- 1Department of Mathematics, COMSATS University Islamabad, Attock Campus, Attock, Pakistan
- 2Department of Mathematics and Statistics, American University of Sharjah, Sharjah, United Arab Emirates
- 3Future Technology Research Center, National Yunlin University of Science and Technology, Douliou, Taiwan
- 4Department of ECE, COMSATS University Islamabad, Islamabad, Pakistan
- 5Department of Mathematics, College of Arts and Science, Prince Sattam bin Abdulaziz University, Al-Kharj, Saudi Arabia
- 6Department of Mathematics, Riphah International University Islamabad, Islamabad, Pakistan
The application of hybrid nanomaterials for the improvement of thermal efficiency of base fluid has increasingly gained attention during the past few decades. The basic purpose of this study is to investigate the flow characteristics along with heat transfer in an unsteady three-dimensional flow of hybrid nanofluid over a stretchable and rotatory sheet (3D-UHSRS). The flow model in the form of PDEs was reduced to the set of ordinary differential equations utilizing the appropriate transformations of similarity. The influence of the rotation parameter, unsteadiness parameter, stretching parameter, radiation parameter, and Prandtl number on velocities and thermal profile was graphically examined. A reference solution in the form of dataset points for the 3D-UHSRS model are computed with the help of renowned Lobatto IIIA solver, and this solution is exported to MATLAB for the proper implementation of proposed solution methodology based on the Levenberg–Marquardt supervised neural networks. Graphical and numerical results based on the mean square error (MSEs), time series response, error distribution plots, and regression plots endorses the precision, validity, and consistency of the proposed solution methodology. The MSE up to the level of 10–12 confirms the accuracy of the achieved results.
Introduction
In the recent past, improvement in heat transfer for various engineering and industrial applications remains the main topic of research for scientists. Improvement in the heat transfer ability can be done through different methods such as use of microchannel, extended surface and through vibrating the surface. Thermal conductivity plays the most important role among all other characteristics of fluid for judging its heat transfer ability. Thermal conductivity of any nanofluid depends on the type, as well as the shape of nanoparticles. There will be less energy loss with the improved thermal efficiency of the fluid, which can further reduce the cost and increase the production in industrial applications. Various conventional fluids, such as water, kerosene oil, and ethylene glycol, are commonly used in many industrial and engineering applications (e.g., chemical manufacturing, water distillation, HVAC systems, medicine, and power-generation systems), but due to inadequate thermal transfer ability, these ordinary fluids lack good efficiency in systems having large thermal transfer requirements. As it is well known, the ability to conduct heat for metals is hundred times greater than that in liquids, which is why it is appropriate to use them in thermal systems [1–5]. Thus, the use of nanoparticles <100 nm not only improved the thermal capabilities of the fluid, but also positively improved the other rheological properties. Initially [6] was the first work to discuss the nature and characteristics of nanofluids. [7] compared the performance of heat transfer between the nanofluid comprised of a single wall and multiwall carbon nanotubes in a magnetohydrodynamic (MHD) flow under the influence of radiative flux.
A new type of nanofluids known as “hybrid nanofluids” is made by dispersing two different types of nanoparticles into base fluid to obtain various synergetic effect of both types of nanomaterial. The development of hybrid nanofluidic system is currently undergoing and under the evaluation to enhance the strength of thermal effects for different flow dynamics. Performance in terms of thermal energy utilization is being tested and assessed by various researchers. Due to its marvelous heat transfer abilities, this new type of nanofluid attracted the attention of researchers and scientists to investigate it in many of the industrial and engineering problems. Hybrid nanofluid have extensive range of application in scientific, industrial, engineering, and medical fields like medicine manufacturing, heating systems, transfer cooling, electronic chips, and solar panels [8]. Of late, several studies have been conducted to analyze the improvement of heat transfer capabilities for hybrid nanofluids as compared to conventional single-particle nanofluids [9–13]. The flow over rotating surfaces has many practical applications such as electrical appliances, cutting discs, data-storing devices, and heavy machinery parts. Song et al. [14] implemented a numerical shooting methodology to analyze the effects of various physical parameters on the velocity and thermal fields in the stagnation point flow of a hybrid nanofluid over a rotatory disc under radiative and activation energy effects. [15] numerically evaluated the three-dimensional MHD flow of hybrid nanofluid over a rotating and stretching sheet. In addition, the behavior of velocity and temperature profile depending on different physical factors has been highlighted through graphs and numerical results.
Any flow in which all of its parameters are independent of time is known as steady flow, whereas the time-dependent flow is known as unsteady flow. Due to excessive applicability in engineering and industrial applications, steady flow has gained a lot of concentration of researchers and scholars. However, recently many studies have been conducted on the behavior and characteristics of unsteady fluid flow systems, automobiles, power-generation systems, and aviation industry [16], explored the influence of variable thermal conductivity, viscosity, and Joule heating on the velocity and thermal field in the unsteady flow of 3D Maxwell nanofluid over a stretching surface. An enhancement in the velocity of the fluid has been observed with increased viscosity. [17] numerically investigated the biconvection flow of cross nanofluid and studied the effects of thermal radiation along with melting phenomenon over a cylinder. [18] studied the considerable effect of external magnetic field acting at an inclined angle on Williamson’s nanofluid over a rotating stretchable surface. [19] conducted a comparative analysis of heat transfer over a porous stretching surface between nanofluid containing nanoparticles of Graphene oxide and the combination of Ag–graphene oxide in kerosene oil as base fluid.
