- 1College of Administrative Sciences and Economics, Koç University, Istanbul, Turkey
- 2Department of Economics, American University of Beirut, Beirut, Lebanon
- 3Centre for Economic Policy Research, London, United Kingdom
In this paper, we construct a Twitter-based high-frequency Economic Policy Uncertainty (TEPU) index built on a select set of Twitter user accounts whose tweets are considered to reflect expert opinion on the topic. We study the relationship between the TEPU index and a set of key financial indicators for tracking financial developments in Turkey over the sample period 2013–2021. Based on the results from a vector autoregressive analysis, we find evidence that changes in expert opinion described by fluctuations in the TEPU index interact with fluctuations in financial indicators such as the exchange rate and the stock market index to capture information about high frequency events during our sample period. Second, fluctuations in the TEPU index emerge as a key indicator that helps to predict the country risk premium measured by the CDS spread. We also find evidence that the conditional volatility of the different series reflects salient events that occurred over our sample period.
1 Introduction
On 20 December 2021, the exchange rate hit a record low at 18.36 Turkish Lira (TRY) against the US Dollar (USD) after falling from a value of 8.29 TRY against the USD on September 2021. It rebounded back to a value of 10.78 TRY per USD by 23 December 2021 following the announcement of two foreign-currency indexed saving schemes that link the interest rate received on Turkish lira deposits to fluctuations in the exchange rate.1 The factors instigating this fall were the removal of two deputy governors and one member of the Monetary Policy Committee (MPC) at the Central Bank of the Republic of Turkey (CBRT) and subsequent cuts in the policy rate of 500 basis points beginning with the MPC meeting on 23 September 2021. These developments were accompanied by large increases in Turkey’s CDS spreads on its sovereign bonds as well as significant volatility in its stock market.2
In this paper, we construct a daily-frequency Twitter-based Economic Policy Uncertainty (TEPU) index that aims to track such high frequency developments in Turkey’s economic and political arena—spanning even a few hours in a given day that cannot be captured through the standard newspaper–based economic policy uncertainty (EPU) indices [1]. To the best of our knowledge, our paper is the first to test the ability of an EPU index for capturing and conveying information about developments in a highly volatile policy environment for a leading emerging market economy, Turkey.
Earlier [2] constructs an EPU index for Turkey using the Dow Jones’ Factiva global news database over the period 1996–2017 and examine the relationship between their EPU index and sectoral data on investment and employment. They argue that their use of mainly foreign newspapers rather than Turkish newspapers as the primary source for this index may be a preferable approach, as foreign newspapers directly capture international perceptions on Turkey’s EPU index and foreign news sources span longer periods than Turkish newspapers. [3] and [4] also create EPU indices for Turkey based on the digital archives of several daily Turkish newspapers but none of these analyses go beyond 2018. By contrast, the usage of Twitter data in our analysis facilitates the creation of an EPU index at monthly, weekly and even daily frequencies. The timely character of Twitter data enables us to update our forward-looking TEPU index in real time. This way, our TEPU index captures the effects of the Covid-19 shock of 2020 as well as the fast-moving anomalous developments that occurred in Turkish financial markets during 2021.
From a practical point of view, there are many reasons to make use of Twitter data as an alternative to those extracted from conventional media platforms. In the past decade, the Twitter platform has provided a direct, instantaneous, and network-enhanced channel through which economic policy experts communicate with the public. Economic policy experts can now freely convey their “real-time” opinions on economic and finance matters to a large social network. As Twitter feeds capture a diversity of opinion in a nearly instantaneous fashion that is typically filtered in newspaper articles and reported with a considerable time lag, our TEPU index enables us to account for a variety of different views transmitted within this network in our analysis.
Recent studies in the relevant literature have examined data sets derived from popular social media platforms such as Twitter, Facebook, blogs etc. [5] use the Twitter Application Programming Interface (API) to extract all tweets containing the words “uncertain,” “uncertainly,” “uncertainties” “uncertainty.” and generate an EPU index. They use geotagged tweets and information about users’ followers to construct their index based on tweets sent by users located in the US. Utilizing the Twitter accounts of 15 Chilean mass media specialized in or with material devoted to economics, [6] constitute a daily-frequency EPU index for Chile based on Twitter data. Their constructed indices seek to capture the level of general disagreement about economic policies and the current economic situation, respectively, over the period 2012–2019 in Chile.
Our paper differs from these existing studies that are typically based on the use of bulk Twitter data and that potentially suffer from noisy observations. In contrast to these analyses, we use a user list curation process to restrict our analysis to a select group of Twitter users. Using web scraping methods, we are able to extract recent tweets posted by these selected accounts easily and use them to update our TEPU index for capturing the extremely fast daily developments in the Turkish economy in a timely manner.3 To avoid any bias in TEPU index that might potentially occur due to the selection of user accounts within our user list curation process, we run the bootstrapping algorithm repetitively with different seed lists, which results in the same final candidate list in all repetitions. Furthermore, our bootstrapping algorithm enables us to prevent errors arising from the Turkish language applications of Natural Language Processing (NLP) methods as well as to present a novel approach to the subject of content-based EPU indexing.
By implementing the different phases of our bootstrapping algorithm, we obtain a group of selected Twitter users that have expertise in economics and finance. The final candidate list of 661 users is comprised of academics, company executives, politicians, consultants, journalists, portfolio managers, and 218 bureaucrats. Figure 1 depicts the connections in the network of this selected group. These connections illustrate the following-follower status of the selected user accounts and the size of the nodes shows their relative influence. This figure suggests that there is a very strong interaction among the Twitter users in this group in that they are influenced by each other through their interactions and moreover, influence the public through their high number of followers. Hence, it is reasonable to think of this group as a viable network of Twitter user accounts by which we can capture the role of expert opinion in identifying economic policy uncertainty in Turkey. Our approach involves building an EPU index based on the real-time interactions of this network, which allows for a fast-moving way of capturing expert opinion in such a volatile environment.
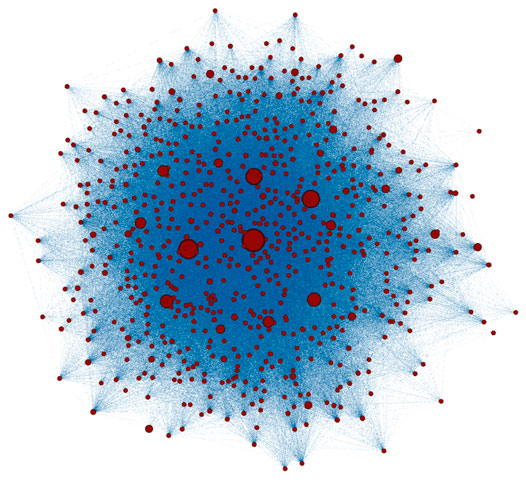
FIGURE 1. Network of selected Twitter users. Nodes indicate the selected user accounts in Twitter. The size of the nodes reflects the number of their followers.
Turkey offers the case of an emerging market economy where market signals regarding monetary policy have become blurred by the pursuit of unconventional monetary policy as well as policy reversals, especially since 2020. In our analysis, we seek to understand the interaction between our measure of expert opinion summarized by the TEPU index and salient financial indicators for tracking the changes in financial markets that have been occurring in Turkey over the sample period. For this purpose, we derive our results from a Structural Vector Autoregressive (SVAR) analysis model that allows for dynamic inter-relationships in the conditional mean of the different series. We also use a Multivariate Generalized Autoregressive Conditional Heteroscadasticity (M-GARCH) model for understanding the volatility dynamics in the financial series [7]. By conducting a weekly frequency-based analysis, we investigate the full sample that covers the period 2013–2021 as well as several sub-samples to understand the effects of monetary policy changes and unconventional monetary policy being conducted during this period.
Our results show that fluctuations in the TEPU index and financial indicators such as the BIST-100 index and the USD/TRY exchange rate interact to capture information about political and economic developments that occur during the sample period 2013–2021. In particular, the TEPU index and the USD/TRY exchange rate are two key indicators which reflect monetary policy surprises and monetary policy announcements arising from the pursuit of unconventional monetary policy in recent years in Turkey. The TEPU index also emerges as a key predictor of changes in the CDS spread, suggesting that expert opinion itself contains information useful for evaluating the country’s risk premium [8]. The fluctuations in the TEPU index together with the USD/TRY exchange rate and the CDS spread also help predict fluctuations in equity markets in Turkey. A novel part of our analysis is that we document how increases in the conditional volatility of one indicator are transmitted to the conditional volatility in the remaining financial indicators. In a tumultuous policy environment similar to the one observed Turkey, we find that the conditional volatility of the different financial indicators respond to salient events that occurred over our sample period.
