- 1Department of Internal Medicine II, University Ulm Medical Center, Ulm, Germany
- 2Department of Radiology, University Ulm Medical Center, Ulm, Germany
Purpose: To adapt the non-uniform Self-Gating (nuSG) method and compare it to established self-gating approaches for lung imaging in uniform and highly irregular respiratory patterns.
Methods: Six healthy volunteers underwent free breathing lung MRI using a radial tiny golden angle ultrashort echo-time sequence. Acquisitions were performed with the volunteer breathing as uniformly as possible and with a deliberately non-uniform respiratory pattern. The acquired data was reconstructed with the nuSG method, previously introduced for cardiac imaging and imaging of the temporomandibular joint (TMJ) and compared to established k-space based and image-based self-gating approaches. Residual motion blur, SNR and functional values were assessed and compared to reference breath-hold acquisitions.
Results: nuSG is capable of reconstructing high-quality images for uniform and non-uniform breathing patterns and is furthermore capable of resolving motion in cases where additional motion is superimposed or no clear motion surrogate exists. Derived functional values do not differ significantly from other image-based gated reconstructions - and in the case of non-uniform respiratory patterns replicate the reference BH values.
Conclusion: Image based approaches are computationally more demanding but yield better results in all aspects. In scenarios with a direct surrogate for respiratory motion (i.e. the lung-liver interface) the extraction of a one-dimensional navigator is sufficient. When there is no direct surrogate for the motion of the target structure available (e.g., considerable through-plane motion or a different source of motion), the two-dimensional correlation-based measure used in nuSG is able to track the motion more accurately.
1 Introduction
Over the last decade, magnetic resonance imaging (MRI) has emerged as a promising alternative to high resolution computed tomography in lung imaging [1, 2]. Its capabilities in the visualization and assessment of functional and morphological information without ionizing radiation makes lung MRI attractive in paediatrics [1, 3], in the early detection of diseases [4], as well as in longitudinal imaging studies [5]. However, its clinical use is still limited due to very short
According to [16], motion correction strategies are divided into three groups, comprising 1) respiratory gating, which utilizes a respiratory gating signal to acquire and reconstruct only data of one specific motion state [17]; 2) image registration techniques, which align all motion states to one image via registration and iterative reconstruction techniques [16, 18] and 3) motion resolved reconstruction, which groups continuously acquired data into defined motion states. Motion resolved reconstructions can generally be accomplished using self-gating techniques, which can either exploit data from the spatial frequency domain (k-space) [19–21] or from navigator-like signals, extracted from low spatial and high temporal resolution images, for which a superior performance was reported [12, 22]. However, self-gating performs best for uniform motion and image quality is compromised in patients with irregular breathing patterns, e.g., due to shortness of breath or coughing.
In this work we adapted the non-uniform self-gating (nuSG) framework [23] to motion resolved lung imaging. The nuSG algorithm was initially introduced to resolve “cyclic motion with a nonuniform pace”, as encountered during arrhythmias in cardiac imaging or the voluntary movement of the temporomandibular joint (TMJ). For lung application, the approach was extented for coping with non-reproducible motion amplitudes and rare motion states. Application of nuSG to lung imaging thus enables dynamic imaging of the (non-uniform) respiratory motion for subsequent quantification of relevant lung function parameters [15, 24, 25] from one single, continuously acquired UTE dataset. Quantitative morphological and functional lung parameters were evaluated for uniform and non-uniform respiratory patterns and directly compared to established self-gating and breath-hold methods. Further, qualitative assessment of applying nuSG to improve reconstruction fidelity of structures (e.g., large vessels), likely experiencing more complex motion patterns (e.g., cardiac and lung motion) is presented.
2 Materials and Methods
All experiments were performed on a clinical 1.5T MRI system (Achieva 1.5 T, Philips, Best, Netherlands) with a dedicated 32 channel anterior/posterior cardiac receive coil. Six healthy volunteers (all male, non-obese, mean age: 27 years) underwent the same MR protocol in supine position (Figure 1). Coronal images were acquired for each volunteer in two slices. For each slice four acquisitions were performed: two breath-hold acquisitions (inspiratory and expiratory) and two free-breathing acquisitions for which each volunteer was instructed to once breathe uniformly and once to breathe non-uniformly for the duration of the acquisition. No further instructions were given on the nature of non-uniform breathing, but it was left to the volunteer to vary the frequency and/or depth of breathing. For a more general assessment of gating strategies, axial images (free breathing only) were additionally acquired with the same acquisition protocol in a single volunteer. The free-breathing acquisitions were reconstructed with the proposed nuSG method (see below) and compared to reconstructions with image-based self-gating using navigator-like signals and two different binning strategies (Figure 2), as well as a k-space based self-gating approach (Figure 3). All images were reconstructed off-line using an in-house built software framework implemented in MATLAB (MathWorks, Natick, Massachusetts, United States). Data from different receive coils were combined by a root-sum-of-squares calculation to avoid influence of erroneous coil sensitivities in the low signal lung regions.