[20] described experimental research of various factors responsible for achieving better thermophysical properties and more stable results in terms of heat transfer in nanofluids and ionanofluids. There are a variety of nanoparticles available for manufacturing of nanofluids and hybrid nanofluid. Each type of material has its own benefits and limitations depending on the material’s characteristics. [21] presented the importance and significance of Aluminum nanoparticles in the industrial and engineering fields and their various advantages over other renowned nanomaterials. [22] explicated an analysis to show the physical and chemical stability and thermophysical properties based on thermal conductivity for the nanofluid comprising TiO2 nanoparticles. Furthermore, different experimental results showing the improvement in thermal properties have also been tabulated in the research. [23] presented a numerical investigation to examine the flow behavior, as well as heat transmission capabilities of a hybrid fluid over a gyrating surface under the influence of a uniform external magnetic field. [24] numerically explained the behavior of mass and heat transfer for the MHD flow of a nanofluid over stretched surface under the heating effects through the radiation phenomenon. [25] used state-of-the-art supervised neural network to solve the hybrid nanofluid flow model with Joule heating and MHD effects. A comparison with an already available solution has also been made to show the accuracy and performance of solution methodology. Among several other key factors, the concentration of nanoparticles also plays an important part in deciding the thermal capability of nanofluids. [26] presented experimental research showing a rapid increase in thermal efficiency of an oil-based nanofluid. In a similar manner, [27] explained the fact that for a nanofluid with TiO2 nanoparticles in water, the negative effect of using a lower concentration of nanoparticles can be overcome by the other important properties such as critical heat flux. [28] utilized the Laplace transformation method to solve the flow model of an incompressible non-Newtonian hybrid nanofluid over permeable surface revolving with uniform acceleration with velocity and thermal slip effects. [29] conducted a numerical analysis of activation energy for the two-dimensional flow of a hybrid nanofluid under the effect of buoyancy force and thermal radiation. Furthermore, the influence of key parameters, such as the Nusselt number and Sherwood number, on the heat and velocity profiles has also been investigated.
Most of the researcher used conventional solution methodologies to explain various fluid models describing the effects of entropy generation, rotating flow problems, and Joule heating [30–36]. The application of modern solution methodologies based on artificial neural networks to solve such problems is an inventive work. Of late, researcher applied these modern solutions depending on artificial intelligence to resolve the problem related to various field such as financial trading [37], rainfall prediction models [38], bioinformatics [39, 40], fluid dynamics [41, 42], energy, HIV virus spread models [40, 43, 44], and coronavirus perdition model [45] (also see [46, 47, 49]). In this study, the authors intended to solve the 3D-UHSRS fluid problem for the first time, to the best of our knowledge, by utilizing the Levenberg–Marquardt supervised neural networks (LM-SNNs) bases solution technique. Theses modern solution approaches were based on state-of-the-art computational algorithms that can easily tackle the nonlinear behavior of flow model’s equations.
The basic purpose of this research work is to investigate and explain the flow feature along with the heat and mass transfer in 3D-UHSRS. The basic feature of purposed solution methodology for 3D-UHSRS are as follows:
• A state-of-the-art mathematical model for the 3D-UHSRS problem has been developed and expressed in terms of PDEs, which are further converted into a set of nonlinear ordinary differential equations (ODEs) using dimensionless similarity variables.
• A numerical solution of the 3D-UHSRS problem was obtained by implementing the Lobatto IIIA solution methodology based on the bvp4c solver in MATLAB. The solution datasets of the 3D-UHSRS problem is then subjected for LM back propagation to carry out the training, validation, and testing of the data set points.
• Different plots describing the effect of physical constants on velocity as well as thermal profile have been presented. The performance, convergence, and accuracy of the solution approach were validated thorough residual error, the number of grid points, ODEs, and BCs evaluations.
• Numerical and graphical results in the form of the MSE curve, error histograms, regression and error plots were authenticated from the performance, precision, and convergence of the proposed LM-SNNs methodology.
Problem Formulation
Assume a 3D unsteady flow of a hybrid nanofluid over a stretchable surface, as shown as Figures 1and 2. The surface is stretched in the
According to abovementioned assumptions, described flow model can be expressed in term of following set of mathematical equations (50) and (51):
In the above system of equations,
Here
Using the value of
Along with the following boundary conditions of the system:
Here, “c” is the stretching coefficient of the surface,
In the above equations,
Values regarding density, electrical, and thermal conductivity and specific heat against used nanoparticles and base fluid has been mentioned in Table 2.