Our results relate to the literature that has found predictive effects of commodity prices such as oil prices on the economic policy index that, in turn, may affect equity prices for developed countries [9,10] as well as studies for emerging market economies that examine the role of economic policy uncertainty on real outcomes in these economies [11]. Our results differ from these papers in that we focus on a period of large monetary shocks and monetary policy reversals for a major emerging market economy. While we do not provide an analysis of the effects of economic policy uncertainty on real outcomes, our results nevertheless provide novel information about the channels through which economic policy uncertainty impacts high-frequency developments observed in Turkish financial markets.
The remainder of this paper is organized as follows. Section 2 provides a literature review. Section 3 explains in detail how we construct the Twitter-based economic policy uncertainty index for Turkey. Section 4 firstly provides details regarding data used in our econometric analysis and secondly conducts an event analysis to show how our TEPU index relates to important political and economic events over the sample period 2013–2021. It also tracks developments in Turkish financial markets during this period. Section 5 is devoted to the details of econometric methodology used in the analysis and explains the results. Section 6 concludes.
2 Literature Review
The question of how households, businesses and policy makers shape their economic activities as a response to any changes in uncertainty has been widely asked in the literature.4
A theoretical strand of the related literature aims to identify the channels through which uncertainty can have an impact on economic outcomes. First, a rise in uncertainty encourages households and businesses to delay their costly-to-reverse expenditures. During the times of high uncertainty households are more likely to delay their purchases on durable goods [12]. When the costs of irreversible investments and hiring decisions are high, firms facing high uncertainty tend to adjust and delay the timing of such actions [13]. [14] and [15] show that heightened uncertainty affects investment behavior by creating an option value of waiting. In uncertain times, firms prefer to delay capital expenditures involving sunk costs until more information is revealed.
Second, uncertainty can also have real option effects on employment. [16] suggests that when search frictions are the only costs associated with the reallocation of labor, real option effects of uncertainty are weak if firms can easily reverse their employment decisions. A rise in uncertainty can also encourage firms to adjust on flexible margins such as part-time employment due to the existence of fixed costs of hiring and firing [17]. Third, it is shown that unexpected changes in uncertainty regarding fiscal policy affect economic activity, leading to a sizable adverse effect on output ([18] and [19]). Fourth, uncertainty that works through growth options mechanism might have a positive impact on long run growth. In a recent study, [20] shows that R&D intensive firms can raise their stock value by taking advantage of growth opportunities particularly at times of high uncertainty. Fifth, studies point to financial frictions as one of the key mechanisms through which fluctuations in uncertainty can have an adverse effect on investment by raising risk premiums ([21] and [22]).
Another strand of the literature examines contractionary effects of uncertainty with reference to decreasing household spending. In this vein, [23] model such behavior as a precautionary response to uncertainty and increasing costs of finance arising from risk premium adjustments. Lastly, a group of studies focuses explicitly on economic policy uncertainty and examines the negative impacts of uncertainty derived solely from policy developments. Among other studies [24–28], and [1] note the depressive effects of uncertainty arising from monetary, fiscal, and regulatory policies on economic aggregates, investment and business cycle volatility. The survey-based evidence shown in [29] suggests that higher trade policy uncertainty since 2017 has dampened U.S. business investment. Moreover, [30] indicate that uncertainty regarding trade policy reduced U.S. business investment by about 1.5% in 2018.
In recent years, many of the empirical studies in the related literature endeavor to develop accurate empirical measures for uncertainty. The reasons for the recent surge in interest range from the ease to accessing online news archives and digital archives of opinionated data, to the availability of firm- and household-level data, to the application of NLP techniques that allows researchers to save memory. A relatively new and growing field aims to develop a measure for economic policy uncertainty by using text search methods. The well-known economic uncertainty index created by [1] is a monthly index for the US economy based on the frequency of a selected list of words in 10 leading US newspapers since 1985.5 More recently, [31] construct a new uncertainty index, the World Uncertainty Index (WUI), by calculating the frequency of the word “uncertainty” within the country reports released by Economist Intelligence Unit for 143 individual countries including Turkey on a quarterly basis from 1996 onward.
The measurement of economic policy uncertainty and its role in affecting real and financial outcomes have been investigated in numerous empirical studies. Using an SVAR framework, [32] studies the impact of heightened uncertainty that is associated with major economic and political shocks like Cuban Missile crisis, the assassination of JFK, the OPEC I oil-price shock, and the 9/11 terrorist attacks. The simulation results of this study show that uncertainty generates a 1% drop and rebound in output, employment, and productivity growth, leading to powerful real-option effects, which are due to firms using their incentive to delay their investment and hiring decisions. [33] develop a time-varying measure of uncertainty as the conditional volatility of the unforeseeable components of the future values of a set of key macroeconomic variables. They report fewer incidences of uncertainty compared to other approaches such as [32] and find that their measure of uncertainty is bunched around three deep recessions. The results of their Vector Autoregressive (VAR) analysis suggest that macro uncertainty explains 29% of the forecast error variance of industrial production while stock returns explain only 9%. In an application that focuses on regulatory risk, [34] studies the effect of the Financial Regulation Policy Uncertainty (FRPU) index on the behavior of credit spreads together with other macroeconomic variables in both a linear SVAR framework and a nonlinear Smooth Transition VAR (STVAR) model. She finds that an increase in the FPRU index leads to an increase in the cost of external finance as well as persistent decline in real activity. Likewise, using an STVAR model, [35] study the effects of an unanticipated increase in economic policy uncertainty on unemployment in recessions and expansions in the United States. [36] use United States interest rate forecasts from Blue Chip Economic Indicators (BCEI) to construct an uncertainty proxy and examine the inter-relationships among monetary policy uncertainty, monetary policy expectations, core inflation, unemployment, and the federal funds rate in a time-varying VAR framework for the United States.
There are also empirical studies conducted in an emerging market economy context. Following the approach in [32], [11] infer a measure of global uncertainty as periods of spikes in the VXO index, which is the implied volatility on a synthetic 30-days option on the S&P 100 stock index.6 They examine the impact of their measure of uncertainty on a set of financial and real variables for emerging market economies by conducting an SVAR analysis. They find that an increase in uncertainty is associated with a much more severe decline in investment for a group of 20 emerging market economies which is also more persistent compared to a group of 20 developed economies. They also find a significant decline in consumption due to a rise in uncertainty measured in this way, in contrast to developed economies where consumption does not deviate significantly from its trend in response to such shocks. [3] estimate an SVAR model for Turkey which includes an EPU index, the real exchange rate, the real interest rate and one of three macroeconomic variables among real consumption expenditures, real investment expenditures and real GDP. They find that higher economic policy uncertainty leads to greater declines in investment compared to consumption or real GDP.
There is also an extensive literature that examines the interaction of economic policy uncertainty with financial market indicators. [9] show that the EPU index can help to predict the daily realized stock market volatility on the S&P 500 index both in-sample and out-of-sample for a sample period 1996–2013. [10] examine the effects of oil price shocks arising from oil supply-side shocks measured as changes in world oil production, aggregate demand shocks and oil-market specific shocks on the US EPU index and aggregate United States real stock returns. [37] study the predictability of the US EPU index using a basket of 23 commodity price changes. They show that commodity price changes can be considered as a leading indicator of US EPU index. This arises from the fact that one of the components used to create the US EPU index is given by forecast disagreement among professional forecasters, which becomes more uncertain during periods of oil price changes.
A number of studies have also examined the role of economic policy uncertainty induced by the Covid-19 crisis. [38] investigate multiple economic uncertainty indicators for the United States and United Kingdom before and during the COVID-19 pandemic. According to Choleski-identified VAR models they fit to monthly U.S. data, an uncertainty shock predicts record high drops in industrial production of 12–19% during the Covid-19 pandemic. [39] construct a monthly index for each state in the United States using digital archives of local newspapers. They show that the COVID-19 pandemic drove huge increases in policy uncertainty and unemployment, more so in states with stricter government-mandated lockdowns. Using NLP techniques, [40] develop an economic policy uncertainty index (EPU-NLP) for Canada and the United States. They also construct a so-called EPU-Boolean index following the approach in [1] and find that their EPU-NLP index leads to larger declines in real GDP, employment, industrial production and the TSX Composite index compared to the EPU-Boolean index for Canada over the period 2015–2020 that includes the Covid-19 crisis. Arbatli [41] show that the EPU index rises in Japan in reaction to financial crises, the consumption tax hikes, Brexit, and COVID-19.
