- 1Center for Advanced Laser Technology, Hebei University of Technology, Tianjin, China
- 2Hebei Key Laboratory of Advanced Laser Technology and Equipment, Tianjin, China
Single-pixel imaging is a novel imaging technique that can obtain image information through a single-pixel detector. It can effectively avoid the problem of lack of high-quality area array detectors in the terahertz band, and has attracted the attention of a large number of researchers in recent years. In this paper, the basic imaging principles, terahertz beam modulation methods and typical image reconstruction algorithms for terahertz single-pixel imaging are introduced and discussed, as well as its research progresses and developing trends.
1 Introduction
Terahertz [1] (THz) waves (0.1–10 THz, 3 mm-30 um) have the characteristics of high penetration, low photon energy and spectral resolution, which makes terahertz imaging a widely technology used in various fields, such as nondestructive testing [2–4], safety monitoring [5–7] and medical applications [8–10].
According to different detection methods, terahertz imaging can be divided into array imaging and point-by-point scanning imaging [11]. Array imaging usually uses CCD cameras [12], CMOS cameras [13] or microbolometer cameras [14] as the detectors, which has advantages of high integration and real-time imaging. However, due to the limitation of THz array detectors, the resolution of array imaging cannot be very high. Point-by-point scanning imaging [15] scans the sample mechanically to obtain the value of every pixel, thereby realizing the imaging of the object. Its spatial resolution is higher than that of array imaging, but the scanning speed will limit the imaging efficiency. So it is impossible to realize high-speed imaging. Based on the shortcomings of the above imaging methods, some researchers applied single-pixel imaging in the field of terahertz imaging. Single-pixel imaging [16] is a new computational imaging technology, whose basic idea is to use a single-pixel detector to collect spatial intensity information of objects and then reconstruct the image using the correlation calculation of intensities between the collected light and the original light with a specific spatial distribution. Since only a single-pixel detector is needed to obtain the image of the object, the system structure can be simplified and the cost can be reduced, which provides a new method for terahertz imaging [17].
2 Basic principle
Single-pixel imaging is a computational imaging method that can be described by a mathematical model. An image can be regarded as a one-dimensional matrix
Where
When the measurement matrix adopts random matrix, it takes more measurement time to recover the object image with considerable quality.
3 Development of terahertz single-pixel imaging
According to the basic principle of single-pixel imaging, the key points is the spatial modulation of the beam, the selection of modulation matrix and the reconstruction algorithm of the image. In the view of the beam modulation methods and imaging algorithms, this section introduces the development of terahertz single-pixel imaging.
3.1 Spatial modulation of terahertz wave
The existing spatial light modulators, such as digital micromirror devices and liquid crystal spatial light modulations, generally work in visible and infrared bands, which cannot directly be used to modulate terahertz waves. Therefore, studying the spatial light modulation methods in the terahertz band is a very important topic to THz single-pixel imaging. Recently, researchers have put forward a great many different solutions to this challenge.
