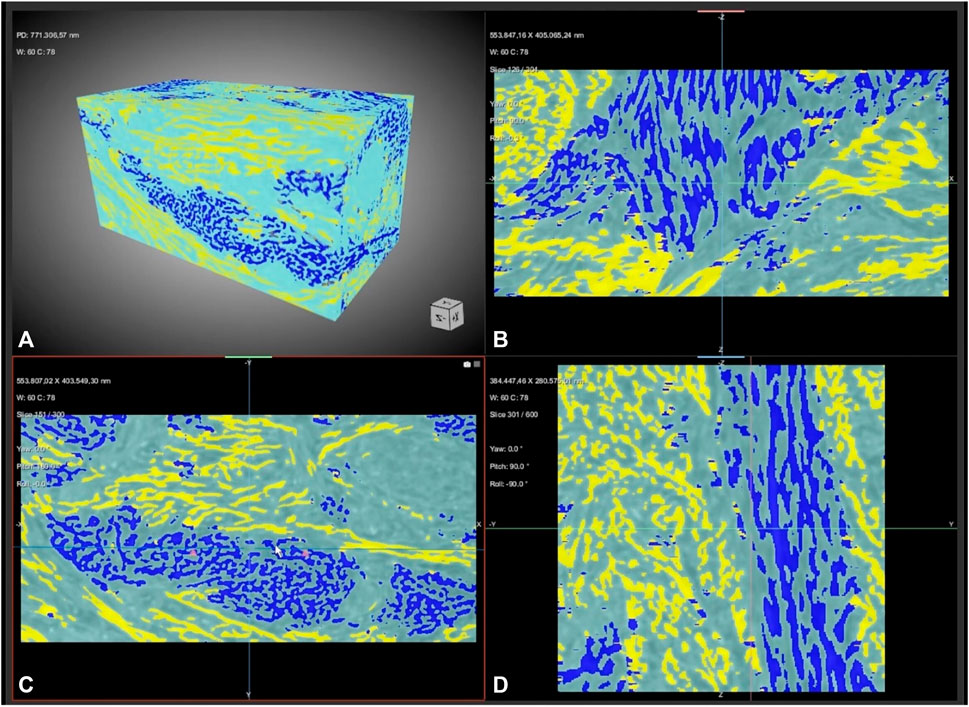
FIGURE 2. Connective tissue rearrangement around a dental implant during the wound healing process. The semantic segmentation, with a training based on three classes, allows to distinguish not only background (light blue) from connective tissue signals but also, unlike conventional threshold-based segmentation, transversal bundles (yellow) from longitudinal bundles (dark blue). (A) 3D reconstruction (B–D) sampling (B) sagittal, (C) axial and (D) frontal slices. Freeze frame from Supplementary Video S1 in Ref ([41]; CC-BY-4.0).
In particular, the semantic segmentation method used by us assigns a label to each pixel of the synchrotron images, based on the morphometric characteristics of the image; for example, if two objects within the image have different shapes or directions, they will be classified as two distinct subgroups.
The use of the semantic segmentation method involves neural networks: U-Net, a convolutional neural network (CNN) designed for segmentation of biomedical images [42], proved to be a good choice in the case of collagenous tissues [41]. With the support of neural networks and deep learning, it has been possible to quantify structures in the samples that had not previously been considered. In particular, the collagen bundles were identified by their orientation and not by their physical density: this is essential to discriminate transverse and longitudinal bundles which, up to now, could not be distinguished using conventional thresholding techniques since their physical density is identical. In practice, we succeeded in creating a neural network capable of separating longitudinal and transverse fibers via U-Net. Furthermore, regarding the connectivity density parameter, it was observed that results obtained in deep learning were higher for all samples than those obtained with conventional thresholding; this fact is certainly attributable to an increased capability to discriminate collagen bundles and therefore their connectivity through artificial intelligence protocols.
In summary, we observed that the introduction of DL-based image analysis allows for a better investigation of the directionality (isotropy/anisotropy) and connectivity of the collagen bundles. These results provide a new method to understand the relationship between collagen network mechanics and microstructure under a broad range of assembly conditions and tissue districts.
Therefore, in the present prospective study, we suggest that the microscopic information on collagen network isotropy/anisotropy and connectivity are parameters that can be easily determined by synchrotron-based phase-contrast imaging processed by DL-based data analysis. These morphometric data of shape complexity are of fundamental importance because they are able to reliably reveal correlations with macroscopic measurements of the nonlinear elastic behavior of collagenous tissues.
