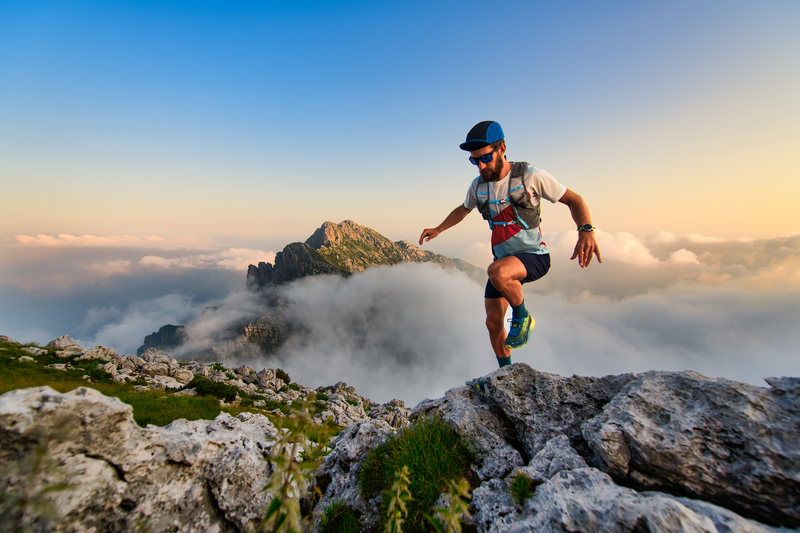
94% of researchers rate our articles as excellent or good
Learn more about the work of our research integrity team to safeguard the quality of each article we publish.
Find out more
ORIGINAL RESEARCH article
Front. Phys. , 09 June 2023
Sec. Quantum Engineering and Technology
Volume 11 - 2023 | https://doi.org/10.3389/fphy.2023.1166275
Athletes often suffer from knee joint injuries because they often use the knee joint to exert force during training. This paper aims to analyze and discuss the auxiliary diagnosis of the knee joint sports injury detection model based on machine learning. This paper expounds the treatment method of knee joint injury, and proposes a machine learning algorithm. On the basis of this research, the auxiliary diagnosis experiment of the knee joint sports injury detection model is analyzed. The experimental results show that after 3 months of machine learning-based rehabilitation training, there is a significant difference in the duration of the balance pad before and after the table tennis players practice. The duration of the athletes on the balance mat has increased, and the increase is relatively large. Among them, the average duration of female athletes on the balance mat increased from 75.5 seconds before training to 141.9 seconds after training, while the average duration of male athletes on the balance mat increased from 66.7 seconds before training to 136.8 seconds after training. Studies have shown that machine learning-based rehabilitation physical training can significantly improve athletes' endurance on balance mats and can improve knee function scores. In summary, machine learning-based rehabilitation physical training can effectively improve knee joint injuries.
Table tennis is a kind of net competition in which players cannot have physical contact. However, in the competition, athletes must carry out high-intensity footwork. When the knee joint flexes for a long time, if the stability of the knee is not good, or if the muscle group close to the knee does not have enough strength, it may cause injury to the knee joint. In the training of table tennis players, coaches focus on special training and ignore other training, such as strength and body conditioning. They do not strengthen the weak ribs of the body, so the incidence of injuries to athletes is getting higher and higher. Second, due to the lack of supervision and protection of the team doctor, once an athlete is injured and the injury is not handled in time, it would affect the development of technology and tactics. In serious cases, this would lead to the athlete not being able to train normally. Sports injuries cause a great load on athletes’ bodies and seriously affect their daily lives and training. Therefore, the development of rehabilitation physical training can prevent and treat knee injuries and improve the function of knee joints, reduce knee joint injuries, and enable athletes to get rid of injuries. The purpose of this paper was to analyze and discuss the auxiliary diagnosis of the knee joint sports injury detection model based on machine learning to make certain contributions to knee joint injury repair.