Following important physical terminologies representing the skin friction and Nusselt number can be written as:
The mathematical evaluation of Eqs. 1 and 2)–5 gives
Here, corresponding BCs are
The physical parameters of 3D-UHSRS flow problem involved in Eqs. 8–12 are
Here,
in which
Results and Discussion
Solution of the system of ODE resenting the flow model has been accomplished in two major phases, in the first phase, set of nonlinear ODEs along with their relevant boundary conditions (Eqs. (1)–(7) are transformed into the first-order ODEs for the solution by using the renowned solution technique “Lobatto IIIA” with the use of MATLAB software. Graphical variation of velocity and temperature profile against various important physical parameters are shown in Figures 3 and 4. Various scenarios and cases are generated based on the variation of involved physical parameters, as described in Table 3.
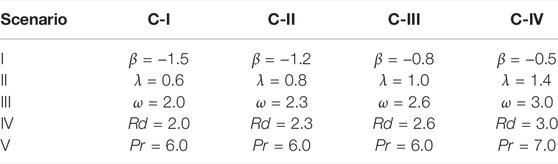
TABLE 3. Variation of parameters for the unsteady three-dimensional flow of hybrid nanofluid over a stretchable and rotatory sheet problem.
Figure 3A ∼ c) presented the impact of
Figures 4A,B presents the influence of
In the second phase, numerical results of the solution containing datasets for each involved variable against all scenarios and cases are generated between a fixed domain, and these dataset points are further subjected to the proper execution of innovative LM-SNNs-based solution. During the operation of proposed methodology out of total points, 80% points were subjected under the network training, whereas the leftover 10% dataset points were used for the operation of validation and testing processes. The number of neuron and delay steps in the calculations were adjusted as per complexity of the problem and required level of accuracy. A two-layer internal structure of the LM-SNNs is presented in Figure 5. Table 4–8 presents the numerical performance indicators comprising of MSE, gradient, Mu, and the number of epochs for scenario (I–V), respectively.
Figure 6A ∼ d) presents the graphical details of the time series response, error distribution plot, MSE, and gradient plots against the second case of the first scenario. Furthermore, MSE for any computational methodology represents the mean of square of differences between the actual value and estimated value. Accuracy and stability of any method can be judged through the MSE value encountered during the computational process. Smaller MSE values correspond to a better solution and an accurate and reliable solution technique. Figures 7A–D were designed to illustrate the MSE plots of the first case of each scenario for training, validation, and testing to compare performances based on MSE for each case.
The reference solution of the problem is available in the form of dataset points that are further categorized into training, validation, and testing processes at a specific ratio. In time series response, a close relationship between target and output values of training, validation, and testing depict the accuracy and precision of the solution methodology. Time series response of any variable is the statistical measure of any characteristics with respect to time. In addition, time series response help the researchers to estimate and understand the performance of a variable through any calculated data. Through these fitness plots one can easily observe the separate accuracy of all training, validation, and testing points as compared to available graphical solution. Figures 8A–D illustrate the fitness plots for first cases of all scenarios.
Error histograms are another way to measure the closeness of predicted values with the reference values. These histograms are actually the distribution of errors of all these computed values from a zero error point. Errors in term of all the achieved values are classified into 20 portions, which are aligned across a line representing the zero error line. More values that lie close to the zero error line indicate more accuracy and precision of the solution methodology. Figures 9A–D were designed to illustrate a comparison of error histograms for first cases of all scenarios.
Regression is a graphical way to present the precision of the predicted values to the reference values separately for training, validation and testing points. In these plots, an available reference solution has been shown by a straight line, whereas the predicted values are shown by dots or small circles. Accuracy and precision of the computation can also be judged through the numerical value of regression. R = 1 means that the predicted values are very close to reference values, and R = 0 means there is a very poor relationship between reference and predicted values. Figures 10A–D exhibit a comparison of regression plots between first cases of all scenarios.
Gradient is actually a vector responsible for guiding the network in the right direction with an accurate magnitude to reach the required solution as early as possible, whereas mu is a factor that controls a certain algorithm. The value of mu directly portraits the convergence of the solution. Figures 11A–D reflect a comparison between the gradient and mu plots of first cases of all scenarios.
Figure 12 portrays the graphic comparison of proposed LM-SNNs base solutions with the already available solutions for all scenarios, and additional comparison of errors for all cases of each scenario are also placed opposite showing the accuracy and precision of the proposed solution methodology.
