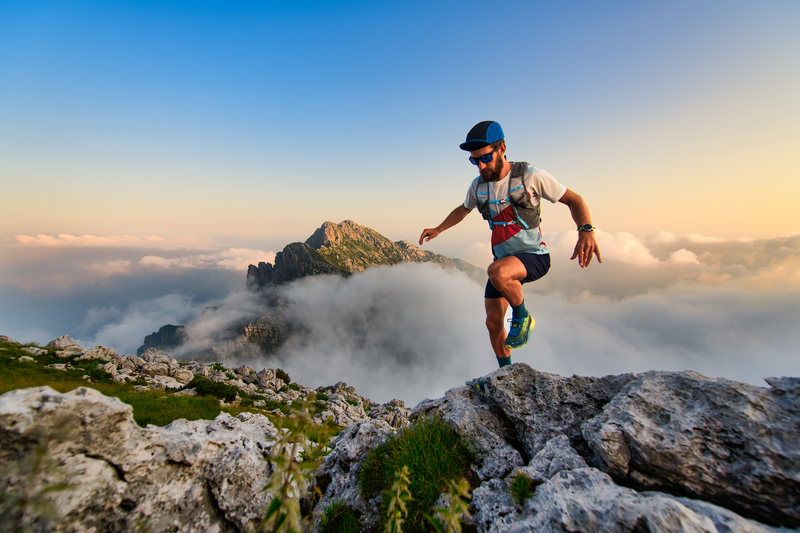
95% of researchers rate our articles as excellent or good
Learn more about the work of our research integrity team to safeguard the quality of each article we publish.
Find out more
ORIGINAL RESEARCH article
Front. Phys. , 01 July 2022
Sec. Biophysics
Volume 10 - 2022 | https://doi.org/10.3389/fphy.2022.904836
This article is part of the Research Topic Active Matter in Complex Environments View all 12 articles
Biota are found in glaciers, ice sheets and permafrost. Ice bound micro-organisms evolve in a complex mobile environment facilitated or hindered by a range of bulk and surface interactions. When a particle is embedded in a host solid near its bulk melting temperature, a melted film forms at the surface of the particle in a process known as interfacial premelting. Under a temperature gradient, the particle is driven by a thermomolecular pressure gradient toward regions of higher temperatures in a process called thermal regelation. When the host solid is ice and the particles are biota, thriving in their environment requires the development of strategies, such as producing exopolymeric substances (EPS) and antifreeze glycoproteins (AFP) that enhance the interfacial water. Therefore, thermal regelation is enhanced and modified by a process we term bio-enhanced premelting. Additionally, the motion of bioparticles is influenced by chemical gradients influenced by nutrients within the icy host body. We show how the overall trajectory of bioparticles is controlled by a competition between thermal regelation and directed biolocomotion. By re-casting this class of regelation phenomena in the stochastic framework of active Ornstein-Uhlenbeck dynamics, and using multiple scales analysis, we find that for an attractive (repulsive) nutrient source, that thermal regelation is enhanced (suppressed) by biolocomotion. This phenomena is important in astrobiology, the biosignatures of extremophiles and in terrestrial paleoclimatology.
Ice sheets are an essential reservoir of information on past climate and they contain an important record of micro-organisms on Earth, recording ice microbes and their viruses over long periods [1, 2]. In these extreme environments, the abundance of virus is well correlated with bacterial abundance, but is 10–100 times lower than in temperate aquatic ecosystems [3]. Even in these harsh conditions, the virus infection rate is relatively high [4], leading to the expectation of low long-term survival rates. However, recent studies have shown that some viruses develop survival strategies to maintain a long-term relationship with their hosts [4, 5], possibly up to thousands of years [6]. For example, viruses such as bacteriophages can switch to a lysogenic life strategy enabling them to replicate and maintain themselves in the bacterial population without lysis over multiple generations [4]. Moreover, among these viruses some can provide immunity to their hosts against other viruses [4, 7], or manipulate their metabolism to facilitate nutrient acquisition by affecting motility genes [6]. Indeed, motile biota are found to be active in ice for substantial periods. For example, recently a 30,000 year old giant virus Pithovirus sibericum was found in permafrost along with microbes and nematodes, and viable bacteria have been found in 750,000 year old glacial ice. Basal ice often contains subglacial debris and sediment, which serve as a source of nutrients and organic matter, providing a habitat for micro-organisms adapted to subfreezing conditions [8, 9]. Additionally, the microbiomes of sediment rich basal ices are distinct from those found in glacial ice and are equivalent to those found in permafrost [8], expanding the nature of subfreezing habitats.
Ice cores provide the highest resolution records of past climate states [10–15]. Of particular relevance to our study is their role as a refuge for micro-organisms, from the recent past [16, 17] to millennia [18–20]. Ice microbes are taxonomically diverse and have a wide range of taxonomic relatives [9, 19, 21–23]. Common algae taxa are centric and pennate diatoms, dinoflagellates and flagellates [24–26], whereas common bacterial taxa are pseudomonadota, actinobacteria, firmicutes and bacteroidetes [6, 27]. Many of these microbes have different motility mechanisms [28, 29] from swimming (e.g., Chlamydomonas nivalis [30] or Methylobacterium [6, 31, 32]) to gliding (e.g., diatoms [33, 34] or Bacillus subtilis [19, 35]), which can be used to assess their locomotion. Examples of biological proxies include diatoms [36] and bacteria colonies [37, 38], reflecting a unique range of physical-biological interactions in the climate system. Therefore, understanding the relationship between the evolution of ice bound micro-organisms and proxy dating methods is a key aspect of understanding the covariation of life and climate.
Finally, such understanding is essential for the study of extraterrestrial life. In our own solar system, despite the debate regarding the existence of bulk water on Mars [39], thin water films, such as those studied here, hold the most potential for harboring life under extreme conditions. Indeed, lipids, nucleic acids, and amino acids influenced by active motility may serve as biosignatures of extra terrestial life. Combining measurements of diffusivity-characterized-motility [40, 41] with bioparticle distribution observed on Earth, provides crucial information for development of new instrumentation to detect the presence of extra terrestrial life [41, 42]. Indeed, recently micro-organisms trapped in primary fluid inclusions in halite for millions of years have been discovered [43], providing promise for both terrestrial and extraterrestrial biosignature detection.