3 The Twitter-Based Economic Policy Index
The need for the perception of opinions, attitudes, emotions, appraisals towards entities such as products, services, organizations, individuals, issues, events, and topics coincides with the rapid growth of social media platforms on the Web. Through multiple platforms including reviews, forum discussions, blogs, microblogs, Twitter, and social networks, we now access digital archives of opinionated data around the world [42].
The number of studies conducting sentiment analysis has proliferated in recent years. For example, [43] investigate public sentiment during the 2008, 2009 election by comparing the measures based on traditional presidential election/approval polls with those based on the text analysis of Twitter data. Sentiment analysis has been widely used in many different fields ranging from computer science, to management science, to health science, and to social sciences such as economics and finance. This increasing popularity of sentiment analysis is due to the fact that opinions shape almost all human activities and have a significant impact on our behaviour [44].
From the perspective of economic analysis, the way agents perceive events, policies and markets, make their choices, and set their expectations heavily depends on how others see and evaluate the world. Therefore, agents generally account for others’ opinions when making their decisions. For the same reason, households, firm managers and policymakers become attuned to expert opinion whenever they are uncertain about economic policy.
In this paper we construct a measure for economic policy uncertainty based on a select group of Twitter accounts whose tweets are considered to reflect expert opinion in the area of economic policy by the general public. In line with the related literature on social media interactions,7 we analyze the interaction of the Twitter accounts of economic policy experts with other accounts in Twitter through the number of their followers. The select group of Twitter users consists of ministers, parliamentarians, academicians, bureaucrats, journalists, and private sector professionals that are considered to have capability to provide expert opinion on economic policy. The assessment of economic policy uncertainty by these selected experts is similar, in some ways, to the assessment of risk and uncertainty by the US economists questioned in the highly regarded Survey of Professional Forecasters that is conducted by the Federal Reserve Bank of Philadelphia.8
With the increased capacity of Twitter to provide up-to-date documentary on world events, it is inevitably getting harder to extract useful information from the bulk data generated daily by users.9 As mentioned in the Introduction, our paper differs from existing studies that use bulk Twitter data. Instead we implement a user list curation process. This alternative approach allows us to restrict our analysis to a select group of Twitter accounts. By doing so, we are able to update our TEPU index and to capture the daily developments in the Turkish economy in a timely manner. To verify that our TEPU index does not suffer from any bias that might potentially arise from the above-mentioned selection of Twitter accounts, we use a bootstrapping algorithm that we run repetitively with different seed lists, which results in the same final candidate list in all repetitions.
3.1 The Selection of Twitter Users
In the related literature researchers use several different methods to identify whether the content is about the “economy,” “policy” and/or “uncertainty” to construct a measure of economic policy uncertainty. Among others, for example [5] extract all tweets from Twitter API data that contains the words “uncertain,” “uncertainly,” “uncertainties,” “uncertainty.“, whereas [40] use NLP methods to determine content. In our paper, we implement a user list curation process that closely follows [45, 46]. This provides us with three advantages: First, it enables us to remove tweets with only a weak impact on public opinion and eliminate the need for scaling noisy data created by bulk retrieval methods.10 Second, it prevents errors arising from the Turkish language applications of NLP methods. Third, it presents a novel approach to the subject of content-based EPU indexing.
Our bootstrapping algorithm consists of three distinct list types:
Seed list: The initial list is used to initialize the process, serving as a nucleus in the bootstrapping algorithm [45]. This list is not called first, second etc. as it is only used to initialize the process.
Recommended List: This list is prepared to be annotated, purified from the users in the seed list and the recommended list formed in the previous phase. These lists are named according to which phase they are formed, first, second, etc.
Candidate List: This list is comprised of experts in economics and finance, according to the annotation results of the recommended list formed in the previous phase. These lists are named according to which phase they are formed, second, final, etc.
As illustrated in Figure 2 we implement three phases to obtain the final candidate list of Twitter accounts whose tweets are used to construct our TEPU index. In what follows, we provide the details of these phases:
(i) to hold a PhD degree in economics or finance
(ii) to possess the highest number of followers (1 million plus).12
To validate our approach, we implement the above-mentioned phases using the following alternative seed lists: 1) the Twitter accounts that are followed by 16 Twitter accounts who have more than 500,000 followers, and 2) the Twitter accounts that are followed by 7 randomly selected Twitter accounts out of the 16 Twitter accounts who have more than 500,000 followers. Both seed lists yield two final lists of users that are identical to the final candidate list of 661 users obtained using the reference seed list.
Table 1 presents descriptive statistics of the selected Twitter user accounts categorized by occupation.15 This table reports the number of followers, followings and tweets, and the registration date corresponding to each occupation. These statistics show that in the sample period 2013–2021, on average, parliamentarians and bureaucrats are the most-followed users whereas they don’t follow people as much as users in other three categories do. Further, on average, experts in all these four categories post at least around 10,000 tweets, suggesting that they seem to actively use the Twitter platform for transmitting their opinions to the public in our sample period. In particular, starting with the seed list of three Twitter user accounts belonging to the most-followed experts in the field of economics and finance in the Turkish community, we obtain a network of users that not only capture the influence of these people on public opinion but also their interaction with other users that they follow.
3.2 Constructing the Daily TEPU Index
To construct the TEPU index, we focus on the sample period from 1 January 2013 to 22 December 2021 because this period coincides with the onset of many events associated with political and economic uncertainty in Turkey.
We use the same search procedure developed by [1]. We treat the Twitter data-based series as if they are extracted from a single newspaper. We use the Twitter API to obtain all of the “following” lists of users. Using the web scraping with Python, we extract the full tweet content that the users posted within the specified time interval. We exclude retweets in the calculation of our TEPU index to avoid duplicates. We transform the letters in the tweets into lower case and use English characters throughout.
[1] use a list of words that captures the policy uncertainty characteristics of advanced economies. However, in our search process, we include the set of words that are considered more relevant for emerging market economies as in [6] and [2]. Table 2 shows the Turkish and English versions of the words that we search for. In each tweet posted by these selected 661 Twitter accounts in the period 2013–2021, we search for keywords and record the word count data. We normalize the raw word count data using the standardization procedure outlined in [1]. In particular, we first count the keywords at the daily frequency.16 Second, we compute the value
4 The TEPU Index and Financial Markets
4.1 Data
In addition to the daily TEPU index, we make use of daily data on the selected financial indicators that we use to track developments in Turkish financial markets over the period 2013–2021.
First, we use the Chicago Board Option Exchange (CBOE) Volatility Index (VIX) to proxy for global risk perceptions. The VIX is a real-time index that represents the market’s expectation of near-term price changes on the S&P 500 Index (SPX). It is measured as the implied volatility on SPX options, which provides a way to gauge market sentiment, and in particular, the degree of fear among market participants. We obtain the end-of-day VIX values from the Federal Reserve Bank of St Louis Economic Database (FRED). The Credit Default Swap (CDS) spread is an indicator that measures the risk of default on Turkey’s sovereign debt. Equivalently, the CDS spread represents the annual premium (the CDS “fee” or “spread”) to be paid to the lender against the risk of default. If the number of years of the loan is not specified in the contract, calculations are made according to the 5-years CDS Spread. The Bloomberg Terminal provides the daily 5-years CDS spread series, which we measure as basis points on US dollar-denominated CDS contracts. The spot USD/TRY exchange rate and the BIST-100 index are obtained from Yahoo! Finance. The first represents units of the domestic currency, namely the Turkish lira, per US dollar. The latter measures the performance of the top 100 stocks traded on the Istanbul Stock Exchange entitled as Borsa Istanbul.17
The daily series are reported as opening, closing, high and low values. In our analysis we use their closing values. We aggregate the resulting daily series into weekly series to be used in our analysis over the period 2013w1 and 2021w51.
4.2 Event Analysis
In what follows, we conduct an event analysis to show how our TEPU index relates to important political and economic events that have occurred in Turkey in the sample period 2013–2021.
