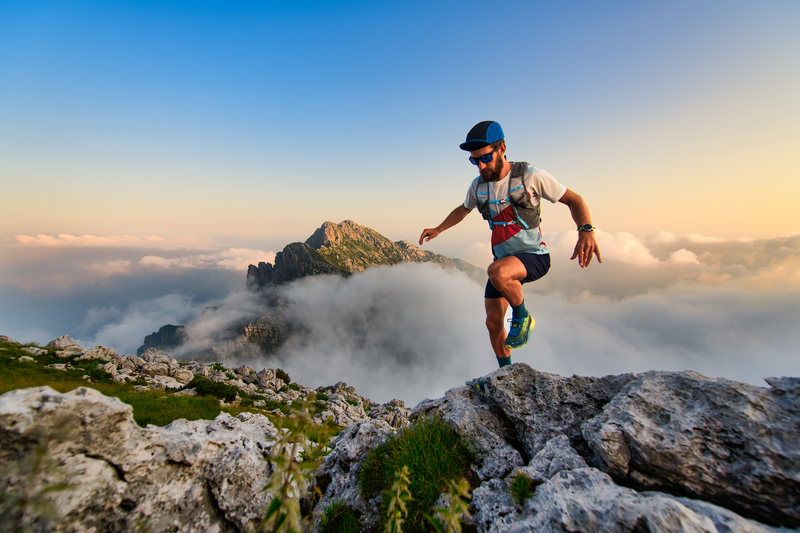
95% of researchers rate our articles as excellent or good
Learn more about the work of our research integrity team to safeguard the quality of each article we publish.
Find out more
ORIGINAL RESEARCH article
Front. Phys. , 22 June 2022
Sec. Social Physics
Volume 10 - 2022 | https://doi.org/10.3389/fphy.2022.902890
This article is part of the Research Topic Human Behavior and Dynamics in Social Systems View all 9 articles
In show business, awards are conferred to persons and films to provide incentives to performers’ future career development through periodic film festivals and events. In this work, we focused on exploring the growth and dynamics of the film award system, the structure of the award network, and the relationships between historical performance, collaborations, and future career success of performers in the movie industry. We collected data from IMDb, which covers more than 3.5K movie events for 520K individuals with their award-winning and career records for over 90 years. By using network analysis and regression models, we find several novel results. At first, we found the exponential proliferation of awards across all genres of films and all professions of individuals and the uneven distribution of the number of awards in careers across time. More than 30% of the performers have won multiple awards. Second, we built an award network to reveal the interlocks between awards based on multiple award-winning phenomena. We found that for prestigious awards, 47% of the linkages were over-representative than the expectations from the null model. Furthermore, the performers’ collaboration network was highly clustered, exhibiting a high propensity of linkages between awarded performers. Lastly, our regression models revealed that multiple factors were related to performers’ early career success and award winning. Specifically, we showed that along with the performers’ historical achievements, their collaborators serve an important role in award winning after being nominated, with the scope and depth of the impact differing in the awards’ prestige. This work has strong implications for the harmonious dynamics of the movie industry and the career development of performers.
Awards are conferred to recognize individuals’ merits of performance across various domains. In science, award winning is a complementary scientific measure of excellence, and awards are always associated with funding, financial incentives, promotion, and prestige. For instance, awarded scientists will attract collaborators, become nominators, and enhance the development of their research fields [1–6]. A typical example in show business is the Oscar award; winners in this event always attract tremendous attention all over the world and will get much more benefits in their future careers [7,8]. There are a large number of works trying to quantitatively understand the system dynamics and predict the behaviors of performers in the movie industry, for example, previous studies include exploring the dynamics of the movie industry [7,9,10], measuring the significance of creative work [11], predicting the movie ratings and success [12,13], the collaboration network analysis between performers [14,15], the gender disparities and age [16–20], and quantifying and predicting the career development for performers [15,21,22]. These sufficient works on the movie industry gave us a comprehensive picture of the film industry, however, there is still a lack of study focusing on film awards, especially putting all the famous film awards together. In science, established studies found that awards play a positive role in inspiring new achievements [2,4,5,23], allocating scientific contribution [24], and signaling scientific credibility [25] and field advancement [6]. Based on these results in science, we are trying to collect a large dataset which will cover all the famous awards in the movie industry, explore the dynamics of the award system and quantify the performers’ career success for future award winning.