When a particle is embedded in ice near the bulk melting temperature, the ice may melt at the particle-ice surface in a process known as interfacial premelting [44]. The thickness of the melt film depends on the temperature, impurities, material properties and geometry. A temperature gradient is accompanied by a thermomolecular pressure gradient that drives the interfacial liquid from high to low temperatures, and hence the particle migrates from low to high temperatures in a process called thermal regelation [44–48]. Thermal regelation of inert particles plays a major role in the redistribution of material inside of ice, which has important environmental and composite materials implications [44–48]. Moreover, surface properties are central to the fact that extremophile organisms in Earth’s cryosphere–glaciers, sea ice and permafrost–develop strategies to persist in challenging environments. Indeed, many biological organisms secrete exopolymeric substance (EPS) [49] or harness antifreeze glycoproteins (AFP) [50, 51] to maintain interfacial liquidity. For example, sea ice houses an array of algae and bacteria, some of which produce EPS to protect them at low temperature and high salinity [52, 53]. Additionally, the enhanced liquidity associated with high concentrations of EPS alters the physical properties of sea ice and thereby play a role in climate change [54, 55].
In bulk solution, active particles act as simple microscopic models for living systems and are particularly accurate at mimicking the propulsion of bacteria or algae [56–60]. By converting energy to motion using biological, chemical, or physical processes, they exhibit rich collective emergent motion from ostensibly simple rules [61, 62]. Algae and bacteria operate in complex geometries and translate environmental conditions into microscopic information that guides their behavior. Examples of such information include quorum sensing (e.g., particle population density), used by bacteria to regulate biofilm formation, defense against competitors and adapt to changing environments [63–65]; chemotaxis (e.g., concentration gradients of nutrients), used by algae/bacteria to direct their motion toward higher concentrations of beneficial, or lower concentrations of toxic, chemicals [66–70]. It is important to emphasize that factors such as surface adhesion, salinity, the segregation of impurities of all types from the ice lattice, among other factors [67, 69, 71, 72], make our treatment of chemotaxis a simplified starting point. However, field samples and laboratory experiments have shown that cell motility is influenced by chemotaxis at low temperature [40, 73, 74]. Thus, although there are many complicated interactions that provide scope for future work, the basic role of chemotaxis in the distribution of biota in ice must start with a self-consistent framework, which is the focus of our work.
The confluence of thermal regelation, bio-enhanced premelting and intrinsic mobility underlie our study. Indeed, intrinsic mobility and chemotaxis may compete with thermal regelation, which constitutes a new area of research–ice bound active particles in premelting ice, as illustrated in Figure 1. Moreover, including micro-organism protection mechanisms that enhance interfacial liquidity, such as the secretion of EPS, constitute a unique class of regelation phenomena. Finally, treating this corpus of processes quantitatively is particularly relevant for climatology and the global carbon cycle [75, 76].
FIGURE 1. Schematic of few active particles embedded in ice under an external temperature gradient ∇T along the
Our framework is the active Ornstein-Uhlenbeck particle (AOUP) [77–83]. The active force is governed by an Ornstein-Uhlenbeck process with magnitude
The paper is organized as follows. In order to make our treatment reasonably self-contained we note that we are generalizing our previous approach [48, 89], which we recover in the appropriate limit. Thus, in §2 we introduce the active Ornstein-Uhlenbeck model for bio-premelted particles and in §3 we derive the associated Fokker-Planck equation using a multiple scale expansion. We then compare our analytic and numerical solutions after which, in §4, we draw conclusions.
Thermal regelation is understood as a consequence of the premelted film around a particle, originally treated as inert, that 1) executes diffusive motion in the ice column with diffusivity
For inert particles with a sufficiently large number of moles of electrolyte impurities per unit area of surface, Ni, the premelted film thickness d ∝ Ni [48, 90]. However, the production of EPS/AFP enhances liquidity at the ice surface by increasing the impurity concentration [9, 54, 72, 91], which we treat here using an enhancement factor as N = nNi, which gives
where the universal gas constant is Rg, the latent heat of fusion per mole of the solid is qm, the molar density of the liquid is ρl, the undercooling is ΔT = Tm − T with Tm = 273.15K the pure bulk melting temperature and T the temperature of ice.
The velocity and premelting-controlled diffusivity are given by
respectively, where
The first term on the right-hand side of Eq. 4 treats the chemotaxis response, representing the effect of the nutrient source of concentration
where
where τa is the noise persistence as noted above. In the small τa limit,
The Langevin Eqs 4, 5, allow us to express the probability of finding a particle at the position
Equations 8, 9 describe the space-time evolution of the probability of finding a particle and the concentration of nutrients respectively, akin to those of [77, 79, 94], but including the effects of thermal regelation discussed above. Both equations contain microscopic and macroscopic scales. The regime of interest is the long time behavior, computed by deriving the effective macroscopic dynamics as described next.
The macroscopic length characterizing the heat flux is
The particle scale l is such that l ≪ L, and hence their ratio defines a small parameter ϵ
We use the microscopic length l and a characteristic time τ, determined a posteriori, and introduce the following dimensionless variables
where
in which we have the following dimensionless numbers,
We identify four characteristic time scales:
The temperature gradient across the entire system drives thermal regelation and hence advection dominates on the macroscopic scale, so that
Now, we let
Next, we use a power series ansatz for the state variables,
to derive a system of equations at each order in ϵ [115], which for the concentration of nutrients, Eq. 19, are
shown to second order. We take the approach described in [116, 117] to solve Eqs 23–25. We integrate by parts over the microscale variables r and use the periodic boundary conditions to obtain the so-called weak formulation [118] of the leading order Eq. 23, the solution of which relies on the following product ansatz
The existence and uniqueness of
from which we find that c0 is stationary over T, leading to
showing that, as expected, the homogenization procedure is consistent with the well-known self-similar behavior of diffusion [119]. The order by order equations for the probability density function described by Eq. 18 are simplified by the observation that C0 and C1 do not depend on r, and C0 only contributes at order
where
Finally, as shown in Supplementary Material Section S1, upon substitution of P1 into Eq. 31 and using the solvability condition, we obtain the effective macroscopic dynamics as
which are the dimensional forms of Eqs 31, 28 respectively. These capture the long time behavior wherein the active force is treated through the effective diffusivity, which is enhanced by thermal regelation, consistent with our previous work [89] and that in active matter systems generally [57, 78, 85].
Equations 32, 33 can be mapped onto the well-known Keller–Segel equations for chemotaxis [93–95, 120], where ρ is the cell density and the sign of βD determines whether a cell is attracted or repelled by the nutrient. Finally, when nutrients are neglected, βD = 0, we recover our previous results [48, 89].Although Eq. 32 has an analytical solution in the large Péclet number limit, which previously allowed us to study the effect of the activity ([48, 89] or Supplementary Material Section S2), here we fix the activity and focus on the competition between thermal regelation and bio-locomotion that require solving Eqs 32, 33 numerically. We show dimensional results because of our specific interest in these processes in ice.