Table 3 records both domestic and foreign events that may have been associated with changes in economic policy uncertainty in Turkey. It considers the timeline of events by making a distinction between political announcements versus the immediate reaction of financial markets. This table indicates episodes of political uncertainty in the weeks or months preceding such events as national elections or referendums whereas it indicates the exact date associated with monetary policy announcements and surprises. As a visual device, Figure 3 plots the daily TEPU index from 1 January 2013 to 22 December 2021 and tracks the evolution of economic policy uncertainty for Turkey. Some of the events associated with spikes in economic policy uncertainty have already been documented by earlier papers [3,4].18 In common with these papers, we find that beginning in 2013, some of the most significant events refer to the occurrence of the Gezi Protests, which erupted due to a disagreement regarding the usage of a municipal park and turned into nation-wide protests against the government, as well as the revelation of corruption allegations against various members of the government during 17–25 December 2013. The TEPU index rises before significant political events such as the national elections which were held on 24 June 2015 and re-scheduled for 1 November 2015. The TEPU index spikes during the failed coup attempt of 15 July 2016. There is also evidence of a general increase in uncertainty in the periods preceding the presidential referendum of 16 April 2017 as well as in the few weeks immediately preceding the elections under the new presidential system that took place on 24 June 2018.
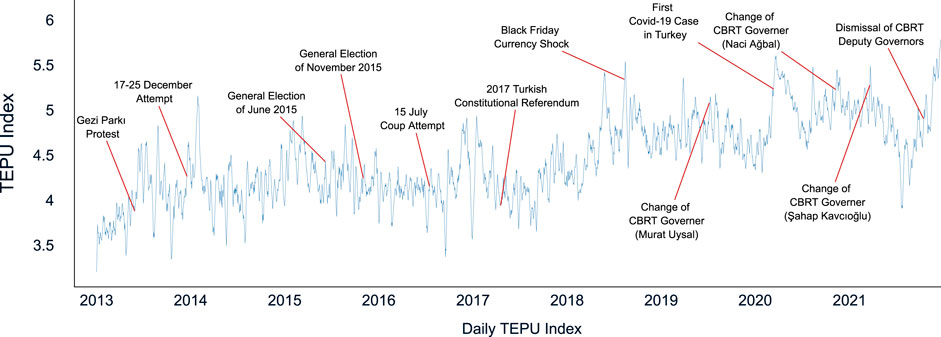
FIGURE 3. The daily TEPU index. This figure plots 7-day moving average of logarithm of TEPU index from January 1, 2013 to December 22, 2021. We provide the details on the construction of this index in Section 3.
As a further way of illustrating the impact of economic policy uncertainty for Turkey, Figure 4 considers two measures of risk assessment by foreign and domestic agents together with our TEPU index. This figure displays the behavior of the TEPU index together with two other indicators that summarize the risk perceptions of foreign investors, namely, the VIX, as well as a measure of country risk premium for Turkey, namely, its CDS spread, over the entire sample period from January 2013 to December 2021. As a significant regularity, we note that the TEPU index moves in tandem with both of these indicators. The TEPU index and the CDS spread, in particular, display a tendency to increase together especially over the period since 2018. One of the major events in 2018 is the Black Friday currency shock and the CBRT’s subsequent decision to increase its policy rate by 625 basis points. As Figure 4 shows, this event is captured by the increase in both the TEPU index and the CDS spread for Turkey in August 2018. Following a sharp depreciation in the Turkish lira from 4 TRY per USD to 7 TRY per USD, the Turkish lira recovers after the large policy rate increase by the CBRT. In July 2019 there is an increase in Turkey’s CDS spread concurrently with a spike in the TEPU index that occurs in response to the replacement of Governor Murat Çetinkaya by Governor Murat Uysal.
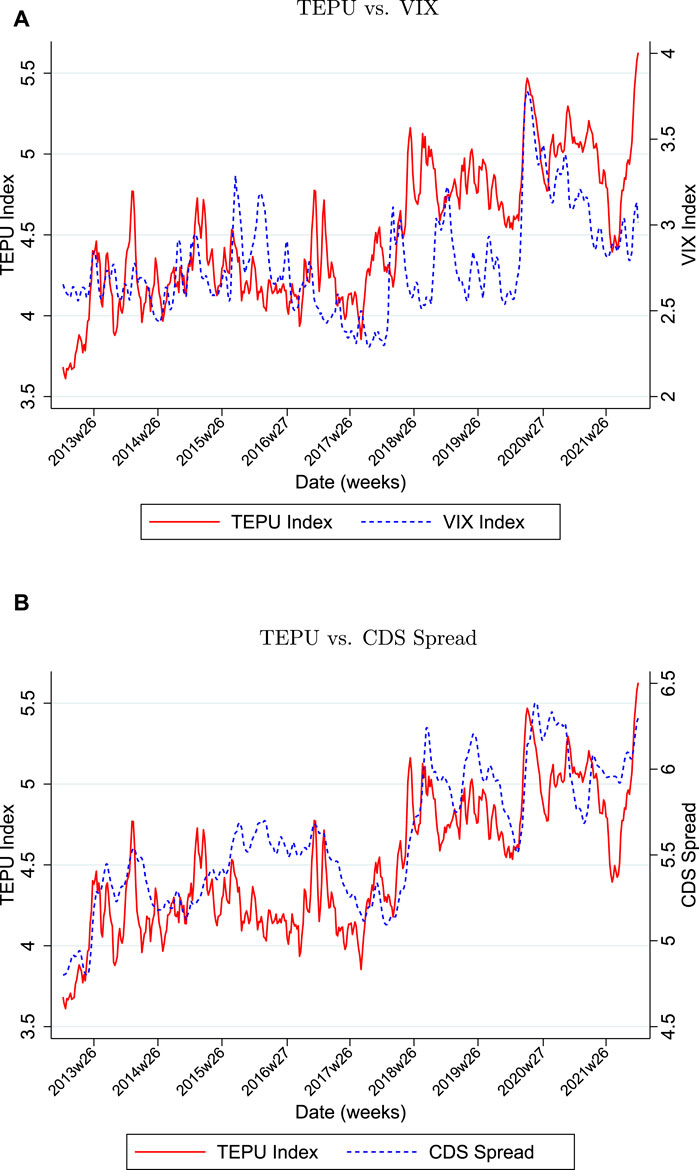
FIGURE 4. The comparison of the TEPU index versus VIX and CDS spread. (A) TEPU vs. VIX (B) TEPU vs. CDS Spread. This figure plots 4-week moving average of the logarithm of the TEPU index against the logarithms of VIX and CDS spread in sub-panels (a)–(b), respectively. See Section 4.1 for further details. Sample period: 2013w1-2021w51.
In March 2020, we observe a large increase in the VIX in response to the onset of the Covid-19 crisis, which is also reflected in a significant increase in Turkey’s CDS spread. As it is well-known, the VIX captures changes in the risk perceptions of foreign investors due to exogenous shocks, external monetary and financial conditions, and developments specific to emerging market economies including the Turkish economy. In this regard, the correlation between the VIX and the TEPU index partially reflects the fact that the Turkish economy is vulnerable to episodes of divergence between its policy actions relative those of the US, as argued by Kalemli-Özcan [48]. This is due to its dependence on international capital flows for financing its current account deficit. By contrast, Turkey’s CDS spread tends to capture a systematic assessment of the uncertainty and risk premium associated with long-term prospects for the Turkish economy. This is evidenced by its sharp rise around the August 2018 currency shock as well as the Covid-19 shock, which tends to persist until the end of 2020. These movements are accompanied by significant increases in the TEPU index as well.
Uncertainty about policy changes as measured by the TEPU index rises sharply in March 2021 when Governor Naci Ağbal, a central official known for his orthodox views on monetary policy, is replaced as governor of the CBRT by Governor Şahap Kavcıoğlu. In response to this event, the daily value of the USD/TRY exchange rate jumps from 7.305 TRY per USD to 8.0954 TRY per USD, indicating a 10% depreciation of the Turkish lira. Turkish bond and stock markets also witness their largest capital outflow of $1.9 billion since 2006.19 As a consequence, the BIST-index falls by around 8% on 21 March 2021, the day following the dismissal of Governor Naci Ağbal. These developments are also reflected in an increase in Turkey’s CDS spread and a smaller increase in the VIX in March 2021.
After September 2021, the effects of the unconventional monetary policy being espoused in Turkey begin to manifest themselves. As Table 3 shows, consecutive decreases in the CBRT’s policy rate are implemented between 23 September 2021 to 16 December 2021. During this period, the CBRT MPC reduces its policy rate by 500 basis points, the last cut of 100 basis points occurring after a MPC meeting on 16 December 2021. These changes lead to an unprecedented increase in the TEPU index. Turkey’s CDS spread shows a tendency to increase rapidly with the TEPU index, rising from a value of around 388 up until the second week of September 2021 to 578 by 17 December 2021.