3.1.1 Metallic masks modulation
As metals have strong absorption and reflection properties for terahertz waves, it is an effective method to modulate terahertz waves by metal masks. As shown in Figure 1AChan et al. partially printed copper on the transparent PCB as a random mask [18] and combined it with the CS algorithm to achieve
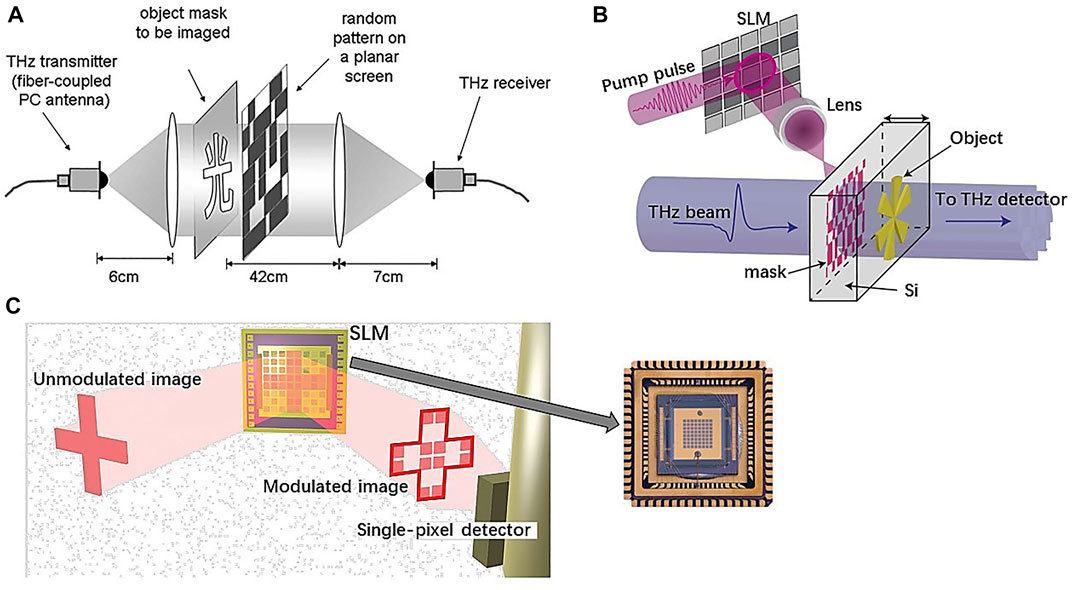
FIGURE 1. (A) Terahertz single-pixel imaging based on metal mask modulator [18], (B) Terahertz single-pixel imaging based on optically controlled semiconductor modulation [25], (C) Terahertz single-pixel imaging based on metamaterial modulation [38].
Some researchers have proposed the use of rotating masks to modulate terahertz waves. In 2012, the University of Liverpool [20] demonstrated for the first time that continuously rotating PCB circular disks with masks can be used to rapidly modulate terahertz. For terahertz imaging, 160 measurements (about 16%) can provide images with acceptable quality. Similarly, Vallés et al. [21] used metal ring with a series of direct perforation random masks to realize 2D imaging with high pixel resolution (1200 × 1200) in the entire terahertz frequency range (3–13 THz). Moreover, the Chinese Academy of Sciences uses the rotation of double amplitude modulation plates [22, 23] to generate reusable measurement matrices, which further improved the acquisition speed of the measurement matrix and imaging quality (7 mm resolution).
3.1.2 Optically controlled semiconductor modulation
In addition to the direct modulation of terahertz waves by metal mask, there is also photo-induced semiconductor modulation technology [23, 24] to be commonly used in recent years. As shown in Figure 1B, its modulation mechanism is that the area of the semiconductor illuminated by light will excite the internal photo-generated carriers, forming a transient region with high absorption or high reflection of terahertz waves, so that the modulation of terahertz waves is converted into modulation of the current carrier in the semiconductor. Therefore, the modulation of terahertz waves can be realized by combining traditional spatial light modulators and semiconductors. Because of its own characteristics, silicon has a band gap width suitable for laser pumping and it is the first time semiconductor material that has been proved to be used to realize optically controlled terahertz modulation devices. In addition, materials such as germanium and gallium arsenic have also been proved to be used as modulation semiconductors.
Busch et al. applied the modulation technology of optically pumped semiconductor materials (25% modulation depth) to terahertz beam control and imaging [25], and obtain a 64 × 64 pixels image of a cross-shaped aluminum sheet by using a series of masks. Hendry’s group [26] used spatially modulated femtosecond laser pulses to pump high-resistance Si, and achieved single-pixel imaging of terahertz with a spatial resolution of
To improve the modulation depth of silicon-based modulators, many researchers have used micro-nano structures to cover the silicon surface, such as graphene [30], metal gratings [31], and so on. Zhu et al. performed a comparative analysis of the THz imaging results between a micro-structure silicon modulator and a conventional high-resistance silicon modulator [32]. The results showed that the micro-structure greatly improved the absorption and utilization rate of silicon for laser and improved the imaging effect.