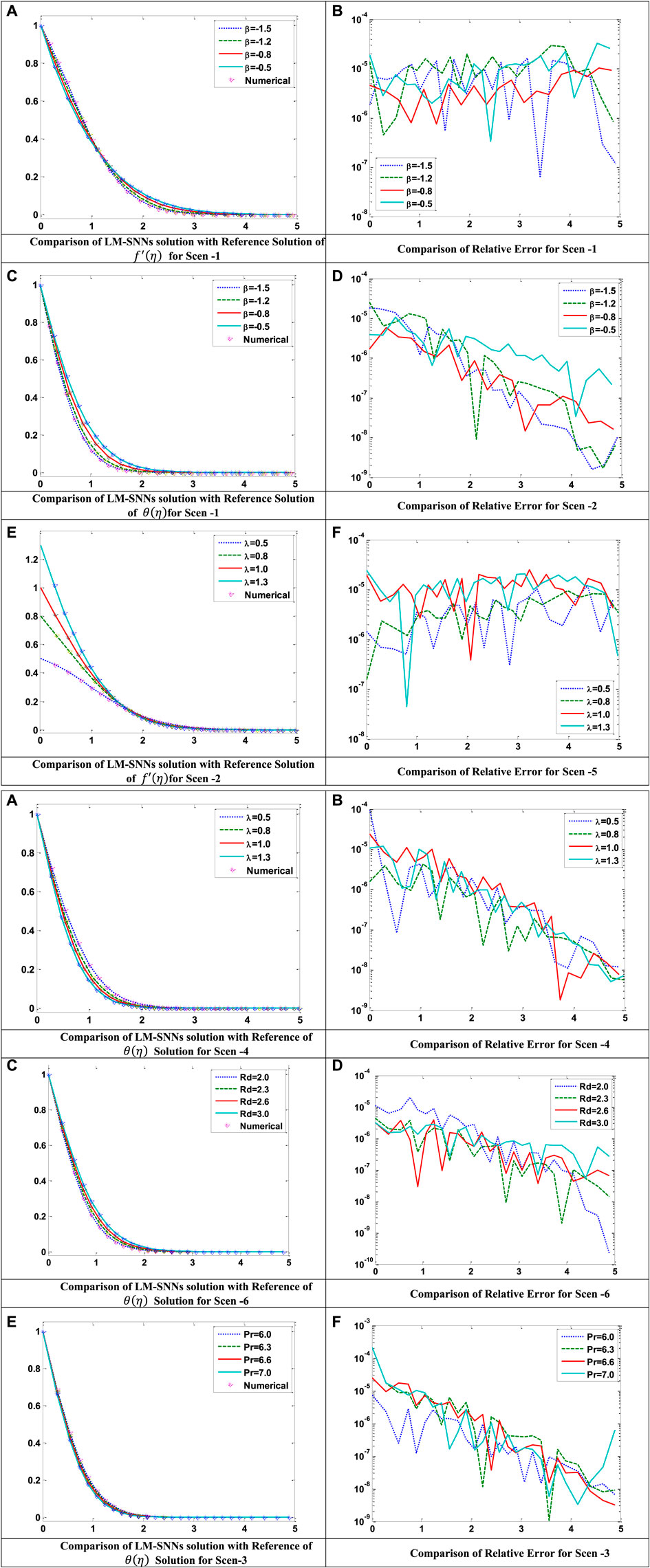
FIGURE 12. Comparison of the Levenberg–Marquardt supervised neural network solution with reference solution along with relative errors for various scenarios. (a), (b).
Conclusion
Here, we employed a numerical study using a novel LM-SNNs–based methodology to investigate the 3D-UHSRS by modeling it in terms of PDEs, which were further reduced to ODEs. A well-renowned solution technique “Lobatto IIIA” was implemented to solve these sets of equation. The influence of various involved physical parameters on velocity and thermal performance are visualized and studied. The solution in respect of each variable in the form of dataset points are acquired and placed in MATLAB for proper operation of the LM-SNNs solution by training, validation, and testing of these dataset points at 80%, 10%, and 10%, respectively. Accuracy, precision, and cogency of the solution were validated through various graphical results consisting of time series, MSE, error distribution, and regression plots.
Followings are few important research outcomes:
• Higher values of
• An increase in velocity and decline in the temperature field were observed with the increasing values of
• A boost in the fluid temperature was observed with increasing values of
Solution methodologies working on the principle of artificial intelligence and machine learning can be more beneficial and valuable in solving the problems related to nano [52–54] and micro fluids [55–61].
Data Availability Statement
The original contributions presented in the study are included in the article/Supplementary Material. Further inquiries can be directed to the corresponding authors.
Author Contributions
MS, MR, and MK contributed to conception and design of the study. SS organized the database. II performed the statistical analysis. MS and II wrote the first draft of the manuscript. KN, MR, and SS wrote sections of the manuscript. All authors contributed to manuscript revision, read, and approved the submitted version.
Conflict of Interest
The authors declare that the research was conducted in the absence of any commercial or financial relationships that could be construed as a potential conflict of interest.
Publisher’s Note
All claims expressed in this article are solely those of the authors and do not necessarily represent those of their affiliated organizations, or those of the publisher, the editors, and the reviewers. Any product that may be evaluated in this article, or claim that may be made by its manufacturer, is not guaranteed or endorsed by the publisher.
Acknowledgments
The work in this study was supported, in part, by the Open Access Program from the American University of Sharjah”. This study represents the opinions of the author(s) and does not mean to represent the position or opinions of the American University of Sharjah.