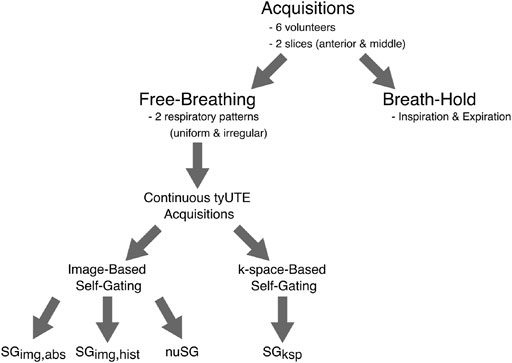
FIGURE 1. Overview of data acquisition and different reconstruction schemes. For each of the six volunteers, images were acquired in two slices (anterior and middle). At each location, two breath-hold acquisitions were performed, one at end-expiration and one at inspiration. These were followed by two free-breathing acquisitions, with the volunteers instructed to breathe as uniform as possible for one acquisition and in an irregular fashion for the other. No further instructions were given. Each of the 24 continuous acquisitions was reconstructed with the proposed nuSG method, two image-based self-gating methods with different binning strategies (as explained in Figure 2) and a k-space-based self-gating algorithm (Figure 3).
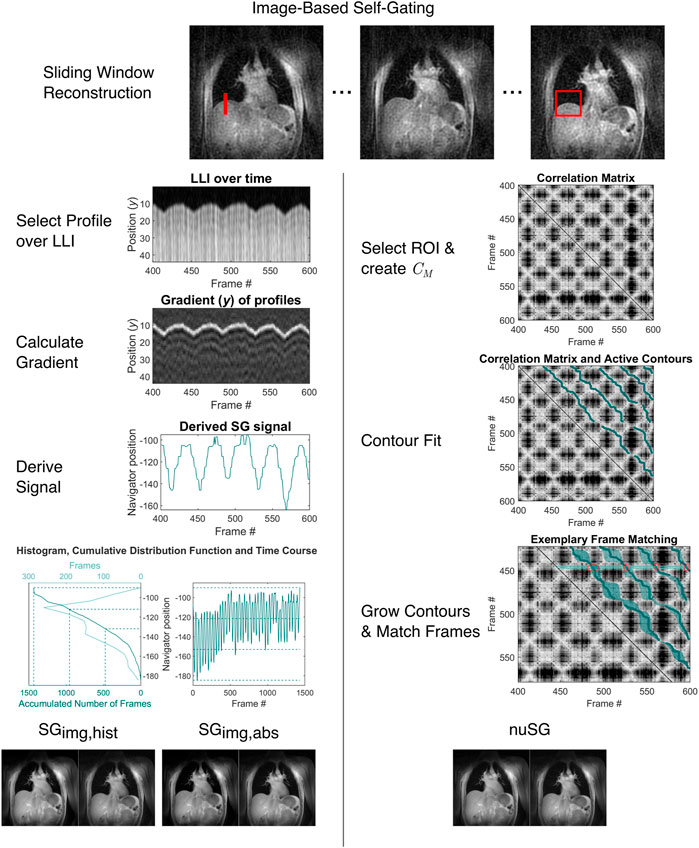
FIGURE 2. Overview of the image-based self-gating algorithms (classical and nuSG). From the continuous acquisition, intermediate sliding-window images with reduced spatial resolution are reconstructed. From these intermediate images, a profile (SGimg, left, red line) covering the lung-liver interface for all respiratory states or a 2D region of interest (nuSG, right, red rectangle) is chosen. For image-based self-gating, the intensity gradient along the chosen profile is calculated and the edge position determined as the maximum of the gradient. The position of the maximum is used as the gating signal. The gating signal is binned by two different methods: 1.) Treating the gating signal as a frequency distribution, thresholds can be found by calculating the percentiles, as illustrated by the histogram and cumulative distribution function. By definition, each bin will contain the same amount of data, however the range of the gating signal might differ. 2.) The range of the gating signal can be divided into equally-spaced intervals, which might lead to different amounts of data between bins. For nuSG, pairwise correlation of all frames is calculated and the correlation matrix derived. Subsequently, active contours are fitted. Before sorting the data from corresponding frames, the contours can be grown.
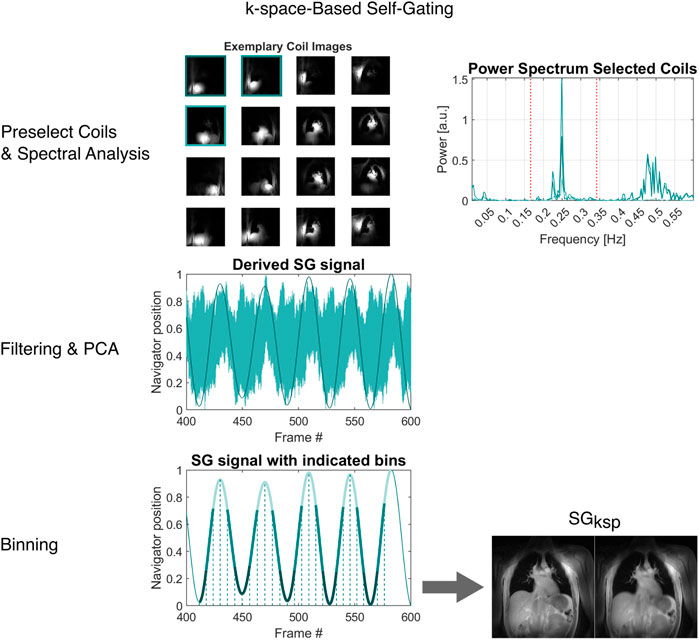
FIGURE 3. Overview of the image-based self-gating algorithm. From the continuous acquisition (or a subset thereof), average images for all coil elements are reconstructed. Coil elements with high sensitivity at the lung-liver interface are selected. The power spectrum of the centre of k-space intensity (|k0|) is then analysed to find appropriate bandpass frequencies and the filtered signals from the selected coil elements are combined via principal component analysis (PCA). The resulting gating signal is binned based on a peak-to-peak algorithm, essentially binning the data on the time axis between successive extremes.