Graphene has also been proved to be useful for the modulation of terahertz waves due to its unique structure and high carrier mobility. Wen et al. designed a graphene based all-optical spatial terahertz modulator [33]. They transferred a layer of graphene on the top of doped germanium, and controlled the transmission of terahertz waves by irradiating the germanium substrate with a laser to generate carriers to diffuse into the graphene layer, thereby realizing the modulation of terahertz waves. The maximum modulation depth of the optically controlled modulator is as high as 90%. In addition, Shenzhen Institute of Advanced Technology used the mono-layer graphene on a high-resistance silicon substrate illuminated by a coded laser beam as a terahertz modulator to realize Fourier single-pixel terahertz imaging [34]. Though the sampling ratio is only 1.6%, the image reconstruction can be completed with a signal-to-noise ratio as large as 5.11. The modulation depth of graphene combined with semiconductor is still limited and its working frequency is narrow. Tianjin University [35] proposed to integrate sub-wavelength metallic grating into silicon on sapphire to design a high-efficiency, ultra-thin and fast THz SLM with high modulation depth (over 60% THz peak amplitude modulation depth at the pump flux of
3.1.3 Metamaterial modulation
Artificial metamaterials combined with tunable semiconductor structures can realize high-speed terahertz modulators. This class of modulators mainly involves fabricating metallic metamaterial structures on semiconductor materials and controls the resonant strength of the metamaterial structure by applying a bias voltage between the metamaterial and the substrate. In 2006, Chen et al. proposed a terahertz modulator [37] with a semiconductor metamaterial structure to obtain a modulation depth of 50% at 0.72 THz. As shown in Figure 1C, Padilla’s team proposed a reconfigurable terahertz spatial light modulator based on metamaterial absorber in 2014, which uses an electronically controlled
Terahertz SLMs (Spatial Light Modulator) are widely adopted in single-pixel imaging, in Table 1, we summarize the representative results of current THz single-pixel imaging. The metal mask controllability over terahertz is not strong, which affects the quality of reconstructed images. The photo-induced carrier concentration in photo-induced semiconductor modulation will directly affect the modulation efficiency. At present, it is difficult for the existing semiconductor materials to meet the requirements of modulation speed and modulation depth at the same time. Therefore, how to improve the carrier concentration in photo-induced semiconductors is a research hotpot. Artificial metamaterial modulation can better control the transmission of terahertz waves, but the design is complex and provides very few controllable pixels.
3.2 Imaging algorithm
Another important factor that affects the imaging quality of terahertz single-pixel is the post-imaging reconstruction algorithm. With the development of single-pixel imaging, many reconstruction algorithms have been proposed to improve imaging quality and efficiency, which can be broadly classified as follows: traditional terahertz single-pixel imaging algorithms developed from computational ghost imaging algorithms, single-pixel imaging algorithms based on compressed sensing, single-pixel imaging algorithms based on base scanning, single-pixel imaging algorithm based on deep learning, etc.
Computational ghost imaging based on spatial light modulation was proposed by Bromberg et al. [39] in 2008. In terms of imaging nature, conventional terahertz single-pixel imaging and computational ghost imaging are mathematically equivalent, as in Eq. 3, which uses an iterative approach for image reconstruction based on correlation algorithms.
It can be seen from the formula that the reconstructed image is equivalent to a weighted sum of modulation masks, where the weight is the detection value of a single-pixel. Usually, this method can only obtain images with high SNR for a larger number of measurements (
In order to overcome the problems of large amount of sampled data and long time in imaging, some studies have proposed combining compressed sensing (CS) techniques with optically controllable terahertz spatial light modulators in single-pixel imaging. Compressed sensing theory [42–44] was first proposed in 2006 by Candès and Donoho et al. It breaks away from the traditional Nyquist sampling theorem by exploiting the sparsity of natural images in the orthogonal transform domain to accurately reconstruct the original target image using a small number of sampled measurements. The CS theory solves the problem of solving the uncertain inverse problem with more unknown values than the available data. In single-pixel imaging [17], this means that the number of mask patterns is less than the total number of pixels used to restore the image, which provides a new method for single-pixel imaging. After the CS theory was proposed, Rice University [45] conducted a single-pixel imaging experiment using the theory, which used a digital micromirror array to modulate visible light, combined the pre-designed observation matrix with the one-dimensional measurements obtained from the single-pixel detector, and then used the compressed sensing reconstruction algorithm to obtain the original image.
