- 1Data Science Research Center, Faculty of Science, Kunming University of Science and Technology, Kunming, China
- 2Potsdam Institute for Climate Impact Research, Potsdam, Germany
Particulate matter (PM2.5 and PM10) and ozone (O3) are the two major air pollutants in China in recent years. The fluctuations of PM2.5, PM10 and O3 strongly depend on the weather processes and anthropogenic emission. These processes may lead to the existence of short- and long-term memory behaviors in air pollutants. Hence, here we use the autoregressive parameter a of the first-order autoregressive process [AR (1)] to characterize the short-term memory effects of pollutants. We estimate the scaling exponent α using detrended fluctuation analysis (DFA) for the long-term memory effects of air pollutants (PM2.5, PM10, and O3) in summer and winter for different cities in China. Our results show that PM2.5, PM10, and O3 have strong short-term and long-term memory characteristics both in summer and winter. Furthermore, both the short- and long-term memory effects are stronger in winter than summer for most cities associated with stronger and longer persistent weather systems in winter. In general, the scaling exponent α of PM2.5 and PM10 are smaller for northern cities than those of southern cities in China. The long-term memory patterns of O3 are stronger in northern cities and weaker in southern cities in relative to those of PM2.5 and PM10 in winter. Our results show that the short- and long-term memory behaviors of air pollutions are dominated by the weather systems with different time scales.
Introduction
In recent decades, air pollution as a by-product of increased industrialization and urbanization, has been highly valued by the public and local government agencies in China. Air pollution regulation, air quality forecast and other related works have become critical issues to scientific researchers [1]. The sources of atmospheric pollutants are divided into natural and anthropogenic [2]. Anthropogenic sources include carbon and organic compounds emitted from heavy industries such as electric power, metal smelting and non-metallic mineral products, or emitted from the motor exhaust gas and coal combustion [3]. During 2013–2017, Beijing and other cities in north China suffered severe and persistent haze events caused by high fine particulate matter (PM2.5) concentrations [4]. The Chinese State Council issued powerful policies to restrict pollution emissions [5]. So far, PM2.5 concentrations have been reduced by 30% in China [5]. However, ozone (O3) concentrations show an increasing trend in recent years [6]. PM2.5 and O3 have been the two major air pollutants in most Chinese cities. Direct or indirect exposure to air pollutions PM2.5 and O3 can seriously damage our physical and mental health, causing respiratory infections, various contact allergies and other diseases [7].
Time dependence and temporal predictability of time series are associated with memory behavior of time series. Nature time series such as earthquake and climate records have been found to widely exit the memory behavior [8–10]. A short-term memory process can be expressed by a first-order autoregressive process and quantified by fitting the parameter of AR (1) [11]. For nature time series, usually both short and long-term memory processes exit. Yuan et al. [12] showed that Antarctic sea ice extent is not a simple short-term persistence time series, but is actually a combination of short- and long-term memory processes. To quantify such long-term memory of time series, Peng et al. [13] proposed Detrended Fluctuation Analysis (DFA) under the fractal theory [14, 15] for the first time to study the memory behavior of internal molecular chains of DNA. This method can filter out the trend component of its own evolution, and the remaining deviation sequence is the component of its own fluctuation [16–19]. Thus, it can eliminate the unreal correlation caused by the non-stationary characteristics of time series [20]. The DFA method has been successfully applied to seismology, stock market, biology, climate and environment, etc. [21–24]. For example, Lennartz et al. [25] and Fan et al. [23] used the DFA method to find that the memory exists in inter-occurrence seismic records. Yang et al. [26] estimate the persistence of precipitation over a wider range of scales and show that precipitation persistence can be described as a varying by DFA. Yuan et al. [27] observed temperature records of 12 stations from Antarctica island, coastline, and continental areas are analyzed by means of DFA and they found different long-term climate memory (LTM) behaviors.