Career development has received various attention, and lots of interesting insights are deserved to mention here. For instance, studies have shown that factors including talent/creativity [26,27], personal characteristics including gender and age [16,17,19,28], social networks like collaborations [29], cumulative advantages [30,31], and social media [32], all will affect the productivity and quality of individuals’ future performance. Specifically, studies have shown that creativity in a career is random regardless of career stages, while there exists hot streaks phenomenon in career development [21,22,33–35]. In addition, awards play an important role in a performer’s career development. Borjas, et. al. found that mathematicians who win Fields medal will have a decline in productivity after the prizewinning year, which indicates the potential expense of exploring new research areas after prizewinning [23], and Reschke, et. al. found that the winner’s “neighbors” may lose attention after the winner’s prizewinning time [36]. Thus, by an analogy between career development in science and show business, the future award winning of performers may be determined by multiple factors, including their self-attributes, the collaboration network, and their performance.
In this work, we are trying to systematically study the dynamics of the award system in the movie industry and figure out what leads to the early career success of award-winning actors and actresses. For policymakers in the movie industry, the work will be useful to help maintain a harmonious award system, and for actors and actresses, it provides sufficient insight for career management. We collected and integrated large-scale data, including movies, actors and actresses, and award events. We focused on film awards that are conferred to individuals for their excellence in filming, with interactions with other individuals in filming and directing. First, we explored the dynamics of the award system and the increase of prizewinning films by genres and actors by profession. Second, we derived the statistical properties of the award network and the collaboration network to further understand the award-winning propensity. Third, we modeled on what led to early career success when actors and actresses were first nominated for an award. We paid attention to the question of how the historical performance of actors and actresses’ filming careers and the quantity and impact of their collaborators would lead to winning at the early stage.
The data we used from IMDb contains over a hundred years of movie records, including films, television cast, and production. For films and actors, it includes the names, principals, artwork information, and movie ratings. We collected extra data from IMDb and Wikipedia (for instance, the wiki Category: Lists of awards received by an actor) which contains the award records for performers. Across wiki and IMDb, the actors/actresses are linked by their IMDb ids starting with “nm,” and the movies are linked by their IMDb ids starting with “tt.” The events include almost all the world’s biggest awards, including the Academy Award, the Golden Globe Award, and the Prime Emmy. We focused on film awards that are conferred to individuals for their excellence in filming, the interactions with other individuals in the movie industry.
Here we developed the h-index for awards by analogy to the h-index in science. We used this because we found that the h-index ranking is closer to reality and easy to calculate. At first, h-index was first proposed by Hirsch to quantify an individual’s research performance [37]. By definition, a scientist’s h-index equals
FIGURE 1. Correlation between page rank and h-index for measuring award prestige. The colors code the groups of awards for prestigious (h-index>100), medium, and unknown (h-index<5). The red line shows their linear relationship, and the Pearson correlation is 0.73.
In the movie industry, there are events/festivals conducted regularly, for example, the Oscar is held each year. And during each event year, there are several awards like the best actor/actress, the best directors, the best writers for individuals, and the best action, best documentary, for the best film work. For each award, it may have more than one nominee in each event, and usually only one will be the winner. We found that among the nomination records of all professions, actors and actresses play leading roles in prizewinning, as shown in Figure 2. The actor and actress nominees have taken up more than 20% of the total nomination records. Further, we found that more than half of the nominees play multiple roles in the movie industry, for example, 7% of the individual awards (8207 awards) are taken up by performers with a composition profession of director, writer, and producer.
FIGURE 2. Composition of primary professions for award winners. Linked dots represent the composition of professions for each kind, and the bar on top represents the number of winning records for each kind. Actresses and actors receive the largest number of awards (22%), with the top 10 compositions accounting for 58% of all individual awards. For example, 7% of the individual awards (8207 awards) are taken up by performers with a composition profession of director, writer, and producer.