2.1 UTE Sequence
A radial 2D spoiled center-out ultrashort echo time (UTE) sequence (TE: 0.39 ms, TR: 2.20 ms, FOV: 450 mm, FA: 3°, Slice Thickness: 8 mm, Resolution: 2 mm) was combined with tiny golden angle [22, 26, 27] angular increments to facilitate a sliding window (SW) reconstruction for retrospective gating. Data were acquired continuously for 7 s (BH) or 120 s (SG) for each slice.
2.2 nuSG Reconstruction
In general, the nuSG reconstruction process follows the detailed description given in [23]. In short (Figure 2), low spatial resolution images with a temporal resolution of ∼ 100 ms (50 TR) were reconstructed using a SW technique. For coronal image orientation, a region of interest (ROI) covering the lung-liver interface (LLI) was manually identified and the pairwise correlation of all frames, yielding the correlation matrix Mc, calculated. Continuous paths of high correlation coefficients leading from the top row to the bottom right-hand side of Mc were identified using a contour fit approach [23, 28] to avoid mixing of data acquired during inspiration and expiration. The contour fit algorithm was adapted to bridge bands of low correlation in Mc, caused by rare respiratory stages such as deep inspiration. In a further step, the contours were grown to achieve greater efficiency. Growing of the contours refers to a search in direct proximity of the contour for further frames with high correlation coefficients (bright areas in Mc), which mostly occur around resting phases. To reconstruct a certain motion state, all data corresponding to the intersection of this motion state (row in Mc) and all grown contour fits were combined. Of note, it would likewise be possible to combine intersecting data from consecutive rows of Mc to increase SNR at the cost of a decreased temporal resolution. Instead of using a region containing the LLI, in axial image orientation the ROI was chosen to cover as much of the right lung as possible while carefully excluding the larger vessels and the heart to reduce the influence of cardiac motion on Mc. Hence, the 2D deformation of the lung governs the correlation matrix and finally determines matching frames. The application of nuSG to a more complex motion pattern was further evaluated by placing the ROI over a vascular structure close to the heart, thus ensuring local cardiac and respiratory motion induced displacement. The correlation of two frames is thus highest, when they are in the same cardiac and respiratory phase. The effects of different ROIs on the Mc are shown in the Supplementary Material S1.
2.3 Self-Gating Reconstruction
k0-based (SGksp): the self-gating signal was derived from the temporal evolution of the amplitude of the center of k-space (DC-signal), which was intrinsically acquired at the beginning of each radial spoke. Coil elements showing high signal intensity at the LLI were identified manually from a time-averaged reconstruction. The raw DC-amplitudes from the selected coil elements were subsequently filtered with a bandpass filter, the cut-off frequencies of which were derived from a power spectrum analysis individually for each volunteer. The filtered signals were combined by principal component analysis yielding the final respiratory navigator signal. The different respiratory stages were identified by equidistant sectioning of the navigator signal on the time axis.
Image-based (SGimg): a respiratory navigator signal was derived from the same low-spatial high-temporal resolution images as used for the nuSG approach. E.g., for coronal image orientation a line over the LLI (Figure 2) was manually identified and the actual location of the LLI in each temporal snapshot used as respiratory navigator. The location of the LLI was identified automatically by a gradient analysis. Respiratory stages were identified either by a histogram-based binning approach (SGimg,hist) ensuring the same amount of data in each stage; or an absolute value-based approach (SGimg,abs), in which the total displacement was equidistantly subdivided. For axial image orientation, instead of the LLI a line perpendicular to the anterior chest wall was used for deriving the respiratory navigator signal.
2.4 Data Analysis
The performance of the investigated gating algorithms was assessed by analysis of the residual motion blur, SNR, lung parenchyma proton fraction (PF) and fractional ventilation (FV) in direct comparison with the breath-hold technique. Further analysis of the gating performance by assessing “pseudo”-2D-volume differences between inspiration and expiration is presented in Supplementary Material S1.
Image sharpness was quantified by assessment of the intensity profile of a single line across the LLI in end-inspiration and end-expiration, as shown in Figure 4B. A standard metric (used e.g., in [23, 29]) identifies the position of pixels corresponding to 25 and 75% of the maximum signal intensity and uses the distance as a measure for image sharpness.