In the absence of nutrients, βD = 0, Figure 2 shows how the distribution of bio-particles parallel to the temperature gradient (the
FIGURE 2. Consequences of bio-enhanced premelting in the absence of nutrients (βD = 0). The evolution of the probability density along the
Figure 3 shows the evolution of the nutrient concentration along the
FIGURE 3. Evolution of the nutrient concentration (in units of M, or mol m−3) along the
In order to study the effect of nutrients on bio-locomotion, we fix the interfacial concentration of impurities and vary the chemotaxis strength βD, where nutrients either attract (βD > 0) or repel (βD < 0) the bio-particles. Because we are interested in the situation wherein the effects of chemotaxis compete with regelation, this constrains the magnitude of βD as follows. We ask for what order of magnitude of βD are the typical chemotactic speeds approximately the same as the regelation velocity in Eq. 4. Figure 3 shows the Gaussian solution of the concentration field, with a flux that becomes arbitrarily small at long times, dominated by the algebraic contribution to
For attractive chemotaxis (βD > 0), we show in Figure 4A the dependence of
FIGURE 4. Effect of nutrients on the particle dynamics. Evolution of the probability density function along the
For repulsive chemotaxis (βD < 0), we see in Figure 4B the broad sharpening of the initial distribution in the lower temperature (large
In Figure 5, we show the combined effects of EPS/AFP surface enhancement of impurities in the absence of chemotaxis (βD = 0), as shown in Figure 2, and the influence of chemotaxis on particle dynamics for fixed surface impurities, as shown in Figure 4. As we vary the surface concentration of impurities we observe the same basic features as described in Figures 2, 4 and hence the same physical description applies in their interpretation. Namely, regardless of whether chemo-attraction or chemo-repulsion is operative, if the interfacial concentration of impurities N is sufficiently large then the interfacial film thicknesses are sufficiently thick that thermal regelation dominates the evolution of
FIGURE 5. The combined effects of the surface concentration of impurities and nutrients. The probability density function along the
Micro-organisms in ice exhibit complex processes to persist and evolve in their harsh environments. They have developed different survival strategies, such as producing exopolymeric substances or antifreeze glycoproteins, and directing their motion toward nutrients or away from waste [34, 69, 125, 126]. We have modeled such micro-organisms using active Ornstein-Uhlenbeck particles subject to thermal regelation and biolocomotion in three dimensions. Firstly, we used a multi-scale expansion to derive the relevant coupled Fokker-Planck and diffusion Eqs 32, 33. Secondly, when nutrients are neglected, and the chemotactic strength βD = 0, we model the bio-production of surface chemicals, such as exopolymeric substances or antifreeze glycoproteins, as a surface colligative effect, and find that the associated bio-enhanced thermal regelation can dominate the distribution of particles in ice. Consistent with previous results [89], in a large Péclet number limit analytical solutions for the particle distributions are possible, and are consistent with the numerical solutions as shown in Figure 2. Thirdly, we studied the competition between thermal regelation and biolocomotion, as function of the chemotaxis strength βD, the interplay between which is shown in Figures 4, 5. The relative importance of chemo-attraction and chemo-repulsion to thermal regelation is captured by the dimensionless ratio
Finally, we describe settings to which our analysis is applicable. It is of general interest to understand how particles in ice migrate in response to environmental forcing, as they are used as proxy to infer past climate [14, 127, 128]. Moreover, bioparticles in ice migrate in response to environmental forcing, and micro-organisms play an important role in climate change [129–131]. For example, an increase in temperature activates algae/bacteria trapped in ice, producing chemicals that increase their mobility [131]. Indeed, an increase in algae/bacteria decreases the albedo of the ice [132–134], thereby enhancing melting. Finally, understanding the distribution and viability of bioparticles in partially frozen media on Earth [135, 136] is essential in astrobiology [41, 42, 137].
The original contribution presented in the study are included in the article/Supplementary Material, further inquiries can be directed to the authors.
JW conceived the project. JV implemented the theory and performed simulations. JW and JV interpreted the data and wrote the paper. All authors contributed to the discussions and the final version of the manuscript.
This work was supported by the Swedish Research Council grant no. 638-2013-9243. Nordita is partially supported by Nordforsk.
The authors declare that the research was conducted in the absence of any commercial or financial relationships that could be construed as a potential conflict of interest.
All claims expressed in this article are solely those of the authors and do not necessarily represent those of their affiliated organizations, or those of the publisher, the editors and the reviewers. Any product that may be evaluated in this article, or claim that may be made by its manufacturer, is not guaranteed or endorsed by the publisher.
We thank Matthias Geilhufe, Navaneeth Marath and István Mátá Szécsényi for helpful conversations.