According to the research progress, different researchers have also made corresponding cooperative research on knee joint sports injuries. Most knee injuries are caused by the increase in vertical pressure, which is generally due to accidental falls, collisions, or high jumps during skiing. Trobec R introduced a new mechanism of non-contact knee joint injury that could occur during gliding [1]. Snooker B found through research that the probability of knee osteoarthritis would be increased by six times after knee joint injury. The most dangerous injuries are cruciate ligament, meniscus tear, and intra-articular fractures [2]. Welsch GH carried out semiquantitative occupational knee MRI examinations in the “medical” stage and studied the relationship between clinical data and knee injury absenteeism rate [3]. Goreta R conducted a new questionnaire survey on knee pain and injuries among 39 top Croatian League A players, and the results showed that the test instrument had good measurement performance [4]. However, these scholars lack some technical demonstration in the research of knee joint sports injuries. It is found that machine learning has a better discovery in the research of knee joint sports injuries. In this regard, the relevant literature on machine learning was consulted.
Some scholars also carried out some research on machine learning. Hinterwimmer F aimed to study whether machine learning models used in artificial knee surgery are feasible and to determine the premises for the effective application of this new technology [5]. The purpose of Smna B is to study a machine learning method based on big data before surgery, which can be used to predict the hospitalization time and hospitalization cost of patients with initial knee replacement [6]. Verstratete M A evaluated the application of a monitored machine learning model to evaluate the orthopedics of total knee surgery based on patients’ special operations [7]. However, these scholars did not analyze and discuss the auxiliary diagnosis of a knee joint sports injury detection model based on machine learning, they just unilaterally discussed its significance.
This paper drew the following conclusions by analyzing the experimental results of the auxiliary diagnosis of the knee joint sports injury detection model. After the rehabilitation physical training based on machine learning, the endurance of athletes on the balance pad could be effectively extended. At the same time, it improved the score of the knee joint function rating scale. In a word, rehabilitation physical training based on machine learning plays a certain role in preventing and treating knee trauma.
The innovations of this paper are as follows: 1) the treatment of knee joint injuries is analyzed, and a method of building a knee joint sports injury detection model based on machine learning is proposed; 2) by taking table tennis players as an example, this paper makes an experimental study on the repair of their knee joint injuries.
The knee joint is the largest and most complex joint composed of the tibiofemoral joint and the patellofemoral joint. The femoral condyle and the nearly flat proximal articular surface of the tibia form a joint, so the stability is poor. This mainly depends on the joint capsule, ligament, meniscus, and surrounding strong muscle groups. The anatomical structure of the knee joint is complex, and it bears the weight and greater stress of the body. Its stability is poor, so the knee joint is easy to be injured [8]. The knee joint injury is shown in Figure 1. After a knee joint injury, surgical treatment is ruled out, and conservative treatment is often performed with physical therapy. Physiotherapy uses physical factors such as force, light, electricity, sound, water, and temperature to treat diseases. Physical therapy commonly used after knee joint injury includes physical factor therapy and exercise therapy.
(1) Physical factor therapy
Physical factor therapy can prevent and treat diseases through various physical factors, including natural physical factors and artificial physical factors. Natural physical factors include seawater, air, sunlight, and mineral springs. Artificial physical factors include electricity, sound, magnetism, light, and heat [9]. Physical factor therapy has the functions of analgesia, anti-inflammatory, improving blood circulation of tissues and organs, relieving muscle spasms, and promoting tissue repair and regeneration. Cold therapy, ultrashort wave therapy, and ultrasound are the commonly used physical factor therapy after knee joint injury. Cold therapy is a kind of therapy that uses ice water or ice cubes that are lower than the body temperature to stimulate the skin or mucous membrane. It has the effect of preventing further tissue swelling and relieving pain. Therefore, cold therapy can be used to treat acute inflammation after knee joint injury [10]. Ultrashort wave therapy belongs to high-frequency electrotherapy, which can produce physiological effects on the human body through non-thermal and thermal effects. It can promote the expansion of capillaries and enhance the permeability of the blood vessel wall [11]. It can also improve blood circulation, inhibit the conduction of sensory nerve fibers, and promote tissue metabolism. Therefore, it can diminish inflammation, relieve pain, and promote the regeneration of connective and granulation tissues.
(2) Exercise therapy
Exercise therapy is an essential rehabilitation method, and physical and mechanical factors are used to carry out exercise therapy. It is a very effective rehabilitation method to correct limb dysfunction and abnormal movement posture through multiple movements [12]. The rehabilitation methods for knee joint injuries include a range of joint motion training, muscle strength training, balance and proprioception training, and functional training.
