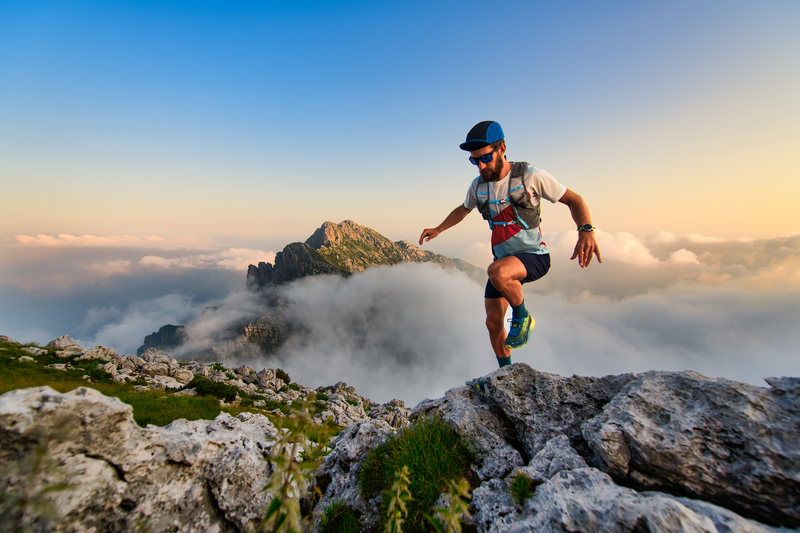
95% of researchers rate our articles as excellent or good
Learn more about the work of our research integrity team to safeguard the quality of each article we publish.
Find out more
REVIEW article
Front. Phys. , 06 June 2022
Sec. Interdisciplinary Physics
Volume 10 - 2022 | https://doi.org/10.3389/fphy.2022.931596
The 2021 Nobel prize for physics was awarded to two climate scientists, Syukuro Manabe and Klaus Hasselmann, and the physicist Giorgio Parisi. While at first sight the work of Parisi seems not to be related to climate science, this is not the case. Giorgio Parisi developed and contributed to many complexity science methods which are nowadays widely used in climate science. Giorgi Parisi also was involved in the development of the “stochastic resonance” idea to explain paleoclimate variability, while Klaus Hasselmann developed stochastic climate models. Here we review and discuss their work from a complex and stochastic systems perspective in order to highlight those aspects of their work. For instance, fractal and multi-fractal analysis of climate data is now widely used and many weather prediction and climate models contain stochastic parameterizations, topics Parisi and Hasselmann have pioneered. Furthermore, Manabe’s work was key to understanding the effects of anthropogenic climate change by the development of key advances in the parameterization of convection and radiative forcing in climate models. We discuss also how their inventive research has shaped current climate research and is still influencing climate modeling and future research directions.
In 2021 the Nobel prize for physics was awarded to Klaus Hasselmann, Syukuro Manabe and Giorgio Parisi for major contributions to complexity science and the understanding and modelling of the climate system [1]. Syukuro Manabe and Klaus Hasselmann have pioneered “the physical modelling of Earth’s climate, quantifying variability and reliably predicting global warming”, while Giorgio Parisi made breakthrough advances in the “discovery of the interplay of disorder and fluctuations in physical systems from atomic to planetary scales” [1]. This was the first Nobel prize for physics awarded to climate scientists. In 1995, Paul Crutzen, Mario Molina, and Sherwood Rowland got awarded the Nobel Prize in Chemistry “for their work in atmospheric chemistry, particularly concerning the formation and decomposition of ozone” [2]; and in 2007 the Intergovernmental Panel on Climate Change was awarded the Nobel Peace prize “for their efforts to build up and disseminate greater knowledge about man-made climate change, and to lay the foundations for the measures that are needed to counteract such change” [3].
The continuing relevance of Syukuro Manabe’s and Klaus Hasselmann’s work can be seen in the latest IPCC reports [4,5], which rely heavily on coupled climate model simulations. Their work was essential in our ability to simulate potential and plausible future evolutions of the coupled Earth system and to unequivocally demonstrate humanities responsibility for global climate change.
The medalists made groundbreaking contributions to climate science, stochastic methods and complexity science for a better understanding of the complex climate system. [6] performed a systematic publication review of the three Nobel laureates; discussing their publication behavior. She also lists their ten most cited papers, which is an indication for their most important work, and they will be discussed in more detail below.
The 2021 Nobel prize is a testimony of the power and wide applicability of physical principles for better understanding and predicting our precious world. We will discuss and review their scientific work from this perspective. We will show how their work has contributed to modern stochastic and complexity science methods and climate modelling, now enabling us to better understand the Earth system and make skillful predictions. They performed this work decades ago, but their work still shapes current research and we believe that it will still be relevant for future research. For this reason a synthesis of these aspects of their work is necessary and useful.
Klaus Hasselmann pioneered multiple areas of climate science; among others he developed optimal finger-printing [7,8] which enables the optimal identification of climate change signals, nonlinear ocean wave dynamics [9,10], Integrated Assessment Models [11], and stochastic climate models [12].
An important topic in climate modelling is the use of stochastic models to represent model error and model uncertainty [13,14]. Klaus Hasselmann’s paper on stochastic climate models [12] is a modern classic and started a new field of climate science and improved our understanding of the origin of climate variability. Hasselmann was motivated to provide an explanation for the observed spectra of climate variability on long time scales, from decadal-scale variability to ice ages and beyond. He observed that these spectra reveal “a continuous variance distribution encompassing all resolvable frequencies, with higher levels at lower frequencies” [12]. This is already a link to fractal methods [15], and the work by Giorgio Parisi, since it has been shown that many climate time series are scaling, which is an imprint of fractals [15,16]. Fractal methods is an area to which Giorgio Parisi also made contributions (e.g., [17]), and which is nowadays widely applied in climate research (e.g., [15,16]).
Stochastic models of climate variability are based on the idea that climate can be decomposed into fast fluctuations, i.e., weather disturbances, and slow variations, i.e., sea-ice or oceanic variations. The fast weather disturbances then produce an integral response of the slow climate variables. Here the fast weather disturbances are represented by a stochastic process. The usefulness of a stochastic process for numerically representing a physically deterministic process can be intuitively understood as follows: from a numerical point of view, the slow variables can be modeled with a much larger time step than the fast variables; the fastest process in a numerical model determines the time step size. While both, slow and fast, processes are deterministic, the fast process decorrelates quickly on the time scale of the slow process. Due to this decorrelation, the fast process can effectively be represented by a stochastic process. On the long time scale the exact physical process representation of the fast process becomes unnecessary. A schematic of this idea is given in Figure 1.
FIGURE 1. Schematic illustration of the underlying idea of stochastic climate modeling. The slow climate model can numerically be modelled with a time step ΔtC (top line), while the fast fluctuation needs a much smaller time step ΔtF (bottom line). While both processes are deterministic, the fast process decorrelates on the slow time scale ΔtC and, thus, can be represented by a stochastic process in an effective climate model which only simulates the slow climate mode.
Hasselmann [12] also argued that the evolution of the corresponding probability density distribution is described by a Fokker-Planck equation [14,18,19]. In the Fokker-Planck equation the stochastic terms appear as diffusion terms. The stochastic term, which has the form of a Wiener process or Brownian motion [14,19–21] would lead to unlimited growth without negative feedbacks or damping. Such stabilising feedbacks lead to a bounded climate probability distribution and consistency with conservation laws, such as energy conservation [22–24]. Thus, it is important that stochastic climate models contain all important physical feedback processes of the climate system. Hasselmann’s stochastic climate model was the first model which reproduced and explained the observed continuous climate variability “without invoking internal instabilities or variable external boundary conditions” [12].
Mathematically, the basic assumption of the Hasselmann stochastic climate model is that the complete state vector z can be decomposed into a fast y and a slow x component: z = (x, y). The equations of motion can now be written as follows:
where F and G are nonlinear functions. Under the assumption of a time scale separation between x and y the following effective stochastic climate model can be written:
where H and Σ are nonlinear functions and W is the Wiener process [20]. Later, Andrew J. Majda (1949–2021) and collaborators developed a systematic approach of deriving stochastic climate models with good predictive skill [14,19,21,22,25–30] which is based on the mathematical theory of adiabatic elimination [20,31–38] which was developed at the same time as Hasselmann’s paper was published.
Hasselmann’s stochastic climate model then pioneered the explanation of climate variability [39–41] and sea ice variability [42]. The importance of Hasselmann’s work lies in the fact, that the stochasic climate model shows that climate as an integrated response to fast weather fluctuation is transforming the white weather noise spectrum into a red spectrum which is widely observed in nature [12].
While the original Hasselmann model is with additive noise, the more general derivation of stochastic climate models showed that the nonlinear interactions between slow and fast fluctuations create multiplicative or state-dependent noise [19,21]. Using the heat budget equation, [43] showed that the Hasselmann model can be extended to include multiplicative noise when considering higher order terms in a Taylor expansion.
Hasselmann’s seminal papers inspired a large body of work leading to stochastic processes nowadays being routinely implemented into operational models [44–48,48–59]. Also empirical approaches are common in fitting stochastic models [22,60–68]. Hence, the Hasselmann’s pioneering work on stochastic climate models is still relevant today.