The Supplementary Material for this article can be found online at: https://www.frontiersin.org/articles/10.3389/fphy.2022.904836/full#supplementary-material
1. Karl DM, Bird DF, Björkman K, Houlihan T, Shackelford R, Tupas L. Microorganisms in the Accreted Ice of Lake Vostok, Antarctica. Science (1999) 286:2144–7. doi:10.1126/science.286.5447.2144
2. Christner BC, Mosley-Thompson E, Thompson LG, Reeve JN. Isolation of Bacteria and 16s rDNAs from Lake Vostok Accretion Ice. Environ Microbiol (2001) 3:570–7. doi:10.1046/j.1462-2920.2001.00226.x
3. Anesio AM, Mindl B, Laybourn-Parry J, Hodson AJ, Sattler B. Viral Dynamics in Cryoconite Holes on a High Arctic Glacier (Svalbard). J Geophys Res Biogeo (2007) 112:1–10. doi:10.1029/2006jg000350
4. Bellas CM, Anesio AM, Barker G. Analysis of Virus Genomes from Glacial Environments Reveals Novel Virus Groups with Unusual Host Interactions. Front Microbiol (2015) 6:656. doi:10.3389/fmicb.2015.00656
5. Heilmann S, Sneppen K, Krishna S. Coexistence of Phage and Bacteria on the Boundary of Self-Organized Refuges. Proc Natl Acad Sci U.S.A (2012) 109:12828–33. doi:10.1073/pnas.1200771109
6. Zhong ZP, Tian F, Roux S, Gazitúa MC, Solonenko NE, Li YF, et al. Glacier Ice Archives Nearly 15,000-Year-Old Microbes and Phages. Microbiome (2021) 9:160–23. doi:10.1186/s40168-021-01106-w
7. Yau S, Lauro FM, DeMaere MZ, Brown MV, Thomas T, Raftery MJ, et al. Virophage Control of Antarctic Algal Host-Virus Dynamics. Proc Natl Acad Sci U.S.A (2011) 108:6163–8. doi:10.1073/pnas.1018221108
8. Doyle SM, Christner BC. Variation in Bacterial Composition, Diversity, and Activity Across Different Subglacial Basal Ice Types. The Cryosphere Discussions (2022) 2022:1–29. doi:10.5194/tc-2022-68
9. Anesio AM, Lutz S, Chrismas NAM, Benning LG. The Microbiome of Glaciers and Ice Sheets. NPJ Biofilms Microbiomes (2017) 3:10–1. doi:10.1038/s41522-017-0019-0
10. Royer A, De Angelis M, Petit JRA. A 30000 Year Record of Physical and Optical Properties of Microparticles from an East Antarctic Ice Core and Implications for Paleoclimate Reconstruction Models. Climatic Change (1983) 5:381–412. doi:10.1007/BF00140802
11. Legrand M, Mayewski P. Glaciochemistry of Polar Ice Cores: A Review. Rev Geophys (1997) 35:219–43. doi:10.1029/96rg03527
12. Stauffer B, Flückiger J, Wolff E, Barnes P. The EPICA Deep Ice Cores: First Results and Perspectives. Ann Glaciol (2004) 39:93–100. doi:10.3189/172756404781814500
13. Alley RB. Reliability of Ice-Core Science: Historical Insights. J Glaciol (2010) 56:1095–103. doi:10.3189/002214311796406130
14. Thomas C, Ionescu D, Ariztegui D, Team DS. Impact of Paleoclimate on the Distribution of Microbial Communities in the Subsurface Sediment of the Dead Sea. Geobiology (2015) 13:546–61. doi:10.1111/gbi.12151
15. Tetzner D, Thomas ER, Allen CS, Wolff EW. A Refined Method to Analyze Insoluble Particulate Matter in Ice Cores, and its Application to Diatom Sampling in the Antarctic Peninsula. Front Earth Sci (2021) 9:20. doi:10.3389/feart.2021.617043
16. Papina T, Blyakharchuk T, Eichler A, Malygina N, Mitrofanova E, Schwikowski M. Biological Proxies Recorded in a Belukha Ice Core, Russian Altai. Clim Past (2013) 9:2399–411. doi:10.5194/cp-9-2399-2013
17. Mao G, Ji M, Xu B, Liu Y, Jiao N. Variation of High (HNA) and Low (LNA) Nucleic Acid-Content Bacteria in Tibetan Ice Cores and Their Relationship to Black Carbon. Front Microbiol (2022) 299. doi:10.3389/fmicb.2022.844432
18. Achberger AM, Brox TI, Skidmore ML, Christner BC. Expression and Partial Characterization of an Ice Binding Protein from a Bacterium Isolated at a Depth of 3,519 Meters in the Vostok Ice Core, Antarctica. Front Microbiol (2011) 2:255. doi:10.3389/fmicb.2011.00255
19. Knowlton C, Veerapaneni R, D'Elia T, Rogers S. Microbial Analyses of Ancient Ice Core Sections from Greenland and Antarctica. Biology (2013) 2:206–32. doi:10.3390/biology2010206
20. Garcia-Lopez E, Moreno A, Bartolomé M, Leunda M, Sancho C, Cid C. Glacial Ice Age Shapes Microbiome Composition in a Receding Southern European Glacier. Front Microbiol (2021) 12:714537. doi:10.3389/fmicb.2021.714537
21. Wilhelm L, Singer GA, Fasching C, Battin TJ, Besemer K. Microbial Biodiversity in Glacier-Fed Streams. ISME J (2013) 7:1651–60. doi:10.1038/ismej.2013.44
22. Garcia-Lopez E, Moreno A, Bartolomé M, Leunda Esnaola M, Sancho C, Cid C. Glacial Ice Age Shapes Microbiome Composition in a Receding Southern European Glacier. Front Mar Sci (2021) 12. doi:10.3389/fmicb.2021.714537
23. Stibal M, Schostag M, Cameron KA, Hansen LH, Chandler DM, Wadham JL, et al. Different Bulk and Active Bacterial Communities in Cryoconite from the Margin and interior of the Greenland Ice Sheet. Environ Microbiol Rep (2015) 7:293–300. doi:10.1111/1758-2229.12246
24. Hop H, Vihtakari M, Bluhm BA, Assmy P, Poulin M, Gradinger R, et al. Changes in Sea-Ice Protist Diversity with Declining Sea Ice in the Arctic Ocean from the 1980s to 2010s. Front Mar Sci (2020) 7:243. doi:10.3389/fmars.2020.00243
25. Kauko HM, Olsen LM, Duarte P, Peeken I, Granskog MA, Johnsen G, et al. Algal Colonization of Young Arctic Sea Ice in spring. Front Mar Sci (2018) 5:199. doi:10.3389/fmars.2018.00199
26. Spilling K, Olli K, Lehtoranta J, Kremp A, Tedesco L, Tamelander T, et al. Shifting Diatom-Dinoflagellate Dominance during Spring Bloom in the Baltic Sea and its Potential Effects on Biogeochemical Cycling. Front Mar Sci (2018) 5:327. doi:10.3389/fmars.2018.00327
27. Itcus C, Pascu MD, Lavin P, Perşoiu A, Iancu L, Purcarea C. Bacterial and Archaeal Community Structures in Perennial Cave Ice. Sci Rep (2018) 8:15671–14. doi:10.1038/s41598-018-34106-2