Machine learning is the use of computer algorithms, statistical models, and computer systems to gradually improve the ability to complete specific tasks. Nowadays, with the wide application of machine learning algorithms in the medical field, many machine learning algorithms that can solve specific medical problems have also been significantly developed [13]. Figure 2 shows the application of machine learning in medicine. In the past 10 years, the use of machine learning algorithms to predict diseases has been rapidly developed [14]. Ranging from macrophysiology and behavior and experimental basic research and imaging research to increasingly “omics” data, machine learning algorithms are applied.
As a machine learning algorithm based on statistical learning, support vector machine (SVM) has a good application prospect in the fields of small sample, non-linear, and high-dimensional pattern recognition [15]. Therefore, by considering the actual medical data collected and the number of data samples, features, and other factors of the public disease dataset used, this study uses SVM to build a knee joint sports injury detection model. SVM is widely used in various pattern recognition problems, such as portrait recognition, text classification, handwritten character recognition, and bioinformatics.
The basic idea of a support vector machine is to segment the dataset on the optimized hyperplane [16]. The purpose of this paper is to use SVM to summarize the data of knee joint sports injury and to determine its diagnosis methods. Given the sports training data
Here, e is the normal vector of the hyperplane and n is the distance from the hyperplane to the origin. The distance between c and the hyperplane can be expressed using the following formula:
If the data in F can be correctly divided by the hyperplane according to the classification requirements, we obtain
The distance of the two nearest points to the hyperplane is as follows:
At this time, the so-called optimal hyperplane is also the hyperplane that maximizes t. The problem for solving the optimal hyperplane can be expressed as follows:
The formula is given as
The model corresponding to the optimal hyperplane obtained by solving Formula 6 can be expressed as follows:
When the sports injury training data are non-linear and separable, there may not be any hyperplane in the original feature space that can accurately divide the data categories. At this time, SVM makes the sample data separable by mapping the sports injury original data to the high-dimensional space. The corresponding model is shown as follows:
Here,
Its dual problem is as follows:
When the characteristic dimension is high, it is difficult to calculate the inner product
After being solved, the formula is simplified as follows:
In addition, in practical problems, it is difficult to determine an appropriate kernel function so that each data sample in the training set can be accurately divided. Therefore, SVM also introduces the concept of the soft interval, which allows it to make mistakes on some samples, that is, some samples cannot meet the constraints of Formula 13.
However, when searching for the optimal hyperplane, the number of samples that do not meet the constraints of Formula 13 is required to be as small as possible, and the relaxation variable
Here, V is the penalty factor. After the concept of kernel function being introduced, the support vector model that is same as shown in Formula 12 can be obtained.
The kernel function and penalty factor V have a great impact on the final performance of SVM [17]. Only proper kernel functions and reasonable parameter settings can make the sample data linearly separable in the feature space. For the penalty factor V, when the value of V is too large, the relaxation variable would be very small and the allowable error would also be smaller. Therefore, the classifier would try to classify the samples correctly to obtain a small spacing hyperplane. This may lead to the poor classification performance of the model for test data, while the classification performance on the training set is very good. On the contrary, if the value of V is too small, the allowable error of the model would be large. Even if the training set is linearly separable, there may be wrongly classified samples, resulting in the phenomenon of under-fitting. With the development of the social medical level, the scale of medical databases is increasing rapidly. Unfortunately, most of the data have not been analyzed to discover valuable hidden laws. Now, people can integrate these data and use machine learning algorithms to discover hidden relationships. The models developed through these technologies would help doctors make correct diagnostic decisions.
The research object is the knee joint injuries of table tennis players in a normal university.
Subjects: 18–24 years old; table tennis level II (or higher); athletes who meet the Functional Movement Screen (FMS) and the knee joint rating scale would get not more than 8 points (12 points in total) in the FMS exam and 90 points (100 points in total) in the knee joint exam.
The rehabilitation physical training program based on machine learning is formulated. This training is conducted in two phases, that is, it is conducted after the table tennis training. During training, the intensity and load of athletes will vary according to their physique and gender. After receiving the body recovery training based on machine learning, athletes must actively cooperate and stop other aspects of training.