Also, previous studies have found significant long-term (short-term) memory in air pollutant concentrations in some regions [28–30]. Liu et al. [31] found that there are different self–organized criticality process for pollutions SO2, NO2, and PM10 and the daily air pollution indices (API) associated with different power–law relations in Shanghai by using DFA and multifractal method. Also, Shi et al. [32] showed that the time series of three pollution indexes (SO2, NO2, and PM10) and the daily air pollution indexes (APIs) in China have a strong long-term memory within a year by three different methods. For other countries, the similar long-term memory behaviors of air pollution were also found i.e., Nikolopoulos et al. [33] used DFA to analyze PM10 time series in the Athens area (GAA) and found the long-memory patterns. Windsor et al. [34] examined the statistical characteristics of United Kingdom pollution time series and found evidences of high persistence and long-term memory of pollutant fluctuations up to 400 days. PM10 and O3 pollutants in the Caribbean region showed the multifractal nature with the significant Hurst parameter [35]. Wu et al. [36] studied the long-term persistence characteristics of several air pollutants (PM2.5 and O3) during the epidemic situation of COVID-19 by using multifractal detrended fluctuation analysis (MFDFA) and they found that the concentrations of three cities (Changsha, Zhuzhou, and Xiangtan) showed strong long-term persistence characteristics and multifractal structures. Shi et al. [37] comparatively analyzed the long-term persistence characteristics of PM2.5 evolution for eight air monitoring stations of Chengdu and the results showed that the spatial and temporal evolution of PM2.5 exhibit a long-term persistence. However, the above studies did not involve the spatial and temporal distribution characteristics of short- and long-term memory of air pollutants over China. In this paper, the DFA method is introduced to study the spatial evolution characteristics of memory of three pollutants (PM2.5, PM10, and O3) in 366 cities of China. Moreover, we compare the memory behaviors between short- and long-term scales.
The structure of this paper is as follows: in Section 2, the source of air pollution data and the data processing method are described. In Section 3, we give the specific steps of eliminating DFA method. Section 4 shows the results. Section 5 further summarizes our findings and draws the conclusion.
Data
Hourly time series of three air pollutants concentrations (PM2.5, PM10, O3) in 366 Chinese cities are downloaded from the website (https://quotsoft.net/air/). The time period is from 2015 to 2020. In this paper, we focus on air pollution concentrations in winter and summer over China. Here, we define November, December, January, and February as winter; and May, June, July, and August are defined as summer. The lengths of PM2.5, PM10, and O3 time series in winter and summer are shown in Table 1.
Methods
First of all, we remove the seasonal and daily trend from the original data, which can be represented as X
where
Short- and long-term correlated time series can be well described as a generalized AR (1) [12]:
where H is order of time step and a is the parameter of the first-order autoregressive process. The short-term memory of time series can be characterized by the parameter a. We can obtain the parameter a according to the least square method to estimate the AR (1) process of the detrended time series of pollution data [38].
In general, the DFA algorithm can be divided into five steps [26]:
1) For the detrended time series
where N is the total length of the time series,
2) Then we divide the sequence
3) We then obtained the regression of fitting trends
and from back to front is:
4) The fluctuation function
5) Taking different time scales
Results
First, we show samples of the detrended times series of PM2.5, PM10, and O3 concentrations as Eq. 1 for Beijing, Shanghai, Chengdu, and Guangzhou in Figure 1. There are larger fluctuations for PM2.5 and PM10 than other cities, especially in winter as shown in Figure 1A. Due to the implementation of environmental protection policies of China in recent years, we can clearly see that the fluctuation of PM2.5 is smaller in recent years in comparison to that of the earlier years. In Figure 1C, Guangzhou shows the smallest fluctuation for the PM pollution than other cities. This fluctuation can be strongly affected by meteorological factors [40–43]. The meteorological field is generally more intense in Northern China than Southern China. For the O3 times series, it shows completely different features with PM. The largest O3 fluctuation appears in summer related to the product of photochemical reactions. Furthermore, the O3 fluctuation seems to be more intense in Guangzhou than other cities. Also, the detrended O3 fluctuation does not show a significant increasing or decreasing trend with time for these four cities in Figure 1. Though the seasonal and daily cycles have been removed for the times series, we can still observe the large different characteristics between winter and summer such that we consider below the memory behaviors for winter and summer, respectively.
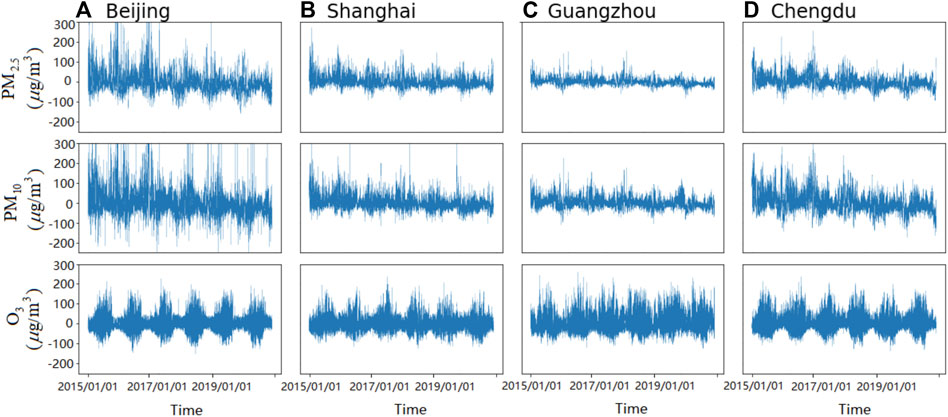
FIGURE 1. Samples of detrended time series of hourly PM2.5, PM10, and O3 concentrations for (A) Beijing, (B) Shanghai, (C) Guangzhou, and (D) Chengdu, respectively. The time span is from 2015.01.01 to 2020.12.31.