The award system has experienced an exponential expansion during the last 3 decades. At first, as the grey bars shows in Figure 3A, for all the prizewinning events, the number of awardees increases exponentially with time, in the 1950s, there were less than 200 awardees each year, however in the 2000s, the awardees reached 3000, this increase is probably due to the increase of the number of unique awards as the film events increase as shown in the blue line in Figure 3A. We can further see that the growth speed is changing with time, and roughly has three periods, i.e., the flat with gradual increase period from 1950 to 1994, the medium-growing period from 1994 to 2009, and the fast-growing period from 2010 to 2019.
FIGURE 3. The number of award winners by year (A) The number of all award winners increases (grey bar) when the number of awards increases (dotted line) on an exponential scale (B) For awards conferred to movies, i.e., for film awardees, different genres have exponential increases with different exponents. Grey bars represent the number of all awarded films by year, and dotted lines indicate the number of annually awarded films by different genres (C) For awards conferred to individuals, all professions have a similar exponent of increase. Grey bars represent the number of individual award winners by year, and dotted lines indicate the number of annual award winners for different professions.
Secondly, during the expansion, the number of awardees in each genre grew exponentially at different rates. As shown in Figure 3B, for awards conferred to movies, i.e., the awardees are films, the films of different genres grow with the exponential trend, with genres of drama having won the largest number of awards, short drama genres having the most abrupt upward trend, which reflects the fashion trend in the last decade. Lastly, when identifying the primary professions of individual award winners, actors receive the largest number of awards, but all professions have a similar rate of increase in the exponent as in Figure 3C.
With the increase of winners in the system, the performers may win multiple awards in their careers, as in the cases studied in science [5]. Thus, in Figure 4, we showed the distribution of the total number of awards a performer won during her/his career. We found that about 70% of the winners only get one award during their careers. We further split our data into three periods, the three periods are set according to the three growth periods in Figure 2A and showed the distribution in Figure 4. We can conclude that the multiple prizewinning cases are increasing slightly (33% win more than one prize in 2010–2019, this percentage is 31% was 1994–2009 and 30% in 1950–1994). Furthermore, we found that during their careers, actors often get more awards than actresses (Inset of Figure 3).
FIGURE 4. Prize number distribution. Plots show the number of prizes per prize-winner for different periods. The three periods are separated by the trend of growth (flat, medium, and fast-growing periods as shown in Figure 2A). We can see that performers are more likely to win a single prize (70%), while multiple prizewinning is slightly frequent in the recent decade. The Inset shows that the number of prizes won by the gender of performers differs a lot.
Due to the 30% of multiple prizewinning in the three different periods, it prompts us to ask how other factors will influence the prizewinning of a performer, for instance, the potential correlation between awards and collaborations between performers. Thus, we constructed two networks, the award network and the performers’ collaboration network. The definitions and analysis of the networks are as follows.
The whole award network in total contains 3634 awards and the weighted links between awards. In the award network, awards are nodes, and weighted links between two awards
The award network shows that there are potentially strong pipelines between different awards. Due to the densely connected property of the award network, we filtered a subgraph by using the award h-index (see data description part for details). In Figure 5, for visualization convenience, we filtered awards having an h-index greater than 250 and showed the relative link weights by the stripe widths. By comparison, 47% of the links have stronger links than expectations (over-representative). For example, the link weight between the César Award and the Silver Ribbon is seven times larger than expected, both are famous events in Europe.
FIGURE 5. Prestigious awards. The top prestigious network selected by h-index. The stripe width between each plot is weighted by the degree of each prize in the co-awarding network compared with the null model (random graph). Compared with random graphs, some awards show very strong connections, for example, the link weight between the César Award and the Silver Ribbon is 7.
The collaboration network contains 52,531 performers (nominees) as nodes and the collaboration relationship in casts as links from 1950 to 2021. Due to the high density of the collaboration network between performers, we extracted the backbone of the network [39] and focused on the top prestigious prizes, including Oscar, BAFTA, Primetime Emmy, Golden Globes, and Saturn Award according to the award h-index. We found that the prize-winners tend to be hubs in the network and have on an average more connections compared to the ones only being nominated but not winning eventually (K-S test statistic 0.031, p-value of 0.024). Furthermore, we found that the chance of winning a prize is getting more difficult in the recent decade, in Figure 6 for the Saturn Award, the proportion of winners among all nominees decreased from 22% in the 1990s to 18% in the 2010s. The connections between winners and nominees indicate that prizewinning actors may affect a performer’s prizewinning propensity after nomination.