Tables 1, 2 show that the body mass index (BMI) and weight of male and female athletes changed little before and after training. Before training, the BMI and average weight of male athletes were 23.35 kg/m2 and 69.50 kg, respectively. After training, BMI and average weight of male athletes were 23.32 kg/m2 and 69.52 kg, respectively. Before and after training, thigh circumference and calf circumference showed significant differences. The average thigh circumference and calf circumference of women before training were 53.72 cm and 33.45 cm, respectively. However, after training, the average thigh circumference and calf circumference were 54.37 cm and 34.17 cm, respectively.
Table 2 shows the comparison of various physical indicators of women table tennis players before and after training studied with the independent sample T after 3 months of rehabilitation training. Before and after training, there was no significant difference in weight and BMI of female athletes. However, there were obvious differences in thigh circumference and calf circumference before training and after training.
Table 3 shows the physical indicators of the male table tennis players studied with an independent sample T before and after 3 months of restorative physical training, indicating that there was no significant difference in body weight and body mass index before and after training, and there was a significant difference in thigh circumference and calf circumference before training and after training. Before training, the average thigh circumference of male athletes was 58.20 cm and the average calf circumference was 39.10 cm. However, after training, the average thigh circumference was 58.92 cm and the average calf circumference was 39.80 cm.
Figure 3 shows the thigh circumference of the athletes before and after training. Figure 3A shows thigh circumference of the male athletes, and Figure 3B shows the thigh circumference of the female athletes. After 3 months of rehabilitation training, the thigh circumference of male table tennis players before and after training was tested with an independent sample T, and the results showed that there was a significant difference in the thigh circumference of male athletes before and after training. The average circumference of the left thigh of male athletes before training was 58.19 cm, and the average circumference of the right thigh of male athletes was 58.31 cm. After training, the left thigh circumference averaged 58.84 cm, and the right thigh circumference averaged 59.01 cm. The thigh circumference of female athletes before and after training was tested with the independent sample T, and the results showed that there was a significant difference in the thigh circumference of female athletes before and after training. Before training, the average circumference of the left thigh of female athletes was 53.35 cm and the average circumference of the right thigh was 54.10 cm. After training, the average circumference of the left thigh was 54.05 cm and the average circumference of the right thigh was 54.70 cm.
FIGURE 3. Thigh circumference of athletes before and after training (unit: cm). (A) Thigh circumference of male athletes. (B) Thigh circumference of female athletes.
Figure 4 shows the calf circumference of athletes before and after training. Figure 4A shows the calf circumference of the male athletes, and Figure 4B shows the calf circumference of the female athletes. After 3 months of restorative exercise, the calf circumference of male athletes was studied with an independent sample T, and the results showed that there were significant differences in the calf circumference of male athletes before and after training. The average circumference of the left leg of male athletes before training was 39.00 cm, and the average circumference of the right leg was 39.20 cm. After training, the average circumference of the left leg was 39.75 cm, and the average circumference of the right leg was 39.85 cm. The calf circumference of female athletes before and after training was tested with the independent sample T, and the results showed that there was a significant difference between the calf circumference of female athletes before and after training. Before training, the average circumference of the left leg of female athletes was 33.61 cm, and the average circumference of the right leg was 33.55 cm. After training, the average circumference of the left leg was 34.10 cm, and the average circumference of the right leg was 34.24 cm.
FIGURE 4. Athletes’ calf circumference before and after training (unit: cm). (A) Calf circumference of male athletes. (B) Calf circumference of female athletes.