In this direction, an immediate challenge is to understand the most suitable sample size to obtain effective and statistically consistent data in the various pathophysiological and tissue regeneration contexts. Our first study in a regenerative context revealed, using a very small sample size, significant differences in the two parameters of interest, namely, the connectivity and the degree of orientation of the collagen bundles [41]. However, it is necessary to be very careful and scrupulous in this area because other studies in the field of oral implantology [43] suggest the need for a larger sample size, at least 8–10 samples per group of study, to obtain possible statistically significant mismatches of the same parameters during clinical staging. Furthermore, a high rigor is needed also in the choice of statistical methods of investigation, evaluating the opportunity to use tests that can release at least two statistical parameters, i.e., the normality and the equivariance of the sampled data distribution.
In fact, especially in tumor contexts, there may be evident morphometric heterogeneities that require advanced statistical tools and, in any case, also the systematic comparison with histopathological findings. To this purpose, an innovative multidisciplinary approach based on SR-PhC-microCT, light and electron microscopy, and Fourier Transform Infrared Imaging Spectroscopy was recently exploited to better characterize microstructural collagen features [38]. Indeed, the cross-linking of high-resolution analytical tools, combining the investigation of the tridimensional organization and of the secondary structure of collagen, was shown to be useful to identify defined markers correlating the status of this protein with specific pathological conditions.
4 Discussion
The DL-based analysis applied to synchrotron-based phase contrast imaging was successfully used not only in studying wound healing but also to detect liver fibrotic progress, including the early stages [44], and osteoarthritis [45]. In these cases, the feasibility of extracting texture features for quantified diagnosis was shown, evaluating the performance of back propagation (BP) neural net classifier [44] or CNNs [45]. These studies showed that these approaches were effective for staging the pathologies, supporting our perspective idea to reliably reveal, by AI approaches, correlations between the 3D micro-texture and the macroscopic nonlinear elastic behavior of collagenous tissues.
In addition to the already shown impact of AI-based workflows in studying wound healing and fibrosis processes, it could be extremely important to apply the same method in future studies aimed at finding the potential influence of organization and function of collagen on tumor invasion and metastasis. In fact, some studies already suggest that the quantification of collagen and its directionality determine a new practicable paradigm for the prediction of cancer survival [46]. Indeed, several characteristics of the tumor ECM have been associated with progression to metastases. Notably, dense collagen regions are often co-localized with aggressive tumor cell phenotypes in numerous solid tumors, including breast, ovarian, pancreatic, and brain cancers. Furthermore, collagen fibers scattered and aligned at the edges of tumors have also been reported to correlate with aggressive disease [47].
In conclusion, combining synchrotron-based phase-contrast microtomography with deep learning-based image segmentation was shown to be a promise method to localise changes in collagen fiber orientation, curvature and strain. This may be correlated to changes in regional strain transfer and mechanical function (e.g., tissue compliance). The full comprehension of these processes would allow to achieve, as final targeting objective, a quantitative intelligible framework to redirect collagen networks towards the desired mechanical properties, which is useful for the mechano-regulation of cell migration, wound healing, and tissue morphogenesis.
Data availability statement
The raw data supporting the conclusions of this article will be made available by the authors, without undue reservation.
Author contributions
MF, NR, and AG contributed to conception and design of the study. AG wrote the first draft of the manuscript. MF, NR, and MDN wrote sections of the manuscript. All authors contributed to manuscript revision, read, and approved the submitted version.
Funding
The APC costs were funded by the University Research Funds (responsible: AG).
Acknowledgments
Figure 2 was generated using Dragonfly software, Version 2022.1 for Windows, Object Research Systems (ORS) Inc., Montreal, Canada, 2020; software available at http://www.theobjects.com/dragonfly (accessed on 30 January 2023).
Conflict of interest
The authors declare that the research was conducted in the absence of any commercial or financial relationships that could be construed as a potential conflict of interest.
Publisher’s note
All claims expressed in this article are solely those of the authors and do not necessarily represent those of their affiliated organizations, or those of the publisher, the editors and the reviewers. Any product that may be evaluated in this article, or claim that may be made by its manufacturer, is not guaranteed or endorsed by the publisher.