28. Miyata M, Robinson RC, Uyeda TQP, Fukumori Y, Fukushima Si., Haruta S, et al. Tree of Motility - A Proposed History of Motility Systems in the Tree of Life. Genes Cells (2020) 25:6–21. doi:10.1111/gtc.12737
29. Hahnke RL, Meier-Kolthoff JP, García-López M, Mukherjee S, Huntemann M, Ivanova NN, et al. Genome-based Taxonomic Classification of Bacteroidetes. Front Microbiol (2016) 7:2003. doi:10.3389/fmicb.2016.02003
30. Hill NA, Häder D-P. A Biased Random Walk Model for the Trajectories of Swimming Micro-organisms. J Theor Biol (1997) 186:503–26. doi:10.1006/jtbi.1997.0421
31. Tsagkari E, Sloan W. The Role of the Motility of Methylobacterium in Bacterial Interactions in Drinking Water. Water (2018) 10:1386. doi:10.3390/w10101386
32. Doerges L, Kutschera U. Assembly and Loss of the Polar Flagellum in Plant-Associated Methylobacteria. Naturwissenschaften (2014) 101:339–46. doi:10.1007/s00114-014-1162-6
33. Svensson F, Norberg J, Snoeijs P. Diatom Cell Size, Coloniality and Motility: Trade-Offs between Temperature, Salinity and Nutrient Supply with Climate Change. PloS One (2014) 9:e109993. doi:10.1371/journal.pone.0109993
34. Aumack CF, Juhl AR, Krembs C. Diatom Vertical Migration within Land-Fast Arctic Sea Ice. J Mar Syst (2014) 139:496–504. doi:10.1016/j.jmarsys.2014.08.013
35. Christner B, Mosley-Thompson E, Thompson LG, Zagorodnov V, Sandman K, Reeve JN. Recovery and Identification of Viable Bacteria Immured in Glacial Ice. Icarus (2000) 144:479–85. doi:10.1006/icar.1999.6288
36. Biswas RK, Choudhury AK. Diatoms: Miniscule Biological Entities with Immense Importance in Synthesis of Targeted Novel Bioparticles and Biomonitoring. J Biosci (2021) 46:1–14. doi:10.1007/s12038-021-00222-x
37. Dong H, Jiang H, Yu B, Liu X, Zhang C, Chan M. Impacts of Environmental Change and Human Activity on Microbial Ecosystems on the Tibetan Plateau, NW China. GSAT (2010) 20:4–10. doi:10.1130/gsatg75a.1
38. Delgado-Baquerizo M, Bissett A, Eldridge DJ, Maestre FT, He J-Z, Wang J-T, et al. Palaeoclimate Explains a Unique Proportion of the Global Variation in Soil Bacterial Communities. Nat Ecol Evol (2017) 1:1339–47. doi:10.1038/s41559-017-0259-7
39. Benningfield D. The Bumpy Search for Liquid Water at the South Pole of Mars. EOS (2022) 103:1–6. doi:10.1029/2022EO220126
40. Lindensmith CA, Rider S, Bedrossian M, Wallace JK, Serabyn E, Showalter GM, et al. A Submersible, off-axis Holographic Microscope for Detection of Microbial Motility and Morphology in Aqueous and Icy Environments. PloS one (2016) 11:e0147700. doi:10.1371/journal.pone.0147700
41. Nadeau J, Lindensmith C, Deming JW, Fernandez VI, Stocker R. Microbial Morphology and Motility as Biosignatures for Outer Planet Missions. Astrobiology (2016) 16:755–74. doi:10.1089/ast.2015.1376
42. Jones RM, Goordial JM, Orcutt BN. Low Energy Subsurface Environments as Extraterrestrial Analogs. Front Microbiol (2018) 9:1605. doi:10.3389/fmicb.2018.01605
43. Schreder-Gomes SI, Benison KC, Bernau JA. 830-million-year-old Microorganisms in Primary Fluid Inclusions in Halite. Geology (2022). (in press). doi:10.1130/G49957.1
44. Dash JG, Rempel AW, Wettlaufer JS. The Physics of Premelted Ice and its Geophysical Consequences. Rev Mod Phys (2006) 78:695. doi:10.1103/RevModPhys.78.695
45. Rempel AW, Wettlaufer JS, Worster MG. Interfacial Premelting and the Thermomolecular Force: Thermodynamic Buoyancy. Phys Rev Lett (2001) 87:088501. doi:10.1103/PhysRevLett.87.088501
46. Wettlaufer JS, Worster MG. Premelting Dynamics. Annu Rev Fluid Mech (2006) 38:427–52. doi:10.1146/annurev.fluid.37.061903.175758
47. Peppin SSL, Spannuth MJ, Wettlaufer JS. Onsager Reciprocity in Premelting Solids. J Stat Phys (2009) 134:701–8. doi:10.1007/s10955-009-9699-z
48. Marath NK, Wettlaufer JS. Impurity Effects in thermal Regelation. Soft Matter (2020) 16:5886–91. doi:10.1039/d0sm00558d
49. Wingender J, Neu TR, Flemming H-C. What Are Bacterial Extracellular Polymeric Substances? In: Microbial Extracellular Polymeric Substances. Berlin, Heidelberg: Springer (1999). p. 1–19. doi:10.1007/978-3-642-60147-7_1
50. Bang JK, Lee JH, Murugan RN, Lee SG, Do H, Koh HY, et al. Antifreeze Peptides and Glycopeptides, and Their Derivatives: Potential Uses in Biotechnology. Mar Drugs (2013) 11:2013–41. doi:10.3390/md11062013
51. Eskandari A, Leow TC, Rahman MBA, Oslan SN. Antifreeze Proteins and Their Practical Utilization in Industry, Medicine, and Agriculture. Biomolecules (2020) 10:1649. doi:10.3390/biom10121649
52. Ewert M, Deming JW. Bacterial Responses to Fluctuations and Extremes in Temperature and Brine Salinity at the Surface of Arctic winter Sea Ice. FEMS Microbiol Ecol (2014) 89:476–89. doi:10.1111/1574-6941.12363
53. Ewert M, Deming JW. Sea Ice Microorganisms: Environmental Constraints and Extracellular Responses. Biology (Basel) (2013) 2:603–28. doi:10.3390/biology2020603
54. Krembs C, Eicken H, Deming JW. Exopolymer Alteration of Physical Properties of Sea Ice and Implications for Ice Habitability and Biogeochemistry in a Warmer Arctic. Proc Natl Acad Sci U.S.A (2011) 108:3653–8. doi:10.1073/pnas.1100701108
55. Decho AW, Gutierrez T. Microbial Extracellular Polymeric Substances (EPSs) in Ocean Systems. Front Microbiol (2017) 8:922. doi:10.3389/fmicb.2017.00922
56. Cates ME. Diffusive Transport without Detailed Balance in Motile Bacteria: Does Microbiology Need Statistical Physics? Rep Prog Phys (2012) 75:042601. doi:10.1088/0034-4885/75/4/042601
57. Bechinger C, Di Leonardo R, Löwen H, Reichhardt C, Volpe G, Volpe G. Active Particles in Complex and Crowded Environments. Rev Mod Phys (2016) 88:045006. doi:10.1103/revmodphys.88.045006