During this period, Turkey’s exchange rate also follows a highly volatile pattern, with the value of the Turkish lira falling by around 120% against the US dollar in a spiraling fashion until the end of December 2021 when the introduction of new foreign-exchange linked saving schemes help to stem the rout. The volatility in the foreign exchange rate market is also accompanied by volatility in Turkey’s stock market, as trading is suspended twice on all listed stocks as the BIST-100 index falls by more than 7%. Countervailing this effect, stock prices nevertheless recover as investors in Turkey seek to take advantage of the price discount on stocks in Turkish lira to protect their savings.20
5 Econometric Methodology
In this section, we consider the dynamic inter-relationships among fluctuations in the TEPU index and a set of key financial indicators for Turkey, including the CDS spread, the BIST-100 stock index and the USD/TRY exchange rate, for the sample period comprising 1 January 2013 to 22 December 2021. In this analysis, we do not seek to jointly model the dynamics of the VIX with the remaining variables, instead considering its lagged values as exogenous control variables.21
Consider a standard VAR of the form
where
In our analysis, we impose a recursive ordering to identify the structural shocks in the estimated SVAR model. In the literature, alternative indices of economic policy uncertainty have typically been placed first based on the notion that shocks to this variable have a contemporaneous impact on the remaining variables while none of the other variables are assumed to have a contemporaneous impact on it. In our analysis, we place the CDS spread first to allow for possible contemporaneous effects from it to affect the TEPU index and the remaining variables. Likewise, each of the remaining variables will have a contemporaneous effect on the variables listed after them but will not affected by them contemporaneously.
5.1 Estimates of the SVAR Model
To estimate the SVAR model, we first test the different series for unit roots based on the Augmented Dickey-Fuller (ADF) test. We cannot reject the null hypothesis of a unit root for the USD/TRY exchange rate and the BIST-100 stock index, suggesting that they are non-stationary. On the other hand, the TEPU index, the VIX and the CDS spread are shown to be trend-stationary.
We use the HP-filter to remove time-varying trends in the different series. While implementing the HP-filter has been criticized as being ad hoc and for inducing spurious dynamics into the series to which it is applied [49], the so-called Hamilton regression filter which uses the residuals from an
In what follows, we estimate an SVAR model with three lags of the endogenous variables and 4 lags of the VIX. Our choice is based on the AIC, while selection criteria such as the SBIC and HQIC which favor more parsimonious specifications choose a lag length of 2. Based on diagnostic tests for the behavior of the SVAR model, we find that evidence of stability in the conditional mean equations in that all of the estimated eigenvalues are inside the unit circle. This suggests that the system we are estimating is stable. Second, the Lagrange Multiplier test for autocorrelation [53] suggests that the absence of serial correlation in the residuals of the SVAR model for almost all lags up to lag 24.23
In our analysis, we make use of the SVAR model to conduct Granger causality tests to understand the dynamic inter-relationships among the different series. Granger causality tests provide a way for examining the predictive power of one variable
Table 4 shows the results of Granger causality tests among expert opinion represented by the TEPU index and Turkey’s financial indicators for three different sample periods. These results show that the TEPU index and financial indicators such the USD/TRY exchange rate and the BIST-100 stock index interact with and influence each other at the weekly frequency. This may have to do with their high-frequency movements in a shallow financial market where news gets transmitted rapidly to economic agents and markets. While lagged values of fluctuations in the exchange rate help to predict both fluctuations in the stock market index and the TEPU index, changes in the TEPU index also help to predict the changes in the exchange rate and in the stock index. These results hold, on the whole, for the three samples that we consider, though they are weaker for the samples ending in 2019 and 2017. The bi-directional relationship between the USD/TRY exchange rate and the TEPU index is among the strongest and holds for all three sample periods. This may occur if fluctuations in the USD/TRY exchange rate transmit signals about monetary policy announcements and other unanticipated policy changes to economic agents, which are then incorporated into expert opinion. On the other hand, changes in expert opinion represented by the TEPU index may also influence the exchange rate through the social media activities of economic experts.
Table 4 also provides information about the determinants of changes in Turkey’s CDS spread. As it is well-known, the CDS spread tends to capture a systematic assessment of the uncertainty and risk premium associated with long-term prospects for a given country. Table 4 shows that changes in the TEPU index emerges as the key predictor for changes in the CDS spread for the samples ending in 2021 and 2019. In a related analysis, [8] create a real-time News-implied Sovereign Risk Index (NSRI) for over 100 countries by applying text search methods to socioeconomic, political and financial news.25 They show that lagged changes in this index are a significant predictor of changes in a country’s CDS spread, especially at the monthly frequency, after controlling for the effects of other domestic and global macro-economic variables. Thus, as in [8], changes in expert opinion about economic policy issues help to predict movements in the CDS spread. Nevertheless, there are some differences between the approach followed by these authors and ours. While [8] implement a panel data analysis based on a bi-variate relation between the CDS spread and their news-based measure of sovereign risk, we find that dynamic inter-relationships among changes in expert opinion and other financial indicators help to determine changes in Turkey’s country risk premium based on a multivariate analysis. This further provides evidence about the interactive relationship between expert opinion represented by the TEPU index and the different financial indicators in our analysis.26
We can relate our results to the literature that studies the interaction of economic policy uncertainty with other financial indicators. Using monthly data, [10] argue that oil prices and economic policy uncertainty are inter-related and influence both stock returns and stock market volatility in that a positive oil-market specific shock raises economic policy uncertainty and reduces real stocks returns. Thus, the endogenous response of economic policy uncertainty amplifies the direct effects of oil price shocks. Likewise, [8] find that spikes in their NSRI “are negatively associated with same-week market returns, which reverses over the next week, indicating that investors might overreact to default risk news.” They further find that the reaction of equity markets in countries that face binding fiscal constraints is more pronounced and persistent. In our analysis, we find that the CDS spread helps predict fluctuations in equity markets. This may occur if expectations about the future of the Turkish economy and hence, its country risk premium, signify changes in the profitability of investing in Turkey.27
5.2 Volatility Dynamics
As we discussed in Section 4, there has been considerable volatility in the sample period that we are analyzing. To make this notion precise, Table 5 provides estimates of a GARCH(1,1) model specified as
To capture such conditional heteroscedasticity in the residuals, we estimate an M-GARCH model over the main sample period. In this specification, the error terms of the SVAR satisfy
where
The dynamics of the M-GARCH model embodied in the matrix
where
In the estimation of the M-GARCH model, we model the conditional volatility in the different series by considering ARCH processes for the conditional variances and covariances that depend only on the second moments of the past shocks in the relevant equation as
Figure 5 displays the predicted values of the conditional variances for the main sample period from 1 January 2013 to 22 December 2021. From Panel (a) of this figure, we observe volatility clusters in the behavior of the conditional volatility of the CDS spread, which reflects the events that we catalogued in Section 4.2. In particular, the Black Friday shock of August 2018, the Covid-19 shock as well as the impacts of the unconventional monetary policy being increasingly pursued during 2020 and 2021 register as increases in the conditional volatility of the CDS spread. For the BIST-100 index, the major source of volatility is the Covid-19 shock of March 2020. By contrast, the August 2018 foreign exchange shock registers as the most important source of volatility for the conditional variance of the USD/TRY exchange rate. The December 2021 exchange rate shock also appears as an important shock to this conditional variance. However, the overall effect of the December 2021 shock on the USD/TRY exchange rate turns out to be smaller compared to the Black Friday shock of August 2018 due to the fact that the time-varying trend in the exchange rate has been increasing over the sample period.