Next, we use the least square method to estimate the AR (1) process of the detrended time series of PM2.5, PM10, and O3 to obtain the autoregressive parameter a in Eq. 3. We show the spatial distribution of the autoregressive parameter a of 366 Chinese cities for summer and winter respectively in Figure 2. By comparing the autoregression parameters a of PM2.5, PM10, and O3, we find that the short-term memory effects of pollutants have seasonal and regional differences. And the short-term scale is hour-to-hour. For summer, particulate matters in central and eastern China show stronger short-term memory behavior, especially around the Sichuan Basin and Hubei Province. The values of individual cities are as high as 0.98. For most winter cites, the short-term memory effects of PM2.5 and PM10 were stronger than those shown in the summer. Compared with PM2.5 and PM10, the short-term memory of O3 varies less, and the a value is stable at around 0.9 or lower in different regions and seasons. The results suggest that compared with O3, PM2.5, and PM10 are more dependent on the local meteorological conditions, which strongly influenced by the short-term memory behavior. In winter, the meteorological conditions are stronger and the boundary layer is lower than in summer leading to the stronger short-term memory behaviors of PM2.5 and PM10. The higher value of a with stronger short memory in PM2.5 and PM10 over southeast China corresponds to higher intrinsic predictability [44]. Studies also show that other meteorological variables over southeast China exhibit higher intrinsic predictability [45]. The results also show the contrast performance of a-values in South and North China, which will be discussed further in later chapters.
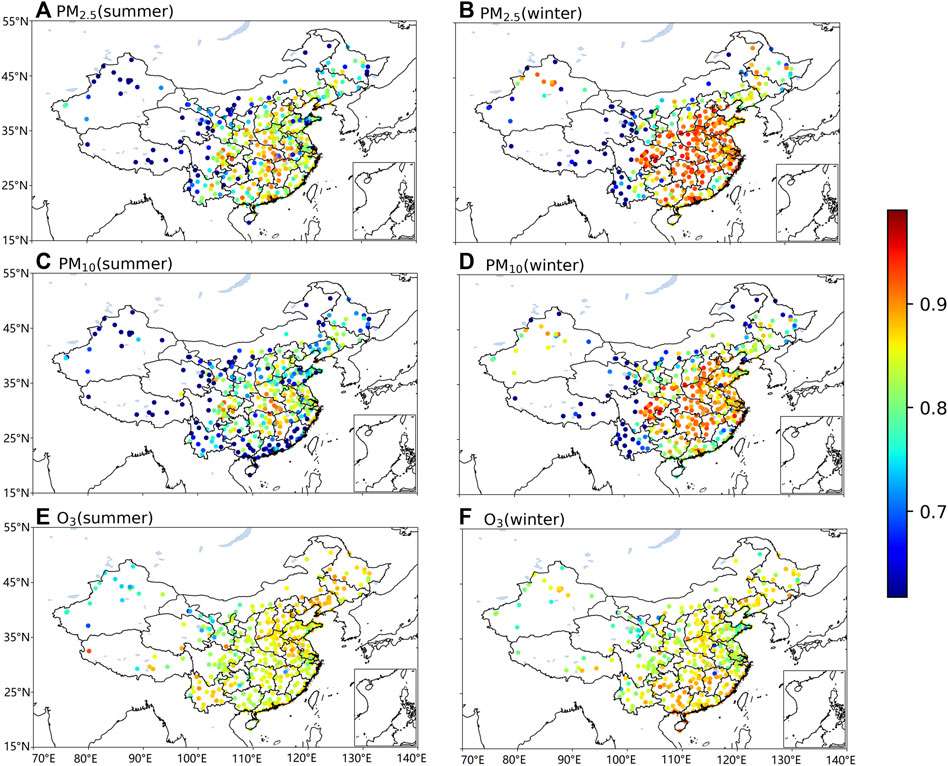
FIGURE 2. (color online) Spatial distributions of the autoregression parameter a for AR (1) process for (A) PM2.5, (C) PM10 and (E) O3 in summer. (B), (D), and (F) are same as (A), (C), and (D) but in winter.