58. Ghosh A, Fischer P. Controlled Propulsion of Artificial Magnetic Nanostructured Propellers. Nano Lett (2009) 9:2243–5. doi:10.1021/nl900186w
59. Kim S, Qiu F, Kim S, Ghanbari A, Moon C, Zhang L, et al. Fabrication and Characterization of Magnetic Microrobots for Three‐Dimensional Cell Culture and Targeted Transportation. Adv Mater (2013) 25:5863–8. doi:10.1002/adma.201301484
60. Jin C, Vachier J, Bandyopadhyay S, Macharashvili T, Maass CC. Fine Balance of Chemotactic and Hydrodynamic Torques: When Microswimmers Orbit a Pillar Just once. Phys Rev E (2019) 100:040601. doi:10.1103/PhysRevE.100.040601
61. Elgeti J, Winkler RG, Gompper G. Physics of Microswimmers-Single Particle Motion and Collective Behavior: A Review. Rep Prog Phys (2015) 78:056601. doi:10.1088/0034-4885/78/5/056601
62. Romanczuk P, Bär M, Ebeling W, Lindner B, Schimansky-Geier L. Active Brownian Particles. Eur Phys J Spec Top (2012) 202:1–162. doi:10.1140/epjst/e2012-01529-y
63. Li Y-H, Tian X. Quorum sensing and Bacterial Social Interactions in Biofilms. Sensors (2012) 12:2519–38. doi:10.3390/s120302519
64. Yan S, Wu G. Can Biofilm Be Reversed through Quorum Sensing in Pseudomonas aeruginosa? Front Microbiol (2019) 10:1582. doi:10.3389/fmicb.2019.01582
65. Lee CK, Vachier J, de Anda J, Zhao K, Baker AE, Bennett RR, et al. Social Cooperativity of Bacteria during Reversible Surface Attachment in Young Biofilms: A Quantitative Comparison of Pseudomonas aeruginosa PA14 and PAO1. MBio (2020) 11:e02644–19. doi:10.1128/mBio.02644-19
66. Wadhams GH, Armitage JP. Making Sense of it All: Bacterial Chemotaxis. Nat Rev Mol Cel Biol (2004) 5:1024–37. doi:10.1038/nrm1524
67. Showalter GM, Deming JW. Low-temperature Chemotaxis, Halotaxis and Chemohalotaxis by the Psychrophilic marine Bacterium Colwellia Psychrerythraea 34h. Environ Microbiol Rep (2018) 10:92–101. doi:10.1111/1758-2229.12610
68. Cremer J, Honda T, Tang Y, Wong-Ng J, Vergassola M, Hwa T. Chemotaxis as a Navigation Strategy to Boost Range Expansion. Nature (2019) 575:658–63. doi:10.1038/s41586-019-1733-y
69. Bar Dolev M, Bernheim R, Guo S, Davies PL, Braslavsky I. Putting Life on Ice: Bacteria that Bind to Frozen Water. J R Soc Interf (2016) 13:20160210. doi:10.1098/rsif.2016.0210
70. Mattingly H, Kamino K, Machta B, Emonet T. Escherichia coli Chemotaxis is Information Limited. Nat Phys (2021) 17:1–6. doi:10.1038/s41567-021-01380-3
71. Bar-Dolev M, Celik Y, Wettlaufer JS, Davies PL, Braslavsky I. New Insights into Ice Growth and Melting Modifications by Antifreeze Proteins. J R Soc Interf (2012) 9:3249–59. doi:10.1098/rsif.2012.0388
72. Hansen-Goos H, Thomson ES, Wettlaufer JS. On the Edge of Habitability and the Extremes of Liquidity. Planet Space Sci (2014) 98:169–81. doi:10.1016/j.pss.2013.04.010
73. Junge K, Eicken H, Deming JW. Motility of Colwellia Psychrerythraea Strain 34H at Subzero Temperatures. Appl Environ Microbiol (2003) 69:4282–4. doi:10.1128/aem.69.7.4282-4284.2003
74. Mudge MC, Nunn BL, Firth E, Ewert M, Hales K, Fondrie WE, et al. Subzero, saline Incubations of Colwellia Psychrerythraea Reveal Strategies and Biomarkers for Sustained Life in Extreme Icy Environments. Environ Microbiol (2021) 23:3840–66. doi:10.1111/1462-2920.15485
75. Wadham JL, Hawkings JR, Tarasov L, Gregoire LJ, Spencer RGM, Gutjahr M, et al. Ice Sheets Matter for the Global Carbon Cycle. Nat Commun (2019) 10:3567–17. doi:10.1038/s41467-019-11394-4
76. Holland AT, Williamson CJ, Sgouridis F, Tedstone AJ, McCutcheon J, Cook JM, et al. Dissolved Organic Nutrients Dominate Melting Surface Ice of the Dark Zone (Greenland Ice Sheet). Biogeosciences (2019) 16:3283–96. doi:10.5194/bg-16-3283-2019
77. Fodor É, Nardini C, Cates ME, Tailleur J, Visco P, van Wijland F. How Far from Equilibrium is Active Matter? Phys Rev Lett (2016) 117:038103. doi:10.1103/PhysRevLett.117.038103
78. Caprini L, Marini Bettolo Marconi U. Active Particles under Confinement and Effective Force Generation Among Surfaces. Soft Matter (2018) 14:9044–54. doi:10.1039/c8sm01840e
79. Martin D, O'Byrne J, Cates ME, Fodor É, Nardini C, Tailleur J, et al. Statistical Mechanics of Active Ornstein-Uhlenbeck Particles. Phys Rev E (2021) 103:032607. doi:10.1103/PhysRevE.103.032607
80. Dabelow L, Eichhorn R. Irreversibility in Active Matter: General Framework for Active Ornstein-Uhlenbeck Particles. Front Phys (2021) 8:516. doi:10.3389/fphy.2020.582992
81. Caprini L, Marini Bettolo Marconi U, Puglisi A. Activity Induced Delocalization and Freezing in Self-Propelled Systems. Sci Rep (2019) 9:1386–9. doi:10.1038/s41598-018-36824-z
82. Bonilla LL. Active Ornstein-Uhlenbeck Particles. Phys Rev E (2019) 100:022601. doi:10.1103/PhysRevE.100.022601
83. Dabelow L, Bo S, Eichhorn R. Irreversibility in Active Matter Systems: Fluctuation Theorem and Mutual Information. Phys Rev X (2019) 9:021009. doi:10.1103/physrevx.9.021009
84. Sevilla FJ, Rodríguez RF, Gomez-Solano JR. Generalized Ornstein-Uhlenbeck Model for Active Motion. Phys Rev E (2019) 100:032123. doi:10.1103/PhysRevE.100.032123
85. Maggi C, Paoluzzi M, Pellicciotta N, Lepore A, Angelani L, Di Leonardo R. Generalized Energy Equipartition in Harmonic Oscillators Driven by Active Baths. Phys Rev Lett (2014) 113:238303. doi:10.1103/physrevlett.113.238303
86. Maggi C, Paoluzzi M, Angelani L, Di Leonardo R. Memory-less Response and Violation of the Fluctuation-Dissipation Theorem in Colloids Suspended in an Active bath. Sci Rep (2017) 7:17588–7. doi:10.1038/s41598-017-17900-2
87. Donado F, Moctezuma RE, López-Flores L, Medina-Noyola M, Arauz-Lara JL. Brownian Motion in Non-equilibrium Systems and the Ornstein-Uhlenbeck Stochastic Process. Sci Rep (2017) 7:12614–7. doi:10.1038/s41598-017-12737-1