Although the introduction of CS algorithms [38, 46, 47] reduces the number of samples and effectively reduces the imaging time, it requires a long time for computation and high computational complexity in the image reconstruction process. In addition to the random matrix modulation, terahertz single-pixel imaging can also be modulated with deterministic orthogonal bases, such as Fourier bases [34, 48, 49], Hadamard bases [50–52], discrete cosine bases [53], and wavelet transform bases [54, 55], etc. This imaging method is also known as base-scan terahertz single-pixel imaging. Since these substrate patterns are orthogonal, by determining the illumination projection of the patterns, the spatial information of the object image in the transform domain can be obtained and the corresponding inverse transformation can be performed to recover the target image. In addition, taking advantage of the sparsity of natural images in the transform domain, it is possible to reconstruct clear images using under-sampled data by measuring coefficients of larger amplitude.
Although the base-scan terahertz single-pixel imaging approach effectively solves the problem of long data acquisition time, the sampling frequency is subjectively selected in the sampling process, which leads to selective loss of image information and does not always exactly match the frequency distribution of the image. In recent years, many researchers have demonstrated the advantages of deep learning [56–63] in the field of single-pixel imaging, which is widely used in the fields of information encryption [64] and image super-resolution [65]. For single-pixel imaging, the image quality is proportional to the number of samples, and how to get effective recovery of the target image under low sampling is an important issue nowadays. Combining deep learning with single-pixel imaging and relying on the deep learning ability of the model can obtain better image quality. In 2017, Lyu et al. applied deep learning [66] to a traditional computational ghost imaging algorithm by using a large number of reconstructed low-quality images at low sampling rates as network inputs and the original images as labels for the network, and iteratively trained the network to obtain high-quality target images at 10% sampling rate. In addition, some researchers used the single-pixel detection signal sequence as the input of the neural network and the image reconstruction result as the output of the neural network to train the neural network to achieve deep learning single-pixel imaging [67]. In terahertz waves single-pixel imaging, most of the existing research uses deep learning techniques to improve the quality of imaging [68–70], and it is also a research direction to investigate how to train modulation masks suitable for terahertz single-pixel imaging to improve the imaging speed in response to the current problems.
4 Discussion and conclusion
Applying single-pixel imaging technique to THz imaging can effectively solve some problems existing in traditional method. With the continuous development of spatial modulation techniques, many terahertz single-pixel imaging techniques have been investigated as described above. This paper summarizes the initial physical metal masks to later methods based on light modulation in semiconductors and artificial metamaterial modulation. In terms of imaging algorithms, the main imaging algorithms applied to terahertz single-pixel imaging are introduced, including the traditional terahertz single-pixel imaging algorithm developed from computational ghost imaging algorithm, single-pixel imaging algorithm based on compressed sensing, single-pixel imaging algorithm based on basic scanning and single-pixel imaging algorithm based on deep learning. At present, how to improve the sampling speed to achieve real-time imaging while ensuring the image SNR is the problem that needs to be solved for terahertz single-pixel imaging. In addition, further improvement of terahertz modulation techniques and exploration of more optimized algorithms are the two most important research directions for terahertz single-pixel imaging.
Author contributions
QH: investigation, writing—original draft. XW: investigation, writing—review and editing. YP: investigation, writing—original draft, writing—review and editing, supervision. LL: investigation, writing—review and editing.
Funding
This work was funded by the Key R&D Program of Hebei Province (20371802D), the National Natural Science Foundation of China (61905063), and the Natural Science Foundation of Hebei Province (F2020202055).
Conflict of interest
The authors declare that the research was conducted in the absence of any commercial or financial relationships that could be construed as a potential conflict of interest.
Publisher’s note
All claims expressed in this article are solely those of the authors and do not necessarily represent those of their affiliated organizations, or those of the publisher, the editors and the reviewers. Any product that may be evaluated in this article, or claim that may be made by its manufacturer, is not guaranteed or endorsed by the publisher.