References
1. Wen D, Ding Y. Effective Thermal Conductivity of Aqueous Suspensions of Carbon Nanotubes (Carbon Nanotube Nanofluids). J Thermophys Heat Transfer (2004) 18:481–5. doi:10.2514/1.9934
2. Raja B, Godson L, Lal DM, Wongwises S. Experimental Investigation on the Thermal Conductivity and Viscosity of Silver-Deionized Water Nanofluid. Exp Heat Transf (2010) 23:317–32. doi:10.1080/08916150903564796
3. Xing M, Yu J, Wang R. Experimental Study on the Thermal Conductivity Enhancement of Water Based Nanofluids Using Different Types of Carbon Nanotubes. Int J Heat Mass Transfer (2015) 88:609–16. doi:10.1016/j.ijheatmasstransfer.2015.05.005
4. Agarwal R, Verma K, Agrawal NK, Duchaniya RK, Singh R. Synthesis, Characterization, thermal Conductivity and Sensitivity of CuO Nanofluids. Appl Therm Eng (2016) 102:1024–36. doi:10.1016/j.applthermaleng.2016.04.051
5. Agarwal R, Verma K, Agrawal NK, Singh R. Sensitivity of thermal Conductivity for Al2O3 Nanofluids. Exp Therm Fluid Sci (2017) 80:19–26. doi:10.1016/j.expthermflusci.2016.08.007
6. Chi SU, Eastman JA. Enhancing Thermal Conductivity of Fluids with Nanoparticles (No. ANL/MSD/CP-84938; CONF-951135-29). IL (United States): Argonne National Lab (1995).
7. Hussain A, Hassan A, Al Mdallal Q, Ahmad H, Rehman A, Altanji M, et al. Heat Transport Investigation of Magneto-Hydrodynamics (SWCNT-MWCNT) Hybrid Nanofluid Under the Thermal Radiation Regime. Case Stud Therm Eng (2021) 27:101244. doi:10.1016/j.csite.2021.101244
8. Babar H, Ali HM. Towards Hybrid Nanofluids: Preparation, Thermophysical Properties, Applications, and Challenges. J Mol Liquids (2019) 281:598–633. doi:10.1016/j.molliq.2019.02.102
9. Babu JAR, Kumar KK, Srinivasa Rao S. State-of-Art Review on Hybrid Nanofluids. Renew Sustainable Energ Rev (2017) 77:551–65. doi:10.1016/j.rser.2017.04.040
10. Gulzar O, Qayoum A, Gupta R. Experimental Study on Stability and Rheological Behaviour of Hybrid Al2O3-TiO2 Therminol-55 Nanofluids for Concentrating Solar Collectors. Powder Technology (2019) 352:436–44. doi:10.1016/j.powtec.2019.04.060
11. Shah TR, Ali HM. Applications of Hybrid Nanofluids in Solar Energy, Practical Limitations and Challenges: A Critical Review. Solar Energy (2019) 183:173–203. doi:10.1016/j.solener.2019.03.012
12. Yang L, Ji W, Mao M, Huang JN. An Updated Review on the Properties, Fabrication and Application of Hybrid-Nanofluids along with Their Environmental Effects. J Clean Prod (2020) 257:120408. doi:10.1016/j.jclepro.2020.120408
13. Nagoor AH, Alaidarous ES, Sabir MT, Shoaib M, Raja MAZ. Numerical Treatment for Three-Dimensional Rotating Flow of Carbon Nanotubes with Darcy-Forchheimer Medium by the Lobatto IIIA Technique. AIP Adv (2020) 10(2):025016. doi:10.1063/1.5135165
14. Song Y-Q, Ali Khan S, Imran M, Waqas H, Ullah Khan S, Ijaz Khan M, et al. Applications of Modified Darcy Law and Nonlinear Thermal Radiation in Bioconvection Flow of Micropolar Nanofluid over an off Centered Rotating Disk. Alexandria Eng J (2021) 60(5):4607–18. doi:10.1016/j.aej.2021.03.053
15. Hussain A, Arshad M, Rehman A, Hassan A, Elagan SK, Ahmad H, et al. Three-Dimensional Water-Based Magneto-Hydrodynamic Rotating Nanofluid Flow over a Linear Extending Sheet and Heat Transport Analysis: A Numerical Approach. Energies (2021) 14(16):5133. doi:10.3390/en14165133
16. Ahmad S, Coban HH, Khan MN, Khan U, Shi Q-H, Muhammad T, et al. Computational Analysis of the Unsteady 3D Chemically Reacting MHD Flow with the Properties of Temperature Dependent Transpose Suspended Maxwell Nanofluid. Case Stud Therm Eng (2021) 26:101169. doi:10.1016/j.csite.2021.101169
17. Imran M, Farooq U, Waqas H, Anqi AE, Safaei MR. Numerical Performance of Thermal Conductivity in Bioconvection Flow of Cross Nanofluid Containing Swimming Microorganisms over a Cylinder with Melting Phenomenon. Case Stud Therm Eng (2021) 26:101181. doi:10.1016/j.csite.2021.101181
18. Srinivasulu T, Goud BS. Effect of Inclined Magnetic Field on Flow, Heat and Mass Transfer of Williamson Nanofluid over a Stretching Sheet. Case Stud Therm Eng (2021) 23:100819. doi:10.1016/j.csite.2020.100819