FIGURE 6. Collaboration network for the Saturn Award. Three collaboration networks from the years 1990–1999, 2000–2009, and 2010–2019 are shown. The number of nominated actors and actresses increases by year, while the chances of winning the prize are getting more difficult in recent years (22%, 23%, and 18%). Green dots represent prize-winners, and orange dots represent nominees without prizewinning.
To further study the role of award and collaboration in a performer’s early career award winning, we developed regression models. In the model, we incorporated potential factors of interest from our data, for example, the acting performance of the individual, the collaboration network, the award h-index (prestige), and the number of competitive nominees prior to prizewinning would all influence the chance of prizewinning. Historical performance of actors/actresses is related to the career length, productivity, quality of work, and influence of works. We also controlled the award types and the periods of the awards conferred.
We used a logistic regression model to predict the probability that a performer wins at the first nomination. The independent variables we used are: 1) number of films the performers participated in before their nomination; 2) average movie ratings of historical works; 3) average number of votes for historical works; 4) length of career prior to nomination; 5) gender; 6) number of awarded films prior to nomination; 7) number of collaborated directors; 8) number of awarded collaborated directors; 9) number of collaborated actors; 10) number of awarded collaborated actors; 11) competitive pool size (number of potential awards which will be conferred in the nomination year); 12) dummy variable for award types; and 13) dummy variable for time periods.
The logistic regression form is as follows:
We performed multiple models for regressions, in Table 1 models M1, M3, and M4 indicate that the performer’s career length and number of awarded films prior to nomination have a significant positive impact on early career prizewinning. All of models M2, M3, and M4 indicate that by collaborating with more prizewinning actors before nomination, the performer is more likely to win. In model M4, the number of a performer’s collaborated directors has a negative impact, indicating when a performer collaborates with a smaller number of directors, he/she has a higher probability of winning. This highlights the importance of long-term and high-quality collaboration with directors.
TABLE 1. Logistic regression models. M1: considers the historical performance of performers with control variables. M2: considers the collaborators’ influence with control variables. M3: considers both the collaborators’ influence and historical performance without control variables. M4: considers both historical performances and collaborators’ influence with control variables. The odds ratios are shown in the table and the significant coefficients are shown in bold.
Due to the small sample of winners compared to non-winning nominees, we further used a complementary log-log model, which is usually used in an asymmetrical case. The result is very similar, with a detected significance on the historical performance and collaborators as shown in Supplementary Table S1 in the supplemental information.. We further controlled the award’s prestige by h-index, and the consistent results are shown in model M5 in Supplementary Table S1, which confirms the robustness of our study.
From the models, we showed that the historical performance plays a positive role in a performer’s future prizewinning. In detail, we showed the predictive impact in Figure 7. Based on model M4, the predictive probability of winning after nomination for performers with career lengths above 8 years is more than 35%, while it drops to 30% for performers with career lengths less than 2 years. The longer the career length is, the more likely the nominees will win the awards. When a performer participated in a larger number of awarded films before being nominated, he/she has a larger probability of prizewinning, Figure 7B shows this trend.
FIGURE 7. Predictive marginal effects for historical performance. (A) Predictive marginal probability with increasing log career length, the predictive probability of winning after being nominated for performers with a career length above 8 years is more than 35%, while it drops to 30% for performers with career lengths less than 2 years. (B) Predictive marginal probability with the increasing number of awarded films participated in. The logarithmic bases are the natural logarithms in the plots.
For the collaborators of a performer, we found that the awarded actor collaborators had a positive influence on the performer’s prizewinning, yet the director collaborators showed negative effects, as shown in Figure 8. This reflects the fact that in the movie industry, collaborating with more prizewinning actors, the performer will benefit from the actors and gain experience in acting, and will have a positive impact on her/his career, as shown in Figure 8A. However, collaborating with more directors, which means the performer has lots of short collaborations, will be harmful to future prizewinning, as shown in Figure 8B.