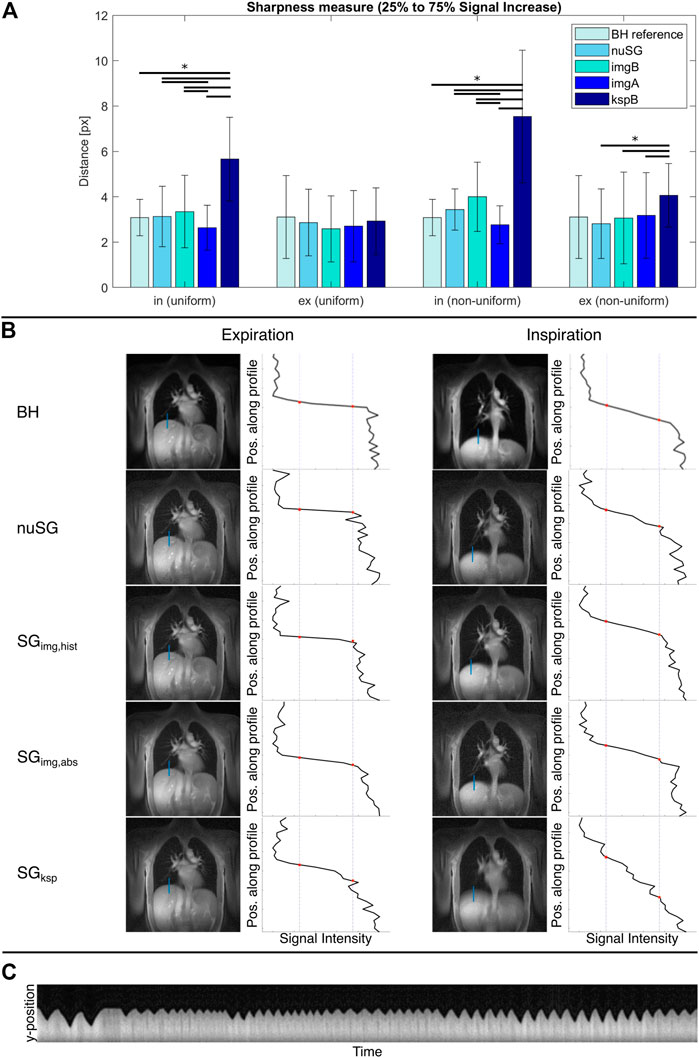
FIGURE 4. Mean sharpness measure for all reconstructions (A) and exemplary sharpness analysis (B). The inspiration and expiration breath-hold (BH) values are independent of the respiratory pattern and just repeated for better comparability. SGksp fails to resolve the inhaled motion state. All self-gated images in (B) are reconstructed from the same acquisition. The time evolution of the respiratory navigator shown in (C) clearly shows that this is a non-uniform breathing pattern. The reference BH images in the top row were acquired with the same geometry parameters. For all images, the lung-liver interface is depicted sharper in expiration compared to inspiration. Only the ksp-based gating technique is not able to resolve the inhaled state, as visible by the blurred lung-liver interface.
Prior to calculation of the SNR, PF and FV, the parenchyma was segmented semi-automatically by first drawing approximate contours around the left and right lungs and then applying a threshold to exclude the vessels and remaining non-lung parenchyma tissue. SNR (of lung parenchyma) was estimated according to [30] by
where SIROI is the mean intensity value within a selected ROI and σBG is the noise standard deviation of all pixel intensities in a selected background ROIBG and the pre-factor correcting for the Rayleigh noise distribution in magnitude images. It should be noted, that SNR estimation with multi-element receiver coils is quite challenging [31]. The calculated SNR should not be seen as absolute value, but rather a means to compare the performance of the gating algorithms within the same setup. As the gated reconstructions have the same underlying raw data and the BH acquisitions are performed with the same geometry parameters, differences in SNR reflect data efficiency or possible confounding factors of the gating algorithms (e.g. preference of certain angular orientations).
For further quantitative analyses, the amount of data used for reconstruction of the selected frames was equalized, i.e., for each slice of each volunteer an approximately equal number of profiles was used to reconstruct inspiration and expiration images with all gating approaches and the BH acquisition. For further details, please refer to the Supplementary Material S1.
The PF was calculated pixelwise according to [32].
with SIlung being the signal intensity of the lung parenchyma, SImuscle the mean signal intensity of a ROI placed in the intercostal muscles, and
After non-rigid image registration of exhaled and inhaled motion states using the Medical Image Registration Toolbox for MatLab (MIRT) [35], FV maps were calculated without noise correction according to [36].
with SIEX and SIIN being the pixel intensities during expiration and inspiration, respectively.
The significance of differences was assessed by a paired two-sided Student’s t-Test. Normal distribution of data was checked by applying a Shapiro-Wilk test. p-values below 0.05 were considered significant.
3 Results
3.1 Sharpness
The results of the sharpness analysis are provided in Figure 4A, with example data provided in Figure 4B. Please note that the presented self-gating results are reconstructed from the same data set. The mean sharpness in the BH images is similar for inspiration and expiration, with a notably larger standard deviation for the exhaled state.