19. Ahmad F, Abdal S, Ayed H, Hussain S, Salim S, Almatroud AO. The Improved Thermal Efficiency of Maxwell Hybrid Nanofluid Comprising of Graphene Oxide Plus Silver/Kerosene Oil over Stretching Sheet. Case Stud Therm Eng (2021) 27:101257. doi:10.1016/j.csite.2021.101257
20. Bakthavatchalam B, Habib K, Saidur R, Saha BB, Irshad K. Comprehensive Study on Nanofluid and Ionanofluid for Heat Transfer Enhancement: A Review on Current and Future Perspective. J Mol Liquids (2020) 305:112787. doi:10.1016/j.molliq.2020.112787
21. Farhana K, Kadirgama K, Rahman MM, Noor MM, Ramasamy D, Samykano M, et al. Significance of Alumina in Nanofluid Technology. J Therm Anal Calorim (2019) 138(2):1107–26. doi:10.1007/s10973-019-08305-6
22. Yasinskiy A, Navas J, Aguilar T, Alcántara R, Gallardo JJ, Sánchez-Coronilla A, et al. Dramatically Enhanced thermal Properties for TiO2-Based Nanofluids for Being Used as Heat Transfer Fluids in Concentrating Solar Power Plants. Renew Energ (2018) 119:809–19. doi:10.1016/j.renene.2017.10.057
23. Ouyang C, Akhtar R, Raja MAZ, Touseef Sabir M, Awais M, Shoaib M. Numerical Treatment with Lobatto IIIA Technique for Radiative Flow of MHD Hybrid Nanofluid (Al2O3—Cu/H2O) over a Convectively Heated Stretchable Rotating Disk with Velocity Slip Effects. AIP Adv (2020) 10(5):055122. doi:10.1063/1.5143937
24. Shoaib M, Raja MAZ, Sabir MT, Islam S, Shah Z, Kumam P, et al. Numerical Investigation for Rotating Flow of MHD Hybrid Nanofluid with Thermal Radiation over a Stretching Sheet. Sci Rep (2020) 10(1):18533–15. doi:10.1038/s41598-020-75254-8
25. Shoaib M, Raja MAZ, Sabir MT, Nisar KS, Jamshed W, Felemban BF, et al. MHD Hybrid Nanofluid Flow Due to Rotating Disk with Heat Absorption and Thermal Slip Effects: An Application of Intelligent Computing. Coatings (2021) 11(12):1554. doi:10.3390/coatings11121554
26. Patel HE, Das SK, Sundararajan T, Nair AS, George B, Pradeep T. Thermal Conductivities of Naked and Monolayer Protected Metal Nanoparticle Based Nanofluids: Manifestation of Anomalous Enhancement and Chemical Effects. Appl Phys Lett (2003) 83(14):2931e2933. doi:10.1063/1.1602578
27. Abbassi Y, Talebi M, Shirani AS, Khorsandi J. Experimental Investigation of TiO2/Water Nanofluid Effects on Heat Transfer Characteristics of a Vertical Annulus with Non-Uniform Heat Flux in Non-Radiation Environment. Ann Nucl Energ (2014) 69:7e13. doi:10.1016/j.anucene.2014.01.033
28. Krishna MV, Ahammad NA, Chamkha AJ. Radiative MHD Flow of Casson Hybrid Nanofluid over an Infinite Exponentially Accelerated Vertical Porous Surface. Case Stud Therm Eng (2021) 27:101229. doi:10.1016/j.csite.2021.101229
29. Suganya S, Muthtamilselvan M, Alhussain ZA. Activation Energy and Coriolis Force on Cu-TiO2/Water Hybrid Nanofluid Flow in an Existence of Nonlinear Radiation. Appl Nanosci (2021) 11(3):933–49. doi:10.1007/s13204-020-01647-w
30. Shoaib M, Raja MAZ, Sabir MT, Awais M, Islam S, Shah Z, et al. Numerical Analysis of 3-D MHD Hybrid Nanofluid over a Rotational Disk in Presence of thermal Radiation with Joule Heating and Viscous Dissipation Effects Using Lobatto IIIA Technique. Alexandria Eng J (2021) 60(4):3605–19. doi:10.1016/j.aej.2021.02.015
31. Alarifi I, Abokhalil A, Osman M, Lund L, Ayed M, Belmabrouk H, et al. MHD Flow and Heat Transfer over Vertical Stretching Sheet with Heat Sink or Source Effect. Symmetry (2019) 11(3):297. doi:10.3390/sym11030297
32. Yousif MA, Ismael HF, Abbas T, Ellahi R. Numerical Study of Momentum and Heat Transfer of MHD Carreau Nanofluid over an Exponentially Stretched Plate with Internal Heat Source/Sink and Radiation. Heat Transfer Res (2019) 50(7), 649. doi:10.1615/heattransres.2018025568
33. Pal D, Chatterjee D, Vajravelu K. Influence of Magneto-Thermo Radiation on Heat Transfer of a Thin Nanofluid Film with Non-Uniform Heat Source/Sink. Propulsion Power Res (2020) 9(2):169–80. doi:10.1016/j.jppr.2020.03.003
34. Iqbal SA, Sajid M, Mahmood K, Naveed M, Khan MY. An Iterative Approach to Viscoelastic Boundary Layer Flows with Heat Source/Sink and Thermal Radiation. Therm Sci (2019) (24):3. doi:10.2298/TSCI180202003I