FIGURE 8. Predictive marginal effects for collaboration network. (A) Predictive marginal probability with increasing number of awarded actors. The number of awarded actors among collaborators will increase the probability of prizewinning. (B) Predictive marginal probability with the increasing number of directors has a negative impact on prizewinning. The logarithmic bases are the natural logarithms in the plots.
In this work, we explored the dynamics of the award system and the award winning in performers’ careers in the movie industry, which has not been well studied before. We found that, as expected, the award system experienced exponential growth during the last 60 years and showed different levels of stratifications in genres and professions. The network science tools are used in the analysis of nominees and awards. By comparing with the null model, the award network illustrates the existence of potential connections between different awards, which will affect multiple prizewinning in a performer’s career. Our regression models showed multiple factors that will affect a performer’s award winning, including the career length, the number of awarded films participated in, and the former collaborators, etc.
Our work provides strong implications for all actors and actresses in the movie industry, especially in their early careers. Based on our regression models, we found that the factors affecting a performer’s first award winning after being nominated are multifaceted. At first, the career length and the number of awarded films participated play positive roles, which probably indicates that in the movie industry, “accumulated experience in filming,” i.e., participating in excellent (awarded) films and keeping the career active (career length), is the key to future performance recognition (award winning) for a performer. Moreover, it is worth noting that collaboration with more famous (prizewinning) actors, sticking to few directors, and building a solid relationship will help the performer gain award-winning probability in the future. Our results also imply the success breeds success phenomenon in the movie industry. If a performer collaborates with more awarded actors and participates in more awarded films, her/his award-winning probability will be improved considerably. This indicates that the success (of awarded films and awarded actors) will influence and ‘breed’ the success of the performer (future award-winning actors), the detailed mechanism behind this is an open question.
Despite the rigorous analysis and modeling in this work, there is still future work in potential directions: On one hand, the potential causal pipelines between awards, i.e., from the award network, there are strong correlations between different awards. However, the underlying mechanism of how wining an award
The original contributions presented in the study are included in the article/Supplementary Material; further inquiries can be directed to the corresponding author.
YL analyzed data, designed the research, and wrote the manuscript, and YM collected data, designed the research, and wrote the manuscript.
This work was supported by the NSFC grant, No. NSFC12031005, and partly sponsored by SUSTech Research Series at the Southern University of Science and Technology, No. SUSTECH2020C007.
The authors declare that the research was conducted in the absence of any commercial or financial relationships that could be construed as a potential conflict of interest.
All claims expressed in this article are solely those of the authors and do not necessarily represent those of their affiliated organizations, or those of the publisher, the editors, and the reviewers. Any product that may be evaluated in this article, or claim that may be made by its manufacturer, is not guaranteed or endorsed by the publisher.
The authors thank IMDb and Wikipedia for the data.
The Supplementary Material for this article can be found online at: https://www.frontiersin.org/articles/10.3389/fphy.2022.902890/full#supplementary-material
1. Merton RK. The Matthew Effect in Science. Science (1968) 159(3810):56–63. doi:10.1126/science.159.3810.56
2. Zuckerman H. Scientific Elite : Nobel Laureates in the United States. New York: Free Press (1977).
3. Zuckerman H. The Proliferation of Prizes: Nobel Complements and Nobel Surrogates in the Reward System of Science. Theor Med Bioeth (1992) 13(2):217–31. doi:10.1007/bf02163629