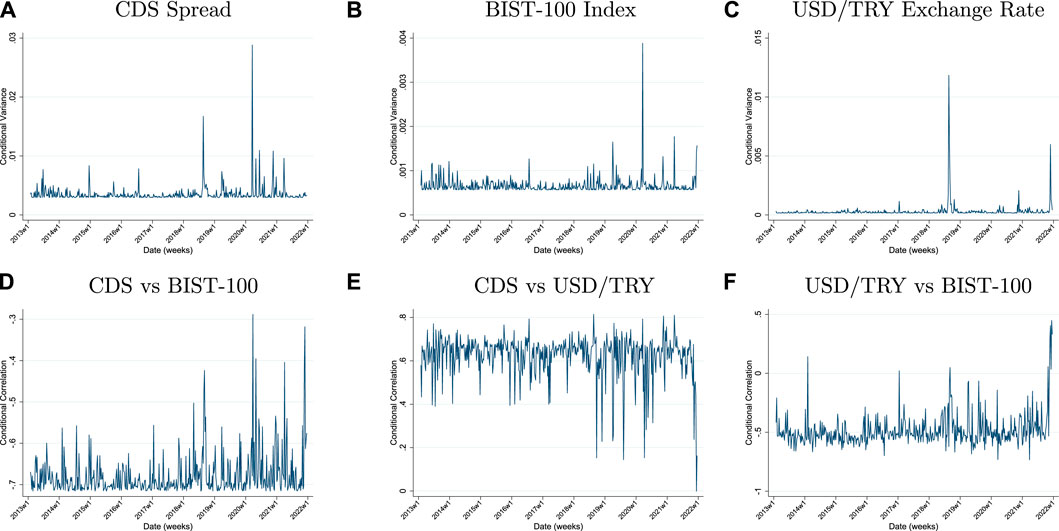
FIGURE 5. Conditional variances and correlations. (A) CDS Spread. (B)BIST-100 Index. (C) USD/TRY Exchange Rate. (D) CDS vs BIST-100. (E) CDS vs USD/TRY. (F) USD/TRY vs BIST-100. Sample covers the period 2013w1-2021w51.
Put differently, this discrepancy in the relative magnitudes of the two above-mentioned volatility shocks might stem from differences in their nature. We may view the Black Friday shock of August 2018 as being exogenous in the sense that it was partly the result of political tensions between the Trump administration and the government of Turkey. Hence, market participants did not anticipate it beforehand and failed to revise their expectations of its effect on the Turkish lira. By contrast, the December 2021 exchange rate shock seems to be endogenous in that it occurred in an environment where the pursuit of unconventional monetary policies was increasingly being espoused by different government officials. As the repeated interest rate cuts eroded the value of the Turkish lira throughout the last 3 months of 2021, the 20 December 2021 shock, though large in absolute magnitude, was most likely incorporated into the expectations of market participants as another shock against a backdrop of deteriorating sentiment regarding the value of Turkey’s national currency.
Another way to examine the volatility behavior of changes in the TEPU index and the remaining financial indicators is to examine their conditional covariance. To ensure that the conditional covariance is not dominated by the estimates of the conditional variances, we calculate the conditional correlation between changes in the exchange rate and the CDS spread. The conditional correlations are defined as
6 Conclusion
In this paper, we construct a Twitter-based high frequency economic policy uncertainty index for Turkey over the period 2013–2021 by using a select set of Twitter accounts whose tweets are considered to reflect expert opinion on economic policy issues in Turkey. This index may be used to track the evolution of economic policy uncertainty for a key emerging market economy, namely, Turkey. The timely character of Twitter data enables us to update our forward-looking TEPU index to follow rapidly changing movements in financial markets through changes in expert opinion and to understand how expert opinion itself is affected by financial indicators in a volatile policy environment.
As in other related research [8], expert opinion as represented by the TEPU index is a key indicator that helps to predict the country risk premium measured by the CDS spread. We further find evidence that the CDS spread itself may be useful for predicting changes on equity markets in Turkey. These two findings suggest a close connection between expert opinion, measures of the domestic risk premium and profit opportunities in an emerging market economy context. Finally, as an additional contribution, we relate the sources of conditional volatility in the CDS spread together with the USD/TRY exchange rate and BIST-100 stock index to salient events that occurred over the sample period 2013–2021.
That economic policy uncertainty stemming from such policy changes will have deleterious effects on investment by private firms in key emerging market economies is well-established; for a recent example, see [56] who aims to quantify the impact of heightened uncertainty associated with the sanctions regime of 2014 on investment behavior by non-financial privately-held firms in Russia. By analyzing a current episode of policy uncertainty that arises from political and economic events in Turkey, we provide a road-map for understanding the effects of economic policy uncertainty for other key emerging market economies which are historically prone to uncertainty and volatility.
Data Availability Statement
The raw data supporting the conclusion of this article will be made available by the authors, without undue reservation.
Author Contributions
All authors listed have made a substantial, direct, and intellectual contribution to the work and approved it for publication.
Conflict of Interest
The authors declare that the research was conducted in the absence of any commercial or financial relationships that could be construed as a potential conflict of interest.
Publisher’s Note
All claims expressed in this article are solely those of the authors and do not necessarily represent those of their affiliated organizations, or those of the publisher, the editors and the reviewers. Any product that may be evaluated in this article, or claim that may be made by its manufacturer, is not guaranteed or endorsed by the publisher.
Acknowledgments
This research is supported by TUBITAK Grant No: 118K268. We are grateful to Cem Çakmaklı for helpful comments. We thank Elif Güneş for her superb research assistance
Footnotes
1https://www.ft.com/content/f6c3f33f-356c-47b8-8156-5fe07ffe6237?shareType=nongift.
2http://www.aljazeera.com/economy/2021/12/17/turkey-stock-trading-halted-twice-as-lira-crashes-to-new-low.
3In contrast to the Twitter API which has rate limits (see https://developer.twitter.com/en/docs/twitter-api/rate-limitslink), the web scraping method that we use is faster in the extraction of data.
4See Bloom [57] for a detailed survey on how uncertainty affects economic activity.
5The authors of Baker et al. [1] develop indices of economic policy uncertainty for countries around the world. In particular, as of March 2022, the most up-to-date EPU indices are provided for 29 countries in their website: https://www.policyuncertainty.com/all_country_data.html.
6They define these events as periods in which the Hodrick–Prescott de-trended VXO series exceeds its mean by more than 1.65 standard deviations. In this way, they obtain six events over the sample period 1990–2010 and generate a measure of uncertainty by weighing these events with the magnitude of the volatility shock.
7Using three measures of influence including in-degree, retweets and mentions, Cha, Haddadi, Benevenuto and Gummadi [58] investigate the dynamics of user influence across topics and time based on these notions. One of their findings shows that influence is typically gained through concerted effort such as limiting tweets to a single topic. Verweij [59] studies the interactions between a group of Dutch journalists and politicians and show that contacts on Twitter are driven by the need for finding and spreading news, as opposed to religious or ideological identity of parties and media.
8The European Central Bank conducts the same survey to obtain the forecasts of the economists located in Euro Area. See https://www.philadelphiafed.org/surveys-and-data/real-time-data-research/survey-of-professional-forecasters and https://www.ecb.europa.eu/stats/ecb_surveys/survey_of_professional_forecasters/html/index.en.html for further details on these surveys.
9Turkey has 16.25 million active Twitter users, ranking as the seventh country in the world as of October 2021, see https://www.statista.com/statistics/242606/number-of-active-twitter-users-in-selected-countries/.
10Likewise, Storyful, a news and intelligence agency, devotes significant time to manually curate content on social networks such as Twitter and YouTube with the aim of filtering news, or newsworthy content, from the vast quantities of noisy data on these platforms.
11Due to the nature of the bootstrapping algorithm, it is immaterial how many people are included in the initial list. It may differ according to the country one focuses on and the selection criteria that is implemented. In our case, we want to restrict our initial list to a select set of people who are expected to be the most influential on public sentiment. This way of identification of influential experts builds on the insights of Weng, Lim, Jiang and He [60] where an influential twitterer is defined as one with certain authority within her social network. To this end, we start with an initial list that comprises of Twitter accounts of three experts in the field of economics and finance that have the highest number of followers.
12In addition to this “in-degree” measure of user influence, the two of these three experts are ranked as the first two twitterers in the Turkish community in 2021 in terms of number of retweets, see https://twitter.com/adbaanalytics/status/148704504764429926 for further statistics released by Adba Analytics, a digital consumer research agency.
13This restriction goes in line with the “homophily” phenomenon. The analysis of Weng et al. [60] shows that “homophily” does exist in Twitter data, implying that the “following” relationships between twitterers are related to their topical similarity.
14Similarly, Saif, Fernandez, He and Alani [61] construct a data set, namely, the Stanford Twitter Sentiment Gold (STS-Gold) that has been used for Twitter Sentiment Analysis. This data set contains 2,034 tweets which are automatically annotated with sentiment labels. This data set also contains 58 entities manually annotated by three different human annotators.
15In the final candidate list of 661 users, 4 users are identified as both academician and parliamentarian/bureaucrat based on their personal information reported in their Twitter accounts.