Then, we study the long-term memory of pollutions and implement the DFA analysis to the detrended time series of PM2.5, PM10, and O3 for the four cities. And the long-term scale is month-to-month. According to Eq. 7, we can obtain the fluctuation F(t) as a function of time scale t for summer (see Figure 3). Previous studies [46, 47] pointed out that due to the effect of limited length of time series, the beginning part of F(t) may be affected by short-term memory effect and lead to a overestimated DFA index. For the AR (1) process, the reliable time scale t to fit scale index
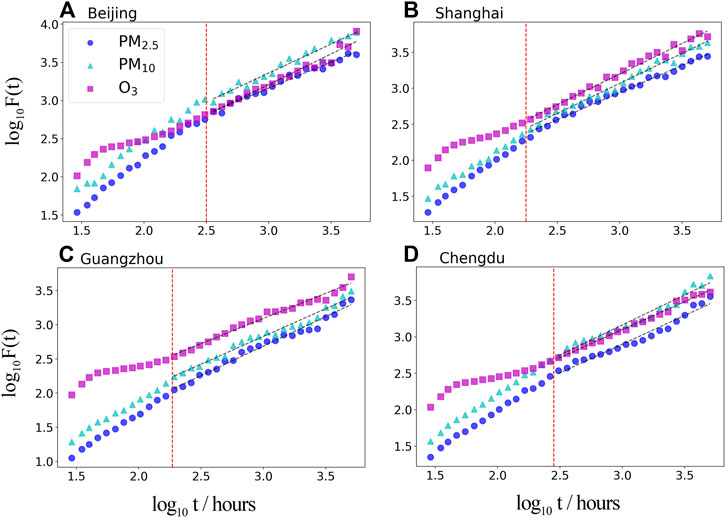
FIGURE 3. (color online) The DFA analysis in summer for (A) Beijing, (B) Shanghai, (C) Guangzhou, and (D) Chengdu. Black dashed lines are fitted power-law curves. Red dashed lines represents the location of the crossover point
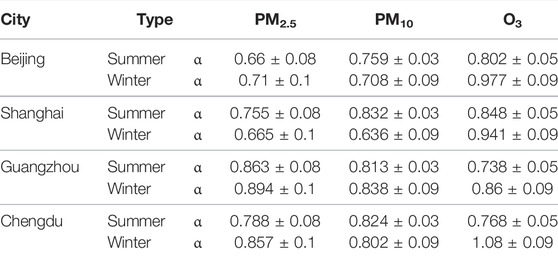
TABLE 2. The DFA scale index
We show the results of winter in Figure 4. There are clear differences between the time scales below and above sx. The index
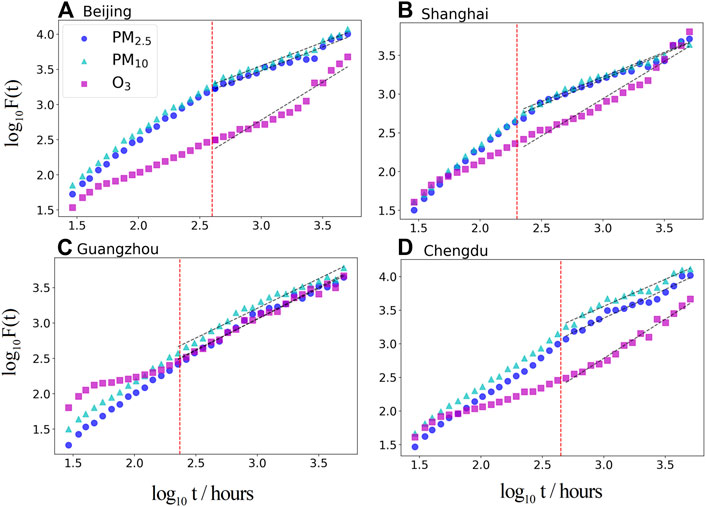
FIGURE 4. (color online) The DFA analysis in winter for (A) Beijing, (B) Shanghai, (C) Guangzhou and (D) Chengdu. Black dashed lines are fitted power-law curves. Red dashed lines represents the location of the crossover point
To comprehensively understand spatial patterns of the memory of pollutants in China, we further study the spatial distribution of the DFA index
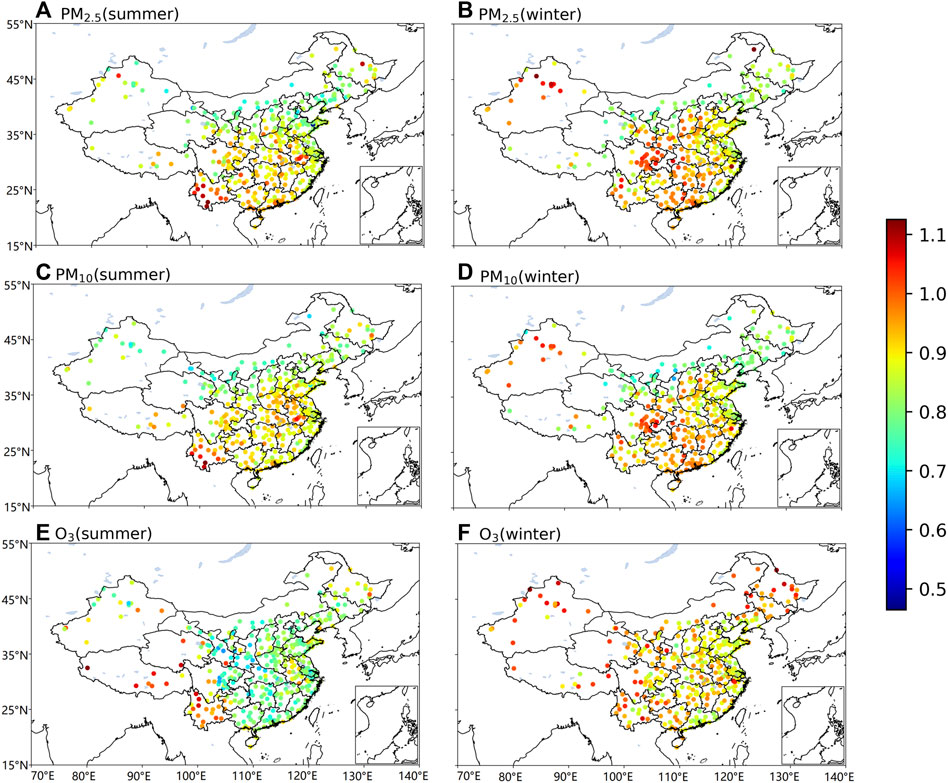
FIGURE 5. (color online) Spatial distributions of the DFA scale exponent
However, the seasonal differences could be affected by the monsoon system. In winter, China is controlled by Siberian high, and the air mass is dry, which is not conducive to precipitation. The atmospheric stratification is relatively stable, and it is easy to accumulate aerosols in the lower atmosphere, which is not conducive to pollutant diffusion. We find that the
Discussions and Conclusion
In this study, we use the AR (1) process and the DFA method to quantify the memory characteristics of the time series of three air pollutants (PM2.5, PM10, O3) in China. According to the AR (1) process, we obtain the spatial distribution of the autoregressive parameter a of 366 Chinese cities for summer and winter respectively which is associated