As most apparent difference, the significantly (p < 0.05) inferior performance of SGksp in inspiration (uniform, non-uniform) and expiration (non-uniform) is obvious. Amongst the image-based approaches, resulting differences are less pronounced. SGimg,abs performs significantly (p < 0.05) better at resolving the inhaled state, while nuSG and SGimg,hist show superior (p > 0.05) sharpness in expiration.
3.2 SNR
The SNR analysis was performed on images reconstructed from the total amount of data after binning (Figure 5A) and from equalized data with similar number of projections per respiratory stage (Figure 5B). In non-uniform respiration, nuSG and SGimg,abs show lower SNR values for inspiration, most likely caused by the rare deep inspiration states and therefore less available data for reconstruction. While the SNR differences appear to be significant when the images are reconstructed from all available data for a certain state, the analysis for an equal amount of data exhibits less significant differences: SNR of all gated acquisitions differ significantly from the BH reference in uniform respiratory patterns. In non-uniform respiration, SGksp exhibits significantly higher SNR in inspiration, in expiration only nuSG differs significantly from the BH reference, but not from the other gated methods.
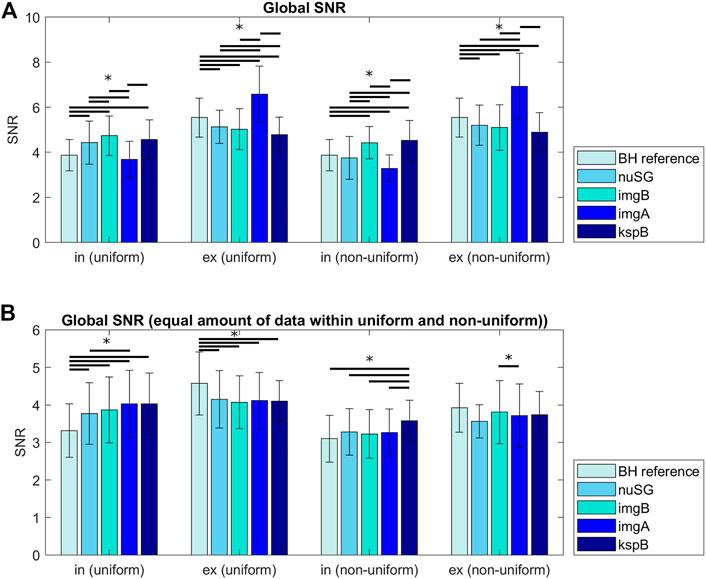
FIGURE 5. Signal to noise ratios (SNR) for all acquisitions differentiated by inspiration, expiration and the reconstruction method. The differences in (A) can partly be explained by the amount of data/number of profiles available for reconstruction of the respective motion state. (B) shows the SNR for images reconstructed from the same amount of data (gating methods with more accepted profiles were randomly undersampled to match the number of profiles). Differences between inspiration and expiration are more pronounced for non-uniform respiratory patterns.
3.3 Proton Fraction and Fractional Ventilation
The results of the PF and FV analysis are summarized in Figure 6. Values do not significantly (p > 0.5) differ in expiration (uniform, non-uniform) between BH reference and any of the investigated self-gating techniques. Significant differences result between the BH reference and self-gating approaches for inspiration (uniform, non-uniform) with significant (p < 0.05) overestimation of the PF for SGksp when compared to the image-based approaches. The image-based approaches only show slight differences between each other (p > 0.05) for non-uniform respiration.
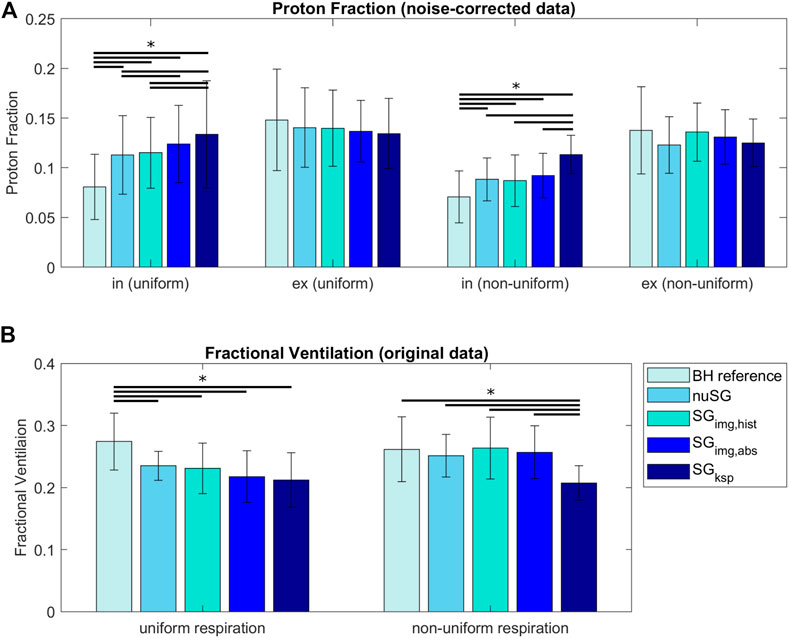
FIGURE 6. Proton Fraction (PF, (A)) and Fractional Ventilation (FV, (B)) for reconstructions with equal amount of data (within each acquisition). For uniform respiratory patterns, PFs are higher in inspiration and lower in expiration compared to the reference BH value. For non-uniform respiratory patterns, the same is true, however differences are smaller. The FV is underestimated for uniform respiration and all gating methods compared to the BH reference. For non-uniform respiration, all three image-based strategies come very close to the BH reference, while k-space based gating is still underestimating the FV. (*: p < 0.05, paired sample t-test).
