- Economics, Claremont Graduate University, Claremont, CA, United States
Human-machine teams or systems are integral parts of society and will likely become more so. Unsettled are the effects of these changes, their mechanism(s), and how to measure them. In this article, I propose a central concept for understanding human-machine interaction: convergent cause. That is, Agent 1’s response to the object is caused by the object and Agent 2’s response, while Agent 2 responds to Agent 1’s response and the object. To the extent a human-machine team acts, AI converges with a human. One benefit of this concept is that it allows degrees, and so avoids the question of Strong or Weak AI. To defend my proposal, I repurpose Donald Davidson’s triangulation as a model for human-machine teams and systems.
1 Introduction
An automated vacuum zig zags across the floor. On less guarded days, it is easy to think the vacuum is “looking” for dirt and is satisfied as it crackles over some. Some of its crossings look random and inefficient, yet as it maneuvers around chair legs, cautiously passes under curtains, tracks walls, and detects streaks of dirt, its motions enforce the impression that it “looks” for dirt. Colloquial explanations of its behavior use words like maneuver, caution, tracking, detection, words that exemplify propositional attitude reporting sentences, or sentences that concern cognitive relations [1]. Standard examples: “Jack likes Jill,” “Jack wants Jill to fetch a pale of water,” and “Jack accidentally broke his crown.” Describing the vacuum’s behavior with such sentences suggests the vacuum has a mental life devoted to cleaning floors. Whether the vacuum is intelligent is less relevant here than our tendency to describe behavior in terms of propositional attitudes. This tendency is my first premise.
Still, there are good reasons to think the vacuum lacks propositional attitudes, like intending to pick up dirt, and these reasons weaken our tendency to think about the vacuum as intentional. Resistance to taking our colloquial way of describing machine behavior seriously qualifies my first premise. The vacuum must believe it is picking up dirt (or failing to) to intend as much—at least, an observer like you or I must infer a belief from its behavior. To intend is to believe, and vice versa. I cannot intend to pick up a cup unless I believe there is a cup nearby, that I can reach it, that extending my hand just so, applying pressure, and retracting my arm will pick it up, et cetera. Another reason to deny the vacuum propositional attitudes is that doing so fails the substitution test [14, pg. 97]. Suppose the vacuum was designed to sense, then report, what it inhales. If the vacuum reports “dirt,” does it also intend to pick up soil? Crumbs? fur? Arguably, no. A vacuum does not distinguish them, nor would more sensitive sensors and precise reports do so. Substitution and synonymy test whether the vacuum has a concept. So although we may describe machine behavior with sentences that report propositional attitudes, the tendency may be weaker or stronger depending on how sophisticated the machine behaves. In the weakest of cases, when machines look unintelligent, our language conveys its own limit. There seems no adequate way to describe events that come between mindless events, on one hand, and thought or action, on the other [14, Essay 9].
An automated vacuum is one appliance within “smart” or “helpful” homes. Others are air purifiers, cameras, thermostats, lights, door bells, displays, garage doors, and apps to control them all. Many are voice, motion, or light activated, too. Control and security are not their sole ends; the house is becoming a human-machine system. If our tendency to ascribe propositional attitudes to machines has degrees, their integration into the home (clothes, cars, business) strengthens this tendency.1 Google’s Rishi Chandra, Vice President of Product and General Manager at Google Nest, said in 2019 that we are transitioning from mobile computing (having a computer on one’s phone, for example) to ambient computing, or “having an always accessible computer right at your fingertips, that understands you, that can do things on your behalf to help you in different ways” [2].2 One AI system will manage various devices and be sensitive to a user’s needs, habits, and desires so that an evolving intelligence forms the environment independent of the person’s actions, yet responsive to their own attitudes and patterns.3 Within such a system, the automated vacuum will be deployed when and where the floor is dirty. Ambient homes (and advances in machine learning, generally) motivate my first premise: colloquial descriptions of machine behavior (will) shape how we perceive their behavior.
Forecasting, prediction, and prophesy are notoriously hard and uncertain. Measuring the effects of AI in society has three associated challenges: 1) incorporating the social, contextual, or purposive nature of action (coordinated or not), 2) conceptualizing a trajectory of development that incorporates human agents,4 and 3) allowing artificial intelligence to differ from expectations in productive ways. These challenges hang together insofar as machines emerge in society as social agents. They operate among others in rapidly changing and unexpected ways. Hence problems of brittleness [3] and perception [, 60, 2, 4]. And, regardless if AI has intelligence proper, the sophistication of these machines are often treated as if they were intelligent, and so behavior adjusts likewise. This may explain human decision biasing in which AI system recommendations lead humans into error [61, 38, 27, 7] as well as loss of situational awareness among humans and performance degradation [53, 45, 20, 62, 55, 11]. Theory accounts for these challenges and the proposal here outlines how certain limits of AI and problematic effects on human behavior are related.5
The question, ‘Do humans change when living in a ‘smart’ home?’ requires a theoretical model with empirical studies.6 A theory informs how we interpret a study’s results, design experiments, select methods, credit some results while discounting others. Theory fixes what to look for, expect, and conclude. The theory proposed in this paper is triangulation, which expresses a trajectory as well as interaction. In mathematics, triangulation is a way of discovering a point’s distance from a baseline by measuring another point systematically related to it. Put within social relationships, triangulation describes how someone conceives an object relative to another person (the baseline relation), who also interacts with the same object. One person correlates their response to an object according to the concurrent response of someone else and, as a result, their responses converge on an object from their mutual correlations. When persons intend their response relative to perceiving another person’s intended response (and the observed person does likewise), their responses causally converge—the basic concept of triangulation. This theory clarifies the dynamic of, and requirements for, human-machine teams and systems. While an argument for triangulation follows, an argument which motivates its use,7 the theory stands or falls from empirical study.
2 Triangulation
Humans tend to talk about machine behavior as if it was intended, and so think of it as such. Acting as if machines were intentional and acting with machines differ, however, since joint action requires aligned intent at minimum. Two or more agents act for the same end in coordination. There is a give and take of deliberation, reasons and counters, adaption to unforeseen circumstances, problem solving. How much AI contributes to these daily processes measure its integration into society. First, I will set out the requirements for joint action, which names a threshold and degrees.8 In doing so, I skirt debate over Strong or Weak AI.9 Triangulation clarifies the extent to which machines can jointly act with humans by meeting certain requirements, although some requirements may be barred in principle.