References
1. Siegel PH. Terahertz technology. IEEE Trans Microwave Theor Techn (2002) 50:910–28. doi:10.1109/22.989974
2. Wang J, Zhang J, Chang T, Liu L, Cui HL. Terahertz nondestructive imaging for foreign object detection in glass fibre-reinforced polymer composite panels. Infrared Phys Technol (2019) 98:36–44. doi:10.1016/j.infrared.2019.02.003
3. Zhang D, Ren J, Gu J, Li LJ, Zhang JY, Xiong WH, et al. Nondestructive testing of bonding defects in multilayered ceramic matrix composites using THz time domain spectroscopy and imaging. Compos Structures (2020) 251:112624. doi:10.1016/j.compstruct.2020.112624
4. Zhang X, Chang T, Wang Z, Cui HL. Three-dimensional terahertz continuous wave imaging radar for nondestructive testing. IEEE Access (2020) 8:144259–76. doi:10.1109/access.2020.3014170
5. Tong H, Pei S, Jiang L, Zhu Y, Xiao L Seventh international green and sustainable computing conference (IGSC). Hangzhou, China: IEEE (2016). 1–5.A low-power-consumption and high efficiency security system for automatic detection of concealed objects in human body
6. Hu S, Chen X, Alfadhl Y. 43rd international conference on infrared, millimeter, and terahertz waves (IRMMW-THz). Nagoya: IEEE (2018). 1–2.A THz imaging system using sparse antenna array for security screening
7. Tzydynzhapov G, Gusikhin P, Muravev V, Dremin A, Nefyodov Y, Kukushkin I. New real-time sub-terahertz security body scanner. J Infrared Millim Terahertz Waves (2020) 41(6):632–41. doi:10.1007/s10762-020-00683-5
8. Yang X, Zhao X, Yang K, Liu Y, Liu Y, Fu W, et al. Biomedical applications of terahertz spectroscopy and imaging. Trends Biotechnol (2016) 34:810–24. doi:10.1016/j.tibtech.2016.04.008
9. Cao Y, Huang P, Li X, Ge W, Hou D, Zhang G. Terahertz spectral unmixing based method for identifying gastric cancer. Phys Med Biol (2018) 63:035016. doi:10.1088/1361-6560/aa9e1a
10. Rong L, Latychevskaia T, Chen C, Wang D, Yu Z, Zhou X, et al. Terahertz in-line digital holography of human hepatocellular carcinoma tissue. Sci Rep (2015) 5:8445. doi:10.1038/srep08445
11. Guerboukha H, Nallappan K, Skorobogatiy M. Toward real-time terahertz imaging. Adv Opt Photon (2018) 10:843–938. doi:10.1364/aop.10.000843
12. Trichopoulos GC, Mosbacker HL, Burdette D, Sertel K. A broadband focal plane array camera for real-time THz imaging applications. IEEE Trans Antennas Propagat (2013) 61:1733–40. doi:10.1109/tap.2013.2242829
13. Schuster F, Coquillat D, Videlier H, Sakowicz M, Teppe F, Dussopt L, et al. Broadband terahertz imaging with highly sensitive silicon CMOS detectors. Opt Express (2011) 19:7827–32. doi:10.1364/oe.19.007827
14. Simoens F, Meilhan J, Gidon S, Lasfargues G, Lalanne Dera J, Ouvrier-Buffet JL, et al. Antenna-coupled microbolometer based uncooled 2D array and camera for 2D real-time terahertz imaging. Proc SPIE 8846 Terahertz Emitters, Receivers, Appl (2013) IV:88460O.