with the short-term memory behavior. The short-term memory of particulate matter in most parts of southern China is stronger, especially in the Yangtze River Basin, while the short-term memory of O3 is slightly different between the north and the south. Moreover, the short-term memory of PM2.5 and PM10 in winter are stronger than in summer related to the stronger short-term and local meteorological field. In the previous studies [31, 32], they found two different scaling exponents of DFA in short- and long-term scales for PM10 in Shanghai in consistent with us. However, our results suggest that using the scaling exponent of DFA in short-term scale to quantify memory is problematic, since the DFA index can by strongly affected by the limited length of time series [46, 47]. Thus, it is better to use the autoregressive parameter of the AR (1) process.
To quantify the long-term memory behavior, we study the DFA index of different pollutants in Beijing, Shanghai, Chengdu and Guangzhou in winter and summer. We find that the three pollutants in four representative cities have strong long-term memory (the DFA index
Data Availability Statement
The original contributions presented in the study are included in the article/Supplementary Material, further inquiries can be directed to the corresponding author.
Author Contributions
YZ designed research; PY performed research; PY, YZ and DN analyzed data; PY, WL, YZ and PQ wrote the paper. PY, PQ, DN, WL and YZ contributed to reviewing the manuscript. All authors have read and approved the final manuscript.
Funding
This work was supported by the National Natural Science Foundation of China (No. 61573173).
Conflict of Interest
The authors declare that the research was conducted in the absence of any commercial or financial relationships that could be construed as a potential conflict of interest.
Publisher’s Note
All claims expressed in this article are solely those of the authors and do not necessarily represent those of their affiliated organizations, or those of the publisher, the editors and the reviewers. Any product that may be evaluated in this article, or claim that may be made by its manufacturer, is not guaranteed or endorsed by the publisher.
Acknowledgments
We are grateful for the data resources provided by https://quotsoft.net/air/.