Klaus Hasselmann also made important contributions to model reduction which is to this day widely used in the development of reduced order stochastic models and data analysis. In a seminal paper [69], he developed a systematic approach to identify the most dynamically important modes of dynamical systems, Principal Interaction Patterns (PIP). PIPs are able to capture the nonlinear dynamics of the full dimensional system in a reduced order subspace [70–75]. While PIPs are a very powerful method, they are computationally expensive to estimate and require the dynamical equations for their estimation. A linearization of PIPs are the Principal Oscillation Patterns (POP) developed by Hasselmann in the same paper [69]. POPs are based on the lagged covariance matrix, where the nonlinearities are represented as a Gaussian white noise forcing [69,76]. Hence, POPs can be straightforwardly estimated directly from data; no knowledge of the underlying dynamical equations are needed. POPs have subsequently been used for a better understanding of climate variability [77–81], and have been further developed into Linear Inverse Models (LIM), which are linear stochastic models where the covariance matrix of the stochastic noise is derived via the Lyapounov equation [82–88]. It has also been shown that PIP, POPs and LIMs can be related to the Koopman operator [89–91]. The Koopman operator is a infinite-dimensional linear operator whose spectral decomposition describes the behavior of nonlinear dynamical systems [91,92]. The approximation of the Koopman operator by Dynamic Mode Decomposition (DMD) [91–94] can be related to the PIP and POP decompositions [91]. This shows the deep connection of PIPs and POPs to the underlying nonlinear dynamics of the system. DMDs are now also getting more popular in climate research [92,95–97].
In earlier work, Hasselmann was one of the first to apply diagrammatic methods to examine energy transfers due to weak nonlinear interactions in random wave fields in order to quantify the surface ocean wave spectra. Hasselmann [10] considered the special case of conservative wave-wave interactions using normal mode coordinates, where the scattering theory is first presented in a Hamiltonian form and the analogy is made to quantum field theory via the interpretation of the transfer expressions in terms of collision processes between hypothetical “particles,” “antiparticles,” and “virtual particles.” A formal perturbation expansion is then applied to the physical energy and momentum transfer rates facilitating expressions for all scattering processes to only a few general interaction rules.
Moving beyond the particle interpretation, this approach was subsequently generalized to include non-conservative interactions between waves and external fields. In this framework, the transfer expressions, summarized in terms of “transfer” diagrams, may still be regarded as corresponding to collision diagrams in the particle picture [98]. Considering interactions between gravity waves and the turbulent atmospheric boundary layer, and applying a closure hypothesis in terms of assumptions of Gaussianity with respect to the cumulants and the linear wave field, Hasselmann was able to derive a complete set of more general lowest order transfer diagrams. At lowest order, these interactions (diagrams) were shown to contain both the Phillips mechanism for the scattering of surface gravity waves by turbulent currents [99–101] and the Miles mechanism of wave generation [101,102]. The combined theory was checked against experimental data by Gilchrist [104]. Hasselmann’s theory extended the Miles-Phillips theory for the directional spectrum of wind-driven surface waves by including additional wave-turbulence interactions associated with energy exchange and production.
These investigations formed the basis of the so-called resonant interaction formalism [10,105] which has proven to provide a key framework for understanding the oceanic internal gravity wave field. The assumption that the timescale for interactions is much longer than the component wave periods, i.e., weak interaction, was challenged in situations where nonlinear effects dominate, such as for interactions between internal gravity waves and turbulence [106,107]. In order to develop a statistical dynamical theory in which all scales and strengths of interactions between waves and turbulence were handled self consistently, Carnevale and Frederiksen [108] employed the prior-time fluctuation dissipation theorem (FDT)
to relate the two-time spectral covariance Ck(t, t′) at wavenumber k to the response function Rk (t, t′) and the prior single-time covariance Ck(t′, t′). In this way the general nonlinear field treats the larger scales as wavelike with turbulence dominating the small scales. Formally, the theory makes no distinction between waves and turbulence. The choice of FDT distinguishes the various statistical closures [109], be they for two- [110] or three-dimensional [111] turbulence or for the two-dimensional internal gravity wave turbulence problem [106,108]. An important class of closures, the Eddy Damped Quasi-Normal Markovian (EDQNM) approximation, has been used to study turbulence interacting with Rossby waves [108,112,113]. The EDQNM reduces to the resonant wave interaction limit of [10] as the damping vanishes (i.e. steady state form of the triad relaxation time μ → 0) [114,115].
The work of Hasselmann occurred at a time when applications of field theoretic methods to problems in geophysical flows were leading to major advances in understanding turbulent flows including the seminal closure works of Kraichnan [110,116], the use of Feynman diagrams for homogeneous isotropic turbulence (HIT) [117] and application to magneto-hydrodynamic turbulence [118]. This work has laid the foundations for subsequent advances in field theoretic approaches to HIT [10,111,119] including functional operator [121,122] and functional (path) integral [123] formalisms. More recent efforts have developed explicit turbulence closures for inhomogeneous turbulence [124,125], including computationally efficient Markovian variants [109] as well as subgrid scale parameterizations directly based on closures [126,127] or data driven approaches inspired by field theoretic approaches for geophysical flows [128–130]. For a comprehensive review of turbulence theories and statistical closures see [131].
Finally it remains to be noted that, in order to be realizable, that is to guarantee non-negative energies in the kinetic energy spectrum, the above mentioned statistical-dynamical approaches must be underpinned by an exact stochastic model representation, i.e., an Ornstein-Uhlenbeck process, or generalised Langevin equation. In this way, statistical and stochastic dynamical systems have a shared underpinning framework.
Giorgio Parisi was awarded the Nobel Prize in Physics in 2021 for his work on disordered systems, and in particular random Ising models and the replica method for the understanding of spin glasses. Nevertheless, Parisi’s connection to climate sciences is manifold, and it descends from his interests on some very fundamental aspects of dynamical systems, especially non-equilibrium and “slightly” non-equilibrium systems. Here, we will focus on disordered systems, as per the scope of the present work, and stochastic resonance, given its close connection to the stochastic models introduced by Hasselmann in the late 70s.
Before we move to these topics, we want to mention, although we will not touch it for sake of brevity, Parisi’s contribution to the modern development of the theory of turbulence. In fact, he was the first, together with Uriel Frisch, to introduce the multifractal formalism [132] to explain the intermittency of velocity fluctuations, overcoming the traditional Kolmogorov’s spectral framework. The rigorous mathematical derivation, though, can be traced back to the works of Frisch [133] and successive studies of Parisi and colleagues (e.g. [17]). This approach has been applied in several studies of turbulence in the atmosphere [134,135], and has been particularly successful in its applications to precipitation (e.g. Venugopal et al. [136]) and clouds (e.g. Lovejoy [137]).
A major part of Giorgio Parisi’s scientific work is devoted to statistical physics of spin glasses, which are disordered magnetic systems formulated within the Ising model with random spin-spin interactions (see the thorough and pedagogical introduction by Castellani and Cavagna [138]). Spin glasses can be modelled by an Ising model with frozen random interactions. The Ising model is a mathematical model for spins σ(i) = ±1, arranged typically on a lattice with grid points i, and with nearest neighbour interactions Jij contributing to the total energy. The complete state of N spins is given by the configuration σ = {σ(i), i = 1 … , N}. The response to an external magnetic field h is probed with the interaction energy contribution − hσ(i) in the Hamiltonian
where Jij are the coupling coefficients in the sum for adjacent spins ⟨ij⟩. In two dimensions, the Ising model shows a phase transition between aligned and disordered spins.
In spin glasses the coupling coefficients Jij are random numbers which remain constant in a quenched spin-glass. In this state of matter a system can be in one of many local minima Nmin separated by large barriers [139] (growing with N), coined as pure states. Complexity Σ (or configurational entropy) is defined by the dependency Nmin ∼ exp (NΣ). Thus, ergodicity is broken and spin glasses are not in a definite pure thermodynamic state, but in a mixture of pure states [140].
Replicas are physically equivalent configurations σa of the system subjected to the same random interactions. The Hamiltonian for a set with n replicas is the sum
for the replicas a, b and where ⟨… ⟩ denotes thermal averages [140]. In the thermodynamic limit the free energy is a function of Q.
The existence of infinitely many pure states can be associated with a spontaneous replica symmetry break. A symmetry break can be detected by a discontinuity in the correlations between two replicas 1 and 2 when the Hamiltonian is perturbed by a small symmetric term,
The calculation of thermodynamic properties of spin glasses is achieved with the so-called replica trick. For a model in contact with a heat bath at temperature T, the partition function Z describes the statistical properties of a system in thermodynamic equilibrium, Z = ∑σ exp (−H [σ]/kbT) and observables can be derived through the free energy F = −kBT ln Z. A main difficulty is the calculation of the mean
The Ising model has been applied to various problems in geosciences with unresolved small scale processes.
• Pleimling et al. [143] consider convection cells in an Ising lattice gas in contact with two thermal reservoirs. In the non-equilibrium stationary state, convection cells emerge which are driven by spontaneous symmetry breaking below the critical temperature.
• Ma et al. [144] describe the formation of ponds in Arctic sea ice during melting in late spring which determine the albedo through their geometric structure. The authors use a binary Ising model which predicts observed power law scaling of the pond size distribution, correlation lengths and fractal dimensions of the clustered ponds. Such results may be useful for the parameterization in global climate models.
• Khouider [145] applies the Ising model as a stochastic multicloud model for organized tropical convection introduced recently to improve the variability in climate models. Each lattice is either clear sky or occupied by one of three cloud types. The coarse-graining could be extended to multi-type particle systems with nearest neighbour interactions and a multi-dimensional birth-death process. Local interactions induce a shift in the climatology and intermittency through coherent cloud clusters and long time excursions.
The idea of long-lived metastable states found in spin glasses has been applied in climate science to explain the recovery from a snow ball Earth, a climatic state with an Earth covered by ice or snow which ended 700 Mio years ago [146]. This state is stable on long time scales due to the ice albedo effect and transitions can be excited by stochastic solar forcing Lucarini and Bódai [147] with a hysteresis effect [148].
Atmospheric circulation regimes [149–151], are metastable states [152] of the atmosphere having a significant impact on surface conditions and predictability, particularly as the formation and decay of such structures on synoptic scales, commonly referred to as “blockings”, are often associated with significant reductions in operational forecast skill. The concept of metastability, i.e., states that are coherent and locally stationary in time, has also been applied to enable the construction of reduced order stochastic models directly from data thereby enabling an understanding of the predictability of atmospheric circulation regimes [22,152–154,156–158] including error growth in weather prediction [159,160] and for the detection and attribution of the external drivers of trends in the frequency of occurrence, persistence and structure of said regimes over time [14].