88. Marini Bettolo Marconi U, Maggi C, Melchionna S. Pressure and Surface Tension of an Active Simple Liquid: A Comparison between Kinetic, Mechanical and Free-Energy Based Approaches. Soft Matter (2016) 12:5727–38. doi:10.1039/c6sm00667a
89. Vachier J, Wettlaufer JS. Premelting Controlled Active Matter in Ice. Phys Rev E (2022) 105:024601. doi:10.1103/PhysRevE.105.024601
90. Wettlaufer JS. Impurity Effects in the Premelting of Ice. Phys Rev Lett (1999) 82:2516–9. doi:10.1103/physrevlett.82.2516
91. Ewert M, Deming J. Sea Ice Microorganisms: Environmental Constraints and Extracellular Responses. Biology (2013) 2:603–28. doi:10.3390/biology2020603
92. Keller EF, Segel LA. Model for Chemotaxis. J Theor Biol (1971) 30:225–34. doi:10.1016/0022-5193(71)90050-6
93. Saha S, Golestanian R, Ramaswamy S. Clusters, Asters, and Collective Oscillations in Chemotactic Colloids. Phys Rev E Stat Nonlin Soft Matter Phys (2014) 89:062316. doi:10.1103/PhysRevE.89.062316
94. Liebchen B, Löwen H. Synthetic Chemotaxis and Collective Behavior in Active Matter. Acc Chem Res (2018) 51:2982–90. doi:10.1021/acs.accounts.8b00215
95. Pohl O, Stark H. Dynamic Clustering and Chemotactic Collapse of Self-Phoretic Active Particles. Phys Rev Lett (2014) 112:238303. doi:10.1103/physrevlett.112.238303
96. Wettlaufer JS, Worster MG, Huppert HE. Natural Convection during Solidification of an alloy from above with Application to the Evolution of Sea Ice. J Fluid Mech (1997) 344:291–316. doi:10.1017/s0022112097006022
97. Rempel AW, Waddington ED, Wettlaufer JS, Worster MG. Possible Displacement of the Climate Signal in Ancient Ice by Premelting and Anomalous Diffusion. Nature (2001) 411:568–71. doi:10.1038/35079043
98. Rempel AW, Wettlaufer JS, Waddington ED. Anomalous Diffusion of Multiple Impurity Species: Predicted Implications for the Ice Core Climate Records. J Geophys Res Solid Earth (2002) 107:ECV 3–1–ECV 3–12. doi:10.1029/2002jb001857
99. Wells AJ, Wettlaufer JS, Orszag SA. Maximal Potential Energy Transport: A Variational Principle for Solidification Problems. Phys Rev Lett (2010) 105:254502. doi:10.1103/physrevlett.105.254502
100. Joanny J-F, Jülicher F, Prost J. Motion of an Adhesive Gel in a Swelling Gradient: A Mechanism for Cell Locomotion. Phys Rev Lett (2003) 90:168102. doi:10.1103/physrevlett.90.168102
101. Peruani F, Morelli LG. Self-propelled Particles with Fluctuating Speed and Direction of Motion in Two Dimensions. Phys Rev Lett (2007) 99:010602. doi:10.1103/PhysRevLett.99.010602
102. Romanczuk P, Schimansky-Geier L. Brownian Motion with Active Fluctuations. Phys Rev Lett (2011) 106:230601. doi:10.1103/physrevlett.106.230601
103. Vandebroek H, Vanderzande C. The Effect of Active Fluctuations on the Dynamics of Particles, Motors and DNA-Hairpins. Soft Matter (2017) 13:2181–91. doi:10.1039/c6sm02568d
104. Price PB. A Habitat for Psychrophiles in Deep Antarctic Ice. Proc Natl Acad Sci U.S.A (2000) 97:1247–51. doi:10.1073/pnas.97.3.1247
105. Campen RK, Sowers T, Alley RB. Evidence of Microbial Consortia Metabolizing within a Low-Latitude Mountain Glacier. Geol (2003) 31:231–4. doi:10.1130/0091-7613(2003)031<0231:eomcmw>2.0.co;2
106. Tung HC, Price PB, Bramall NE, Vrdoljak G. Microorganisms Metabolizing on clay Grains in 3-Km-Deep Greenland Basal Ice. Astrobiology (2006) 6:69–86. doi:10.1089/ast.2006.6.69
107. Mader HM, Pettitt ME, Wadham JL, Wolff EW, Parkes RJ. Subsurface Ice as a Microbial Habitat. Geol (2006) 34:169–72. doi:10.1130/g22096.1
108. Rohde RA, Price PB. Diffusion-controlled Metabolism for Long-Term Survival of Single Isolated Microorganisms Trapped within Ice Crystals. Proc Natl Acad Sci U.S.A (2007) 104:16592–7. doi:10.1073/pnas.0708183104
109. Vancoppenolle M, Goosse H, De Montety A, Fichefet T, Tremblay B, Tison JL. Modeling Brine and Nutrient Dynamics in Antarctic Sea Ice: The Case of Dissolved Silica. J Geophys Res Oceans (2010) 115:1–18. doi:10.1029/2009jc005369
110. Wu M, Roberts JW, Kim S, Koch DL, DeLisa MP. Collective Bacterial Dynamics Revealed Using a Three-Dimensional Population-Scale Defocused Particle Tracking Technique. Appl Environ Microbiol (2006) 72:4987–94. doi:10.1128/aem.00158-06
111. Ben Amar M. Collective Chemotaxis and Segregation of Active Bacterial Colonies. Sci Rep (2016) 6:21269–9. doi:10.1038/srep21269
112. Wåhlin J, Klein-Paste A. The Effect of Mass Diffusion on the Rate of Chemical Ice Melting Using Aqueous Solutions. Cold Regions Sci Techn (2017) 139:11–21. doi:10.1016/j.coldregions.2017.04.001
113. Dabelow L, Bo S, Eichhorn R. How Irreversible Are Steady-State Trajectories of a Trapped Active Particle? J Stat Mech Theor E. (2021) 2021:033216. doi:10.1088/1742-5468/abe6fd
114. Caprini L, Marconi UMB, Wittmann R, Löwen H. Dynamics of Active Particles with Space-dependent Swim Velocity. Soft Matter (2022) 18:1412–22. doi:10.1039/d1sm01648b
115. Bender CM, Orszag SA. Advanced Mathematical Methods for Scientists and Engineers: Asymptotic Methods and Perturbation Theory. New York, NY: Springer (2013).
116. Pavliotis G, Stuart A. Multiscale Methods: Averaging and Homogenization. New York, NY: Springer (2008).
117. Aurell E, Bo S, Dias M, Eichhorn R, Marino R. Diffusion of a Brownian Ellipsoid in a Force Field. EPL (2016) 114:30005. doi:10.1209/0295-5075/114/30005
118. Chipot M. Weak Formulation of Elliptic Problems. In: Elliptic Equations: An Introductory Course. Basel: Birkhäuser, Basel (2009). p. 35–42. doi:10.1007/978-3-7643-9982-5_3
119. Barenblatt GI. Scaling, Self-Similarity, and Intermediate Asymptotics. Cambridge, UK: Cambridge University Press (1996).