16To correct for intra-week variation in the number of tweets, we scale the daily TEPU index by the average number of tweets for each day of the week during the year 2017.
17According to the 2020/26 numbered notification of Borsa Istanbul published on 4 April 2020, especially from BIST-30 and BIST-100, two zeros were removed from the BIST Equity Indexes as of Monday 27 July 2020 and the index value has continued to be displayed as two digits after the comma. In order to have a consistent format in these series, we divide the values before 27 July 2020 by 100.
18For the period 2000–2018, Topçu and Oran [4] find that most of the uncertainty in Turkey over the period 2000–2018 arises from events that trigger political uncertainties such as elections and the changes in policy that these may induce.
19http://www.bloomberg.com/news/articles/2021-04-01/turkey-s-central-bank-revamp-spurs-biggest-outflows-in-15-years.
20http://www.reuters.com/markets/stocks/turkish-stocks-open-down-after-sharp-friday-selloff-2021-12-20/.
21To the extent that the variables in our study reflect the domestic dynamics of financial markets in Turkey, especially at the weekly frequency, inclusion of the VIX as an endogenous variable is unlikely to yield information about its comovement with the remaining variables.
22The boosting involves applying the HP-filter multiple times based on stopping criteria from a machine-learning algorithm.
23We include 4 lags of the VIX, as it helps to reduce the autocorrelation in the residuals from the estimated SVAR.
24In this definition,
25They implement machine learning algorithms such as topic modelling and text similarity to identify sovereign default risk concerns in newspaper articles. They consider the world stock market index from MSCI, the US EPU index from Baker et al. [1], the US macroeconomic activity index, namely Aruoba-Diebold-Scotti (ADS) of Aruoba, Diebold and Scotti [62] from the Federal Reserve Bank of Philadelphia, and the VIX as controls.
26We also create Impulse Response Functions (IRFs) to trace the dynamic response of the different series to each of the structural innovations. These IRFs show that a one-standard deviation shock to the TEPU index tends to increase the CDS spread and the USD/TRY exchange rate while a similar shock to the USD/TRY exchange rate increases the TEPU index. Finally, contemporaneous changes in the CDS spread are reflected in declines in the BIST-100 index and increases in the USD/TRY exchange rate and the TEPU index. These results are available upon request.
27In our analysis, we use the nominal values of the USD/TRY exchange rate and the BIST-100 index. To check the robustness of our results, we also create real values of the USD/TRY exchange rate and the BIST-100 index over our sample period. However, the price level series required to convert nominal values to real values is available only at the monthly frequency. Hence, we divide the (levels) of the nominal series by the monthly series on Consumer Price Index (2003 = 100) released by the Turkish Statistical Agency (TURKSTAT); see https://data.tuik.gov.tr/Kategori/GetKategori?p=Enflasyon-ve-Fiyat-106. The correlations between the real and nominal monthly series for HP-filtered versions of each of the two financial indicators - the stock index and the exchange rate - are both around 0.98, suggesting that any inflationary bias induced in the relevant nominal series is filtered out through the time-varying trend created by the HP-filter.
28If we allow for a
29This yields the DVECH model studied by Bollerslev, Engle and Wooldridge [63]. Other versions of the M-GARCH model are considered by Baba, Engle, Kraft and Kroner [64].
30An asymmetric version of the M-GARCH model is used by de Goeij and Marquering [65] to analyse dynamics for the conditional variances and covariances of bond and stock returns for the US.
References
1. Baker SR, Bloom N, Davis SJ. Measuring Economic Policy Uncertainty*. Q J Econ (2016) 131(4):1593–636. doi:10.1093/qje/qjw024
2. Jirasavetakul L-BF, Spilimbergo A. Economic Policy Uncertainty in Turkey. IMF Working Papers 18/272, International Monetary Fund; Washington, DC (2018).
3. Sahinoz S, Erdogan Cosar E. Economic Policy Uncertainty and Economic Activity in Turkey. Appl Econ Lett (2018) 25(21):1517–20. doi:10.1080/13504851.2018.1430321
4. Topçu G, Oran J. Measuring Economic Policy Uncertainty in Turkey. Ijepee (2021) 14(3):288–305. doi:10.1504/ijepee.2021.114962
5. Baker SR, Bloom N, Renault T. Twitter-Derived Measures of Economic Uncertainty (2021). Available at: https://www.policyuncertainty.com/media/Twitter Uncertainty 5 13 2021.pdf.
6. Becerra JS, Sagner A. Twitter-Based Economic Policy Uncertainty Index for Chile. Technical Report 883. Santiago, Chile: Working Papers of the Central Bank of Chile (2020).
7. Laurent S, Bauwens L, Rombouts J. Multivariate GARCH Models: A Survey. J Appl Econom (2006) 21(1):79–109.
8. Dim C, Koerner K, Wolski M, Swart S. News-based Sovereign Default Risk. European Investment Bank Working Paper 2022/06 (2022). doi:10.2867/661002
9. Liu L, Zhang T. Economic Policy Uncertainty and Stock Market Volatility. Finance Res Lett (2015) 15(C):99–105. doi:10.1016/j.frl.2015.08.009
10. Kang W, Ratti RA. Oil Shocks, Policy Uncertainty and Stock Market Return. J Int Financial Markets, Institutions Money (2013) 26(C):305–18. doi:10.1016/j.intfin.2013.07.001
11. Carrière-Swallow Y, Céspedes LF. The Impact of Uncertainty Shocks in Emerging Economies. J Int Econ (2013) 90(2):316–25. doi:10.1016/j.jinteco.2013.03.003
12. Eberly JC. Adjustment of Consumers' Durables Stocks: Evidence from Automobile Purchases. J Polit Economy (1994) 102(3):403–36. doi:10.1086/261940
13. Bernanke BS. Irreversibility, Uncertainty, and Cyclical Investment. Q J Econ (1983) 98(1):85–106. doi:10.2307/1885568
14. Dixit A. Entry and Exit Decisions under Uncertainty. J Polit Economy (1989) 97(3):620–38. doi:10.1086/261619
15. Dixit RK, Dixit AK, Pindyck RS. Investment under Uncertainty. Princeton, NJ: Princeton University Press (1994).
16. Schaal E. Uncertainty and Unemployment. Econometrica (2017) 85(6):1675–721. doi:10.3982/ecta10557
17. Valletta RG, Bengali L, Van der List C. Cyclical and Market Determinants of Involuntary Part-Time Employment. J Labor Econ (2020) 38(1):67–93. doi:10.1086/704496
18. Fernández-Villaverde J, Guerrón-Quintana P, Kuester K, Rubio-Ramírez J. Fiscal Volatility Shocks and Economic Activity. Am Econ Rev (2015) 105(11):3352–84. doi:10.1257/aer.20121236
19. Johannsen BK. When Are the Effects of Fiscal Policy Uncertainty Large? In: Finance and Economics Discussion Series 2014-40. Washington, DC: Board of Governors of the Federal Reserve System (U.S.) (2014).
20. Kraft H, Schwartz E, Weiss F. Growth Options and Firm Valuation. Eur Financial Manage (2018) 24(2):209–38. doi:10.1111/eufm.12141
21. Christiano LJ, Motto R, Rostagno M. Risk Shocks. Am Econ Rev (2014) 104(1):27–65. doi:10.1257/aer.104.1.27
22. Gilchrist S, Sim JW, Zakrajšek E. Uncertainty, Financial Frictions, and Investment Dynamics. NBER Working Paper w20038. Cambridge, MA: National Bureau of Economic Research (2014).
23. Pástor Ľ, Veronesi P. Political Uncertainty and Risk Premia. J Financial Econ (2013) 110(3):520–45. doi:10.1016/j.jfineco.2013.08.007
25. Rodrik D. Policy Uncertainty and Private Investment in Developing Countries. J Develop Econ (1991) 36(2):229–42. doi:10.1016/0304-3878(91)90034-s
26. Higgs R. Regime Uncertainty: Why the Great Depression Lasted So Long and Why Prosperity Resumed after the War. Independent Rev (1997) 1(4):561.
27. Hassett KA, Metcalf GE. Investment with Uncertain Tax Policy: Does Random Tax Policy Discourage Investment. Econ J (1999) 109(457):372–93. doi:10.1111/1468-0297.00453
28. Altug S, Demers FS, Demers M, Demers M. The Investment Tax Credit and Irreversible Investment. J Macroeconomics (2009) 31(4):509–22. doi:10.1016/j.jmacro.2009.01.001
29. Altig D, Bloom N, Davis SJ, Meyer B, Parker N. Tariff Worries and US Business Investment, Take Two. Federal Reserve Bank of Atlanta Macro Blog Post. Atlanta, GA: Policy HUB (2019).