Across essays, the philosopher, Donald Davidson, proposed triangulation as an analogy, a model, and an argument.10 Commentators disagree on what the argument is or whether one name stands for two arguments. Myers and Verheggen note, “…there is no such thing as ‘the’ triangulation argument explicitly laid out in Davidson’s writings” [17], so the argument below is not strictly his. Adding to the ambiguity are the various conclusions Davidson inferred from triangulation: language is social (or there is no language only one person understands) [14, Essay 8], communication requires the concept of an intersubjective world [14, Essay 7], language is required for thoughts [14, Essay 7], and that stimuli become an object when two people recognize one another reacting to that stimuli in similar ways [14, Essay 8]. More commitments are at stake under the heading, “triangulation,” than I will defend—broader views on thought, language, action, subjectivity, and objectivity—since Davidson’s system threads through triangulation. Yet he never polished a formal argument. By repurposing it for human-machine teams and systems, I underscore its empirical bearing (abstract as it is). This move, if prompting select interpretations of experiment, is my main contribution.
Triangulation models how interaction shapes an intersubjective reality that is never given once and for all. Ideally, the model has empirical purchase (explanatory and predictive) and is falsifiable. Arguments couple then with testing. Davidson’s remarks on decision theory generalize: tests only partially support theory insofar as tests depend on how the theory is applied [14, pgs. 125-126]. Experiment design, in other words, assumes theoretical commitments. Before testing a theory, we expect an argument for why the theory nears truth.
2.1 The argument
The threshold from stimulus to object, conditioned reflexes to thought and action, marks the difference between one agent acting as if an object had agency to acting with another agent. Triangulation defines this threshold. When machines obtain agency, and so pass the threshold, they enter society. Theorizing intelligent (in the sense of mental) interaction also explains and predicts how humans will respond to AI systems in teams. Convergent causality sets the trajectory and critical point, and includes requirements for human-machine action, how activity changes with machines, and how objects change as well. Again, convergent causality is how two beings simultaneously correlate their responses to the same object in light of one another. Triangulation, then, fixes the irreducible elements from which causal convergence occurs. The stakes are set.
Some definitions are in order. An object is something taken as such and as existing independently of the one so taking. A language is an abstract object composed of a finite list of expressions, rules for combining them, and interpretations of these expressions according to how they are combined [14, pg. 107]. An action is something done with a belief and an intent. These definitions are meant as weak, ordinary senses of “object,” “language,” and “action” to get us going. More precision comes in the argument for triangulation since these concepts draw from each other.
Mental content will be synonymous with conceptual or intentional content here [34, pg. 12], and so triangulation concerns requirements for concepts or intent. Other prevailing notions of the mental, such as non-conceptual [18], representational [19], phenomenal [20], and intuitional content [21], are left out.11 Propositional attitudes (id est, mental content) have three properties, which are described below and assumed. Contestable, though plausible.12 Each depends on a close parallel between thought and the meaning of sentences [14, pg. 57], and so may be dubiously assumed in an argument that language is sufficient for thought. Still, there are reasons for accepting them.
First, propositional attitudes can be expressed using sentences that are true or false. So when Archidamus exclaims, “I think there is not in the world either malice or matter to alter it,” speaking of Sicilia and Bohemia’s alliance, his sentence is true or false.13 Davidson argues that meaning is truth-conditional by recycling Tarski’s theory of truth [22] as a theory of meaning, which leaves truth undefined [23]. For every sentence, the theory generates a T-sentence: “‘s” is true if and only if p,’ or ‘s means p.’ That is, “‘Archidamus thinks x” if and only if Archidamus thinks x,’ and so the sentence is dequoted, such that any speaker of the language used by the T-sentence would know the original sentence’s truth conditions. The theory works if it successfully sets criteria for understanding a language, describes what a speaker intuitively knows about their language, and can be used to interpret their utterances [14, pg. 132].
Second, sentences about someone’s propositional attitudes are opaque semantically because their meaning depends on belief and intent. So “Archidamus thinks x” is true or false relative to Archidamus’ beliefs. He may change his mind so a sentence once true become false. Or a hearer misinterpret a joke as an avowal. For a third person who did not hear Archidamus’ utterance but a report about his beliefs, the best they can do to verify is ask Archidamus. Meaning cannot be reduced to extension, which spans behavior, gestures, acts, or objects [24]. A speaker must intend their words to be taken as such by a hearer and the hearer rightly pick up on that intention [ [25], Essays 5 and 6]. Attitudes, like intent, belief, and desire, inform an utterance’s meaning.
The third, and last, trait of propositional attitudes is mental holism, which, in Davidson’s words, means “the interdependence of various aspects of mentality” [14, pg. 124]. Intent clings to belief, belief to intent, and to parse one from the other distorts both. An intention cannot be understood without beliefs, and beliefs are mute without intentions that express them. This is not to say that all beliefs are public, but that all we have to go on for understanding another person’s beliefs must be.14 More, a single attitude requires mastery of many concepts, just as possessing one concept assumes many. Consider what must be in place to misapply a concept. Besides a concept in question, other concepts pick out a spectrum of relevance for what rightly or wrongly falls under the concept. Invoked by the concept, ‘dirt,’ are cleanliness, a distinction between indoors and outdoors, an entryway and a bedroom, work boots and high heels, soil, sand, and so on, with each assuming their own concepts. This is why discriminating between fur, crumbs, or hair differs from mastering the concept, as noted in my opening example.
With traits of propositional attitudes in place, the argument can be put within two thought experiments.15 The first argues extension is limited by indeterminacy, whereas the second expands indeterminacy to words themselves. Triangulation hones in on the requirements for successful communication despite.
Indeterminacy, “inscrutability of reference,” or “ontological relativity” were introduced by W. V. O. Quine [26]. His claim, a step toward mental holism, is that a word cannot be fixed to one object. Speakers cannot divulge word meaning from ostention alone; hearers understand the utterance and act within a purposive context, that is, by ascribing intention and beliefs. The richer this purposive context (more precise concepts shared by persons), the more likely communication succeeds since agents can navigate situations of high uncertainty (such as meeting strangers). Quine argues for indeterminacy with a thought experiment called radical translation.
Imagine this scenario [40, pgs. 28-30]. A field linguist meets a speaker from an unknown land, who speaks a language unlike any she knows. The linguist has only query and ostension at her disposal. As she gestures at objects to elicit a response, a rabbit jumps out of a bush and runs between them. The unknown speaker looks down, gestures at the rabbit, and exclaims, “Gavagai.” The linguist jots down the words, “gavagai” and “rabbit.” Another rabbit appears shortly after. The linguist gestures and prompts, “Gavagai?” and the man nods. Once the linguist has done the same with other speakers of the same language, she can be confident in her translation. Even so, indeterminacy surfaces. ‘Gavagai,’ that is, can mean “rabbit,” “undetached rabbit part,” “rabbit stage,” ‘the unique appearance of the rabbit’s left foot while running less than 20 miles per hour,’ and so on, and no number of queries settles things.