4. Meyers M. Prize Fight: The Race and the Rivalry to Be the First in Science. New York: St. Martin's Press (2012).
5. Ma Y, Uzzi B. Scientific Prize Network Predicts Who Pushes the Boundaries of Science. Proc Natl Acad Sci U.S.A (2018) 115(50):12608–15. doi:10.1073/pnas.1800485115
6. Jin C, Ma Y, Uzzi B. Scientific Prizes and the Extraordinary Growth of Scientific Topics. Nat Commun (2021) 12(1):1–11. doi:10.1038/s41467-021-25712-2
7. Zhuang W, Babin B, Xiao Q, Paun M. The Influence of Movie's Quality on its Performance: Evidence Based on Oscar Awards. Manag. Serv. Qual.:Inter. J. 24 (2), 122–138. doi:10.1108/MSQ-11-2012-0162
8. Aray H. Oscar Awards and Foreign Language Film Production: Evidence for a Panel of Countries. J Cult Econ (2021) 45(3):435–57. doi:10.1007/s10824-020-09402-3
9. Wasserman M, Mukherjee S, Scott K, Zeng XHT, Radicchi F, Amaral LAN. Correlations between User Voting Data, Budget, and Box Office for Films in the Internet Movie Database. J Assn Inf Sci Tec (2015) 66(4):858–68. doi:10.1002/asi.23213
10. Almadi K. Quantitative Study of the Movie Industry Based on IMDb Data. Boston: Massachusetts Institute of Technology (2017).
11. Wasserman M, Zeng XHT, Amaral LAN. Cross-evaluation of Metrics to Estimate the Significance of Creative Works. Proc Natl Acad Sci U.S.A (2015) 112(5):1281–6. doi:10.1073/pnas.1412198112
12. Oghina A, Breuss M, Tsagkias M, Rijke Md. Predicting Imdb Movie Ratings Using Social mediaEuropean Conference on Information Retrieval. Springer (2012).
13. Mestyán M, Yasseri T, Kertész J. Early Prediction of Movie Box Office success Based on Wikipedia Activity Big Data. PloS one (2013) 8(8):e71226.
14. Ramasco JJ, Dorogovtsev SN, Pastor-Satorras R. Self-organization of Collaboration Networks. Phys Rev E Stat Nonlin Soft Matter Phys (2004) 70(3):036106. doi:10.1103/PhysRevE.70.036106
15. Krauss J, Nann S, Simon D, Fischbach K, Gloor P (2008). "Predicting Movie success and Academy Awards through Sentiment and Social Network Analysis."
16. Jones BF, Weinberg BA. Age Dynamics in Scientific Creativity. Proc Natl Acad Sci U.S.A (2011) 108(47):18910–4. doi:10.1073/pnas.1102895108
17. Pohlhaus JR, Jiang H, Wagner RM, Schaffer WT, Pinn VW. Sex Differences in Application, Success, and Funding Rates for NIH Extramural Programs. Acad medicinejournal Assoc Am Med Colleges (2011) 86:759–67. doi:10.1097/acm.0b013e31821836ff
18. Otterbacher J. Gender, Writing and Ranking in Review Forums: a Case Study of the IMDb. Knowl Inf Syst (2013) 35(3):645–64. doi:10.1007/s10115-012-0548-z
19. Ma Y, Oliveira DFM, Woodruff TK, Uzzi B. Women Who Win Prizes Get Less Money and Prestige. Nature (2019) 565:287–8. doi:10.1038/d41586-019-00091-3
20. Amaral LAN, Moreira JAG, Dunand ML, Tejedor Navarro H, Lee HA. Long-term Patterns of Gender Imbalance in an Industry without Ability or Level of Interest Differences. PloS one (2020) 15(4):e0229662. doi:10.1371/journal.pone.0229662
21. Williams OE, Lacasa L, Latora V. Quantifying and Predicting success in Show Business. Nat Commun (2019) 10(1):2256–8. doi:10.1038/s41467-019-10213-0
22. Janosov M, Battiston F, Sinatra R. Success and luck in Creative Careers. EPJ Data Sci (2020) 9(1):9. doi:10.1140/epjds/s13688-020-00227-w
23. Borjas GJ, Doran KB. Prizes and Productivity. J Hum Resour (2015) 50(3):728–58. doi:10.3368/jhr.50.3.728
24. Shen H-W, Barabási A-L. Collective Credit Allocation in Science. Proc Natl Acad Sci U.S.A (2014) 111(34):12325–30. doi:10.1073/pnas.1401992111
25. English JF, English JF. The Economy of Prestige: Prizes, Awards, and the Circulation of Cultural Value. Harvard University Press (2009).