The idea for what would have been later referred to as “stochastic resonance”, arose when an important aspect of the Milankovitch explanation of glacial-interglacial periods succession, was left unanswered, given that the periodicities in orbital parameters were unable to capture the 105 year periodicity in the Milankovitch spectrum [161]. When Parisi, together with his colleagues Roberto Benzi, Alfonso Sutera and Angelo Vulpiani at Rome La Sapienza University, started to work on a possible solution to this problem, the seminal work by Hasselmann [12] (see section 2) had already stressed that the long-term variability of the climate system could be treated as long-term forced variations with a stochastic fast-scale perturbation superimposed on it. Sutera [162] had already demonstrated that such fast-scale stochastic perturbations would be able to induce random transitions in a Budyko-Sellers type Energy Balance Model (EBM) [163,164], for which multiple stable states, namely a glacial and an inter-glacial state, were identified. Similar arguments were also brought up by Nicolis and Nicolis [165] in the very same year. Despite being able to exhibit random transitions induced by stochastic noise, this model was not capable of transitioning between glacial and interglacial states, no matter what the variance attributed to the noise was. Indeed, Parisi and his colleagues demonstrated that no transition would occur in this stochastically perturbed EBM, unless a periodic forcing (the Milankovitch forcing, in their probe at the 105 year frequency) would be introduced. In other words, the concept of “stochastic resonance” relies on the fact that the stochastic noise introduced in the model introduces the possibility of a transition between observable states of the system, when the variance of the noise “couples” with the amplitude of a periodic forcing. For a rigorous derivation of the concept, one might refer to Benzi et al. [166]. In the following, we will discuss some of the fundamental passages of the application in the context of paleoclimate, as described in Benzi et al. [167].
As a starting point, they consider a Battacharya-Ghil model [168,169], that relies on a parametrization of the albedo allowing for two stable states separated by a 10 K global mean temperature difference. The model can be written as:
where:
• F (T, t) is a function of temperature and time, whose integral is the pseudo-potential Φ(T, t). Maxima and minima of Φ represent the stable and unstable solutions of the budget Equation (7);
• C is the thermal capacity of the Earth;
• ϵ is the global mean emissivity of the Earth;
• μ(t) is the Milankovitch periodic forcing, in our case written as μ(t) = 1 + 0.0005 cos (ωt) with ω = 2π/105 year, thus representing the 50,000 years periodicity;
• T1, T2 and T3 are the global mean equilibrium temperatures for two stable regimes (T1, T3) and one unstable state (T2). Here, it is assumed that ΔT = (T3 − T1)/2 is equal to 5 K;
• β is a dimensionless parameter, a function of the decaying time τ, so that
• σ is the variance associated with a Wiener process represented by η(t). This member of the equation represents the stochastic part of the model.
It is worth noticing that similar results were achieved through the usage of a Fokker-Planck equation, and that the Equation 7 can be non-dimensionalized, noticing that we can define a new variable
where A(t) is again the Milankovitch forcing, that we assume for the sake of simplicity to oscillate between A0 and − A0.
From the theory of stochastic differential equations, it is known that the average random transition time between one stable state and another (in the absence of forcing), is given by (e.g. [170]):
When the Milankovitch forcing is also included, and in the two cases ± A0, we have:
In other words, it is found that when the amplitude of the stochastic noise is sufficiently large, and the noise gets in phase with the Milankovitch periodic forcing, its effect can be exponentially amplified or dampened, depending on the sign. This was the main result of the Benzi et al. [167] paper.
A question that was left unanswered, at that time, was whether a specific physical mechanism had to be invoked, triggering the transition, or the noise, somehow reaching the phase and amplitude for the stochastic resonance, was randomly pushing the system towards a transition. From the theory of stochastic differential equations, it was shown that the probability for a random transition with characteristic timescale τ is given by:
meaning that the transition could be understood as a “rare event” and large deviation theory would be sufficient to investigate the effect of the noise. It was not immediately clear, though, how the noise generated by turbulence, where the turbulent cascade does not allow for a clear scale separation between noise and the large-scale behavior, would ever be useful to obtain this kind of transitions in the system. This problem was later addressed in Benzi [171], by showing that a Sabra shell model of turbulence would exhibit stochastic resonant behavior.
As argued at the beginning of the section, the concept of stochastic resonance has its roots in paleoclimate studies, and found a wide range of applications going well beyond this initial field of research. A few of them are reviewed in Gammaitoni et al. [172]. Here, we briefly describe a selected number of related works that have proved their relevance for climate sciences:
• Ganopolski and Rahmstorf [173] propose the stochastic resonance mechanism to explain the shifts in Northern Atlantic freshwater formation regions as a trigger of millennial climate variability during glacial times;
• As reviewed in Crucifix [174], there have been several studies attempting to investigate the onset of Dansgaard-Oeschger (DO) events in terms of stochastic resonance, either relying on the 1,500 occurrence time of these events [175], or in terms of the synchronisation between noise and different periodicities of the solar cycle [176,177];
• The large deviation algorithm by Ragone et al. [178] relies on the probability of rare events to help the detection of extreme events in modelling studies;
A historical perspective on the achievements by Parisi and his team on the topic of stochastic resonance, in the context of the 2021 Nobel Prize, is also found in a recent contribution on the Nature Italy blog [179].
Syukuro Manabe has, in contrast to Klaus Hasselmann and Giorgio Parisi, not made any direct contributions to complexity science. However, he was instrumental in the development of three dimensional numerical coupled Atmosphere-Ocean models [180,181], which are some of the most complex numerical models in science which are running on the fastest and biggest super computers in the world nowadays. Due to limited computing power in the 1960s these numerical models of the complex Earth system had to be simplified in such a way that they were computationally feasible but still contained the most important aspects of the climate system. With the model in whose development he was instrumental, he showed the impact of increasing greenhouse gases concentrations on Earth climate, evidencing how they lead to increasing surface temperatures. In order to accomplish the development of three dimensional models, Syukuro Manabe had to develop a one-dimensional model of the atmosphere [182] with which they estimated the climate sensitivity, i.e. the amount of warming a doubling of CO2 would produce. The climate sensitivity is nowadays an important aspect of climate change science [183–186] and is an important parameter of Integrated Assessment Models [187–192]. To investigate the climate sensitivity Syukuro Manabe developed a radiative-convective model. With this model he explored the role played by atmospheric gases such as water vapor, carbon dioxide and ozone in setting the thermal structure of the atmosphere. This model was a major step towards the development of the three dimensional model with which he made poineering contributions to understanding of the anthropogenic greenhouse effect [193–195].
Like Giorgio Parisi, Syukuro Manabe worked on paleoclimate [196–198]. He was one of the first to simulate abrupt climate change. Traditionally, it was thought that climate evolved in a slow and smooth way and abrupt climate changes were considered unlikely. However, paleoclimate records showed evidence for abrupt climate changes during glacial periods [199]. In order to examine such abrupt climate changes, he simulated the influx of freshwater from melting ice sheets. This freshwater flux into the North Atlantic caused a shutdown of the Atlantic Meridional Overturning Circulation (AMOC). This shutdown of the ocean circulation has a strong impact on the heat transport, leading to rapid changes in temperature and, hence abrupt climate change. A shutdown of the AMOC is considered a potential tipping element in a warmer world [200] and there is evidence that the AMOC is slowing down in recent years [201].
His work started numerical modeling of the complex Earth system. In 2021 European Union scientists started the project “Destination Earth”, aimed at building a digital twin of the Earth system by 2030, which will enable the mapping of the evolution of the climate system and simulation of extreme events in time and space [202]. In about 50 years the climate science community made the journey from what we nowadays consider to be very simplified, if not crude, climate models to aiming to develop a twin of our Earth in unprecedented high resolution and process representation and which can resolve and simulate “the regional impacts of climate change, natural hazards, marine ecosystems or urban spaces” [202].
The 2021 Nobel prize for physics was awarded to work mainly done in the 1970s and 1980s. However, the pioneering work done by Klaus Hasselmann, Syukuro Manabe and Giorgio Parisi is still relevant and influential today. For instance, while stochastic parameterizations are incorporated in more and more operational weather and climate prediction models [13], there is still a lot of research going on in this area, involving energy consistent stochastic schemes [30,59,97,203–209,211] or the systematic inclusion of memory terms [127,128,130,212]. Combined with complexity science, scaling and fractional noise ideas are becoming more widespreadly used [15,213,214,214–216].
Still an important issue, not only of fundamental interest but also of practical importance, is climate sensitivity. The current generation of Earth system models has a higher climate sensitivity than previous generations [217]. This high climate sensitivity is, however, inconsistent with paleoclimate data [218]. Suggesting, a problem pioneered using numerical models by Syukuro Manabe, still is unsolved and implies large uncertainty about the future evolution of the climate system. Further research is needed, to reveal whether this uncertainty is intrinsic to the complex Earth system with its many interlinked components or whether new generations of models with improved process representation and stochastic parameterizations will reduce this uncertainty. Stochastic and complexity science methods will surely prove essential in this task.
CF conceived the idea of this manuscript. All authors contributed equally to the writing of the manuscript.
CF was supported by the Institute for Basic Science (IBS), Republic of Korea, under IBS-R028-D1, and Pusan National University Research Grant 2021.
The authors declare that the research was conducted in the absence of any commercial or financial relationships that could be construed as a potential conflict of interest.
All claims expressed in this article are solely those of the authors and do not necessarily represent those of their affiliated organizations, or those of the publisher, the editors and the reviewers. Any product that may be evaluated in this article, or claim that may be made by its manufacturer, is not guaranteed or endorsed by the publisher.
We thank two reviewers for their comments.
1.NobelPrize.org. The Nobel Prize in Physics 2021. In: Tech. Rep. Cambridge, MA, USA: Nobel Price (2021) (Accessed February 12, 2022).