120. Liebchen B, Löwen H. Modeling Chemotaxis of Microswimmers: From Individual to Collective Behavior. In: Chemical Kinetics: Beyond the Textbook. New Jersey: World Scientific (2020). p. 493–516.
121. Theurkauff I, Cottin-Bizonne C, Palacci J, Ybert C, Bocquet L. Dynamic Clustering in Active Colloidal Suspensions with Chemical Signaling. Phys Rev Lett (2012) 108:268303. doi:10.1103/physrevlett.108.268303
122. Jin C, Krüger C, Maass CC. Chemotaxis and Autochemotaxis of Self-Propelling Droplet Swimmers. Proc Natl Acad Sci U.S.A (2017) 114:5089–94. doi:10.1073/pnas.1619783114
123. Salek MM, Carrara F, Fernandez V, Guasto JS, Stocker R. Bacterial Chemotaxis in a Microfluidic T-Maze Reveals strong Phenotypic Heterogeneity in Chemotactic Sensitivity. Nat Commun (2019) 10:1877–11. doi:10.1038/s41467-019-09521-2
124. Hokmabad BV, Agudo-Canalejo J, Saha S, Golestanian R, Maass CC. Chemotactic Self-Caging in Active Emulsions (2022) 119 (24):e2122269119. doi:10.1073/pnas.2122269119
125. Price PB. Microbial Life in Glacial Ice and Implications for a Cold Origin of Life. FEMS Microbiol Ecol (2007) 59:217–31. doi:10.1111/j.1574-6941.2006.00234.x
126. Stocker R, Seymour JR. Ecology and Physics of Bacterial Chemotaxis in the Ocean. Microbiol Mol Biol Rev (2012) 76:792–812. doi:10.1128/mmbr.00029-12
127. Miteva V. Bacteria in Snow and Glacier Ice. In: Psychrophiles: From Biodiversity to Biotechnology. Berlin, Heidelberg: Springer (2008). p. 31–50. doi:10.1007/978-3-540-74335-4_3
128. Han D, Nam SI, Kim JH, Stein R, Niessen F, Joe YJ, et al. Inference on Paleoclimate Change Using Microbial Habitat Preference in Arctic Holocene Sediments. Sci Rep (2017) 7:9652–11. doi:10.1038/s41598-017-08757-6
129. Mitchell JG, Kogure K. Bacterial Motility: Links to the Environment and a Driving Force for Microbial Physics. FEMS Microbiol Ecol (2006) 55:3–16. doi:10.1111/j.1574-6941.2005.00003.x
130. Dutta H, Dutta A. The Microbial Aspect of Climate Change. Energ Ecol Environ (2016) 1:209–32. doi:10.1007/s40974-016-0034-7
131. Cavicchioli R, Ripple WJ, Timmis KN, Azam F, Bakken LR, Baylis M, et al. Scientists' Warning to Humanity: Microorganisms and Climate Change. Nat Rev Microbiol (2019) 17:569–86. doi:10.1038/s41579-019-0222-5
132. Ryan JC, Hubbard A, Stibal M, Irvine-Fynn TD, Cook J, Smith LC, et al. Dark Zone of the Greenland Ice Sheet Controlled by Distributed Biologically-Active Impurities. Nat Commun (2018) 9:1065–10. doi:10.1038/s41467-018-03353-2
133. Perini L, Gostinčar C, Anesio AM, Williamson C, Tranter M, Gunde-Cimerman N. Darkening of the Greenland Ice Sheet: Fungal Abundance and Diversity Are Associated with Algal Bloom. Front Microbiol (2019) 10:557. doi:10.3389/fmicb.2019.00557
134. Williamson CJ, Cook J, Tedstone A, Yallop M, McCutcheon J, Poniecka E, et al. Algal Photophysiology Drives Darkening and Melt of the Greenland Ice Sheet. Proc Natl Acad Sci U.S.A (2020) 117:5694–705. doi:10.1073/pnas.1918412117
135. Van Leeuwe MA, Tedesco L, Arrigo KR, Assmy P, Campbell K, Meiners KM, et al. Microalgal Community Structure and Primary Production in Arctic and Antarctic Sea Ice: A Synthesis. Element Sci Anthropocene (2018) 6:1–25. doi:10.1525/elementa.267
136. Cimoli E, Lucieer V, Meiners KM, Chennu A, Castrisios K, Ryan KG, et al. Mapping the In Situ Microspatial Distribution of Ice Algal Biomass through Hyperspectral Imaging of Sea-Ice Cores. Sci Rep (2020) 10:21848–17. doi:10.1038/s41598-020-79084-6
137. Wettlaufer JS. Sea Ice and Astrobiology. In: D Thomas, and G Dieckmann, editors. Sea Ice. 2nd ed. Oxford: Wiley-Blackwell (2010). p. 579–94. chap. 15.
138. Risken H. Fokker-Planck Equation. In: The Fokker-Planck Equation. Berlin, Heidelberg: Springer (1996). p. 63–95. doi:10.1007/978-3-642-61544-3_4
139. Celani A, Vergassola M. Bacterial Strategies for Chemotaxis Response. Proc Natl Acad Sci U.S.A (2010) 107:1391–6. doi:10.1073/pnas.0909673107
Keywords: bioparticles, premelting, biolocomotion, active matter, Ornstein-Uhlenbeck process, extremophiles
Citation: Vachier J and Wettlaufer JS (2022) Biolocomotion and Premelting in Ice. Front. Phys. 10:904836. doi: 10.3389/fphy.2022.904836
Received: 25 March 2022; Accepted: 03 June 2022;
Published: 01 July 2022.
Edited by:
Sujit Datta, Princeton University, United StatesReviewed by:
Jay Nadeau, Portland State University, United StatesCopyright © 2022 Vachier and Wettlaufer . This is an open-access article distributed under the terms of the Creative Commons Attribution License (CC BY). The use, distribution or reproduction in other forums is permitted, provided the original author(s) and the copyright owner(s) are credited and that the original publication in this journal is cited, in accordance with accepted academic practice. No use, distribution or reproduction is permitted which does not comply with these terms.
*Correspondence: Jérémy Vachier , amVyZW15LnZhY2hpZXJAc3Uuc2U=, John S. Wettlaufer , am9obi53ZXR0bGF1ZmVyQHlhbGUuZWR1
Disclaimer: All claims expressed in this article are solely those of the authors and do not necessarily represent those of their affiliated organizations, or those of the publisher, the editors and the reviewers. Any product that may be evaluated in this article or claim that may be made by its manufacturer is not guaranteed or endorsed by the publisher.
Research integrity at Frontiers
Learn more about the work of our research integrity team to safeguard the quality of each article we publish.