15. Hu B, Nuss MC. Imaging with terahertz waves. Opt Lett (1995) 20:1716–8. doi:10.1364/ol.20.001716
16. Edgar MP, Gibson GM, Padgett MJ. Principles and prospects for single-pixel imaging. Nat Photon (2019) 13:13–20. doi:10.1038/s41566-018-0300-7
17. Duarte MF, Davenport MA, Takhar D, Laska JN, Sun T, Kelly KF, et al. Single-pixel imaging via compressive sampling. IEEE Signal Process Mag (2008) 25:83–91. doi:10.1109/msp.2007.914730
18. Chan WL, Charan K, Takhar D, Kelly KF, Baraniuk RG, Mittleman DM. A single-pixel terahertz imaging system based on compressed sensing. Appl Phys Lett (2008) 93:121105. doi:10.1063/1.2989126
19. Duan P, Wang Y, Xu D, Yan C, Yang Z, Xu W, et al. Single pixel imaging with tunable terahertz parametric oscillator. Appl Opt (2016) 55:3670–5. doi:10.1364/ao.55.003670
20. Shen H, Gan L, Newman N, Dong Y, Li C, Huang Y, et al. Spinning disk for compressive imaging. Opt Lett (2012) 37:46–8. doi:10.1364/ol.37.000046
21. Vallés A, He J, Ohno S, Omatsu T, Miyamoto K. Broadband high-resolution terahertz single-pixel imaging. Opt Express (2020) 28:28868–81. doi:10.1364/oe.404143
22. Yan H, Zhao C, Xu W, Li Shuofeng . Terahertz ghost imaging based on imaging-transformation measurement matrices. Chin. J. Lasers (2019) 46:1214001. (in Chinese). doi:10.3788/cjl201946.1214001
23. Shang Y, Wang X, Sun W, Han P, Ye J, Feng S, et al. Terahertz image reconstruction based on compressed sensing and inverse Fresnel diffraction. Opt Express (2019) 27:14725–35. doi:10.1364/oe.27.014725
24. Shrekenhamer D, Watts CM, Padilla WJ. Terahertz single pixel imaging with an optically controlled dynamic spatial light modulator. Opt Express (2013) 21(10):12507–18. doi:10.1364/oe.21.012507
25. Busch S, Scherger B, Scheller M, Koch M. Optically controlled terahertz beam steering and imaging. Opt Lett (2012) 37:1391–3. doi:10.1364/ol.37.001391
26. Stantchev RI, Sun B, Hornett SM, Hobson PA, Gibson GM, Padgett MJ, et al. Noninvasive, near-field terahertz imaging of hidden objects using a single-pixel detector. Sci Adv (2016) 2(6):e1600190. doi:10.1126/sciadv.1600190
27. Stantchev RI, Phillips DB, Hobson P, Hornett SM, Padgett MJ, Hendry E. Compressed sensing with near-field THz radiation. Optica (2017) 4:989–92. doi:10.1364/optica.4.000989
28. Stantchev RI, Yu X, Blu T, Pickwell-MacPherson E. Real-time terahertz imaging with a single-pixel detector. Nat Commun (2020) 11(1):2535. doi:10.1038/s41467-020-16370-x
29. Zanotto L, Piccoli R, Dong J, Caraffini D, Morandotti R, Razzari L. Time-domain terahertz compressive imaging. Opt Express (2020) 28:3795–802. doi:10.1364/oe.384134
30. Mittendorff M, Li S, Murphy TE. Graphene-based waveguide-integrated terahertz modulator. ACS Photon (2017) 4:316–21. doi:10.1021/acsphotonics.6b00751
31. Wen T, Zhang D, Wen Q, Liao Y, Zhang C, Li J, et al. Enhanced optical modulation depth of terahertz waves by self-assembled monolayer of plasmonic gold nanoparticles. Adv Opt Mater (2016) 4:1974–80. doi:10.1002/adom.201600248
32. Zhu Y, Shen Z, Zhang H, He Y, Wen Q Fast imaging technology of terahertz wave based on spatial modulator. J Terahertz Sci Electron Inf Tech (2020) 18(3):351∼357. (in Chinese).