2.NobelPrize.org. The Nobel Prize in Chemistry 1995. In: Tech. Rep. Cambridge, MA, USA: Nobel Price (1995) (Accessed April 25, 2022).
3.NobelPrize.org. The Nobel Peace Prize 2007. In: Tech. Rep. Cambridge, MA, USA: Nobel Price (2007) (Accessed April 25, 2022).
4. Masson-Delmotte V, Zhai P, Pirani A, Connors S, Péan C, Berger S, et al. Climate Change 2021: The Physical Science Basis: Working Group I Contribution to the Sixth Assessment Report of the Intergovernmental Panel on Climate Change. Cambridge: Cambridge University Press (2021).
5. Pörtner H, Roberts D, Tignor M, Poloczanska E, Mintenbeck K, Alegría A, et al. Climate Change 2022: Impacts, Adaptation, and Vulnerability. Contribution of Working Group II to the Sixth Assessment Report of the Intergovernmental Panel on Climate Change, Vol. In Press. Cambridge: Cambridge University Press (2022).
6. Bohémier KA. Analysis for Science Librarians of the 2021 Nobel Prize in Physics: Climate, Spin Glass, and Complex Systems. Sci Technol Libraries (2022) 41:1–23.
7. Hasselmann K. On the Signal-To-Noise Problem in Atmospheric Response Studies. In: D Shaw, editor. Meteorology of Tropical Oceans. London: Royal Meteorological Society (1979). p. 251–9.
8. Hasselmann K. Optimal Fingerprints for the Detection of Time-dependent Climate Change. J Clim (1993) 6:1957–71. doi:10.1175/1520-0442(1993)006<1957:offtdo>2.0.co;2
9. Hasselmann K. On the Non-linear Energy Transfer in a Gravity-Wave Spectrum Part 1. General Theory. J Fluid Mech (1962) 12:481–500. doi:10.1017/s0022112062000373
10. Hasselmann K. Feynman Diagrams and Interaction Rules of Wave-Wave Scattering Processes. Rev Geophys (1966) 4:1–32. doi:10.1029/rg004i001p00001
11. Weber M, Barth V, Hasselmann K. A Multi-Actor Dynamic Integrated Assessment Model (Madiam) of Induced Technological Change and Sustainable Economic Growth. Ecol Econ (2005) 54:306–27. doi:10.1016/j.ecolecon.2004.12.035
12. Hasselmann K. Stochastic Climate Models Part I. Theory. Tellus (1976) 28:473–85. doi:10.3402/tellusa.v28i6.11316
13. Berner J, Achatz U, Batté L, Bengtsson L, Cámara Ad. l., Christensen HM, et al. Stochastic Parameterization: Toward a New View of Weather and Climate Models. Bull Amer Meteorol Soc (2017) 98:565–88. doi:10.1175/bams-d-15-00268.1
14. Franzke CLE, O'Kane TJ, Berner J, Williams PD, Lucarini V. Stochastic Climate Theory and Modeling. Wires Clim Change (2015) 6:63–78. doi:10.1002/wcc.318
15. Franzke CLE, Barbosa S, Blender R, Fredriksen H-B, Laepple T, Lambert F, et al. The Structure of Climate Variability across Scales. Rev Geophys (2021) 2021:e2019RG000657.
16. Lovejoy S, Schertzer D. The Weather and Climate: Emergent Laws and Multifractal Cascades. Cambridge: Cambridge University Press (2013).
17. Benzi R, Paladin G, Parisi G, Vulpiani A. On the Multifractal Nature of Fully Developed Turbulence and Chaotic Systems. J Phys A: Math Gen (1984) 17:3521–31. doi:10.1088/0305-4470/17/18/021
19. Majda AJ, Timofeyev I, Vanden Eijnden E. Models for Stochastic Climate Prediction. Proc Natl Acad Sci U.S.A (1999) 96:14687–91. doi:10.1073/pnas.96.26.14687
20. Gardiner CW. Stochastic Methods: A Handbook for the Natural and Social Sciences, Vol. 4. Berlin, Germany: Springer Berlin (2009).
21. Majda AJ, Timofeyev I, Vanden Eijnden E. A Mathematical Framework for Stochastic Climate Models. Comm Pure Appl Math (2001) 54:891–974. doi:10.1002/cpa.1014
22. Majda AJ, Franzke C, Crommelin D (2009). Normal Forms for Reduced Stochastic Climate Models. Proc Natl Acad Sci U.S.A 106, 3649–53. doi:10.1073/pnas.0900173106
23. Majda AJ. Statistical Energy Conservation Principle for Inhomogeneous Turbulent Dynamical Systems. Proc Natl Acad Sci U.S.A (2015) 112:8937–41. doi:10.1073/pnas.1510465112
24. Peavoy D, Franzke CLE, Roberts GO. Systematic Physics Constrained Parameter Estimation of Stochastic Differential Equations. Comput Stat Data Anal (2015) 83:182–99. doi:10.1016/j.csda.2014.10.011
25. Majda A, Timofeyev I, Vanden-Eijnden E. A Priori tests of a Stochastic Mode Reduction Strategy. Physica D: Nonlinear Phenomena (2002) 170:206–52. doi:10.1016/s0167-2789(02)00578-x
26. Majda AJ, Timofeyev I, Vanden-Eijnden E. Systematic Strategies for Stochastic Mode Reduction in Climate. J Atmos Sci (2003) 60:1705–22. doi:10.1175/1520-0469(2003)060<1705:ssfsmr>2.0.co;2
27. Majda AJ, Franzke C, Khouider B. An Applied Mathematics Perspective on Stochastic Modelling for Climate. Phil Trans R Soc A (2008) 366:2427–53. doi:10.1098/rsta.2008.0012
28. Franzke C, Majda AJ, Vanden-Eijnden E. Low-order Stochastic Mode Reduction for a Realistic Barotropic Model Climate. J Atmos Sci (2005) 62:1722–45. doi:10.1175/jas3438.1
29. Franzke C, Majda AJ. Low-order Stochastic Mode Reduction for a Prototype Atmospheric GCM. J Atmos Sci (2006) 63:457–79. doi:10.1175/jas3633.1
30. Franzke CLE, Oliver M, Rademacher JDM, Badin G. Multi-scale Methods for Geophysical Flows. In: A Iske, and C Eden, editors. Energy Transfers in Atmosphere and Ocean. Berlin, Germany: Springer (2019). p. 1–51. doi:10.1007/978-3-030-05704-6_1
31. Kurtz TG. A Limit Theorem for Perturbed Operator Semigroups with Applications to Random Evolutions. J Funct Anal (1973) 12:55–67. doi:10.1016/0022-1236(73)90089-x
32. Kurtz TG. Semigroups of Conditioned Shifts and Approximation of Markov Processes. Ann Probab (1975) 1975:618–42. doi:10.1214/aop/1176996305
33. Papanicolaou GC, Kohler W. Asymptotic Theory of Mixing Stochastic Ordinary Differential Equations. Commun Pure Appl Maths (1974) 27:641–68.
34. Papanicolaou GC. Some Probabilistic Problems and Methods in Singular Perturbations. Rocky Mountain J Maths (1976) 6. doi:10.1216/rmj-1976-6-4-653
35. Pavliotis GA, Stuart A. Multiscale Methods: Averaging and Homogenization. Berlin, Germany: Springer Science & Business Media (2008).
36. Monahan AH, Culina J. Stochastic Averaging of Idealized Climate Models. J Clim (2011) 24:3068–88. doi:10.1175/2011jcli3641.1
37. Culina J, Kravtsov S, Monahan AH. Stochastic Parameterization Schemes for Use in Realistic Climate Models. J Atmos Sci (2011) 68:284–99. doi:10.1175/2010jas3509.1
38. Arnold L. Hasselmann's Program Revisited: the Analysis of Stochasticity in Deterministic Climate Models. In: Stochastic Climate Models. Berlin, Germany: Springer (2001). p. 141–57. doi:10.1007/978-3-0348-8287-3_5
39. Frankignoul C, Hasselmann K. Stochastic Climate Models, Part II Application to Sea-Surface Temperature Anomalies and Thermocline Variability. Tellus (1977) 29:289–305. doi:10.3402/tellusa.v29i4.11362
40. Lemke P. Stochastic Climate Models, Part 3. Application to Zonally Averaged Energy Models. Tellus (1977) 29:385–92. doi:10.3402/tellusa.v29i5.11371
41. Hasselmann K. Construction and Verification of Stochastic Climate Models. In: Climatic Variations and Variability: Facts and Theories. Berlin, Germany: Springer (1981). p. 481–97. doi:10.1007/978-94-009-8514-8_28
42. Lemke P, Trinkl EW, Hasselmann K. Stochastic Dynamic Analysis of Polar Sea Ice Variability. J Phys Oceanogr (1980) 10:2100–20. doi:10.1175/1520-0485(1980)010<2100:sdaops>2.0.co;2
43. Sura P, Sardeshmukh PD. A Global View of Air-Sea thermal Coupling and Related Non-gaussian SST Variability. Atmos Res (2009) 94:140–9. doi:10.1016/j.atmosres.2008.08.008
44. Buizza R, Milleer M, Palmer T. Stochastic Representation of Model Uncertainties in the Ecmwf Ensemble Prediction System. Quart J R Meteorol Soc (1999) 125:2887–908.