26. Busse TV, Mansfield RS. Theories of the Creative Process: A Review and a Perspective. J Creat Behav (1980) 14(2):91132–2. doi:10.1002/j.2162-6057.1980.tb00232.x
27. Stumpf H. Scientific Creativity: A Short Overview. Educ Psychol Rev (1995) 7(3):225–41. doi:10.1007/bf02213372
28. Yang Y, Chawla NV, Uzzi B. A Network's Gender Composition and Communication Pattern Predict Women's Leadership success. Proc Natl Acad Sci U.S.A (2019) 116(6):2033–8. doi:10.1073/pnas.1721438116
29. Ebadi A, Schiffauerova A. How to Become an Important Player in Scientific Collaboration Networks? J Informetrics (2015) 9(4):809–25. doi:10.1016/j.joi.2015.08.002
30. Petersen AM, Jung W-S, Yang J-S, Stanley HE. Quantitative and Empirical Demonstration of the Matthew Effect in a Study of Career Longevity. Proc Natl Acad Sci U.S.A (2011) 108(1):18–23. doi:10.1073/pnas.1016733108
31. Petersen AM, Riccaboni M, Stanley HE, Pammolli F. Persistence and Uncertainty in the Academic Career. Proc Natl Acad Sci U.S.A (2012) 109(14):5213–8. doi:10.1073/pnas.1121429109
32. Ke Q, Ahn Y-Y, Sugimoto CR. A Systematic Identification and Analysis of Scientists on Twitter. PLoS one (2017) 12(4):e0175368. doi:10.1371/journal.pone.0175368
33. Sinatra R, Wang D, Deville P, Song C, Barabási AL. Quantifying the Evolution of Individual Scientific Impact. Science (2016) 354(6312). doi:10.1126/science.aaf5239
34. Fraiberger SP, Sinatra R, Resch M, Riedl C, Barabási A-L. Quantifying Reputation and success in Art. Science (2018) 362(6416):825–9. doi:10.1126/science.aau7224
35. Liu L, Wang Y, Sinatra R, Giles CL, Song C, Wang D. Hot Streaks in Artistic, Cultural, and Scientific Careers. Nature (2018) 559(7714):396–9. doi:10.1038/s41586-018-0315-8
36. Reschke BP, Azoulay P, Stuart TE. Status Spillovers: The Effect of Status-Conferring Prizes on the Allocation of Attention. Administrative Sci Q (2018) 63(4):819–47. doi:10.1177/0001839217731997
37. Hirsch JE. An index to Quantify an Individual's Scientific Research Output. Proc Natl Acad Sci U.S.A (2005) 102(46):16569–72. doi:10.1073/pnas.0507655102
38. Page L, Brin S, Motwani R, Winograd T. The PageRank Citation Ranking: Bringing Order to the Web. Stanford InfoLab (1999).
Keywords: movie industry, award, career development, future success, computational social science
Citation: Liu Y and Ma Y (2022) Quantifying Award Network and Career Development in the Movie Industry. Front. Phys. 10:902890. doi: 10.3389/fphy.2022.902890
Received: 23 March 2022; Accepted: 16 May 2022;
Published: 22 June 2022.
Edited by:
Jian Gao, Northwestern University, United StatesReviewed by:
Lokman Meho, American University of Beirut, LebanonCopyright © 2022 Liu and Ma. This is an open-access article distributed under the terms of the Creative Commons Attribution License (CC BY). The use, distribution or reproduction in other forums is permitted, provided the original author(s) and the copyright owner(s) are credited and that the original publication in this journal is cited, in accordance with accepted academic practice. No use, distribution or reproduction is permitted which does not comply with these terms.
*Correspondence: Yifang Ma, bWF5ZkBzdXN0ZWNoLmVkdS5jbg==
Disclaimer: All claims expressed in this article are solely those of the authors and do not necessarily represent those of their affiliated organizations, or those of the publisher, the editors and the reviewers. Any product that may be evaluated in this article or claim that may be made by its manufacturer is not guaranteed or endorsed by the publisher.
Research integrity at Frontiers
Learn more about the work of our research integrity team to safeguard the quality of each article we publish.