The FV values derived from non-uniform respiration and image-based gating methods are in better accordance to the reference BH than the values derived from uniform respiration. For uniform respiration, differences between all reconstructions and the BH reference are significant. For non-uniform respiration, only the FV values derived from SGksp are significantly different from the BH reference.
A table containing all statistical tests and significance levels is provided in Supplementary Material S2.
3.4 Non-LLI Based Gating
Figure 7A shows example images of a nuSG reconstruction with the correlation ROI placed across the vessels in the upper left lung, close to the heart in direct comparison to an SGimg,abs gated image in end-expiration. Where in the conventional image-based reconstruction SGimg,abs the cardiac motion component is lost, nuSG appears able to resolve the cardiac motion as well as the lung motion as visible in the close-ups.
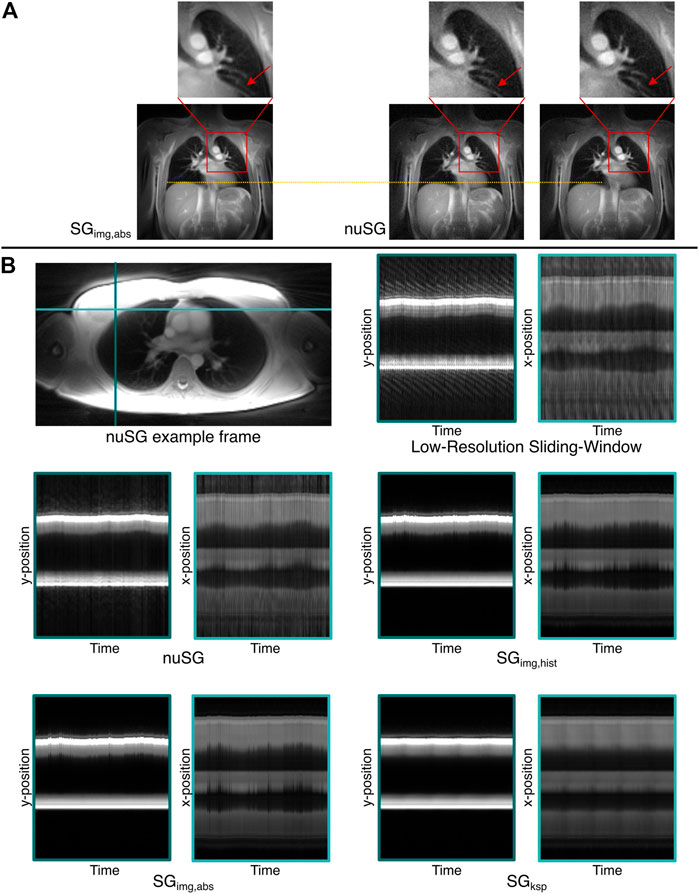
FIGURE 7. Proof-of-concept for the versatility of the nuSG algorithm. (A) shows nuSG reconstructions with the correlation calculated across the region indicated by the red square, while for SGimg,abs the same profile was chosen as in the standard reconstructions. The ability of nuSG to not only resolve respiratory but also cardiac motion, can be appreciated in the closeup (red arrow). Since the simultaneous consideration of cardiac and respiratory motion reduces the amount of data available, the SNR deteriorates. (B) shows an axial slice of the lung and the time evolution along the left-right (light blue) and anterior-posterior (turquoise) axis (nonuniform respiratory pattern). nuSG comes closest to the original motion, shown in the low-resolution sliding-window frames. While SGimg,abs and SGimg,hist are somewhat close to the original frames, SGksp completely fails to resolve the motion.
The superior performance of nuSG in case of more complex motion patterns can be appreciated from Figure 7B, showing an axial slice (top left) with resulting intensity profiles over time (top right) derived from two orthogonal lines as indicated in the axial image. As the ability to resolve non-uniform motion might not be visible in static images due to the smaller changes in the axial orientation, m-mode like plots were derived. For this purpose, the final gated frames were assigned to all profiles that were originally used to reconstruct the frame. This way, an image series could be created matching the original motion pattern from which intensity profiles could be extracted.