45. Shutts G. A Kinetic Energy Backscatter Algorithm for Use in Ensemble Prediction Systems. Q.J.R Meteorol Soc (2005) 131:3079–102. doi:10.1256/qj.04.106
46. Jung T, Palmer TN, Shutts GJ. Influence of a Stochastic Parameterization on the Frequency of Occurrence of North pacific Weather Regimes in the Ecmwf Model. Geophys Res Lett (2005) 32:L23811. doi:10.1029/2005GL024248
47. Berner J, Shutts GJ, Leutbecher M, Palmer TN. A Spectral Stochastic Kinetic Energy Backscatter Scheme and its Impact on Flow-dependent Predictability in the Ecmwf Ensemble Prediction System. J Atmos Sci (2009) 66:603–26. doi:10.1175/2008jas2677.1
48. Hermanson L, Hoskins B, Palmer T. A Comparative Method to Evaluate and Validate Stochastic Parametrizations. Q.J.R Meteorol Soc (2009) 135:1095–103. doi:10.1002/qj.436
49. Dawson A, Palmer TN. Simulating Weather Regimes: Impact of Model Resolution and Stochastic Parameterization. Clim Dyn (2015) 44:2177–93. doi:10.1007/s00382-014-2238-x
50. Davini P, von Hardenberg J, Corti S, Christensen HM, Juricke S, Subramanian A, et al. Climate Sphinx: Evaluating the Impact of Resolution and Stochastic Physics Parameterisations in the Ec-Earth Global Climate Model. Geosci Model Dev (2017) 10:1383–402. doi:10.5194/gmd-10-1383-2017
51. Christensen HM, Moroz IM, Palmer TN. Stochastic and Perturbed Parameter Representations of Model Uncertainty in Convection Parameterization*. J Atmos Sci (2015) 72:2525–44. doi:10.1175/jas-d-14-0250.1
52. Tagle F, Berner J, Grigoriu MD, Mahowald NM, Samorodnitsky G. Temperature Extremes in the Community Atmosphere Model with Stochastic Parameterizations*. J Clim (2016) 29:241–58. doi:10.1175/jcli-d-15-0314.1
53. Christensen HM, Lock SJ, Moroz IM, Palmer TN. Introducing Independent Patterns into the Stochastically Perturbed Parametrization Tendencies (SPPT) Scheme. Q.J.R Meteorol Soc (2017) 143:2168–81. doi:10.1002/qj.3075
54. Juricke S, Palmer TN, Zanna L. Stochastic Subgrid-Scale Ocean Mixing: Impacts on Low-Frequency Variability. J Clim (2017) 30:4997–5019. doi:10.1175/jcli-d-16-0539.1
55. Strommen K, Christensen HM, MacLeod D, Juricke S, Palmer T. Progress towards a Probabilistic Earth System Model: Examining the Impact of Stochasticity in Ec-Earth V3. 2. Geoscientific Model Develop (2019) 12. doi:10.5194/gmd-12-3099-2019
56. Palmer TN. Stochastic Weather and Climate Models. Nat Rev Phys (2019) 1:463–71. doi:10.1038/s42254-019-0062-2
57. Sakradzija M, Seifert A, Heus T. Fluctuations in a Quasi-Stationary Shallow Cumulus Cloud Ensemble. Nonlin Process. Geophys (2015) 22:65–85. doi:10.5194/npg-22-65-2015
58. Bengtsson L, Bao J-W, Pegion P, Penland C, Michelson S, Whitaker J. A Model Framework for Stochastic Representation of Uncertainties Associated with Physical Processes in NOAA's Next Generation Global Prediction System (NGGPS). Mon Wea Rev (2019) 147:893–911. doi:10.1175/mwr-d-18-0238.1
59. Dwivedi S, Franzke CLE, Lunkeit F. Energetically Consistent Stochastic and Deterministic Kinetic Energy Backscatter Schemes for Atmospheric Models. Q J Roy Meteorol Soc (2019) 145:1–11. doi:10.1002/qj.3625
60. Kravtsov S, Kondrashov D, Ghil M. Multilevel Regression Modeling of Nonlinear Processes: Derivation and Applications to Climatic Variability. J Clim (2005) 18:4404–24. doi:10.1175/jcli3544.1
61. Kondrashov D, Kravtsov S, Ghil M. Empirical Mode Reduction in a Model of Extratropical Low-Frequency Variability. J Atmos Sci (2006) 63:1859–77. doi:10.1175/jas3719.1
62. Crommelin DT, Vanden-Eijnden E. Fitting Timeseries by Continuous-Time Markov Chains: A Quadratic Programming Approach. J Comput Phys (2006) 217:782–805. doi:10.1016/j.jcp.2006.01.045
63. Crommelin D, Vanden-Eijnden E. Reconstruction of Diffusions Using Spectral Data from Timeseries. Commun Math Sci (2006) 4:651–68. doi:10.4310/cms.2006.v4.n3.a9
64. Crommelin D, Vanden-Eijnden E. Diffusion Estimation from Multiscale Data by Operator Eigenpairs. Multiscale Model Simul (2011) 9:1588–623. doi:10.1137/100795917
65. Crommelin D. Estimation of Space-dependent Diffusions and Potential Landscapes from Non-equilibrium Data. J Stat Phys (2012) 149:220–33. doi:10.1007/s10955-012-0597-4
66. Siegert S, Friedrich R, Peinke J. Analysis of Data Sets of Stochastic Systems. Phys Lett A (1998) 243:275–80. doi:10.1016/s0375-9601(98)00283-7
67. Siegert S, Friedrich R. Modeling of Nonlinear Lévy Processes by Data Analysis. Phys Rev E Stat Nonlin Soft Matter Phys (2001) 64:041107. doi:10.1103/PhysRevE.64.041107
68. Berner J. Linking Nonlinearity and Non-gaussianity of Planetary Wave Behavior by the Fokker-Planck Equation. J Atmos Sci (2005) 62:2098–117. doi:10.1175/jas3468.1
69. Hasselmann K. PIPs and POPs: The Reduction of Complex Dynamical Systems Using Principal Interaction and Oscillation Patterns. J Geophys Res (1988) 93:11015–21. doi:10.1029/jd093id09p11015
70. Achatz U, Schmitz G, Greisiger K-M. Principal Interaction Patterns in Baroclinic Wave Life Cycles. J Atmos Sci (1995) 52:3201–13. doi:10.1175/1520-0469(1995)052<3201:pipibw>2.0.co;2
71. Achatz U, Schmitz G. On the Closure Problem in the Reduction of Complex Atmospheric Models by Pips and Eofs: A Comparison for the Case of a Two-Layer Model with Zonally Symmetric Forcing. J Atmos Sci (1997) 54:2452–74. doi:10.1175/1520-0469(1997)054<2452:otcpit>2.0.co;2
72. Kwasniok F. The Reduction of Complex Dynamical Systems Using Principal Interaction Patterns. Physica D: Nonlinear Phenomena (1996) 92:28–60. doi:10.1016/0167-2789(95)00280-4
73. Kwasniok F. Empirical Low-Order Models of Barotropic Flow. J Atmos Sci (2004) 61:235–45. doi:10.1175/1520-0469(2004)061<0235:elmobf>2.0.co;2
74. Kwasniok F. Reduced Atmospheric Models Using Dynamically Motivated Basis Functions. J Atmos Sci (2007) 64:3452–74. doi:10.1175/jas4022.1
75. Crommelin DT, Majda AJ. Strategies for Model Reduction: Comparing Different Optimal Bases. J Atmos Sci (2004) 61:2206–17. doi:10.1175/1520-0469(2004)061<2206:sfmrcd>2.0.co;2
76. von Storch H, Bruns T, Fischer-Bruns I, Hasselmann K. Principal Oscillation Pattern Analysis of the 30- to 60-day Oscillation in General Circulation Model Equatorial Troposphere. J Geophys Res (1988) 93:11022–36. doi:10.1029/jd093id09p11022
77. von Storch H, Xu J. Principal Oscillation Pattern Analysis of the 30- to 60-day Oscillation in the Tropical Troposphere. Clim Dyn (1990) 4:175–90. doi:10.1007/bf00209520
78. von Storch H, Baumhefner DP. Principal Oscillation Pattern Analysis of the Tropical 30- to 60-day Oscillation. Clim Dyn (1991) 6:1–12. doi:10.1007/bf00210577
79. Schnur R, Schmitz G, Grieger N, von Storch H. Normal Modes of the Atmosphere as Estimated by Principal Oscillation Patterns and Derived from Quasigeostrophic Theory. J Atmos Sci (1993) 50:2386–400. doi:10.1175/1520-0469(1993)050<2386:nmotaa>2.0.co;2
80. Gehne M, Kleeman R, Trenberth KE. Irregularity and Decadal Variation in Enso: A Simplified Model Based on Principal Oscillation Patterns. Clim Dyn (2014) 43:3327–50. doi:10.1007/s00382-014-2108-6
81. Cash BA, Lee S. Observed Nonmodal Growth of the Pacific-North American Teleconnection Pattern. J Clim (2001) 14:1017–28. doi:10.1175/1520-0442(2001)014<1017:ongotp>2.0.co;2
82. Penland C. Random Forcing and Forecasting Using Principal Oscillation Pattern Analysis. Mon Wea Rev (1989) 117:2165–85. doi:10.1175/1520-0493(1989)117<2165:rfafup>2.0.co;2
83. Penland C, Magorian T. Prediction of Niño 3 Sea Surface Temperatures Using Linear Inverse Modeling. J Clim (1993) 6:1067–76. doi:10.1175/1520-0442(1993)006<1067:ponsst>2.0.co;2
84. Penland C, Sardeshmukh PD. The Optimal Growth of Tropical Sea Surface Temperature Anomalies. J Clim (1995) 8:1999–2024. doi:10.1175/1520-0442(1995)008<1999:togots>2.0.co;2
85. Penland C. A Stochastic Model of Indopacific Sea Surface Temperature Anomalies. Physica D: Nonlinear Phenomena (1996) 98:534–58. doi:10.1016/0167-2789(96)00124-8
86. Alexander MA, Matrosova L, Penland C, Scott JD, Chang P. Forecasting pacific Ssts: Linear Inverse Model Predictions of the Pdo. J Clim (2008) 21:385–402. doi:10.1175/2007jcli1849.1