References
1. Tong R, Wang Y, Zhao X, Ma X, Yang X. Comprehensive Comparative Analysis of Air Pollutants Exposure in Different Regions of mainland China: Assessment of Health Impacts and Economic burden. Atmos Pollut Res (2021) 12(10):101210. doi:10.1016/j.apr.2021.101210
2. Song J, Wang B, Yang W, Duan H, Liu X. Extracting Critical Supply Chains Driving Air Pollution in China. J Clean Prod (2020) 276:124282. doi:10.1016/j.jclepro.2020.124282
3. Liu H, Fang C, Zhang X, Wang Z, Bao C, Li F. The Effect of Natural and Anthropogenic Factors on Haze Pollution in Chinese Cities: a Spatial Econometrics Approach. J Clean Prod (2017) 165:323–33. doi:10.1016/j.jclepro.2017.07.127
4. Zhang Z, Zhang X, Gong D, Quan W, Zhao X, Ma Z, et al. Evolution of Surface O3 and PM2.5 Concentrations and Their Relationships with Meteorological Conditions over the Last Decade in Beijing. Atmos Environ (2015) 108:67–75. doi:10.1016/j.atmosenv.2015.02.071
5. Zhang Q, Zheng Y, Tong D, Shao M, Wang S, Zhang Y, et al. Drivers of Improved PM 2.5 Air Quality in China from 2013 to 2017. Proc Natl Acad Sci U.S.A (2019) 116:24463–9. doi:10.1073/pnas.1907956116
6. Shao M, Wang W, Yuan B, Parrish DD, Li X, Lu K, et al. Quantifying the Role of PM2.5 Dropping in Variations of Ground-Level Ozone: Intercomparison between Beijing and Los Angeles. Sci. Total Environ. (2021) 788(20):147712. doi:10.1016/j.scitotenv.2021.147712
7. Song Y, Zhang Y, Wang T, Qian S, Wang S. Spatio-temporal Differentiation in the Incidence of Influenza and its Relationship with Air Pollution in China from 2004 to 2017. Chin Geogr Sci (2021) 31(5):815–28. doi:10.1007/s11769-021-1228-2
8. Zhang Y, Zhou D, Fan J, Marzocchi W, Ashkenazy Y, Havlin S. Improved Earthquake Aftershocks Forecasting Model Based on Long-Term Memory. New J Phys (2021) 23:042001. doi:10.1088/1367-2630/abeb46
9. Zhang Y, Fan J, Marzocchi W, Shapira A, Hofstetter R, Havlin S, et al. Scaling Laws in Earthquake Memory for Interevent Times and Distances. Phys Rev Res (2020) 2:013264. doi:10.1103/PhysRevResearch.2.013264
10. Nian D, Yuan N, Ying K, Liu G, Fu Z, Qi Y, et al. Identifying the Sources of Seasonal Predictability Based on Climate Memory Analysis and Variance Decomposition. Clim Dyn (2020) 55(11):3239–52. doi:10.1007/s00382-020-05444-7
11. Franzke CLE, Barbosa S, Blender R, Fredriksen HB, Laepple T, Lambert F, et al. The Structure of Climate Variability across Scales. Rev Geophys (2020) 58(2):e2019RG000657. doi:10.1029/2019RG000657
12. Yuan N, Ding M, Ludescher J, Bunde A. Increase of the Antarctic Sea Ice Extent Is Highly Significant Only in the Ross Sea. Sci Rep (2017) 7:41096. doi:10.1038/srep41096
13. Peng C-K, Buldyrev SV, Havlin S, Simons M, Stanley HE, Goldberger AL. Mosaic Organization of DNA Nucleotides. Phys Rev E (1994) 49(2):1685–9. doi:10.1103/physreve.49.1685
14. Potter KW. Annual Precipitation in the Northeast United States: Long Memory, Short Memory, or No Memory? Water Resour Res (1979) 15(2):340–6. doi:10.1029/WR015i002p00340
15. Yuan N, Fu Z, Mao J. Different Scaling Behaviors in Daily Temperature Records over China. Physica A: Stat Mech its Appl (2010) 389(19):4087–95. doi:10.1016/j.physa.2010.05.026
16. Talkner P, Weber RO. Power Spectrum and Detrended Fluctuation Analysis: Application to Daily Temperatures. Phys Rev E (2000) 62(1):150–60. doi:10.1103/PhysRevE.62.150
17. Costa RL, Vasconcelos GL. Long-range Correlations and Nonstationarity in the Brazilian Stock Market. Physica A (2003) 329(1):231–48. doi:10.1016/S0378-4371(03)00607-1
18. Lin G, Chen X, Fu Z. Temporal-spatial Diversities of Long-Range Correlation for Relative Humidity over China. Physica A: Stat Mech Appl (2007) 383(2):585–94. doi:10.1016/j.physa.2007.04.059
19. Govindan RB, Vjushin D, Brenner S, Bunde A, Havlin S, Schellnhuber HJ. Long-range Correlations and Trends in Global Climate Models: Comparison with Real Data. Physica A (2001) 294(1-2):239–48. doi:10.1016/S0378-4371(01)00110-8