4 Discussion
The use of breath-holds for quantitative lung imaging might be limited. Even though motion artefact free images might be obtained, the related low SNR often causes insufficient data fidelity for subsequent quantitative data analysis, especially in patients with impaired lung function. Acquisition over several breathing cycles may yield higher SNR, but to avoid severe image blur and to enable multi-phase reconstruction, retrospective gating is required. In this context, self-gating methods either based on intensity variations of the k-space center (SGksp [19]) or based on low-resolution images (SGimg [12, 22]) have been introduced to relate each spoke to a specific respiratory motion state. Where in case of uniform respiratory motion, sorting of the data into equally spaced bins (SGksp, SGimg,hist) works well, non-uniform motion such as deep breathes or irregular respiratory frequencies often impair the resulting image quality. Similar limitations in cardiac imaging (arrhythmia) and imaging of the temporomandibular joint [23] have been addressed by using a two-dimensional correlation approach ensuring sorting of the data according to the real motion state. In both cases, however, the range of movement is more limited than for breathing. Rare motion states, such as deep inspiration, typically do not occur very often and lead to black bands (areas of low correlation) in the correlation matrix. The fitting of active contours has thus to be adapted to bridge these bands of low correlation. In this contribution we could show that the image quality of lung imaging can be increased in comparison with established self-gating strategies, especially in case of non-uniform motion by using a distance based metric (SGimg,abs) or the aforementioned two-dimensional correlation (nuSG). Where in this work the resulting multi-phase images were predominantly used for deriving functional information of the parenchyma, it might also enable the further analysis of clinically relevant respiratory patterns.
SGksp
In the presented measurements, SGksp performed worst, especially for non-uniform respiratory patterns. While on the one hand side, filtering of the signal with bandpass filters might cut off information from very short or long respiratory cycles, the method ultimately recognizes trigger-like signals (i.e., extrema in the gating signal) without information on the actual position of the LLI. The exhaled motion state can be sufficiently well resolved, while the inhaled state proves to be more challenging, since only the exhaled motion state appears constant throughout almost all respiratory cycles (Figure 8). A blurred LLI leads to erroneous segmentations and general image blur, which will in turn distort PF and FV values.
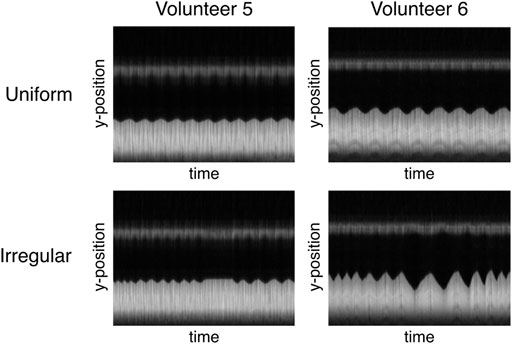
FIGURE 8. Different interpretation of “irregular breathing” for two volunteers illustrated by the time course of the profile used for the image-based navigator. While regular breathing is very similar for both volunteers (constant amplitude and frequency), volunteer 5 mainly varies the respiration frequency at constant amplitude and volunteer six exhibits different lengths and amplitudes for subsequent respiratory cycles.
SGimg and nuSG
The histogram-based binning SGimg,hist suppresses extreme states (outliers), by grouping this data with less extreme positions. If e.g., deep inspiration appears only once or twice, its impact on the final image quality might be negligible as most of the inspiration bin data stems from a “normal” inspiration state. However, as deep inspiration becomes more common (without being the main inhaled state), data over a larger number of displacements is averaged, leading to unsharp reconstructions. For rather uniform respiration, SGimg,hist provides a good gating option as the amount of data is equal for all states which facilitates analysis (same SNR level, easier calculation of PF and FV).
For coronal slices, SGimg,abs can be considered as an excellent and simple to use approach, as the real displacement of the LLI is used for deriving the gating signal, which as such allows for simple identification of irregular motion cycles and a more accurate binning of the data. SGimg,abs yields excellent results as long as the irregular motion is restricted to a single dimension. Results of similar quality were achieved with the nuSG approach for the image sharpness of the LLI. For uniform motion, SGimg,abs resolves the inhaled state significantly better than all other gating methods (18% mean advantage over nuSG). nuSG itself offers a mean improvement of 6.4% compared to SGimg,hist and 44.7% compared to SGksp. For the exhaled state, the sharpness of all gating methods is comparable (less than 10% deviation from nuSG’s sharpness value). For non-uniform respiratory patterns, nuSG resolves the inhaled motion state better than SGimg,hist (14.1%) and SGksp (54.4%). While SGimg,abs once again has better sharpness values for the inhaled state (24.3%), nuSG exhibits better sharpness values for the exhaled state (11.4%). However, nuSG has the intrinsic further advantage of not relying on displacements in 1D intensity profiles, but considering general 2D motion and distortions as visible in the underlying images used for the correlation. This resulted in a clear advantage for the depiction of structures underlying a more complex motion pattern, such as e.g., the vasculature close to the heart. Further, with the introduced contours, the nuSG matrix formalism yields the advantage of intrinsically separating data acquired during inhalation from data acquired during exhalation.
All image-based approaches have in common that they rely on a sufficient temporal resolution of the SW reconstruction. Gating quality might be impaired by motion blur in the SW images due to very fast respiratory motion, and a rejection of blurred input images might be considered. Further, the amount of data used for the reconstruction of a certain motion state is not constant for the displacement and nuSG approaches. Especially extreme motion states, which can only be resolved with nuSG or the image-based method with absolute binning, appear less often, leading to less projections and hence lower SNRs even in case of perfect gating, as shown by the SNR comparison between the different gating approaches. This has to be carefully considered during subsequent quantitative data analysis, since especially for low SNR data, the noise floor impacts the resulting figures. As suggested previously ([32]), subtraction of the noise floor is highly recommended to minimize impact on the PF. Further discussion of the influence of noise correction is presented in the Supporting Information 3. In the presented comparison, similar amounts of data were used for the reconstruction of all images to guarantee comparable noise levels and to such minimize the impact of the noise floor. With equalized amounts of data, SNR resulted in similar values for all gating approaches.