87. Newman M, Sardeshmukh PD, Penland C. Stochastic Forcing of the Wintertime Extratropical Flow. J Atmos Sci (1997) 54:435–55. doi:10.1175/1520-0469(1997)054<0435:sfotwe>2.0.co;2
88. Lou J, O’Kane TJ, Holbrook NJ. A Linear Inverse Model of Tropical and South pacific Climate Variability: Optimal Structure and Stochastic Forcing. J Clim (2021) 34:143–55. doi:10.1175/JCLI-D-19-0964.1
89. Koopman BO. Hamiltonian Systems and Transformation in hilbert Space. Proc Natl Acad Sci U.S.A (1931) 17:315–8. doi:10.1073/pnas.17.5.315
91. H. Tu J, Rowley CW, W. Rowley C, M. Luchtenburg D, L. Brunton S, Nathan Kutz J. On Dynamic Mode Decomposition: Theory and Applications. J Comput Dyn (2014) 1:391–421. doi:10.3934/jcd.2014.1.391
92. Kutz JN, Brunton SL, Brunton BW, Proctor JL. Dynamic Mode Decomposition. Philadelphia, PA: Society for Industrial and Applied Mathematics (2016). doi:10.1137/1.9781611974508
93. Schmid PJ. Dynamic Mode Decomposition of Numerical and Experimental Data. J Fluid Mech (2010) 656:5–28. doi:10.1017/S0022112010001217
94. Schmid PJ, Li L, Juniper MP, Pust O. Applications of the Dynamic Mode Decomposition. Theor Comput Fluid Dyn (2011) 25:249–59. doi:10.1007/s00162-010-0203-9
95. Kutz JN, Fu X, Brunton SL. Multiresolution Dynamic Mode Decomposition. SIAM J Appl Dyn Syst (2016) 15:713–35. doi:10.1137/15m1023543
96. Gottwald GA, Gugole F. Detecting Regime Transitions in Time Series Using Dynamic Mode Decomposition. J Stat Phys (2019) 2019:1–18. doi:10.1007/s10955-019-02392-3
97. Gugole F, Franzke CL. Spatial Covariance Modeling for Stochastic Subgrid-Scale Parameterizations Using Dynamic Mode Decomposition. J Adv Mod Earth Sys (2020) 12:e2020MS002115. doi:10.1029/2020ms002115
98. Hasselmann K. Nonlinear Interactions Treated by the Methods of Theoretical Physics (With Application to the Generation of Waves by Wind). Proc R Soc Lond A (1967) 299:77–103. doi:10.1098/rspa.1967.0124
99. Phillips OM. On the Generation of Waves by Turbulent Wind. J Fluid Mech (1957) 2:417–45. doi:10.1017/s0022112057000233
100. Phillips OM. The Scattering of Gravity Waves by Turbulence. J Fluid Mech (1959) 5:177–92. doi:10.1017/s0022112059000143
101. Phillips OM. On the Dynamics of Unsteady Gravity Waves of Finite Amplitude Part 1. The Elementary Interactions. J Fluid Mech (1960) 9:193–217. doi:10.1017/s0022112060001043
102. Miles JW. On the Generation of Surface Waves by Shear Flows. J Fluid Mech (1957) 3:185–204. doi:10.1017/s0022112057000567
103. Miles JW. On the Generation of Surface Waves by Turbulent Shear Flows. J Fluid Mech (1960) 7:469–78. doi:10.1017/s0022112060000220
104. Gilchrist AWR. The Directional Spectrum of Ocean Waves: an Experimental Investigation of Certain Predictions of the Miles-Phillips Theory of Wave Generation. J Fluid Mech (1965) 25:795–816.
105. Olbers DJ. Nonlinear Energy Transfer and the Energy Balance of the Internal Wave Field in the Deep Ocean. J Fluid Mech (1976) 74:375–99. doi:10.1017/s0022112076001857
106. Frederiksen JS. Interactions of Nonlinear Internal Gravity Waves and Turbulence. Ann Geophysicae (1984) 2:421–32.
107. Finnigan JJ. Kinetic Energy Transfer between Internal Gravity Waves and Turbulence. J Atmos Sci (1988) 45:486–505. doi:10.1175/1520-0469(1988)045<0486:ketbig>2.0.co;2
108. Carnevale GF, Frederiksen Jr. S. A Statistical Dynamical Theory of Strongly Nonlinear Internal Gravity Waves. Geophys Astrophysical Fluid Dyn (1983) 23:175–207. doi:10.1080/03091928308209042
109. Frederiksen JS, O’Kane TJ. Markovian Inhomogeneous Closures for Rossby Waves and Turbulence over Topography. J Fluid Mech (2019) 858:45–70. doi:10.1017/jfm.2018.784
110. Kraichnan RH. The Structure of Isotropic Turbulence at Very High reynolds Numbers. J Fluid Mech (1959) 5:497–543. doi:10.1017/s0022112059000362
111. McComb WD. A Local Energy-Transfer Theory of Isotropic Turbulence. J Phys A: Math Nucl Gen (1974) 7:632–49. doi:10.1088/0305-4470/7/5/013
112. Holloway G, Hendershott MC. Stochastic Closure for Nonlinear Rossby Waves. J Fluid Mech (1977) 82:747–65. doi:10.1017/s0022112077000962
113. Carnevale GF, Martin PC. Field Theoretical Techniques in Statistical Fluid Dynamics: With Application to Nonlinear Wave Dynamics. Geophys Astrophysical Fluid Dyn (1982) 20:131–63. doi:10.1080/03091928208209002
114. Newell AC, Rumpf B. Wave Turbulence. Annu Rev Fluid Mech (2011) 43:59–78. doi:10.1146/annurev-fluid-122109-160807
115. Sagaut P, Cambon C. Homogeneous Turbulence Dynamics. Berlin, Germany: Springer Nature (2018). doi:10.1007/978-3-319-73162-9
116. Kraichnan RH. Decay of Isotropic Turbulence in the Direct-Interaction Approximation. Phys Fluids (1964) 7:1030–48. doi:10.1063/1.1711319
117. Wyld HW. Formulation of the Theory of Turbulence in an Incompressible Fluid. Ann Phys (1961) 14:143–65. doi:10.1016/0003-4916(61)90056-2
118. Lee LL. A Formulation of the Theory of Isotropic Hydromagnetic Turbulence in an Incompressible Fluid. Ann Phys (1965) 32:292–321. doi:10.1016/0003-4916(65)90019-9
119. Herring JR. Self-consistent-field Approach to Turbulence Theory. Phys Fluids (1965) 8:2219–25. doi:10.1063/1.1761185
120. Herring JR. Self-consistent-field Approach to Nonstationary Turbulence. Phys Fluids (1966) 9:2106–10. doi:10.1063/1.1761579
121. Martin PC, Siggia ED, Rose HA. Statistical Dynamics of Classical Systems. Phys Rev A (1973) 8:423–37. doi:10.1103/physreva.8.423
122. Phythian R. The Operator Formalism of Classical Statistical Dynamics. J Phys A: Math Gen (1975) 8:1423–32. doi:10.1088/0305-4470/8/9/011
123. Jensen RV. Functional Integral Approach to Classical Statistical Dynamics. J Stat Phys (1981) 25:183–210. doi:10.1007/bf01022182
124. Okane TJ, Frederiksen JS. The Qdia and Regularized Qdia Closures for Inhomogeneous Turbulence over Topography. J Fluid Mech (2004) 504:133–65. doi:10.1017/S0022112004007980
125. Frederiksen JS, O'Kane TJ. Inhomogeneous Closure and Statistical Mechanics for Rossby Wave Turbulence over Topography. J Fluid Mech (2005) 539:137–65. doi:10.1017/S0022112005005562
126. Frederiksen JS. Subgrid-scale Parameterizations of Eddy-Topographic Force, Eddy Viscosity, and Stochastic Backscatter for Flow over Topography. J Atmos Sci (1999) 56:1481–94. doi:10.1175/1520-0469(1999)056<1481:sspoet>2.0.co;2
127. O'Kane TJ, Frederiksen JS. Statistical Dynamical Subgrid-Scale Parameterizations for Geophysical Flows. Phys Scr (2008) T132:014033. doi:10.1088/0031-8949/2008/T132/014033
128. Zidikheri MJ, Frederiksen JS. Stochastic Subgrid-Scale Modelling for Non-equilibrium Geophysical Flows. Phil Trans R Soc A (2010) 368:145–60. doi:10.1098/rsta.2009.0192
129. Frederiksen JS, Kitsios V, O’Kane TJ, Zidikheri MJ. Stochastic Subgrid Modelling for Geophysical and Three-Dimensional Turbulence. In: CLE Franzke, and T O’Kane, editors. Nonlinear and Stochastic Climate Dynamics. Cambridge: Cambridge University Press (2017). p. 241–75.
130. Kitsios V, Frederiksen JS. Subgrid Parameterizations of the Eddy-Eddy, Eddy-Mean Field, Eddy-Topographic, Mean Field-Mean Field, and Mean Field-Topographic Interactions in Atmospheric Models. J Atmos Sci (2019) 76:457–77. doi:10.1175/jas-d-18-0255.1
131. Zhou Y. Turbulence Theories and Statistical Closure Approaches. Phys Rep (2021) 935:1–117. doi:10.1016/j.physrep.2021.07.001
132. Frisch U, Parisi G (1985). Turbulence and Predictability of Geophysical Flows and Climate Dynamics. In Proceedings of the International School of Physics “Enrico Fermi,” Course LXXXVIII; Varenna. p. 1983.
133. Frisch U. In: N Ghil, R Benzi, and G Parisi, editors. Turbulence and Predictability of Geophysical Flows and Climate Dynamics, Varenna Summer School LXXXVIII (1983).
134. Lovejoy S, Schertzer D. The Weather and Climate: Emergent Laws and Multifractal Cascades. Cambridge: Cambridge University Press (2018).
135. Roşu IA, Cazacu MM, Ghenadi AS, Bibire L, Agop M. On a Multifractal Approach of Turbulent Atmosphere Dynamics. Front Earth Sci (2020) 8:216.