References
1. Jansen KA, Licup AJ, Sharma A, Rens R, MacKintosh FC, Koenderink GH. The role of network architecture in collagen mechanics. Biophysical J (2018) 114(11):2665–78. doi:10.1016/j.bpj.2018.04.043
2. Kai F, Laklai H, Weaver VM. Force matters: Biomechanical regulation of cell invasion and migration in disease. Trends Cell Biology (2016) 26(7):486–97. doi:10.1016/j.tcb.2016.03.007
3. Song K, Yu Z, Zu X, Li G, Hu Z, Xue Y. Collagen remodeling along cancer progression providing a novel opportunity for cancer diagnosis and treatment. Int J Mol Sci (2022) 23(18):10509. doi:10.3390/ijms231810509
4. Fung Y. Structure and stress-strain relationship of soft tissues. Am Zool (1984) 24:13–22. doi:10.1093/icb/24.1.13
5. Shadwick RE. Mechanical design in arteries. J Exp Biol (1999) 202(23):3305–13. doi:10.1242/jeb.202.23.3305
6. Orgel JP, Irving TC, Miller A, Wess TJ. Microfibrillar structure of type I collagen in situ. Proc Natl Acad Sci United States America (2006) 103(24):9001–5. doi:10.1073/pnas.0502718103
7. Misof K, Rapp G, Fratzl P. A new molecular model for collagen elasticity based on synchrotron X-ray scattering evidence. Biophysical J (1997) 72(3):1376–81. doi:10.1016/S0006-3495(97)78783-6
8. Brauer E, Lippens E, Klein O, Nebrich G, Schreivogel S, Korus G, et al. Collagen fibrils mechanically contribute to tissue contraction in an in vitro wound healing scenario. Adv Sci (Weinheim, Baden-Wurttemberg, Germany) (2019) 6(9):1801780. doi:10.1002/advs.201801780
10. Pabst AM, Wagner W, Kasaj A, Gebhardt S, Ackermann M, Astolfo A, et al. Synchrotron-based X-ray tomographic microscopy for visualization of three-dimensional collagen matrices. Clin Oral Invest (2015) 19(2):561–4. doi:10.1007/s00784-014-1312-4
11. Liou H, Lin H, Chen W, Liou W, Hwu Y. Tomography observations of osteoblast seeding on 3-D collagen scaffold by synchrotron radiation hard X-ray. J Biomimetics, Biomater Tissue Eng (2009) 3:93–101. doi:10.4028/www.scientific.net/jbbte.3.93
12. Snigirev S, Snigireva I, Kohn V, Kuznetsov S, Schelokov I. On the possibilities of X-ray phase contrast microimaging by coherent high-energy synchrotron radiation. Rev Sci Instrum (1995) 66:5486–92. doi:10.1063/1.1146073
13. Wilkins SW, Gureyev TE, Gao D, Pogany A, Stevenson AW. Phase-contrast imaging using polychromatic hard X-rays. Nature (1996) 384:335–8. doi:10.1038/384335a0
14. Cloetens P, Luding W, Baruchel J, Van Dyck D, Van Landuyt J, Guigay JP, et al. Holotomography: Quantitative phase tomography with micrometer resolution using hard synchrotron radiation x rays. Appl Phys Lett (1999) 75:2912–4. doi:10.1063/1.125225
15. Chapman D, Thomlinson W, Johnston RE, Washburn D, Pisano E, Gmür N, et al. Diffraction enhanced x-ray imaging. Phys Med Biol (1997) 42(11):2015–25. doi:10.1088/0031-9155/42/11/001
16. Kitchen MJ, Paganin DM, Uesugi K, Allison BJ, Lewis RA, Hooper SB, et al. Phase contrast image segmentation using a Laue analyser crystal. Phys Med Biol (2011) 56(3):515–34. doi:10.1088/0031-9155/56/3/001
17. Olivo A, Ignatyev K, Munro PRT, Speller RD. A coded-aperture based method allowing non-interferometric phase contrast imaging with incoherent X-ray sources. Nucl Instr Methods Phys Res Section A: Acc Spectrometers, Detectors Associated Equipment (2011) 648(1):S28–31. doi:10.1016/j.nima.2011.01.018
18. Olivo A, Diemoz PC, Bravin A. Amplification of the phase contrast signal at very high x-ray energies. Opt Lett (2012) 37(5):915–7. doi:10.1364/OL.37.000915
19. Olivo A, Gkoumas S, Endrizzi M, Hagen CK, Szafraniec MB, Diemoz PC, et al. Low-dose phase contrast mammography with conventional x-ray sources. Med Phys (2013) 40(9):090701. doi:10.1118/1.4817480
20. Momose A. Demonstration of phase-contrast X-ray computed tomography using an x-ray interferometer. Nucl Instrum Methods A (1995) 352:622–8. doi:10.1016/0168-9002(95)90017-9
21. Momose A. Phase-sensitive imaging and phase tomography using X-ray interferometers. Opt express (2003) 11(19):2303–14. doi:10.1364/oe.11.002303