35. Ramadevi B, Kumar KA, Sugunamma V, Sandeep N. Influence of Non-Uniform Heat Source/Sink on the Three-Dimensional Magnetohydrodynamic Carreau Fluid Flow Past a Stretching Surface with Modified Fourier's Law. Pramana - J Phys (2019) 93(6):86. doi:10.1007/s12043-019-1847-7
36. Khan I, Raja MAZ, Shoaib M, Kumam P, Alrabaiah H, Shah Z, et al. Design of Neural Network with Levenberg-Marquardt and Bayesian Regularization Backpropagation for Solving Pantograph Delay Differential Equations. IEEE Access (2020) 8:137918–33. doi:10.1109/access.2020.3011820
37. Bukhari AH, Raja MAZ, Sulaiman M, Islam S, Shoaib M, Kumam P. Fractional Neuro-Sequential ARFIMA-LSTM for Financial Market Forecasting. IEEE Access (2020) 8:71326–38. doi:10.1109/access.2020.2985763
38. Bukhari AH, Sulaiman M, Islam S, Shoaib M, Kumam P, Zahoor Raja MA. Neuro-Fuzzy Modeling and Prediction of Summer Precipitation with Application to Different Meteorological Stations. Alexandria Eng J (2020) 59(1):101–16. doi:10.1016/j.aej.2019.12.011
39. Raja MAZ, Shah FH, Syam MI. Intelligent Computing Approach to Solve the Nonlinear Van der Pol System for Heartbeat Model. Neural Comput Applic (2018) 30(12):3651–75. doi:10.1007/s00521-017-2949-0
40. Umar M. Stochastic Numerical Technique for Solving HIV Infection Model of CD4+ T Cells. The Eur Phys J Plus (2020) 135(6):403. doi:10.1140/epjp/s13360-020-00417-5
41. Ahmad I, Ilyas H, Urooj A, Aslam MS, Shoaib M, Raja MAZ. Novel Applications of Intelligent Computing Paradigms for the Analysis of Nonlinear Reactive Transport Model of the Fluid in Soft Tissues and Microvessels. Neural Comput Applic (2019) 31(12):9041–59. doi:10.1007/s00521-019-04203-y
42. Raja MAZ, Manzar MA, Shah SM, Chen Y. Integrated Intelligence of Fractional Neural Networks and Sequential Quadratic Programming for Bagley–Torvik Systems Arising in Fluid Mechanics. J Comput Nonlinear Dyn (2020) 15(5), 051003. doi:10.1115/1.4046496
43. Umar M, Sabir Z, Raja MAZ, Shoaib M, Gupta M, Sánchez YG. A Stochastic Intelligent Computing with Neuro-Evolution Heuristics for Nonlinear SITR System of Novel COVID-19 Dynamics. Symmetry (2020) 12(10):1628. doi:10.3390/sym12101628
44. Cheema TN, Raja MAZ, Ahmad I, Naz S, Ilyas H, Shoaib M. Intelligent Computing with Levenberg-Marquardt Artificial Neural Networks for Nonlinear System of COVID-19 Epidemic Model for Future Generation Disease Control. Eur Phys J Plus (2020) 135(11):932–5. doi:10.1140/epjp/s13360-020-00910-x
45. Shoaib M, Raja MAZ, Sabir MT, Bukhari AH, Alrabaiah H, Shah Z, et al. A Stochastic Numerical Analysis Based on Hybrid NAR-RBFs Networks Nonlinear SITR Model for Novel COVID-19 Dynamics. Computer Methods Programs Biomed (2021) 202:105973. doi:10.1016/j.cmpb.2021.105973
46. Maqsood N, Mustafa M, Khan JA. Numerical Tackling for Viscoelastic Fluid Flow in Rotating Frame Considering Homogeneous-Heterogeneous Reactions. Results Phys (2017) 7:3475–81. doi:10.1016/j.rinp.2017.09.011
47. Mohd Sohut NFH, Soid SK, Abu Bakar S, Ishak A. Unsteady Three-Dimensional Flow in a Rotating Hybrid Nanofluid over a Stretching Sheet. Mathematics (2022) 10(3):348. doi:10.3390/math10030348
48. Waini I, Ishak A, Pop I. Unsteady Flow and Heat Transfer Past a Stretching/Shrinking Sheet in a Hybrid Nanofluid. Int J Heat Mass Transfer (2019) 136:288–97. doi:10.1016/j.ijheatmasstransfer.2019.02.101
49. Huminic G, Huminic A. Heat Transfer Capability of the Hybrid Nanofluids for Heat Transfer Applications. J Mol Liquids (2018) 272:857–70. doi:10.1016/j.molliq.2018.10.095
50. Awais M, Raja MAZ, Awan SE, Shoaib M, Ali HM. Heat and Mass Transfer Phenomenon for the Dynamics of Casson Fluid through Porous Medium over Shrinking wall Subject to Lorentz Force and Heat Source/Sink. Alexandria Eng J (2021) 60(1):1355–63. doi:10.1016/j.aej.2020.10.056
51. Uddin I, Akhtar R, Zhiyu Z, Islam S, Shoaib M, Raja MAZ. Numerical Treatment for Darcy-Forchheimer Flow of Sisko Nanomaterial with Nonlinear thermal Radiation by Lobatto IIIA Technique. Math Probl Eng (2019) 2019. doi:10.1155/2019/8974572