136. Venugopal V, Basu S, Foufoula-Georgiou E. A New Metric for Comparing Precipitation Patterns with an Application to Ensemble Forecasts. J Geophys Res Atmospheres (2005) 110. doi:10.1029/2004jd005395
137. Lovejoy S. Scaling Fluctuation Analysis and Statistical Hypothesis Testing of Anthropogenic Warming. Clim Dyn (2014) 42:2339–51. doi:10.1007/s00382-014-2128-2
138. Castellani T, Cavagna A. Spin-glass Theory for Pedestrians. J Stat Mech (2005) 2005:P05012. doi:10.1088/1742-5468/2005/05/p05012
139. Kurchan J, Parisi G, Virasoro MA. Barriers and Metastable States as Saddle Points in the Replica Approach. J Phys France (1993) 3:1819–38. doi:10.1051/jp1:1993217
140. Parisi G. Order Parameter for Spin-Glasses. Phys Rev Lett (1983) 50:1946–8. doi:10.1103/physrevlett.50.1946
142. Mézard M, Parisi G, Sourlas N, Toulouse G, Virasoro M. Nature of the Spin-Glass Phase. Phys Rev Lett (1984) 52:1156.
143. Pleimling M, Schmittmann B, Zia RKP. Convection Cells Induced by Spontaneous Symmetry Breaking. Europhys Lett (2010) 89:50001. doi:10.1209/0295-5075/89/50001
144. Ma Y-P, Sudakov I, Strong C, Golden KM. Ising Model for Melt Ponds on Arctic Sea Ice. New J Phys (2019) 21:063029. doi:10.1088/1367-2630/ab26db
145. Khouider B. A Coarse Grained Stochastic Multi-type Particle Interacting Model for Tropical Convection: Nearest Neighbour Interactions. Commun Math Sci (2014) 12:1379–407. doi:10.4310/cms.2014.v12.n8.a1
146. Hoffman PF, Kaufman AJ, Halverson GP, Schrag DP. A Neoproterozoic Snowball Earth. Science (1998) 281:1342–6. doi:10.1126/science.281.5381.1342
147. Lucarini V, Bódai T. Transitions across Melancholia States in a Climate Model: Reconciling the Deterministic and Stochastic Points of View. Phys Rev Lett (2019) 122:158701. doi:10.1103/physrevlett.122.158701
148. Lucarini V, Fraedrich K, Lunkeit F. Thermodynamic Analysis of Snowball Earth Hysteresis experiment: Efficiency, Entropy Production and Irreversibility. Q.J.R Meteorol Soc (2010) 136:2–11. doi:10.1002/qj.543
149. Cheng X, Wallace JM. Cluster Analysis of the Northern Hemisphere Wintertime 500-hpa Height Field: Spatial Patterns. J Atmos Sci (1993) 50:2674–96. doi:10.1175/1520-0469(1993)050<2674:caotnh>2.0.co;2
150. Kimoto M, Ghil M. Multiple Flow Regimes in the Northern Hemisphere winter. Part I: Methodology and Hemispheric Regimes. J Atmos Sci (1993) 50:2625. doi:10.1175/1520-0469(1993)050<2625:mfritn>2.0.co;2
151. Ghil M, Robertson AW. "Waves" vs. "particles" in the Atmosphere's Phase Space: A Pathway to Long-Range Forecasting? Proc Natl Acad Sci U.S.A (2002) 99:2493–500. doi:10.1073/pnas.012580899
152. Majda AJ, Franzke CL, Fischer A, Crommelin DT. Distinct Metastable Atmospheric Regimes Despite Nearly Gaussian Statistics: A Paradigm Model. Proc Natl Acad Sci U.S.A (2006) 103:8309–14. doi:10.1073/pnas.0602641103
153. Horenko I. On the Identification of Nonstationary Factor Models and Their Application to Atmospheric Data Analysis. J Atmos Sci (2010) 67:1559–74. doi:10.1175/2010jas3271.1
154. Franzke C, Crommelin D, Fischer A, Majda AJ. A Hidden Markov Model Perspective on Regimes and Metastability in Atmospheric Flows. J Clim (2008) 21:1740–57. doi:10.1175/2007jcli1751.1
155. Franzke C, Horenko I, Majda AJ, Klein R. Systematic Metastable Atmospheric Regime Identification in an Agcm. J Atmos Sci (2009) 66:1997–2012. doi:10.1175/2009jas2939.1
156. Risbey JS, O’Kane TJ, Monselesan DP, Franzke C, Horenko I. Metastability of Northern Hemisphere Teleconnection Modes. J Atmos Sci (2015) 72:35–54. doi:10.1175/jas-d-14-0020.1
157. Hannachi A, Straus DM, Franzke CLE, Corti S, Woollings T. Low-frequency Nonlinearity and Regime Behavior in the Northern Hemisphere Extratropical Atmosphere. Rev Geophys (2017) 55:199–234. doi:10.1002/2015RG000509
158. O’Kane TJ, Risbey JS, Franzke CLE, Horenko I, Monselesan D. Changes in the Metastability of the Midlatitude Southern Hemisphere Circulation and the Utility of Nonstationary Cluster Analysis and Split-Flow Blocking Indices as Diagnostic Tools. J Atmos Sci (2013) 70:824–42.
159. Quinn CR, O’Kane TJ, Harries D. Dynamical Analysis of a Reduced Model for the north atlantic Oscillation. J Atmos Sci (2021) 78:1647–71. doi:10.1175/jas-d-20-0282.1
160. Quinn C, O’Kane TJ, Harries D. Systematic Calculation of Finite-Time Mixed Singular Vectors and Characterization of Error Growth for Persistent Coherent Atmospheric Disturbances over Eurasia. Chaos (2022) 32:023126. doi:10.1063/5.0066150
161. Milankovitch M. Mathematische klimalehre und astronomische theorie der klimaschwankungen. Handbuch der Klimatologie (1930) 1.
162. Sutera A. On Stochastic Perturbation and Long-Term Climate Behaviour. Q J R Meteorol Soc (1981) 107:137–51.
163. Budyko MI. The Effect of Solar Radiation Variations on the Climate of the Earth. Tellus (1969) 21:611–9. doi:10.3402/tellusa.v21i5.10109
164. Sellers WD. A Global Climatic Model Based on the Energy Balance of the Earth-Atmosphere System. J Appl Meteorol (1969) 8:392–400. doi:10.1175/1520-0450(1969)008<0392:agcmbo>2.0.co;2
165. Nicolis C, Nicolis G. Stochastic Aspects of Climatic Transitions-Additive Fluctuations. Tellus (1981) 33:225–34. doi:10.3402/tellusa.v33i3.10710
166. Benzi R, Sutera A, Vulpiani A. The Mechanism of Stochastic Resonance. J Phys A: Math Gen (1981) 14:L453–L457. doi:10.1088/0305-4470/14/11/006
167. Benzi R, Parisi G, Sutera A, Vulpiani A. Stochastic Resonance in Climatic Change. Tellus (1982) 34:10–6. doi:10.1111/j.2153-3490.1982.tb01787.x
168. Bhattacharya K, Ghil M. An Energy-Balance Model with Multiply-Periodic and Quasi-Chaotic Free Oscillations. In: Evolution of planetary atmospheres and climatology of the earth; International Conference (1979).
169. Bhattacharya K, Ghil M, Vulis IL. Internal Variability of an Energy-Balance Model with Delayed Albedo Effects. J Atmos Sci (1982) 39:1747–73. doi:10.1175/1520-0469(1982)039<1747:ivoaeb>2.0.co;2
170. Benzi R. Stochastic Resonance: from Climate to Biology. Nonlin Process. Geophys (2010) 17:431–41. doi:10.5194/npg-17-431-2010
171. Benzi R. Flow Reversal in a Simple Dynamical Model of Turbulence. Phys Rev Lett (2005) 95:024502. doi:10.1103/PhysRevLett.95.024502
172. Gammaitoni L, Hänggi P, Jung P, Marchesoni F. Stochastic Resonance. Rev Mod Phys (1998) 70:223–87. doi:10.1103/revmodphys.70.223
173. Ganopolski A, Rahmstorf S. Abrupt Glacial Climate Changes Due to Stochastic Resonance. Phys Rev Lett (2002) 88:038501. doi:10.1103/PhysRevLett.88.038501
174. Crucifix M. Oscillators and Relaxation Phenomena in Pleistocene Climate Theory. Phil Trans R Soc A (2012) 370:1140–65. doi:10.1098/rsta.2011.0315
175. Timmermann A, Gildor H, Schulz M, Tziperman E. Coherent Resonant Millennial-Scale Climate Oscillations Triggered by Massive Meltwater Pulses. J Clim (2003) 16:2569–85. doi:10.1175/1520-0442(2003)016<2569:crmcot>2.0.co;2
176. Braun H, Ganopolski A, Christl M, Chialvo DR. A Simple Conceptual Model of Abrupt Glacial Climate Events. Nonlin Process. Geophys (2007) 14:709–21. doi:10.5194/npg-14-709-2007
177. Braun H, Kurths J. Were Dansgaard-Oeschger Events Forced by the Sun? Eur Phys J Spec Top (2010) 191:117–29. doi:10.1140/epjst/e2010-01345-5
178. Ragone F, Wouters J, Bouchet F. Computation of Extreme Heat Waves in Climate Models Using a Large Deviation Algorithm. Proc Natl Acad Sci U.S.A (2018) 115:24–9. doi:10.1073/pnas.1712645115
179.[Dataset] De Domenico M, Vulpiani A. Understanding Climate and Turbulence: The Mark of Giorgio Parisi (2021). Available from: https://www.nature.com/articles/d43978-021-00128-0 (Accessed April 22, 2022).