In the proclivity of the native to say “gavagai” and English-speakers, “rabbit,” that is, their speech dispositions, Quine argues for persisting indeterminacy. A more complex syntactic apparatus enables the linguist to pick out rabbits, their parts, and stages within the other’s tongue, but that apparatus is relative to an entire catalogue of phrase pairings (what Quine calls a translation manual). Catalogue in hand, indeterminacy seems to disappear, but only seems. Whole catalogues can be compiled for every speech disposition of the language consistently, yet these catalogues rival one another by offering inconsistent interpretations of a given utterance [[27], pg. 73]. Their internal coherence and explanatory power cannot rule out rivals. Put again, one language cannot perfectly and uniquely map onto the words, phrases, references, or meanings of another. By a backdoor of indeterminacy, we come to triangulation. Davidson calls triangulation before language primitive.
Convergent cause is the basis of interaction in triangulation. Davidson glosses, “Each creature learns to correlate the reactions of other creatures with changes or objects in the world to which it also reacts” [14, pg. 128]. Responses to environs or objects are tailored to others’ responses. In Quine’s scenario, the field linguist supposes the rabbit prompted the speaker to say “gavagai.” Organisms discriminate a like cause apart from language in primitive triangulation as conditioned responses to stimuli. When one deer hears a predator and runs, other deer run, too, even if they did not hear the predator. These responses are learned, much like Pavlov’s salivating dogs. Learned discrimination is part of mental life, but it does not pass the threshold of conceptual, or intentional, content, which requires beliefs as well, so the stimuli are not conceptualized as such either.
Discerning a threat or food source differs from applying a concept since the latter assumes the possibility of misapplying. Except from our thoughtful vantage, a creature’s behavior apart from language does not evince defeasible beliefs. Deer may return to a meadow after a predator does not appear, but their return does not suggest the notion of a false alarm. That said, this claim is not to deny the possibility that deer have a rich mental life. They may even have their own language, and so have concepts and beliefs. There is no way for us to know without more precise ways of communicating. Their triangulation is primitive to us. Describing the deer’s behavior as a false alarm projects our concepts, but recourse to our own propositional attitudes does not justify inferring concepts about them. Again, behavior alone does not sufficiently evince one or the other. It is indeterminate, as is the object. The stimulus that caused the coordinated responses does not reify into an object as such because there is no criteria for right or wrong responses.
By contrast, a solitaire, someone who never observes someone else, does not have discernible thoughts either.16 Davidson’s remarks suggest a solitaire has a poorer mental life than mute creatures who triangulate. Lacking shared stimuli, responses are conditioned to a narrow sequence of stimulus and response. There is little “distance” between the solitaire and the stimulus because there is no one else to observe responding to the same. Once another creature enters the scene, the response separates from the stimulus since it is one’s own rather than the other’s response. There is a perception of the stimulus and the perception of the other responding to the stimulus, and so an added dimension of correlation. In this way, the solitaire differs from primitive triangulators.
The scenario of primitive triangulation names a requirement for shared stimuli. Like uttering “gavagai,” the stimulus harbors indeterminacy. The linguist banks on the dramatic moment when the rabbit bounds out of the bush. Maybe the speaker responds to the event otherwise than the linguist expects (and so calls for a hunt or invokes a god). Without words, responses to stimuli lack a mechanism for specifying what causes the response. Davidson mentions two ambiguities [14, pgs. 129-130]: first, those features of the total cause that are relevant to the response; second, whether the stimulus is proximal or distal. The former explains how creatures correlate responses. One creature must be able to recognize in another creature’s response what that other creature is responding to. And the second ambiguity concerns the stimulus itself. Is it the rabbit itself, a rabbit part, the suddenness of the event, or its wider social significance (such as a good or bad omen). Until these ambiguities are overcome, creatures do not identify a cause from mutual responses to stimuli since evidence lacks that the creatures are responding to the same thing [34, pg. 17]. A cause proper must be socially identified, public and precise. In sum, the stimulus and correlated responses are underdetermined until creatures evoke language.
Met, the requirements for successful (linguistic) communication identify a cause, and so surmount the aforementioned ambiguities. Stimulus becomes concept, assuming a plethora of other concepts. Davidson specifies the requirements with an idealized model [17, Essay 7], which does not present what happens in the mind or self-aware expectations. People talk without applying an internal dictionary and grammar. The model below serves a distinct purpose: it concerns communication, whereas triangulation depicts how thought and language are mutually social. Still, these requirements inform the baseline of the triangle (the interaction of agents), which, in turn, enables agents to identify and respond to the same cause.
Say a speaker has a theory for how to speak so that a hearer will rightly hear her and the hearer has a theory for how to make sense of the speaker’s words. Each theory splits into a priory theory, or ways of interpreting an utterance before the uttering, and a passing theory, which form during the occasion of utterance (how the words are voiced and heard in the moment). Prior theories consist in knowledge of grammar, idioms, definitions, past uses. A hearer anticipates a speaker and the speaker a hearer according to prior theories. Passing theories are how this hearer interprets this speaker’s utterances, and how the speaker voices them. If a speaker slips, saying, “Our watch, sir, have indeed comprehended two auspicious persons,” the hearer may rightly understand ‘comprehended’ as ‘apprehended’ and “auspicious” as “suspicious.” If so, passing theories converge without loss. Maybe the mistake was never made before nor again so that past uses do not prepare us for one-off utterances. Less dramatic examples bare this out as hearers make sense of utterances never heard before. Their prior theories do not align. So successful communication only requires that a hearer pick up a speaker’s intent—that is, passing theories converge.
The intent behind an utterance becomes more precise as grunts and gestures become proper names and predicates, truth functional connectives (“and,” “or,” “not,” “if … then”), and quantification (“some,” “all,” “this”). Better specification of intent conveys the same cause for the utterance and obtains a threshold to move from primitive triangulation to its mature form [14, pg. 130]. Complex as language is, though, the indeterminacy our earlier linguist faces confronts neighbors.17 Robust prior theories do not secure interpretations of utterances. Similar words or phrases may be used differently across persons, in endless reams of contexts, or with various forces (asserting, exclaiming, asking, joking). Still, hearers often hear rightly, which narrows on linguistic competence. The formal apparatus is not enough to communicate, though required.
For passing theories to converge, speaker and hearer must (largely) share the same world.18 Davidson gets at this when he claims that speaker and hearer must agree on most things to disagree [23]. The point of contention assumes other concepts are shared. Widespread agreement also facilitates communication. That is, the intersubjective world of objects and concepts enables creatures to overcome indeterminacy. Hearers make sense of speakers by taking cues from how they interact with the world. Note the circular reasoning, which may be virtuous or vicious. Thought requires two creatures to interact since the cause of thought must be a certain shared stimulus. The creatures correlate responses, but that correlation is not action proper until one creature recognizes the other’s intent.19 A formal apparatus with signs does not suffice due to persisting indeterminacy (and the nature of linguistic competence [17, Essay 7]). Objects and concepts, the stuff of mental life, allows creatures to express their intention. Thus, convergent cause relies on the social bearing of language and thought simultaneously.