20. Kantelhardt JW, Koscielny-Bunde E, Rego HHA, Havlin S, Bunde A. Detecting Long-Range Correlations with Detrended Fluctuation Analysis. Physica A (2001) 295(3):441–54. doi:10.1016/S0378-4371(01)00144-3
21. Auno S, Lauronen L, Wilenius J, Peltola M, Vanhatalo S, Palva JM. Detrended Fluctuation Analysis in the Presurgical Evaluation of Parietal Lobe Epilepsy Patients. Clin Neurophysiol (2021) 132(7):1515–25. doi:10.1016/j.clinph.2021.03.041
22. Sanyal MK, Ghosh I, Jana RK. Characterization and Predictive Analysis of Volatile Financial Markets Using Detrended Fluctuation Analysis, Wavelet Decomposition, and Machine Learning. IJDA (2021) 2(1):1–31. doi:10.4018/IJDA.2021010101
23. Fan J, Zhou D, Shekhtman LM, Shapira A, Hofstetter R, Marzocchi W, et al. Possible Origin of Memory in Earthquakes: Real Catalogs and an Epidemic-type Aftershock Sequence Model. Phys Rev E (2019) 99(4-1):042210. doi:10.1103/PhysRevE.99.042210
24. Lachowycz SM, Pyle DM, Mather TA, Varley NR, Odbert HM, Cole PD, et al. Long-range Correlations Identified in Time-Series of Volcano Seismicity during Dome-Forming Eruptions Using Detrended Fluctuation Analysis. J Volcanol Geothermal Res (2013) 264:197–209. doi:10.1016/j.jvolgeores.2013.07.009
25. Lennartz S, Livina VN, Bunde A, Havlin S. Long-term Memory in Earthquakes and the Distribution of Interoccurrence Times. Europhys Lett (2008) 81(6):69001. doi:10.1209/0295-5075/81/69001
26. Yang L, Fu Z. Process-dependent Persistence in Precipitation Records. Physica A: Stat Mech Appl (2019) 527:121459. doi:10.1016/j.physa.2019.121459
27. Yuan N, Ding M, Huang Y, Fu Z, Xoplaki E, Luterbacher J. On the Long-Term Climate Memory in the Surface Air Temperature Records over Antarctica: A Nonnegligible Factor for Trend Evaluation. J Clim (2015) 28(15):5922–34. doi:10.1175/JCLI-D-14-00733.1
28. Zhang Y, Chen D, Fan J, Havlin S, Chen X. Correlation and Scaling Behaviors of fine Particulate Matter (PM 2.5 ) Concentration in China. EPL (2018) 122(5):58003. doi:10.1209/0295-5075/122/58003
29. Lin W, Xu X, Ma Z, Zhao H, Liu X, Wang Y. Characteristics and Recent Trends of Sulfur Dioxide at Urban, Rural, and Background Sites in North China: Effectiveness of Control Measures. J Environ Sci (2012) 24(1):34–49. doi:10.1016/S1001-0742(11)60727-4
30. Mouzourides P, Kyprianou A, Neophytou MK-A. Exploring the Multi-Fractal Nature of the Air Flow and Pollutant Dispersion in a Turbulent Urban Atmosphere and its Implications for Long Range Pollutant Transport. Chaos (2021) 31(1):013110. doi:10.1063/1.5123918
31. Liu Z, Wang L, Zhu H. A Time-Scaling Property of Air Pollution Indices: a Case Study of Shanghai, China. Atmos Pollut Res (2015) 6(5):886–92. doi:10.5094/APR.2015.098
32. Kai S, Chun-qiong L, Nan-shan A, Xiao-hong Z. Using Three Methods to Investigate Time-Scaling Properties in Air Pollution Indexes Time Series. Nonlinear Anal Real World Appl (2008) 9(2):693–707. doi:10.1016/j.nonrwa.2007.06.003
33. Nikolopoulos D, Moustris K, Petraki E, Cantzos D. Long-memory Traces in $$\hbox {PM}_{10}$$ Time Series in Athens, Greece: Investigation through DFA and R/S Analysis. Meteorol Atmos Phys (2021) 133(2):261–79. doi:10.1007/s00703-020-00744-3
34. Windsor HL, Toumi R. Scaling and Persistence of UK Pollution. Atmos Environ (2001) 35(27):4545–56. doi:10.1016/S1352-2310(01)00208-4
35. Plocoste T, Calif R, Jacoby-Koaly S. Temporal Multiscaling Characteristics of Particulate Matter PM10 and Ground-Level Ozone O3 Concentrations in Caribbean Region. Atmos Environ (2017) 169:22–35. doi:10.1016/j.atmosenv.2017.08.068
36. Wu B, Liu CQ, Zhang J, Li YH, Chen YB, Wen Y, et al. Nonlinear Dynamic Mechanism of Regional Air Pollution during the COVID-19 Lockdown. China Environ.Sci (2021) 41(5):2028–39. doi:10.19674/j.cnki.issn1000-6923.2021.0213
37. Wu SH, Shi K, Liu CQ, Li SC, Huang Y, Yin H. Long-term Persistence Characteristics of PM2.5 Evolution during a Typical Haze Episode in Chengdu. Environ Sci Technol (2014) 37(10):9–14. doi:10.3969/j.issn.1003-6504.2014.10.002