180. Manabe S. Climate and the Ocean Circulation 1. Mon Wea Rev (1969) 97:739–74. doi:10.1175/1520-0493(1969)097<0739:catoc>2.3.co;2
181. Manabe S. Climate and the Ocean Circulation. Mon Wea Rev (1969) 97:775–805. doi:10.1175/1520-0493(1969)097<0775:catoc>2.3.co;2
182. Manabe S, Wetherald RT. Thermal Equilibrium of the Atmosphere with a Given Distribution of Relative Humidity. J Atmos Sci (1967) 24:241–59. doi:10.1175/1520-0469(1967)024<0241:teotaw>2.0.co;2
183. Roe GH, Baker MB. Why Is Climate Sensitivity So Unpredictable? Science (2007) 318:629–32. doi:10.1126/science.1144735
184. Sherwood SC, Webb MJ, Annan JD, Armour KC, Forster PM, Hargreaves JC, et al. An Assessment of Earth's Climate Sensitivity Using Multiple Lines of Evidence. Rev Geophys (2020) 58:e2019RG000678. doi:10.1029/2019RG000678
185. Meehl GA, Senior CA, Eyring V, Flato G, Lamarque JF, Stouffer RJ, et al. Context for Interpreting Equilibrium Climate Sensitivity and Transient Climate Response from the Cmip6 Earth System Models. Sci Adv (2020) 6:eaba1981. doi:10.1126/sciadv.aba1981
186. Nijsse FJMM, Cox PM, Williamson MS. Emergent Constraints on Transient Climate Response (Tcr) and Equilibrium Climate Sensitivity (Ecs) from Historical Warming in Cmip5 and Cmip6 Models. Earth Syst Dynam (2020) 11:737–50. doi:10.5194/esd-11-737-2020
187. Nordhaus WD. Estimates of the Social Cost of Carbon: Background and Results from the RICE-2011 Model. In: Tech. Rep. Cambridge, MA, USA: National Bureau of Economic Research (2011). doi:10.3386/w17540
188. Petschel-Held G, Schellnhuber H-J, Bruckner T, Tóth FL, Hasselmann K. The Tolerable Windows Approach: Theoretical and Methodological Foundations. Climatic Change (1999) 41:303–31. doi:10.1023/a:1005487123751
189. Czupryna M, Franzke CLE, Hokamp S, Scheffran J. An Agent-Based Approach to Integrated Assessment Modelling of Climate Change. J Artif Soc. Soc Simu. (2020) 23:1–7. doi:10.18564/jasss.4325
190. Waldhoff S, Anthoff D, Rose S, Tol RS. The Marginal Damage Costs of Different Greenhouse Gases: An Application of Fund. Economics (2014) 8:1. doi:10.5018/economics-ejournal.ja.2014-31
191. Weitzman ML. On Modeling and Interpreting the Economics of Catastrophic Climate Change. Rev Econ Stat (2009) 91:1–19. doi:10.1162/rest.91.1.1
192. Dietz S, van der Ploeg F, Rezai A, Venmans F. Are Economists Getting Climate Dynamics Right and Does it Matter? J Assoc Environ Resource Economists (2021) 8:895–921. doi:10.1086/713977
193. Manabe S, Stouffer RJ. Sensitivity of a Global Climate Model to an Increase of CO2concentration in the Atmosphere. J Geophys Res (1980) 85:5529–54. doi:10.1029/jc085ic10p05529
194. Manabe S, Stouffer RJ, Spelman MJ, Bryan K. Transient Responses of a Coupled Ocean-Atmosphere Model to Gradual Changes of Atmospheric CO2. Part I. Annual Mean Response. J Clim (1991) 4:785–818. doi:10.1175/1520-0442(1991)004<0785:troaco>2.0.co;2
195. Sarmiento JL, Hughes TMC, Stouffer RJ, Manabe S. Simulated Response of the Ocean Carbon Cycle to Anthropogenic Climate Warming. Nature (1998) 393:245–9. doi:10.1038/30455
196. Manabe S, Stouffer RJ. Simulation of Abrupt Climate Change Induced by Freshwater Input to the north atlantic Ocean. Nature (1995) 378:165–7. doi:10.1038/378165a0
197. Manabe S, Broccoli AJ. The Influence of continental Ice Sheets on the Climate of an Ice Age. J Geophys Res (1985) 90:2167–90. doi:10.1029/jd090id01p02167
198. Manabe S, Stouffer RJ. Study of Abrupt Climate Change by a Coupled Ocean-Atmosphere Model. Quat Sci Rev (2000) 19:285–99. doi:10.1016/s0277-3791(99)00066-9
199. Broecker WS. Abrupt Climate Change: Causal Constraints provided by the Paleoclimate Record. Earth-Science Rev (2000) 51:137–54. doi:10.1016/s0012-8252(00)00019-2
200. Lenton TM, Schellnhuber HJ. Tipping the Scales. Nat Clim Change (2007) 1:97–8. doi:10.1038/climate.2007.65
201. Boers N. Observation-based Early-Warning Signals for a Collapse of the atlantic Meridional Overturning Circulation. Nat Clim Chang (2021) 11:680–8. doi:10.1038/s41558-021-01097-4
202.European Commission. Destination Earth. Tech Rep (2021). Available from: https://digital-strategy.ec.europa.eu/en/policies/destination-earth (Accessed 04 17, 2022).
203. Holm DD. Variational Principles for Stochastic Fluid Dynamics. Proc R Soc A (2015) 471:20140963. doi:10.1098/rspa.2014.0963
204. Cotter C, Crisan D, Holm DD, Pan W, Shevchenko I. Numerically Modeling Stochastic Lie Transport in Fluid Dynamics. Multiscale Model Simul (2019) 17:192–232. doi:10.1137/18m1167929
205. Mémin E. Fluid Flow Dynamics under Location Uncertainty. Geophys Astrophysical Fluid Dyn (2014) 108:119–46.
206. Resseguier V, Mémin E, Chapron B. Geophysical Flows under Location Uncertainty, Part I Random Transport and General Models. Geophys Astrophysical Fluid Dyn (2017) 111:149–76. doi:10.1080/03091929.2017.1310210
207. Jansen MF, Held IM. Parameterizing Subgrid-Scale Eddy Effects Using Energetically Consistent Backscatter. Ocean Model (2014) 80:36–48. doi:10.1016/j.ocemod.2014.06.002
208. Jansen MF, Held IM, Adcroft A, Hallberg R. Energy Budget-Based Backscatter in an Eddy Permitting Primitive Equation Model. Ocean Model (2015) 94:15–26. doi:10.1016/j.ocemod.2015.07.015
209. Zurita-Gotor P, Held IM, Jansen MF. Kinetic Energy-Conserving Hyperdiffusion Can Improve Low Resolution Atmospheric Models. J Adv Model Earth Syst (2015) 7:1117–35. doi:10.1002/2015ms000480
210. Gugole F, Franzke CLE. Numerical Development and Evaluation of an Energy Conserving Conceptual Stochastic Climate Model. Math Clim Wea Forecast (2019) 5:45–64. doi:10.1515/mcwf-2019-0004
211. Frank JE, Gottwald GA. Stochastic Homogenization for an Energy Conserving Multi-Scale Toy Model of the Atmosphere. Physica D: Nonlinear Phenomena (2013) 254:46–56. doi:10.1016/j.physd.2013.03.010
212. Gottwald G, Crommelin D, Franzke CLE. Stochastic Climate Theory. In: CLE Franzke, and T O’Kane, editors. Nonlinear and Stochastic Climate Dynamics. Cambridge: Cambridge University Press (2017). p. 209–40.
213. Franzke CLE, Graves T, Watkins NW, Gramacy RB, Hughes C. Robustness of Estimators of Long-Range Dependence and Self-Similarity under Non-gaussianity. Phil Trans R Soc A (2012) 370:1250–67. doi:10.1098/rsta.2011.0349
214. Penland C, Ewald BD. On Modelling Physical Systems with Stochastic Models: Diffusion versus Lévy Processes. Phil Trans R Soc A (2008) 366:2455–74. doi:10.1098/rsta.2008.0051
215. Penland C, Sardeshmukh PD. Alternative Interpretations of Power-Law Distributions Found in Nature. Chaos (2012) 22:023119. doi:10.1063/1.4706504
216. Thompson WF, Kuske RA, Monahan AH. Stochastic Averaging of Dynamical Systems with Multiple Time Scales Forced with $\alpha$-Stable Noise. Multiscale Model Simul (2015) 13:1194–223. doi:10.1137/140990632
217. Zelinka MD, Myers TA, McCoy DT, Po-Chedley S, Caldwell PM, Ceppi P, et al. Causes of Higher Climate Sensitivity in Cmip6 Models. Geophys Res Lett (2020) 47:e2019GL085782. doi:10.1029/2019gl085782
Keywords: climate change, climate modeling, stochastic climate model, subgrid-scale parameterization, stochastic resonance, complexity science, model reduction
Citation: Franzke CLE, Blender R, O’Kane TJ and Lembo V (2022) Stochastic Methods and Complexity Science in Climate Research and Modeling. Front. Phys. 10:931596. doi: 10.3389/fphy.2022.931596
Received: 29 April 2022; Accepted: 16 May 2022;
Published: 06 June 2022.
Edited by:
Jingfang Fan, Beijing Normal University, ChinaReviewed by:
Qimin Deng, China University of Geosciences Wuhan, ChinaCopyright © 2022 Franzke, Blender, O’Kane and Lembo. This is an open-access article distributed under the terms of the Creative Commons Attribution License (CC BY). The use, distribution or reproduction in other forums is permitted, provided the original author(s) and the copyright owner(s) are credited and that the original publication in this journal is cited, in accordance with accepted academic practice. No use, distribution or reproduction is permitted which does not comply with these terms.
*Correspondence: Christian L. E. Franzke, Y2hyaXN0aWFuLmZyYW56a2VAcHVzYW4uYWMua3I=
Disclaimer: All claims expressed in this article are solely those of the authors and do not necessarily represent those of their affiliated organizations, or those of the publisher, the editors and the reviewers. Any product that may be evaluated in this article or claim that may be made by its manufacturer is not guaranteed or endorsed by the publisher.
Research integrity at Frontiers
Learn more about the work of our research integrity team to safeguard the quality of each article we publish.