Setting out a few commitments, then: the content of a belief comes from its cause, its causes are the object and the correlated responses of at least two persons, and one person perceives the intent of another in their simultaneous and mutual response to the object. Triangulation moves from primitive to robust with language since the two ambiguities from before can be resolved. An agent specifies relevance within the total cause through linguistic precision. In doubt, a hearer queries for more information to know what a speaker means. The same can be said for whether the cause is proximal or distal. Degrees matter since indeterminacy threatens fluent agents, yet these ambiguities are more or less resolved as they act in an intersubjective world. From shared objects, concepts, and beliefs, a hearer can navigate malapropisms and other novel, idiosyncratic utterances.
Triangulation (by which I mean robust, or linguistic, triangulation from here on) explains how thought is objective. Truth or falsity is independent of the thinker [14, pg. 129]. To apply a concept, someone must have the notion of misapplying. In this way someone thinks “this” rather than “that,” oaks not elms. These distinctions come from diverging responses to the same cause, as when I stand beside an arborist and exclaim, “What a beautiful elm!” and she replies, “That is an oak.” Triangulators correlate responses to specify the same cause by getting it right and, sometimes, wrong. Without frustrated or vague attempts that are corrected by others, triangulation would not rise beyond discerning stimuli. Such interactions engender external criteria for right and wrong uses of concepts.
Besides explaining how thought is objective, convergent cause objectifies the cause and so enables a hearer to interpret a speaker since the hearer can refer the speaker’s utterance to its cause. But Davidson grants the notion of convergent cause—the crux notion—remains unclear and uncertain [[28], pg. 85]. Hence his initial use of triangulation as an analogy. Triangulation depicts requirements that approximate it, yet empirical analysis may clarify and test convergence. So my recommendation of the recycled theory has two ends: 1) to guide studies on human-machine interaction and 2) to illumine the theory itself. Triangulation picks out sufficient conditions for thought and language, but, here, convergence has not been shown as necessary for thought and language. More on this shortly. We posit that, absent another agent, a shared cause, or language, there is no thought or action, if action is understood as doing something intentionally or for reasons [29]. But, with them, agents have everything they need.
This section began with traits of propositional attitudes, exponed primitive triangulation and two persisting ambiguities, and how linguistic triangulation resolves those ambiguities through convergence. An upshot is that first, second, and third person lose primacy to the irreducible relation between two agents and a mutual object [34, ft [30]] [31]. Action expresses a robust correlation of responses to the same. In the triangle, focus shifts off a given entity to an interaction according to an object, loosely defined. Convergence is the pith and marrow. Primitive and linguistic triangulation mark a threshold in which conditioned reflexes to stimuli refine into thoughts with convergence of simultaneous responses. Let me address some objections to better position triangulation with respect to human-machine interaction.
2.2 Objections
Triangulation has critics. Recall that Davidson never shaped one argument for its defense. Most readers of him, according to Verheggen and Myers [34, pg. 11], find two arguments: one concluding that triangulation fixes meanings; another that triangulation is required for the concept of objectivity. Critics pick apart each in turn. The notion of convergent cause, by contrast, offers a central concept for objectivity and meaning, grounding one argument. Above, I sketched such an argument to shift presumption in favor of triangulation as a theory for human-machine teaming. More argument will be needed to resolve objections than provided here, but my aims are modest. The theory of triangulation merits testing.
Verheggen and Myers note four lines of critique, but two bear on my recycling [34, Ch.1, Section 3]. The first states that perceiving objects fixes meaning, not language. Burge champions this objection, appealing to perceptual psychology, and uses empirical evidence to support the claim that perception picks out and specifies objects by observing a creature’s behavior to a stimulus alongside one’s own.20 Due to the nature of perception, in other words, primitive triangulation suffices. Discernment and detection identify the cause of the other’s behavior with which one correlates one’s response.
This first objection entails that sensing enables joint action rather than language. Before responding, these objections merit a brief foray into their consequences for development. At stake are how we allocate resources, what to expect from our successes, and how to understand our failures. Burge’s view puts perceptual mechanism at the center of human-machine teams. AI recognizes an object, an agent, and an agent’s reaction to that object, and as perceptual limits are overcome, AI will enter society as contributing agents. Language is a helpful appendage, streamlines certain activities, and encourages trust. And how humans perceive machines changes their own behavior, linguistic competencies aside. Convergence, on Burge’s view, results from perceiving the same and coordinating.
For a response, here is a low-hanging fruit: we are concerned with propositional content, Burge with perceptual content. If that is all, better to prefer perception to triangulation since the latter demands more than the former. Triangulation requires perceptual sophistication and then some: linguistic competency, teleological behavior, and, ultimately, intelligence. One reason for adopting triangulation is that perception is not enough for joint action. This motive is bolstered by a recent publication of the National Academies of Sciences, Engineering, and Medicine, which finds that more than perception is required for coordination [32].
Perception, however acute, cannot proffer objectivity because its content cannot be true or false. The requirement for a truth value is an external standard, which, in turn, requires the notion of misapplied concepts. While perception responds to a stimulus, Burge must add that perceiving the stimulus causes a belief, mental content that is either true or false to the perceiver. Again, there is no satisfying evidence that mute perception contains propositional content. Burge is right that perception (in the wide sense of interacting with objects and agents via the senses) is required for mental content. Ambiguities of scope and depth frustrate the identification of a cause that one creature simultaneously responds to in light of another creature’s response (who also responds to the first creature’s response to the object). Perceived content couples with predicates when involving belief and intent, but predicates are expressed via language. Only then do we have information that resolves ambiguity, underdeterminacy, and indeterminacy, however defeasibly. The content of our (human) perception is always more than sheer perception.21
A second objection deserves pause. Scholars criticize triangulation as a circular account of language and thought. This is either a bug or a feature. Given circularity, triangulation is vicious (the charge), uninformative, which can be decided by experimentation, or beneficial as a consistent non-reductive account of language, thought, and action. The circularity surfaces in the move from a primitive triangle to a robust, linguistic one. If there is language, there is thought, but language requires thought. Objectivity, too, can replace either “language” or “thought” in the prior sentence. One assumes the others.
A vicious circle means that at least one of the triangle’s three points reduces to another, and so triangulation distorts the relation. The theory puts undue burden on human-machine action. More damning still, convergence collapses. An agent no longer acts by responding to an object in view of another agent’s response. On triangulation, the task of picking up a cup differs from refilling a mug with coffee, a bottle with water, and emptying a cup of grease. Triangulation explains how closed contexts, such as programming for a specific task, differ from open contexts with uncertainty (and so theorizes brittleness). If wrong, human-machine teams may enter open contexts gradually by programming machines to identify select tasks from a catalogue of closed contexts according to a set of rules. Such task-based development is severely limited if triangulation is right.