(1) FMS test scores of athletes before and after rehabilitation training
Figure 5 shows the scores of athletes’ FMS tests. Figure 5A shows the FMS test scores of female athletes, and Figure 5B shows the FMS test scores of male athletes. After 3 months of recovery, the FMS scores of female athletes before and after training were tested with the independent sample T. The results showed that there were significant differences in the performance of female athletes in squats, hurdles, lunge squats, straight knee lifts, and other events. Among them, the average score of female athletes before training was 1.7 points for deep squats, 1.8 points for hurdles, 1.9 points for bow squats, and 2.1 points for straight knee lifting; after training, the average score of female athletes was 2.3 points for squats, 2.4 points for hurdles, 2.4 points for lunge squats, and 2.6 points for straight knee lifting. Before training, the knee joint stability of female athletes was poor, and the female athletes showed obvious shaking in the hurdle test, with an average score of 1.8 points. However, after training, the average score of female athletes in hurdles rose to 2.4. Through the independent sample T, male athletes showed significant differences in the scores of squats before and after training, and there were significant differences in the scores of hurdles, lunge squat, straight knee, and leg lifting. Before training, the average score for male athletes was 1.8 points for squats, 1.9 points for hurdles, 1.9 points for lunge squat, and 1.9 points for straight knee lifting; after training, the average score of male athlete was 2.5 points for squats, 2.3 points for hurdles, 2.4 points for lunge squat, and 2.6 points for straight knee lifting.
(2) Jump performance of athletes before and after rehabilitation training
FIGURE 5. Athletes’ FMS test scores (unit: points). (A) FMS test scores for female athletes. (B) FMS test scores for male athletes.
It can be seen from Figure 6 that after 3 months of rehabilitation training, athletes were conducted the vertical jump with an independent sample T before and after training, and the results showed that there was a significant difference between athletes’ jumping ability before and after training. The average longitudinal jump performance of male athletes before training was 33.30 cm, and the average longitudinal jump performance after training was 35.90 cm.
(3) Peak torque of knee flexor and extensor muscles of athletes before and after rehabilitation training
Figure 7 shows the peak torque of the athletes’ flexors and extensors at the speed of 60/s. Figure 7A shows the peak torque of male athletes, and Figure 7B shows the peak torque of female athletes. After 3 months of rehabilitation training, the flexor and extensor torque tests of male athletes before and after training were conducted with an independent sample T, and the results showed that the flexor and extensor torques of male athletes before and after training were significant. Before training, the average values of left bending, left extension, right bending, and right extension of male athletes were 79.4 N·m, 155.7 N·m, 85.9 N·m, and 158.1 N·m, respectively. After training, the average value of left bending was 99.2 N·m, left extension was 177.6 N·m, right bending was 126.0 N·m, and right extension was 180.3 N·m for male athletes. The flexion and extension muscle torque test results of female athletes before and after training with an independent sample T showed that there was a significant difference between the flexion and extension muscle torque of female athletes before and after training. Before training, the average value of left bending was 40.2 N·m, left extension was 84.3 N·m, right bending was 41.1 N·m, and right extension was 87.8 N·m for female athletes. After training, the average value of left bending was 54.6 N·m, left extension was 115.5 N·m, right bending was 61.8 N·m, and right extension was 118.4 N·m for female athletes.
FIGURE 7. Peak moment of flexors and extensors of athletes at 60°/s (unit: N·m). (A) Peak torque for male athletes. (B) Peak torque for female athletes.
(1) Duration of athletes’ balance pads before and after rehabilitation training
Figure 8 shows the results of the duration of the balance pad of athletes before and after training with an independent sample T after 3 months of recovery physical exercise, and there was a significant difference in the duration of the balance pad of athletes before and after training. Among them, the average duration of male athletes’ balance pads before training was 66.7 s, and the average duration of female athletes’ balance pads was 75.5 s. However, after training, the average duration of male athletes’ balance pads was 136.8 s and the average duration of female athletes’ balance pads was 141.9 s. Both male and female athletes have increased the duration of the balance pad.
(2) The score of the knee joint functional evaluation scale of athletes before and after rehabilitation training
Figure 9 shows the scores of the quantitative table of knee joint functional evaluation of athletes before and after training tested with an independent sample T after 3 months of rehabilitation and physical training, and the results showed that there were significant differences in the scores of the quantitative table of knee joint function evaluation before and after training. Among them, the average score of male athletes’ knee joint functional evaluation before training was 88.6 points, and the average score of female athletes’ knee joint functional evaluation was 89.0 points. After training, the average score of male athletes’ knee joint functional evaluation was 93.0 points, and that of female athletes was 92.8 points.