22. Momose A, Takeda T, Itai Y, Hirano K. Phase-contrast X-ray computed tomography for observing biological soft tissues. Nat Med (1996) 2(4):473–5. doi:10.1038/nm0496-473
23. Albertini G, Giuliani A, Komlev V, Moroncini F, Pugnaloni A, Pennesi G, et al. Organization of extracellular matrix fibers within polyglycolic acid-polylactic acid scaffolds analyzed using X-ray synchrotron-radiation phase-contrast micro computed tomography. Tissue Engineering C, Methods (2009) 15(3):403–11. doi:10.1089/ten.tec.2008.0270
24. Giuliani A, Moroncini F, Mazzoni S, Belicchi ML, Villa C, Erratico S, et al. Polyglycolic acid-polylactic acid scaffold response to different progenitor cell in vitro cultures: A demonstrative and comparative X-ray synchrotron radiation phase-contrast microtomography study. Tissue Engineering Part C, Methods (2014) 20(4):308–16. doi:10.1089/ten.TEC.2013.0213
25. Langer M, Pacureanu A, Suhonen H, Grimal Q, Cloetens P, Peyrin F. X-ray phase nanotomography resolves the 3D human bone ultrastructure. PloS one (2012) 7(8):e35691. doi:10.1371/journal.pone.0035691
26. Stockhausen KE, Qwamizadeh M, Wölfel EM, Hemmatian H, Fiedler IAK, Flenner S, et al. Collagen fiber orientation is coupled with specific nano-compositional patterns in dark and bright osteons modulating their biomechanical properties. ACS nano (2021) 15(1):455–67. doi:10.1021/acsnano.0c04786
27. Disney CM, Eckersley A, McConnell JC, Geng H, Bodey AJ, Hoyland JA, et al. Synchrotron tomography of intervertebral disc deformation quantified by digital volume correlation reveals microstructural influence on strain patterns. Acta Biomater (2019) 92:290–304. doi:10.1016/j.actbio.2019.05.021
28. Jiang Y, Tong Y, Xiao T, Lu S. Phase-contrast microtomography with synchrotron radiation technology: A new noninvasive technique to analyze the three-dimensional structure of dermal tissues. Dermatology (Basel, Switzerland) (2012) 225(1):75–80. doi:10.1159/000341530
29. Horng A, Stroebel J, Geith T, Milz S, Pacureanu A, Yang Y, et al. Multiscale X-ray phase contrast imaging of human cartilage for investigating osteoarthritis formation. J Biomed Sci (2021) 28(1):42. doi:10.1186/s12929-021-00739-1
30. Giuliani A, Mazzoni S, Mele L, Liccardo D, Tromba G, Langer M. Synchrotron phase tomography: An emerging imaging method for microvessel detection in engineered bone of craniofacial districts. Front Physiol (2017) 8:769. doi:10.3389/fphys.2017.00769
31. Einarsson E, Pierantoni M, Novak V, Svensson J, Isaksson H, Englund M. Phase-contrast enhanced synchrotron micro-tomography of human meniscus tissue. Osteoarthritis and cartilage (2022) 30(9):1222–33. doi:10.1016/j.joca.2022.06.003
32. Gigante A, Busilacchi A, Lonzi B, Cecconi S, Manzotti S, Renghini C, et al. Purified collagen I oriented membrane for tendon repair: An ex vivo morphological study. J orthopaedic Res : official Publ Orthopaedic Res Soc (2013) 31(5):738–45. doi:10.1002/jor.22270
33. Pierantoni M, Silva Barreto I, Hammerman M, Verhoeven L, Törnquist E, Novak V, et al. A quality optimization approach to image Achilles tendon microstructure by phase-contrast enhanced synchrotron micro-tomography. Scientific Rep (2021) 11(1):17313. doi:10.1038/s41598-021-96589-w
34. Zhang X, Yang XR, Chen Y, Li HQ, Li RM, Yuan QX, et al. Visualising liver fibrosis by phase-contrast X-ray imaging in common bile duct ligated mice. Eur Radiol (2013) 23(2):417–23. doi:10.1007/s00330-012-2630-z
35. Fu Y, Peng HJ, Zhang X, Peng WJ, Wu J, Wang SP, et al. Assessment of fibrotic tissue and microvascular architecture by in-line phase-contrast imaging in a mouse model of liver fibrosis. Eur Radiol (2016) 26(9):2947–55. doi:10.1007/s00330-015-4173-6
36. Planinc I, Ilic I, Dejea H, Garcia-Canadilla P, Gasparovic H, Jurin H, et al. A novel three-dimensional approach towards evaluating endomyocardial biopsies for follow-up after heart transplantation: X-ray phase contrast imaging and its agreement with classical histopathology. Transpl Int : official J Eur Soc Organ Transplant (2023) 36:11046. doi:10.3389/ti.2023.11046