33. Wen Q, Tian W, Mao Q, Chen Z, Liu WW, Yang QH, et al. Graphene based all-optical spatial terahertz modulator. Sci Rep (2015) 4:7409. doi:10.1038/srep07409
34. She R, Liu W, Lu Y, Zhou Z, Li G. Fourier single-pixel imaging in the terahertz regime. Appl Phys Lett (2019) 115:021101. doi:10.1063/1.5094728
35. Yang X, Tian Z, Chen X, Hu M, Yi Z, Ouyang C, et al. Terahertz single-pixel near-field imaging based on active tunable subwavelength metallic grating. Appl Phys Lett (2020) 116:241106. doi:10.1063/5.0010531
36. Chen S, Du L, Meng K, Li J, Zhai ZH, Shi QW, et al. Terahertz wave near-field compressive imaging with a spatial resolution of over λ/100. Opt Lett (2019) 44:21–4. doi:10.1364/ol.44.000021
37. Chen H, Padilla WJ, Zide JMO, Gossard AC, Taylor AJ, Averitt RD. Active terahertz metamaterial devices. Nature (2006) 444:597–600. doi:10.1038/nature05343
38. Watts CM, Shrekenhamer D, Montoya J, Lipworth G, Hunt J, Sleasman T, et al. Terahertz compressive imaging with metamaterial spatial light modulators. Nat Photon (2014) 8:605–9. doi:10.1038/nphoton.2014.139
39. Bromberg Y, Katz O, Silberberg Y. Ghost imaging with a single detector. Phys Rev A (Coll Park) (2009) 79(5):053840. doi:10.1103/physreva.79.053840
40. Ferri F, Magatti D, Lugiato LA, Gatti A. Differential ghost imaging. Phys Rev Lett (2010) 104:253603. doi:10.1103/physrevlett.104.253603
41. Sun B, Welsh SS, Edgar MP, Shapiro JH, Padgett MJ. Normalized ghost imaging. Opt Express (2012) 20:16892–901. doi:10.1364/oe.20.016892
42. Candes EJ, Romberg J, Tao T. Robust uncertainty principles: Exact signal reconstruction from highly incomplete frequency information. IEEE Trans Inform Theor (2006) 52:489–509. doi:10.1109/tit.2005.862083
43. Tsaig Y, Donoho DL. Extensions of compressed sensing. Signal Process. (2006) 86:549–71. doi:10.1016/j.sigpro.2005.05.029
44. Donoho DL. Compressed sensing. IEEE Trans Inform Theor (2006) 52:1289–306. doi:10.1109/tit.2006.871582
45. Takhar D, Laska J, Wakin M, Duarte MF, Baraniuk RG Proc IS&T/SPIE symposium on electronic imaging (2006).A new Compressive Imaging camera architecture using optical-domain compression
46. Clemente P, Durán V, Tajahuerce E, Andres P, Climent V, Lancis J. Compressive holography with a single-pixel detector. Opt Lett (2013) 38(14):2524–7. doi:10.1364/ol.38.002524
47. Zhao C, Gong W, Chen M, Li E, Wang H, Xu W, et al. Ghost imaging lidar via sparsity constraints. Appl Phys Lett (2012) 101:141123. doi:10.1063/1.4757874
48. Zhang Z, Ma X, Zhong J. Single-pixel imaging by means of Fourier spectrum acquisition. Nat Commun (2015) 6:6225. doi:10.1038/ncomms7225
49. Zhang Z, Wang X, Zheng G, Zhong J. Hadamard single-pixel imaging versus Fourier single-pixel imaging. Opt Express (2017) 25:19619. doi:10.1364/oe.25.019619
50. Wang L, Zhao S. Fast reconstructed and high-quality ghost imaging with fast Walsh–Hadamard transform. Photon Res (2016) 4(6):240–4. doi:10.1364/prj.4.000240
51. Radwell N, Mitchell KJ, Gibson GM, Edgar MP, Bowman R, Padgett MJ. Single-pixel infrared and visible microscope. Optica (2014) 1:285–9. doi:10.1364/optica.1.000285
52. Vasile T, Damian V, Coltuc D, Petrovici M. Single pixel sensing for THz laser beam profiler based on Hadamard Transform. Opt Laser Tech (2016) 79:173–8. doi:10.1016/j.optlastec.2015.12.009
53. Liu BL, Yang ZH, Liu X, Wu LA. Coloured computational imaging with single-pixel detectors based on a 2D discrete cosine transform. J Mod Opt (2017) 64(3):259–64. doi:10.1080/09500340.2016.1229507
54. Li M, Yan L, Yang R, Liu YX. Fast single-pixel imaging based on optimized reordering Hadamard basis. Acta Phys Sin (2019) 68(6):064202. doi:10.7498/aps.68.20181886