\After 3 months of rehabilitation and physical training, the knee joint functional assessment scale showed significant differences before and after training. Athletes started table tennis training at an early age and lack knowledge of sports injury prevention, and coaches rarely conduct rehabilitation physical training. Therefore, long-term special training has caused chronic injuries to athletes’ knees and other parts. In addition, table tennis players have no warm-up activities before special training. After training, relaxation without traction is likely to lead to muscle fatigue. Some athletes said that when the exercise intensity is high, the knee joint would have slight discomfort. Therefore, in rehabilitation training, it is necessary to constantly remind and guide athletes to join in dynamic stretching, muscle activation, and other warm-up activities before special training; after the training, it is necessary to carry out fascia relaxation, static traction, and other relaxation activities. After rehabilitation and physical training, the strength of the muscles around the knee joint of the athletes would be improved through strength training, such as resistance knee extension training and resistance knee flexion training of the legs. This can improve the stability of the athletes’ knee joints and reduce the injuries of athletes in high-intensity sports. After 3 months of rehabilitation training, the scores of the table tennis players on the knee function assessment scale have improved. The average score of female athletes’ knee joints increased from 89.0 before training to 92.8 after training; the average score of male sports knee joints increased from 88.6 before training to 93 after training. This shows that the rehabilitation physical training based on machine learning improves the score of the knee joint function scoring scale.
Machine learning can improve people’s ability to solve problems and draw reasonable inferences from a large number of problems, including assisting in disease diagnosis and developing solutions to global climate change. The purpose of this paper was to discuss the value of the auxiliary diagnosis of knee joint sports injury detection models based on machine learning. Rehabilitation training based on machine learning plays a key role in the prevention and treatment of knee joint injuries. The use of fascia relaxation, balance pads, and other methods could improve the movement ability and stability of the knee joint and reduce damage to the knee joint. At the same time, the strength of the muscles around the knee joint such as the quadriceps femoris could be strengthened through rehabilitation training such as resistance training of flexors and extensors and static squatting training by machine learning to prevent knee injuries. Therefore, machine learning is an effective method for rehabilitation training. However, due to the particularity of the athletes’ physiques, it was necessary to formulate corresponding rehabilitation training plans for different athletes during the recovery process. However, due to the limitation of time and technology, the specific causes of knee joint injuries have not been analyzed and discussed in detail in this paper, which would be further studied in the future.
The original contributions presented in the study are included in the article/Supplementary Material; further inquiries can be directed to the corresponding author.
The author confirms being the sole contributor of this work and has approved it for publication.
The author declares that the research was conducted in the absence of any commercial or financial relationships that could be construed as a potential conflict of interest.
All claims expressed in this article are solely those of the authors and do not necessarily represent those of their affiliated organizations, or those of the publisher, the editors, and the reviewers. Any product that may be evaluated in this article, or claim that may be made by its manufacturer, is not guaranteed or endorsed by the publisher.
1. Trobec R, Kosec G, Veselko M. A model for potential non-contact ski injuries of the knee. Sports Med Health Sci (2020) 2(3):126–31. doi:10.1016/j.smhs.2020.08.003
2. Snooker B, Turkiewicz A, Magnusson K, Frobell R, Englund M. Risk of knee osteoarthritis after different types of knee injuries in young adults: A population-based cohort study. Br J Sports Med (2019) 54(12):1–6.
3. Welsch GH, Behr AM, Frosch KH, Tahir E, Pachowsky M, Henes FO, et al. Semi-quantitative magnetic resonance imaging scoring of the knee detects previous injuries in professional soccer players. Knee Surg Sports Traumatol Arthrosc (2022) 30(4):1161–8. doi:10.1007/s00167-022-06897-5
4. Goreta R, Posavi M, Penga L, Vrdoljak D, Mili M. Knee injuries in Croatian soccer players of both gender. J Sports Sci (2021) 12(2):37–42.