37. Giuliani A, Greco S, Pacilè S, Zannotti A, Delli Carpini G, Tromba G, et al. Advanced 3D imaging of uterine leiomyoma's morphology by propagation-based phase-contrast microtomography. Scientific Rep (2019) 9(1):10580. doi:10.1038/s41598-019-47048-0
38. Belloni A, Furlani M, Greco S, Notarstefano V, Pro C, Randazzo B, et al. Uterine leiomyoma as useful model to unveil morphometric and macromolecular collagen state and impairment in fibrotic diseases: An ex-vivo human study. Mol basis Dis (2022) 1868(12):166494. doi:10.1016/j.bbadis.2022.166494
39. Bailly L, Cochereau T, Orgéas L, Henrich Bernardoni N, Rolland du Roscoat S, McLeer-Florin A, et al. 3D multiscale imaging of human vocal folds using synchrotron X-ray microtomography in phase retrieval mode. Scientific Rep (2018) 8(1):14003. doi:10.1038/s41598-018-31849-w
40. Iezzi G, Di Lillo F, Furlani M, Degidi M, Piattelli A, Giuliani A. The symmetric 3D organization of connective tissue around implant abutment: A key-issue to prevent bone resorption. Symmetry (2021) 13:1126. doi:10.3390/sym13071126
41. Riberti N, Furlani M, D’Amico E, Comuzzi L, Piattelli A, Iezzi G, et al. Deep learning for microstructural characterization of synchrotron radiation-based collagen bundle imaging in peri-implant soft tissues. Appl Sci (2023) 13:4423. doi:10.3390/app13074423
42. Ronneberger O, Fischer P, Brox T. U-Net: Convolutional networks for biomedical image segmentation. In: N Navab, J Hornegger, W Wells, and A Frangi, editors. Medical image computing and computer-assisted intervention – miccai 2015. Miccai 2015. Cham: Springer (2015).
43. Canullo L, Giuliani A, Furlani M, Menini M, Piattelli A, Iezzi G. Influence of abutment macro- and micro-geometry on morphologic and morphometric features of peri-implant connective tissue. Clin Oral Implants Res (2023):1–14. doi:10.1111/clr.14118
44. Wang J, Wang M, Gao S, Li H. Evaluation of texture features at staging liver fibrosis based on phase contrast X-ray imaging. Biomed Eng Online (2018) 17(1):179. doi:10.1186/s12938-018-0612-3
45. Stroebel J, Horng A, Armbruster M, Mittone A, Reiser M, Bravin A, et al. Convolutional neuronal networks combined with X-ray phase-contrast imaging for a fast and observer-independent discrimination of cartilage and liver diseases stages. Sci Rep (2020) 10:20007. doi:10.1038/s41598-020-76937-y
46. Conklin MW, Eickhoff JC, Riching KM, Pehlke CA, Eliceiri KW, Provenzano PP, et al. Aligned collagen is a prognostic signature for survival in human breast carcinoma. Am J Pathol (2011) 178(3):1221–32. doi:10.1016/j.ajpath.2010.11.076
Keywords: collagen, synchrotron radiation, phase-contrast, deep learning, wound healing, fibrosis, cancer
Citation: Furlani M, Riberti N, Di Nicola M and Giuliani A (2023) Unraveling the biomechanical properties of collagenous tissues pathologies using synchrotron-based phase-contrast microtomography with deep learning. Front. Phys. 11:1220575. doi: 10.3389/fphy.2023.1220575
Received: 10 May 2023; Accepted: 10 July 2023;
Published: 19 July 2023.
Edited by:
Sandro Donato, Department of Physics, University of Calabria, ItalyReviewed by:
Maria Lasalvia, University of Foggia, ItalyCopyright © 2023 Furlani, Riberti, Di Nicola and Giuliani. This is an open-access article distributed under the terms of the Creative Commons Attribution License (CC BY). The use, distribution or reproduction in other forums is permitted, provided the original author(s) and the copyright owner(s) are credited and that the original publication in this journal is cited, in accordance with accepted academic practice. No use, distribution or reproduction is permitted which does not comply with these terms.
*Correspondence: Alessandra Giuliani, YS5naXVsaWFuaUB1bml2cG0uaXQ=
†These authors have contributed equally to this work