Proof for triangulation depends on 1) showing that no element reduces to another, 2) closing off alternative theories, and 3) offering a convincing account of how and why the elements hang together. I return to 2) in a moment. On 1) and 3), Davidson grants that triangulation stems from conviction in humanity’s sociability.22 This conviction is either empirical or a priori depending on the status of mental capacity. Empirical, if one takes facts about speaking and thinking as natural facts about how we speak and think. A priori, if triangulation presents what the concepts of speaking, thinking, and acting mean [24]. Triangulation is theoretical in either case such that empirical testing is at best indirect. Experiments assume theory. An experiment that seems to justify or falsify the theory can be explained away. But how well triangulation makes sense of successes and failures, not to mention spawn development and illuminate tests, favors the theory. A social theory of thought, language, and action would benefit AI research. That said, if designs based on reductive theories widely and repeatedly succeed, triangulation may rightly be discarded.
Alternative theories have attracted support in AI (and so we come to 2) above). Major contenders come from Language of Thought and Computational Theory of Mind [42, 33]. A full defense of triangulation must engage with these theories. My modest aim has been met if the theory seems plausible, attractive, and beneficial. Triangulation names sufficient conditions for thought, language, and action, and so articulates a threshold for human-machine teams to act jointly. More, the distinction between primitive and robust triangulation expresses the grey area before AI comes to its own rich mental life, yet is treated as such by humans. This natural default leads to application.
3 AI in the triangle
If triangulation as a non-reductive account in the end depends on conviction, that is, one is convicted over what language and thought are as natural facts, then empirical tests shoulder or dampen the conviction. Davidson uses triangulation as an example [14, pg. 105] and analogue [14, Essay 9] for describing a set of conceptual claims. Experiments put flesh on these claims and their underlying conviction.23 Applying triangulation may also gain a better understanding of convergence, and so clarify the argument. But I step between planes, if you will, by “applying” triangulation: from conceptual argument to empirical theory and analysis. This move can be opposed by someone who agrees with triangulation as a set of claims yet objects to its refashioning as empirical theory. Or by someone who objects to the refashioning below but accepts another.
My main research question, recall, concerns how humans will act with machines, especially in teams. The irreducibly social element of triangulation means that communication is bound up in joint action and thought. More, how humans describe events, objects, and persons contribute to how they think of them, and humans lack a vocabulary to describe the murky area between thoughtless objects or coincident events, on one hand, and intention-filled ones, on the other hand. Yet AI, as neither an inert object, nor fully rational agent, falls somewhere in this blur. Triangulation, I now argue, helps us conceptualize this situation and the trajectory for human-machine interaction.
The theory looks like a three dimensional triangle (see Figure 1 below). The back plane depicts primitive triangulation, where two languageless creatures discern conditioned by past experiences. The front plane represents robust triangulation. As the baseline interaction becomes more complex, it is as if the triangle slides forward. This motion is represented by the lines joining the points. Robust triangulation is a solid line because it marks the threshold for thought, language, and action.
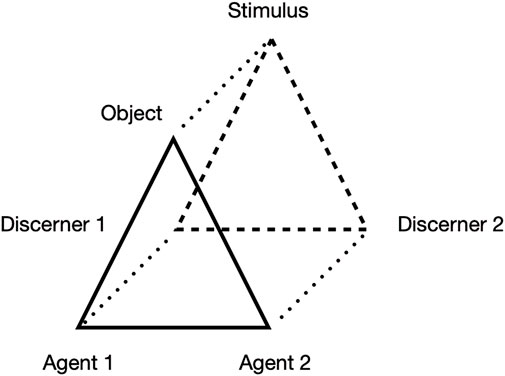
FIGURE 1. Depiction of triangulation. Front surface presents robust triangulation, while back surface presents primitive triangulation. The motion forward, as baseline between creatures becomes more rich, presented by lines connecting points. A respondent has conditioned reflexes to stimuli, whereas an agent conceptualizes that which prompts their response, given another agent doing likewise. While this distinction is a matter of degrees, the requirements for robust triangulation define a threshold. For autonomous human-machine teams, AI systems need to respond to objects in light of their human partner’s response, and vice versa.
The triangle helps us answer two main questions: What is relevant for placing AI between discerner and agent? And what is required to move toward agency? These questions are closely tied since agency depends on how one is perceived by others. Not only must Agent 1 correlate their responses with Agent 2, Agent 2 must correlate theirs with Agent 1.24 One way to think of AI considers how it operates, its hardware, and programming. Since few know or engage with machines according to hardware, we can put materials aside. AI as a social actor does not depend on silicon rather than graphene. That leaves us with how a machine operates and its programming. The latter is indecisive for our questions.
3.1 Programs
A language is a recursively axiomatized system: a set of finite rules joined to a finite vocabulary that produce an indefinite number of expressions. Programming languages are formal since they operate by explicit rules.25 Computation is a formal system that lacks insight or ingenuity, and so is closed, and has explicit, inviolable rules [[35], pg. 17]. Order of input from a fixed set of rules outputs predictably. The function does not assign meaning to the variables since the input results in a set output apart from an interpretation of the input. That is, the operation is “blind.” A calculator computes 1 + 1 irrespective of whether the numbers represent tangible objects or not, though the algorithms of machine learning dwarf basic arithmetic.
Computation has a few properties, such as requiring a sequential, definite, and finite sequence of steps.26 The output forms according to rules and protocols so that the result can be traced back through the program. A program can also be operated by anyone with the same result (it is worrisome if an analysis cannot be replicated). Also, a concrete, external symbolic system makes up the language [37, pgs. 25-27]. On its own terms, there is no indeterminacy in the program until the variables inputted and outputted occur within a purposive or intentional context–that is, until a machine acts among humans.
Put again, syntax lacks semantics until the output makes sense to others, expresses an intent, and endorses some beliefs as opposed to others. Davidson points out, for this reason, that exhaustive knowledge of how a machine works does not entail an interpretation of how the machine acts in the world. While software and hardware limit and sculpt behavior, design and function do not fix meaning (assuming machines can generate meaningful expressions and acts). As a result, computational language’s definition and properties cannot make sense of how machines enter society. The program does not surface in the triangle. Limits and possibilities may be set, but these bounds do not give content to their realizations. How AI operates does.
Nor can material capacities or constraints bar AI from entering society in principle (at least, a conclusive argument has yet to appear). And even if Strong AI is impossible, machines may discern and come close to agency. More, humans may take machines as agents with whom to decide and act. Relevant evidence will come from human and machine behavior as their responses to stimuli converge.
3.2 Convergence
Machines in the triangle respond to an agent and an object (or event) concurrently. A solitaire, as opposed to a triangulator, lives in the world responding to stimuli apart from another agent with whom to correlate. One reason to think AI systems operate as a solitaire is that they respond to an agent or object, not an object in light of an agent’s response to the same object. Humans may help machines correlate through teams since conditioned responses to either agent or object alone do not rise to convergence. Through teams, machines may become more sensitive to context. Supposing machines are not solitaires does not mean they triangulate. A human-machine team does not guarantee triangulation if the machine’s response is not correlated from the human’s response. A threshold must be passed cruxing on convergence.27 And even if machines triangulate, it does not follow that humans triangulate with them. Humans may treat them as objects regardless. As promised, triangulation enlightens the grey area before machines have agency proper.