30. Caldara D, Iacoviello M, Molligo P, Prestipino A, Raffo A. The Economic Effects of Trade Policy Uncertainty. J Monetary Econ (2020) 109:38–59. doi:10.1016/j.jmoneco.2019.11.002
31. Ahir H, Bloom N, Furceri D. The World Uncertainty Index. Stanford University (2018). Available at SSRN: https://ssrn.com/abstract=3275033 (Accessed October 29, 2018).
33. Jurado K, Ludvigson SC, Ng S. Measuring Uncertainty. Am Econ Rev (2015) 105(3):1177–216. doi:10.1257/aer.20131193
34. Nodari G. Financial Regulation Policy Uncertainty and Credit Spreads in the US. J Macroeconomics (2014) 41:122–32. doi:10.1016/j.jmacro.2014.05.006
35. Caggiano G, Castelnuovo E, Figueres JM. Economic Policy Uncertainty and Unemployment in the United States: A Nonlinear Approach. Econ Lett (2017) 151(C):31–4. doi:10.1016/j.econlet.2016.12.002
36. Doehr R, Martínez-García E. Monetary Policy Uncertainty and Economic Fluctuations at the Zero Lower Bound. Globalization Institute Working Paper 412. Federal Reserve Bank of Dallas (2021). Available at: http://doi.org/10.24149/gwp412.
37. Wang Y, Zhang B, Diao X, Wu C. Commodity Price Changes and the Predictability of Economic Policy Uncertainty. Econ Lett (2015) 127:39–42. doi:10.1016/j.econlet.2014.12.030
38. Altig D, Baker S, Barrero JM, Bloom N, Bunn P, Chen S, et al. Economic Uncertainty before and during the COVID-19 Pandemic. J Public Econ (2020) 191:104274. doi:10.1016/j.jpubeco.2020.104274
39. Baker SR, Davis SJ, Levy JA. State-Level Economic Policy Uncertainty. Working Paper 2022-21. Chicago, IL: Becker Friedman Institute for Economics at UChicago (2022).
40. Qureshi S, Chu B, Demers FS, Demers M. Using Natural Language Processing to Measure COVID-19-Induced Economic Policy Uncertainty for Canada and the US. Carleton Economics Working Papers (CEWP) 22-01. Ottawa, Canada: Carleton University (2022).
41. Arbatli Saxegaard EC, Davis SJ, Ito A, Miake N, Miake N. Policy Uncertainty in Japan. J Jpn Int Economies (2022) 64:101192. doi:10.1016/j.jjie.2022.101192
42. Liu B. Sentiment Analysis: Mining Opinions, Sentiments, and Emotions. Cambridge University Press (2020).
43. O’Connor B, Balasubramanyan R, Routledge BR, Smith NA. From Tweets to Polls: Linking Text Sentiment to Public Opinion Time Series. In: Fourth International AAAI Conference on Weblogs and Social Media (2010).
44. Zhang L, Wang S, Liu B. Deep Learning for Sentiment Analysis: A Survey. Wiley Interdiscip Rev Data Mining Knowledge Discov (2018) 8(4):e1253. doi:10.1002/widm.1253
45. Greene D, Reid F, Sheridan G, Cunningham P. Supporting the Curation of Twitter User Lists (2011). arXiv preprint arXiv:1110.1349.
46. Greene D, Sheridan G, Smyth B, Cunningham P. Aggregating Content and Network Information to Curate Twitter User Lists. In: Proceedings of the 4th ACM RecSys Workshop on Recommender Systems and the Social Web (2012). p. 29–36. doi:10.1145/2365934.2365941
48. Kalemli-Özcan S. US Monetary Policy and International Risk Spillovers. National Bureau of Economic Research Working Papers w26297. Cambridge, MA: National Bureau of Economic Research (2019). Available at: http://doi.org/10.3386/w26297.
49. Cogley T, Nason JM. Effects of the Hodrick-Prescott Filter on Trend and Difference Stationary Time Series: Implications for Business Cycle Research. J Econ Dyn Control (1995) 19(1-2):253–78. doi:10.1016/0165-1889(93)00781-x
50. Schuler YS. On the Cyclical Properties of Hamilton’s Regression Filter. Discussion Papers 03/2018. Frankfurt, Germany: Deutsche Bundesbank (2018). Available at: http://dx.doi.org/10.2139/ssrn.3127430.
51. Phillips PCB, Shi Z. Boosting: Why You Can Use the HP Filter. Int Econ Rev (2021) 62(2):521–570. doi:10.1111/iere.12495
52. Ravn MO, Uhlig H. On Adjusting the Hodrick-Prescott Filter for the Frequency of Observations. Rev Econ Stat (2002) 84(2):371–6. doi:10.1162/003465302317411604
53. Johansen S. Likelihood-Based Inference in Cointegrated Vector Autoregressive Models. Oxford University Press (1995).
55. Lütkepohl H, Martin K. Applied Time Series Econometrics. Cambridge: Cambridge University Press (2004).
56. Altug S, Yeşiltaş S. Investment under Stormy Skies: The Case of Russian Firms during 2004-2016. CEPR Discussion Papers 16646. London, UK: Centre for Economic Policy Research (2021).
57. Bloom N. Fluctuations in Uncertainty. J Econ Perspect (2014) 28(2):153–76. doi:10.1257/jep.28.2.153
58. Cha M, Haddadi H, Benevenuto F, Gummadi K. Measuring User Influence in Twitter: The Million Follower Fallacy. In: Proceedings of the International AAAI Conference on Web and Social Media (2010) 4(1):10–17. Available at: https://ojs.aaai.org/index.php/ICWSM/article/view/14033.
59. Verweij P. Twitter Links between Politicians and Journalists. Journalism Pract (2012) 6(5-6):680–91. doi:10.1080/17512786.2012.667272
60. Weng J, Lim E-P, Jiang J, Qi H. TwitterRank: Finding Topic-Sensitive Influential Twitterers. In: Proceedings of the Third ACM International Conference on Web Search and Data Mining. New York, NY, USA: WSDM ’10 Association for Computing Machinery (2010). p. 261–70.
61. Saif H, Fernandez M, He Y, Alani H. Evaluation Datasets for Twitter Sentiment Analysis: A Survey and A New Dataset, the STS-Gold. In: 1st Interantional Workshop on Emotion and Sentiment in Social and Expressive Media: Approaches and Perspectives from AI (EESEM 2013); 2013 December 3; Turin, Italy (2013). Available at: http://www.di.unito.it/patti/essem13/index.html.
62. Aruoba SB, Diebold FX, Scotti C. Real-time Measurement of Business Conditions. J Business Econ Stat (2009) 27(4):417–27. doi:10.1198/jbes.2009.07205
63. Bollerslev T, Engle RF, Wooldridge JM. A Capital Asset Pricing Model with Time-Varying Covariances. J Polit Economy (1988) 96(1):116–31. doi:10.1086/261527
64. Baba Y, Engle RF, Kraft DF, Kroner KF. Multivariate Simultaneous Generalized ARCH. San Diego: mimeo, Department of Economics, University of California (1990).
Keywords: Twitter-based data, economic policy uncertainty, monetary policy, financial markets, Turkey
Citation: Yeşiltaş S, Şen A, Arslan B and Altuğ S (2022) A Twitter-Based Economic Policy Uncertainty Index: Expert Opinion and Financial Market Dynamics in an Emerging Market Economy. Front. Phys. 10:864207. doi: 10.3389/fphy.2022.864207
Received: 01 February 2022; Accepted: 22 April 2022;
Published: 30 May 2022.
Edited by:
Federico Rossi, University of Siena, ItalyReviewed by:
Lee A. Smales, University of Western Australia, AustraliaMarcin Wolski, European Investment Bank, Luxembourg
Copyright © 2022 Yeşiltaş, Şen, Arslan and Altuğ. This is an open-access article distributed under the terms of the Creative Commons Attribution License (CC BY). The use, distribution or reproduction in other forums is permitted, provided the original author(s) and the copyright owner(s) are credited and that the original publication in this journal is cited, in accordance with accepted academic practice. No use, distribution or reproduction is permitted which does not comply with these terms.
*Correspondence: Sumru Altuğ, sa287@aub.edu.lb