38. Chen BW, Xia L. Research on Parameter Estimation of AR(p) Model Based on Approximate Bayesian Computation. Adv Appl Math (2021) 10(3):680–8. doi:10.12677/AAM.2021.103074
39. Peng CK, Hausdorff JM, Havlin S, Mietus JE, Stanley HE, Goldberger AL. Multiple-time Scales Analysis of Physiological Time Series under Neural Control. Physica A (1998) 249(1):491–500. doi:10.1016/s0378-4371(97)00508-6
40. Tai APK, Mickley LJ, Jacob DJ. Correlations between fine Particulate Matter (PM2.5) and Meteorological Variables in the United States: Implications for the Sensitivity of PM2.5 to Climate Change. Atmos Environ (2010) 44(32):3976–84. doi:10.1016/j.atmosenv.2010.06.060
41. Li L, Qian J, Ou C-Q, Zhou Y-X, Guo C, Guo Y. Spatial and Temporal Analysis of Air Pollution Index and its Timescale-dependent Relationship with Meteorological Factors in Guangzhou, China, 2001-2011. Environ Pollut (2014) 190:75–81. doi:10.1016/j.envpol.2014.03.020
42. Yang X, Wang S, Zhang W, Zhan D, Li J. The Impact of Anthropogenic Emissions and Meteorological Conditions on the Spatial Variation of Ambient SO2 Concentrations: A Panel Study of 113 Chinese Cities. Sci Total Environ (2017) 584-585:318–28. doi:10.1016/j.scitotenv.2016.12.145
43. Zhang Y, Fan J, Chen X, Ashkenazy Y, Havlin S. Significant Impact of Rossby Waves on Air Pollution Detected by Network Analysis. Geophys Res Lett (2019) 46(21):12476–85. doi:10.1029/2019GL084649
44. Huang Y, Fu Z. Enhanced Time Series Predictability with Well-Defined Structures. Theor Appl Climatol (2019) 138:373–85. doi:10.1007/s00704-019-02836-6
45. Fu S, Huang Y, Feng T, Nian D, Fu Z. Regional Contrasting DTR's Predictability over China. Physica A: Stat Mech Appl (2019) 521(1):282–92. doi:10.1016/j.physa.2019.01.077
46. Höll M, Kantz H. The Fluctuation Function of the Detrended Fluctuation Analysis - Investigation on the AR(1) Process. Eur Phys J B (2015) 88(5):1–9. doi:10.1140/epjb/e2015-60143-1
47. Maraun D, Rust HW, Timmer J. Tempting Long-Memory - on the Interpretation of DFA Results. Nonlin Process. Geophys (2004) 11:495–503. doi:10.5194/npg-11-495-2004
48. Zhao Z, Xi H, Russo A, Du H, Gong Y, Xiang J, et al. The Influence of Multi-Scale Atmospheric Circulation on Severe Haze Events in Autumn and Winter in Shanghai, China. Sustainability (2019) 11(21):5979. doi:10.3390/su11215979
49. Leung DM, Tai APK, Mickley LJ, Moch JM, van Donkelaar A, Shen L, et al. Synoptic Meteorological Modes of Variability for fine Particulate Matter (PM2.5) Air Quality in Major Metropolitan Regions of China. Atmos Chem Phys (2018) 18:6733–48. doi:10.5194/acp-18-6733-2018
50. Wise E, Comrie A. Meteorologically Adjusted Urban Air Quality Trends in the Southwestern United States. Atmos Environ (2005) 39(16):2969–80. doi:10.1016/j.atmosenv.2005.01.024
Keywords: memory behavior, air pollutions, PM2.5, PM10, O3, China
Citation: Yu P, Nian D, Qiao P, Liu W and Zhang Y (2022) Memory Behaviors of Air Pollutions and Their Spatial Patterns in China. Front. Phys. 10:875357. doi: 10.3389/fphy.2022.875357
Received: 14 February 2022; Accepted: 23 March 2022;
Published: 12 April 2022.
Edited by:
Jingfang Fan, Beijing Normal University, ChinaReviewed by:
Rudy Calif, Université des Antilles, GuadeloupeJun Meng, Beijing University of Posts and Telecommunications (BUPT), China
Copyright © 2022 Yu, Nian, Qiao, Liu and Zhang. This is an open-access article distributed under the terms of the Creative Commons Attribution License (CC BY). The use, distribution or reproduction in other forums is permitted, provided the original author(s) and the copyright owner(s) are credited and that the original publication in this journal is cited, in accordance with accepted academic practice. No use, distribution or reproduction is permitted which does not comply with these terms.
*Correspondence: Yongwen Zhang, emhhbmd5b25nd2VuNzdAZ21haWwuY29t