55. Alemohammad M, Stroud JR, Bosworth BT, Foster MA. High-speed all-optical Haar wavelet transform for real-time image compression. Opt Express (2017) 25(9):9802–11. doi:10.1364/oe.25.009802
56. Shimobaba T, Endo Y, Nishitsuji T, Takahashi T, Nagahama Y, Hasegawa S, et al. Computational ghost imaging using deep learning. Opt Commun (2018) 413:147–51. doi:10.1016/j.optcom.2017.12.041
57. Rizvi S, Cao J, Zhang K, Hao Q. DeepGhost: Real-time computational ghost imaging via deep learning. Sci Rep (2020) 10:11400. doi:10.1038/s41598-020-68401-8
58. Sui L, Zhang L, Cheng Y, Zhaolin X, Ailing T, Anand A. Computational ghost imaging based on the conditional adversarial network. Opt Commun (2021) 492:126982. doi:10.1016/j.optcom.2021.126982
59. Wu H, Wang R, Zhao G, Xiao H, Liang J, Wang D, et al. Deep-learning denoising computational ghost imaging. Opt Lasers Eng (2020) 134:106183. doi:10.1016/j.optlaseng.2020.106183
60. Wang F, Wang H, Wang H, Li G, Situ G. Learning from simulation: An end-to-end deep-learning approach for computational ghost imaging. Opt Express (2019) 27:25560. doi:10.1364/oe.27.025560
61. He Y, Wang G, Dong G, Zhu S, Chen H, Zhang A, et al. Ghost imaging based on deep learning. Sci Rep (2018) 8:6469. doi:10.1038/s41598-018-24731-2
62. Zhang L, Bian Z, Ye H, Zhang D, Wang K. Research on photon-level ghost imaging restoration based on deep learning. Opt Commun (2022) 504:127479. doi:10.1016/j.optcom.2021.127479
63. Wu H, Wang R, Zhao G, Xiao H, Wang D, Liang J, et al. Sub-Nyquist computational ghost imaging with deep learning. Opt Express (2020) 28:3846. doi:10.1364/oe.386976
64. Sui L, Du C, Xu M, Ailing T, Anand A. Information encryption based on the customized data container under the framework of computational ghost imaging. Opt Express (2019) 27(12):16493–506. doi:10.1364/oe.27.016493
65. Tian C, Fei L, Zheng W, Xu Y, Zuo W, Lin CW. Deep learning on image denoising: An overview. Neural Networks (2020) 131:251–75. doi:10.1016/j.neunet.2020.07.025
66. Lyu M, Wang W, Wang H, Li G, Chen N, et al. Deep-learning-based ghost imaging. Sci Rep (2017) 7(1):17865–6. doi:10.1038/s41598-017-18171-7
67. Shang R, Hoffer-Hawlik K, Wang F, Situ G, Luke GP. Two-step training deep learning framework for computational imaging without physics priors. Opt Express (2021) 29(10):15239–54. doi:10.1364/oe.424165
68. Wang Y, Qi F, Wang J. Terahertz image super-resolution based on a complex convolutional neural network. Opt Lett (2021) 46(13):3123–6. doi:10.1364/ol.422684
69. Li Y, Hu W, Zhang X, Xu Z, Ni J, Ligthart LP. Adaptive terahertz image super-resolution with adjustable convolutional neural network. Opt Express (2020) 28(15):22200–17. doi:10.1364/oe.394943
Keywords: terahertz imaging, single-pixel imaging, terahertz beam modulation, compressed sensing, reconstruction algorithm
Citation: Hu Q, Wei X, Pang Y and Lang L (2022) Advances on terahertz single-pixel imaging. Front. Phys. 10:982640. doi: 10.3389/fphy.2022.982640
Received: 30 June 2022; Accepted: 08 August 2022;
Published: 30 August 2022.
Edited by:
Zhi-Han Zhu, Harbin University of Science and Technology, ChinaCopyright © 2022 Hu, Wei, Pang and Lang. This is an open-access article distributed under the terms of the Creative Commons Attribution License (CC BY). The use, distribution or reproduction in other forums is permitted, provided the original author(s) and the copyright owner(s) are credited and that the original publication in this journal is cited, in accordance with accepted academic practice. No use, distribution or reproduction is permitted which does not comply with these terms.
*Correspondence: Yajun Pang, eWpwYW5nQGhlYnV0LmVkdS5jbg==; Liying Lang, bGFuZ2xpeWluZ0BoZWJ1dC5lZHUuY24=