In a team, machines are more than solitaires if less than agents because humans interact with them toward an end. Art objects are an analogue. Artefacts of writing, for example, deviate from the original triangle with a lapse in time from the original inscription to the reading, and the settings differ [17, pg. 161]. A reader is blind to the writer’s facial expressions, gestures, breathing, pace, and posture when the words were written. Instead, the writer uses textual cues to let the reader know what they mean. Through inscriptions, a successful author brings a reader into a shared conceptual space akin (not the same as) a shared world evoked by the triangle. The analogy misleads, however, if someone takes a machine’s output to express the programmer’s intent, as if the machine mediates an interaction between the human teammate and the programmer. A programming language cannot give meaning to the output since the programmer is no better off in interpreting a machine’s behavior. As AI advances, machines will more frequently act unexpectedly.
The key insight from art is that certain objects gain meaning from how they elicit a response from a reader or viewer. While a written statement refers to something beyond the page, sculptures do not (except for monuments). A sculpture does not prompt the thought that the piece resembles a person qua art, but mimics the experience of meeting them [17, pg. 162]. They elicit a response through stone. But AI is also unlike sculpture insofar as it moves, recognizes, responds, makes noise, completes tasks. So machines may not make meaning per se until they obtain agency, yet elicit meaning from persons. My claim is that machines in teams act as more than solitaires because their behavior elicits and gains meaning from human responses, which machines respond to in turn for the sake of an end. Besides behaving as a programmer intends, machines facilitate human partnerships or not. And the success of teams depends on this facilitation. Again similar to art, success depends on the elicited response (among other conditions).
To the extent someone presumes a machine’s intent from their elicited response, the machine’s behavior converges toward human action. The presumption measures how far behavior converges, at least from the standpoint of a human agent. Risking redundancy, complete convergence means that 1) machines behave so as to respond to the agent as the agent responds to the object and 2) for the agent to perceive this response alongside their response to the object and act accordingly. Correlation is a step toward joint action and collaboration. But as long as the machine’s behavior seems to express a specifiable intent (since intention cannot be hardwired), the machine elicits a response from humans that may adjust their behavior, beliefs, or the end for which they act. The response will be stronger and more precise as machine behavior becomes more precise, familiar, reliable, and consistent across time. Linguistic capacities, appearance, and conventions (even fabricated ones) will cultivate the response effected by machines. The human default to presume an intent is how we make sense of someone else’s behavior: we will presume an intent until interaction suggests otherwise. Although an elicited response stands in for an intended act, there is a degree of potential convergence since 2) is met.
So measuring an elicited response is a test of convergence, but, as I argue in the next section, this effect is hard to isolate. The theoretical reasons for testing convergence have been stated. Humans lack the concepts or language that fill in the degrees from inanimate things to animate ones,28 or living things from thinking ones. For this reason, humans default to presume an intent for behavior. That is, we make sense of activity by acting as if said activity expresses an intent until the presumption no longer makes sense. Depending on the strength of the default, humans presume an intent from AI and, given certain conditions of machine behavior, the presumption has more or less precision and effect. Triangulation exposes broader, contextual requirements for convergence since well-designed AI systems mesh with human routine, expectations, conversation, and so on. Insofar as systems succeed, humans will presume an intent behind machine behavior and act accordingly.
3.3 Social robot
Davidson’s criticisms of the Turing Test frame the requirements for convergence, which define the threshold of, and trajectory toward, agency and autonomy. Triangulation severely qualifies the results of narrow experiments with a subject and a machine performing a task or interacting in a lab. First, let me describe the classic test. Turing argued that the question whether computers think can be answered by examining how humans understand them [36]. In his test, a participant sat at a screen and could type questions into the consul. Another person sat at another, hidden consul, an automated system operated another consul, and both attempted to convince the questioner that they are human and the other is the computer. The questioner only sees their answers on the screen. At the end of a short period, the participant would be asked which of the two was human and which the computer. Turing’s test focuses on how someone interacts with a machine instead of asking about its isolated nature.29 If thought is social, this interaction determines the nature of AI’s operation—whether a machine has a mental life, agency, and autonomy.
Triangulation helps us spot limits with Turing’s Test. Linguistic output on a screen leaves ambiguous whether the words were intended, manufactured, and elicit presumed intent from the questioner. A person cannot tell whether the answerer is thinking apart from deciding what the answerer thinks. Words cannot distinguish a person typing a response of their own or typing a prewritten response intended by someone else, which means intention cannot be recognized by the output. Evidence for a semantics of properly formed expressions consists in the following: 1) words refer to objects in the world, 2) predicates are true of things in the world, and 3) to specify the cause of uttering the words is to know the words’ truth conditions [16, pg. 83]. These are conditions for ascribing propositional attitudes, for a hearer to think a speaker means something by their words. Davidson believes Turing subtracts vital evidence. A questioner before a screen cannot see how the answerer relates in a setting so that the questioner has less reasons for presuming the answerer’s mental life and insufficient evidence for testing it.
How AI is housed, positioned in social situations, and navigates them reveals the extent humans believe the systems think. Humans likewise respond when teamed with machines from a presumed intent that is not frustrated from divergence (or frustrated attempts to correlate responses).30 Using triangulation as a guide, Davidson states three conditions for something to think:
• Understood by a human interpreter;
• Resembles humans in certain ways;
• Possesses the appropriate history of observing causal interactions that prompt select utterances [16, pg. 86]
Turing held the first condition, though impoverishing how humans understand another, and excluded the second and third. A machine’s behavior must make sense: humans recognize an intent that is consistent with apparent beliefs—both expressed in behavior, linguistic and otherwise—and the design of the machine facilitate such recognition. If a robot has a random tick, say, it ‘comes off’ as defective and hinders interaction. The last condition is hard to quantify, Davidson grants, since it brings out the holism of the mental. Using sentences goes beyond information since it draws from causal relations people have experienced. The conceptual map forms and evolves organically, or through a history of learned and correlated responses.
Controlled experiments enable us to isolate effects, yet risk removing needed assumptions of the variable of interest. So Davidson argues for Turing’s Test. This paper began with claims represented by triangulation: mainly, that convergent cause is required for thought and action, which in turn requires language. This concept names the social nature of action. Objections bring out how theoretical commitments lead us to anticipate the role of machines, design experiments, and interpret successes or failures. Then we exponed the theory for application with a foray into the arts to argue that elicited responses from presumed intent should be the variable of interest as AI continues to develop, which presents a trajectory alongside the conditions for thought. Whether human-machine teams succeed depends on how machines elicit responses over time and how humans correlate their own responses as a result. This interaction allows flexibility for machines to behave in surprising ways without ‘breaking’ the interaction. Humans only need to be able to correlate their responses. Experiments can be designed that respect the aforementioned three conditions for thought since the conditions also name the setting in which humans interact among themselves as thinking animals. How well the theory makes sense of past experiments, prompts illuminating new ones, and upholds results from isolating elicited responses from humans marks the theory’s success or failure.