52. Uddin I, Akhtar R, Khan MAR, Zhiyu Z, Islam S, Shoaib M, et al. Numerical Treatment for Fluidic System of Activation Energy with Non-Linear Mixed Convective and Radiative Flow of Magneto Nanomaterials with Navier's Velocity Slip. AIP Adv (2019) 9(5):055210. doi:10.1063/1.5099030
53. Sapuppo F, Bucolo M, Intaglietta M, Johnson PC, Fortuna L, Arena P. An Improved Instrument for Real-Time Measurement of Blood Flow Velocity in Microvessels. IEEE Trans Instrum Meas (2007) 56(6):2663–71. doi:10.1109/tim.2007.907959
54. Schembri F, Sapuppo F, Bucolo M. Experimental Classification of Nonlinear Dynamics in Microfluidic Bubbles' Flow. Nonlinear Dyn (2012) 67(4):2807–19. doi:10.1007/s11071-011-0190-4
55. Cairone F, Anandan P, Bucolo M. Nonlinear Systems Synchronization for Modeling Two-Phase Microfluidics Flows. Nonlinear Dyn (2018) 92(1):75–84. doi:10.1007/s11071-017-3819-0
56. Cairone F, Gagliano S, Bucolo M. Experimental Study on the Slug Flow in a Serpentine Microchannel. Exp Therm Fluid Sci (2016) 76:34–44. doi:10.1016/j.expthermflusci.2016.02.011
57. Anandan P, Gagliano S, Bucolo M. Computational Models in Microfluidic Bubble Logic. Microfluid Nanofluid (2015) 18(2):305–21. doi:10.1007/s10404-014-1434-7
58. Cairone F, Gagliano S, Carbone DC, Recca G, Bucolo M. Micro-Optofluidic Switch Realized by 3D Printing Technology. Microfluidics and nanofluidics (2016) 20(4):1–10. doi:10.1007/s10404-016-1727-0
59. Abukhaled M, Khuri S, Rabah F. Solution of a Nonlinear Fractional COVID-19 Model. Heat Fluid Flow (2022). ahead-of-print No. ahead-of-print. doi:10.1108/HFF-01-2022-0042
60. Saravanakumar S, Eswari A, Rajendran L, Abukhaled M. A Mathematical Model of Risk Factors in HIV/AIDS Transmission Dynamics: Observational Study of Female Sexual Network in India. Appl Math Inf Sci (2020) 14:967–76. doi:10.18576/amis/140603
61. Mary MLC, Devi MC, Meena A, Rajendran L, Abukhaled M. Mathematical Modeling of Immobilized Enzyme in Porous Planar, Cylindrical, and Spherical Particle: A Reliable Semi-Analytical Approach. Reac Kinet Mech Cat (2021) 134:641–51. doi:10.1007/s11144-021-02088-4
Nomenclature
Symbols
u, v, w (ms−1) Velocity components
CP (m2s−2K−1) Specific heat
λ (m−1) Stretching coefficient
T (K) Temperature
K (mkgs−3K−1) Thermal conductivity
Abbreviations
LM Levenberg–Marquardt
SNN Supervised neural networks
C Case
S Scenario
nf Nanofluid
hnf Hybrid nanofluid
f, g Dimensionless velocity components
Nu Nusselt number
Re Reynolds number
SWCNTs Single wall CNTs
MWCNTs Multiwall CNTs
MSE Mean square error
MHD Magnetohydrodynamics
ODEs Ordinary differential equations
PDEs Partial differential equations
Keywords: Lobatto IIIA, hybrid nanofluid, unsteadiness parameter, stretching parameter, Prandtl number
Citation: Shoaib M, Abukhaled M, Raja MAZ, Khan MAR, Sabir MT, Nisar KS and Iltaf I (2022) Heat and Mass Transfer Analysis for Unsteady Three-Dimensional Flow of Hybrid Nanofluid Over a Stretching Surface Using Supervised Neural Networks. Front. Phys. 10:949907. doi: 10.3389/fphy.2022.949907
Received: 21 May 2022; Accepted: 14 June 2022;
Published: 06 September 2022.
Edited by:
Luigi Fortuna, University of Catania, ItalyReviewed by:
Arturo Buscarino, Università degli Studi di Catania, ItalySalvina Gagliano, Università degli Studi di Catania, Italy
Lucia Valentina Gambuzza, University of Catania, Italy
Copyright © 2022 Shoaib, Abukhaled, Raja, Khan, Sabir, Nisar and Iltaf. This is an open-access article distributed under the terms of the Creative Commons Attribution License (CC BY). The use, distribution or reproduction in other forums is permitted, provided the original author(s) and the copyright owner(s) are credited and that the original publication in this journal is cited, in accordance with accepted academic practice. No use, distribution or reproduction is permitted which does not comply with these terms.
*Correspondence: Muhammad Asif Zahoor Raja, rajamaz@yuntech.edu.tw; Kottakkaran Sooppy Nisar, ksnisar1@gmail.com