5. Hinterwimmer F, Lazic I, Suren C, Hirschmann MT, Pohlig F, Rueckert D, et al. Machine learning in knee arthroplasty: Specific data are key—a systematic review. Knee Surg Sports Traumatol Arthrosc (2022) 30(2):376–88. doi:10.1007/s00167-021-06848-6
6. Smna B, Eyw B, Hsh B, Mam C, Vek D, Bmpm D, et al. Machine learning and primary total knee arthroplasty: Patient forecasting for a patient-specific payment model. The J Arthroplasty (2018) 33(12):3617–23. doi:10.1016/j.arth.2018.08.028
7. Verstraete MA, Moore RE, Roche M, Conditt MA. The application of machine learning to balance a total knee arthroplasty. Bone Jt Open (2020) 1(6):236–44. doi:10.1302/2633-1462.16.bjo-2020-0056.r1
8. Khalil A, Resnick D, Zeid A, Zaiton F. The role of magnetic resonance musculoskeletal imaging in evaluation of sports injuries of the knee joint. Zagazig Univ Med J (2017) 20(6):1–10. doi:10.21608/zumj.2017.4447
9. KompelAndrew J, Murakami A, M, Engebretsen L, Forster BB, Lotfi M, Jarraya M, et al. MRI-detected sports-related knee injuries and abnormalities at the rio de Janeiro 2016 summer olympic games. AJR: Am J Roentgenology: Including Diagn Radiol Radiat Oncol Nucl Med Ultrasonography Relat Basic Sci (2018) 211(4):880–6. doi:10.2214/ajr.17.19334
10. Gaal BT, Knapik DM, Gilmore A. Patient-reported outcome measures following surgical intervention for pediatric sports-related injuries to the knee: A systematic review. Curr Rev Musculoskelet Med (2022) 15(3):187–93. doi:10.1007/s12178-022-09756-5
11. Shinichi Y. Rehabilitation medicine for sports-related knee injuries and disorders. Jpn J Rehabil Med (2019) 56(12):1027–31.
12. Dosher WB, Maxwell GT, Warth RJ, Harner CD. Multiple ligament knee injuries: Current state and proposed classification. Clin Sports Med (2019) 38(2):183–92. doi:10.1016/j.csm.2018.11.006
13. Ackerman IN, Kemp JL, Crossley KM, Culvenor AG, Hinman RS. Hip and knee osteoarthritis affects younger people, too. J Orthop Sports Phys Ther (2017) 47(2):67–79. doi:10.2519/jospt.2017.7286
14. Fister I, Fister D, Deb S, Mlakar U, Brest J. Post hoc analysis of sport performance with differential evolution. Neural Comput Applic (2020) 32:10799–808. doi:10.1007/s00521-018-3395-3
15. Tayfur B, Charuphongsa C, Morrissey D, Miller SC. Neuromuscular function of the knee joint following knee injuries: Does it ever get back to normal? A systematic review with meta-analyses. Sports Med (2021) 51(2):321–38. doi:10.1007/s40279-020-01386-6
16. Gan C, Yang Y, Zhu Q, Kumar Jain D, StrucDHF-Net V. DHF-net: A hierarchical feature interactive fusion network for dialogue emotion recognition. Expert Syst Appl (2022) 210:118525. doi:10.1016/j.eswa.2022.118525
Keywords: knee sports injury diagnosis, machine learning, support vector machine, auxiliary diagnosis, sports injury diagnosis
Citation: Liu H (2023) Value evaluation of knee joint sports injury detection model-aided diagnosis based on machine learning. Front. Phys. 11:1166275. doi: 10.3389/fphy.2023.1166275
Received: 15 February 2023; Accepted: 23 May 2023;
Published: 09 June 2023.
Edited by:
Amrit Mukherjee, University of South Bohemia in České Budějovice, CzechiaReviewed by:
Chu Shiwei, Beijing Forestry University, ChinaCopyright © 2023 Liu. This is an open-access article distributed under the terms of the Creative Commons Attribution License (CC BY). The use, distribution or reproduction in other forums is permitted, provided the original author(s) and the copyright owner(s) are credited and that the original publication in this journal is cited, in accordance with accepted academic practice. No use, distribution or reproduction is permitted which does not comply with these terms.
*Correspondence: Hao Liu, bGl1aGFvQHh5dnRjLmVkdS5jbg==
Disclaimer: All claims expressed in this article are solely those of the authors and do not necessarily represent those of their affiliated organizations, or those of the publisher, the editors and the reviewers. Any product that may be evaluated in this article or claim that may be made by its manufacturer is not guaranteed or endorsed by the publisher.
Research integrity at Frontiers
Learn more about the work of our research integrity team to safeguard the quality of each article we publish.