Data availability statement
The original contributions presented in the study are included in the article/Supplementary material, further inquiries can be directed to the corresponding author.
Author contributions
The author confirms being the sole contributor of this work and has approved it for publication.
Acknowledgments
Thanks are due to the reviewers, Chris Arledge and Laurent Mary Chaudron, for reading and commenting on an early draft of this paper. Their feedback led to significant improvements, though all remaining errors are my own. I am also thankful to the editor’s invitation to contribute to this special edition.
Conflict of interest
The author declares that the research was conducted in the absence of any commercial or financial relationships that could be construed as a potential conflict of interest.
Publisher’s note
All claims expressed in this article are solely those of the authors and do not necessarily represent those of their affiliated organizations, or those of the publisher, the editors and the reviewers. Any product that may be evaluated in this article, or claim that may be made by its manufacturer, is not guaranteed or endorsed by the publisher.
Footnotes
1Their integration also enables increased autonomy of the human-machine system, though I put this aside for future work.
2Think, too, of Weiser’s “ubiquitous computing,” in which computer chips permeate one’s environment and body [37]. Also see Kaku’s prediction of the next hundred years for AI [ [38], Ch. 2].
3And so these systems will be autonomous since they will perform tasks without continuous human input [39] and possess intelligence-based capacities, that is, responding to situations that were not anticipated in the design [40] and function as a proxy for human decisions [41]. AI also approximates human activities like the “ability to reason, discover meaning, generalize, or learn from past experiences” [42]. This understanding of intelligence adds specificity to McCarthy’s claim that artificial intelligence is goal-directed activity, though it is important to note that his definition is intentionally open-ended [43].
4This paper assumes AI has reasoning-like processes and these will likely become more sophisticated and sensitive. This second challenge involves placing evolving capacities among persons.
5And so this paper joins those responding to Wiener’s earlier call for philosophy in light of rapid technological progress [44].
6On the importance of theory for empirical analysis, see [45].
7To be clear, the argument is incomplete since my purpose here is to defend triangulation’s plausibility for use in research. More rigorous argumentation, however, is needed.
8My concern is not AI-mediated forms of communication platforms, such as social media. A main difference is that users are largely unaware of how machine-learning algorithms respond to and anticipate their choices for information. This is not human-machine interaction as I conceive it here, which requires transparency and mutual responsiveness.
9And so sidestep Searle’s famous Chinese Room thought experiment, which argues that strong AI is impossible [46]. For a later reflection of his, see [47].
10Davidson has been criticized for obscuring its status. He invokes model and argument in “The Emergence of Thought” [14, Essay 9; pgs. 128-134].
11Davidson never defended his view on mental content, though acknowledging other options [48].
12Following Myers and Verheggen [34, pgs. 12-15], I begin with propositional attitudes. I do not begin with the first property, the holism of the mental, since I find it the most questionable.
13From Shakespeare’s A Winter’s Tale, I.1.
14This does not entail that meaning is extensional. Davidson explains, “Propositional attitudes can be discovered by an observer who witnesses nothing but behavior without the attitudes being in any way reducible to behavior” [14, pg. 100].
15Ludwig alludes to the same [ [49], pg. 81].
16There may never be an actual solitaire. The idea of a solitaire is hypothetical and meant to draw out commitments.
17This is his thesis of radical interpretation [13, Essay 9].
18And so radical interpretation theorizes the requirement for a “common ontology” to share meaning in a multi-agent system [50]. At the same time, an implication of radical interpretation is that, assuming a largely common ontology, two agents can recognize and navigate discrepancies in their use of words.
19Ascribing propositional attitudes happens within the time and place of speech [16, Essay 5].
21A point eloquently argued by McDowell [53].
22Which is not to say that triangulation is immune from argument.
23Davidson writes that “his version of externalism depends on what I think to be our actual practice” [54].
24Yet the situation is more complex: there are often three, four, five, or more agents, temporal lapses between various agents’ responses, agents responding to different objects at staggered times, and a history of past interactions with other agents to the same object or the same agents with different objects. These factors are likewise implicated in convergence.
25‘Formal language’ has various meanings [55]. I adopt computable language.
26This holds in the case of parallel processing and an indefinite loop.
27More on this point shortly.
28For an overview of the shades between inanimate and animate things that challenge its clean distinction, see [56].
29For appraisals of Turing’s Test, see [8, 4, 9, 5, 57].
30As argued by [62], there is a decision of one agent to communicate as well, which means that full triangulation may be blocked if one agent decides not to communicate.
References
1. Nelson M. Propositional attitude reports. In: EN Zalta, editor. The stanford Encyclopedia of philosophy. Spring. Metaphysics Research Lab, Stanford University (2022).
2. Phelan D. Google exec on the future of nest: ”No one asked for the smart home” (2019). url: Available at: https://www.forbes.com/sites/davidphelan/2019/07/20/google-exec-no-one-asked-for-the-smart-home/?sh=3cb8f0bf3f3d July, 2019) (Accessed September 27, 2022).
3. Woods DD. The risks of autonomy: Doyle’s catch. J Cogn Eng Decis Making (2016) 102:131–3. doi:10.1177/1555343416653562
4. Akhtar N, Mian A. Threat of adversarial attacks on deep learning in computer vision: A survey. IEEE Access (2018) 6:14410–30. doi:10.1109/access.2018.2807385
5. Alcorn MA, Li Q, Gong Z, Wang C, Mai L, Ku WS, Nguyen A. Strike (with) A pose: Neural networks are easily fooled by strange poses of familiar objects. In: Proceedings of the IEEE/CVF conference on computer vision and pattern recognition IEEE/CVF. Veranst (2019). p. 4845–54.
6. Yadav A, Patel A, Shah M. A comprehensive review on resolving ambiguities in natural language processing. AI Open (2021) 2:85–92. doi:10.1016/j.aiopen.2021.05.001
7. Endsley MR, Jones DG. Designing for situation awareness: An approach to human-centered design. 2nd. London: Taylor & Francis (2012).
8. Layton C, Smith PJ, McCoy CE. Design of a cooperative problem-solving system for en-route flight planning: An empirical evaluation. Hum Factors (1994) 361:94–119. doi:10.1177/001872089403600106
9. Olson WA, Sarter NB. Supporting informed consent in human machine collaboration: The role of conflict type, time pressure, and display design. In: Proceedings of the human factors and ergonomics society annual meeting bd. 43 human factors and ergonomics society. Veranst (1999). p. 189–93.
10. Yeh M, Wickens C, Seagull F. J.. Target cueing in visual search: The effects of conformity and display location on the allocation of visual attention. Hum Factors (1999) 41:27–32.
12. Wiener EL, Curry RE. Flight deck automation: Promises and problems. Ergonomics (1980) 2310:995–1011. doi:10.1080/00140138008924809
13. Young LRA. On adaptive manual control. Ergonomics (1969) 12:635–74. doi:10.1080/00140136908931083
14. Endsley MR, Kiris EO. The out-of-the-loop performance problem and level of control in automation. Hum Factors (1995) 372:381–94. doi:10.1518/001872095779064555
15. Sebok A, Wickens CD. Implementing lumberjacks and black swans into model-based tools to support human-automation interaction. Hum Factors (2017) 59:189–203. doi:10.1177/0018720816665201
16. Wickens CD. The tradeoff of design for routine and unexpected PerformanceDaytona beach. Daytona Beach, FL: Implications of Situation Awareness Embry-Riddle Aeronautical University Press (1995). p. 57–64.
17. Myers RH, Verheggen C. Donald Davidson’s triangulation argument: A philosophical inquiry. Oxfordshire: Routledge (2016).
21. McDowell J. Avoiding the myth of the given. In: J Lindgaard, editor. John McDowell: Experience, norm and nature. Oxford: Blackwell Publishing, Ltd. (2008). p. 1–14.
22. Tarski A. The concept of truth in formalized languages. In: JH Woodger, editor. Logic, semantics, metamathematics: Papers from 1923 to 1938. Oxford: Clarendon Press (1956). p. 8.
24. Davidson D. Comments on karlovy vary papers. In: P Kotatko, editor. Pagin, peter (hrsg.) ; segal, gabriel (hrsg.): Interpreting Davidson. Stanford: CSLI Publications (2001).
26. Quine WVO. Three indeterminacies. In: D Fèllesdal, and DB Quine, editors. Confessions of a confirmed extentionalist: And other essays. Harvard University Press (2008). p. 368386.
31. Stoutland F. Critical notice. Int J Philos Stud (2006) 141:579–96. doi:10.1080/09672550601003454
32. Endsley MR. Human-AI teaming: State-of-the-Art and research needs. Washington, DC: The National Academies Press (2022).
34. Schneider S. the language of thought: A new philosophical direction. Cambridge: MIT Press (2011).
35. Novaes CD. Formal languages in logic: A philosophical and cognitive analysis. Cambridge University Press (2012).
36. Turing AM. I.—computing machinery and intelligence. Mind (1950) 433–60. doi:10.1093/mind/lix.236.433
37. Weiser M. The computer for the 21st century (1991). URL Available at: https://www.scientificamerican.com/article/the-computer-for-the-21st-century/ (Accessed September 27, 2022).
38. Kaku M. Physics of the future: How science will shape human destiny and our daily lives by the year 2100. New York and London: Doubleday (2011).
39. Groover M. Automation, production systems, and computer-integrated manufacturing. 5th. New York: Pearson (2020).
40.USAF. Air force research laboratory autonomy science and technology strategy/United States air force. Wright-Patterson Air Force Base (2013). Forschungsbericht. URL Available at: https://web.archive.org/web/20170125102447/http://www.defenseinnovationmarketplace.mil/resources/AFRL_Autonomy_Strategy_DistroA.pdf.
41.USAF. Autonomous horizons: The way forward/office of the U.S. Air force chief scientist. Washington, DC: Forschungsbericht (2015).
42. Copeland BJ. Artificial intelligence (2021). URL Available at: https://www.britannica.com/technology/artificial-intelligence. (Accessed September 27, 2022)
43. McCarthy J. What is artificial intelligence? (2007). URL Available at: http://jmc.stanford.edu/articles/whatisai/whatisai.pdf (Zugriffsdatum: 11/24/2007.
44. Wiener EL. Cockpit automation: In need of a philosophy. In: Fourth aerospace behavioral engineering technology conference proceedings SAE. Veranst (1985).
45. Wolpin KI. Limits of inference without theory. Cambridge: MIT Press (2013). (Tjalling C. Koopmans Memorial Lectures).
46. Searle J. Minds, brains, and programs. Behav Brain Sci (1980) 3:417–24. doi:10.1017/s0140525x00005756
47. Searle J. Twenty-one years in the Chinese Room. In: J Preston, editor. Views into the Chinese Room: New essays on Searle and artificial intelligence. Oxford: Clarendon Press (2002). p. 51–69.
48. Davidson D. Responses to barry stroud, john McDowell, and tyler Burge. Philos Phenomenol Res (2003) 67:691–9. doi:10.1111/j.1933-1592.2003.tb00317.x
49. Ludwig K. Triangulation triangulated. In: MC Amoretti, and G Preyer, editors. Triangulation: From an epistemological point of view. Berlin: De Gruyter (2013). p. 69–95.
50. Williams AB. Learning to share meaning in a multi-agent system. Autonomous Agents Multi-Agent Syst (2004) 82:165–93. doi:10.1023/b:agnt.0000011160.45980.4b
51. Burge T. Social anti-individualism, objective reference. Philos Phenomenol Res (2003) 67:682–90. doi:10.1111/j.1933-1592.2003.tb00316.x
52. Bridges J. Davidson’s transcendental externalism. Philos Phenomenol Res (2006) 732:290–315. doi:10.1111/j.1933-1592.2006.tb00619.x
55. Novaes CD. The Different Ways in which Logic is (said to be) Formal. Hist Philos Logic (2011) 32:303–32. doi:10.1080/01445340.2011.555505
57. Siegelmann HT. Computation beyond the turing limit. Science (1995) 268:545–8. doi:10.1126/science.268.5210.545
58. Bringsjord S, Bello P, Ferrucci D. Creativity, the turing test, and the (better) lovelace test. Minds and Machines (2001) 11:3–27. doi:10.1023/a:1011206622741
60. Bringsjord S. The symbol grounding problem .remains unsolved. J Exp Theor Artif Intell (2015) 27:63–72. doi:10.1080/0952813x.2014.940139
61. Clark M, Atkinson DJ. (Is there) A future for lying machines? In: Proceedings of the 2013 deception and counter-deception symposium (2013).
Keywords: triangulation, intersubjectivity, object constitution, social reality, human-machine interaction, action, language
Citation: Quandt RP (2022) AI in society: A theory. Front. Phys. 10:941824. doi: 10.3389/fphy.2022.941824
Received: 11 May 2022; Accepted: 20 September 2022;
Published: 06 October 2022.
Edited by:
William Frere Lawless, Paine College, United StatesReviewed by:
Chris Arledge, Johns Hopkins University, United StatesLaurent Mary Chaudron, Aix Marseille Université, France
Copyright © 2022 Quandt. This is an open-access article distributed under the terms of the Creative Commons Attribution License (CC BY). The use, distribution or reproduction in other forums is permitted, provided the original author(s) and the copyright owner(s) are credited and that the original publication in this journal is cited, in accordance with accepted academic practice. No use, distribution or reproduction is permitted which does not comply with these terms.
*Correspondence: Ryan Phillip Quandt, ryan.quandt@cgu.edu