Reconstruction times among the different image-based methods are similar, as the most time-consuming step is the reconstruction of intermediate low-resolution images. Placement of the navigator column for classical gating and the ROI for nuSG has been performed manually but could potentially be automated in the future. Depending on the size of the ROI and its contents, the values of the correlation matrix might differ. While fitting of the active contours is independent, the subsequent growing step is implemented with a threshold and has to be specified for a given scenario (anatomy, temporal resolution of SW images). All coronal images used for quantitative evaluation were reconstructed with the same parameters.
Breath-Hold vs. Self-Gating
The direct comparison between the BH and SG acquisitions is difficult, since subjects naturally breathe deeper in breath-hold acquisitions than during continuous breathing. This effect is supposed to be more pronounced in inspiration than in expiration. This is nicely reflected in the outcome of the PF and FV figures. Where in expiration no significant differences are observed for the PF between the SG and BH method, inspiration values are significantly higher for the SG approaches. This is most likely related to the higher respiratory amplitude during BH acquisitions. The higher deviation for SGksp may be caused by the inferior image sharpness. The lower respiratory amplitude is also reflected in the FV figures. Deeper respiration in non-uniform motion causes FV values closer to the BH situation. As in PF, SGksp performs worse, most likely due to increased image blur.
General Limitations of PF and FV
In general, absolute PF values must be interpreted with care, since correction with an assumed
In case of a single dominant motion direction, as in the coronal slices, consideration of the real displacement (SGimg,abs) works well, even in case of non-uniform motion. In case of more complex motion and deformation as e.g. in case of axial imaging or imaging of the structures close to the heart, utilization of the full image information as done in nuSG appears clearly advantageous. In both cases, nuSG is able to resolve the motion. However, since this means that only less data can be considered, the SNR is reduced.
CONCLUSION
Image-based gating strategies perform superior to gating methods utilizing the center of k-space intensity. While in the case of uniform motion all investigated self-gating techniques provide almost reasonable results, strategies taking into account the actual displacement result in superior image quality for non-uniform motion. In this context, the presented nuSG approach represents an attractive alternative by full consideration of the 2D image information. This even allows complex movement patterns to be taken into account in the gating process.
Data Availability Statement
The datasets presented in this article are not readily available because of the privacy of the volunteers. Requests to access the datasets should be directed to Volker Rasche, volker.rasche@uni-ulm.de.
Ethics Statement
The studies involving human participants were reviewed and approved by the Ethics Committee of Ulm University. The patients/participants provided their written informed consent to participate in this study.
Author Contributions
PM, MB, WR, and VR contributed to conception and design of the study. FS adapted the nuSG algorithm to lung imaging. PM optimized and standardized the nuSG algorithm and all other gating techniques. PM, FS, and KS performed all measurements. PM, HF, and FS carried out all reconstructions, the statistical analysis and interpreted the results. PM and TS wrote the first draft of the manuscript. All authors contributed to manuscript revision, read and approved the submitted version.
Funding
This work was funded by the German Research Foundation under grant agreement 465599659.
Conflict of Interest
The authors declare that the research was conducted in the absence of any commercial or financial relationships that could be construed as a potential conflict of interest.
Publisher’s Note
All claims expressed in this article are solely those of the authors and do not necessarily represent those of their affiliated organizations, or those of the publisher, the editors and the reviewers. Any product that may be evaluated in this article, or claim that may be made by its manufacturer, is not guaranteed or endorsed by the publisher.
Acknowledgments
The authors thank the Ulm University center for Translational Imaging MoMAN for its support. The authors would like to acknowledge Stefan Wundrak, PhD, for providing the original cardiac/TMJ implementation of the non-uniform self-gating algorithm and for helpful discussions. The authors declare that this study received continuous technical support from Philips Healthcare. Philips Healthcare was not involved in the study design, collection, analysis, interpretation of data, the writing of this article or the decision to submit it for publication.
Supplementary Material
The Supplementary Material for this article can be found online at: https://www.frontiersin.org/articles/10.3389/fphy.2022.836178/full#supplementary-material
References
1. Hirsch FW, Sorge I, Vogel-Claussen J, Roth C, Gräfe D, Päts A, et al. The Current Status and Further Prospects for Lung Magnetic Resonance Imaging in Pediatric Radiology. Pediatr Radiol (2020) 50:734–49. doi:10.1007/s00247-019-04594-z
2. Serra G, Milito C, Mitrevski M, Granata G, Martini H, Pesce AM, et al. Lung Mri as a Possible Alternative to Ct Scan for Patients with Primary Immune Deficiencies and Increased Radiosensitivity. Chest (2011) 140:1581–9. doi:10.1378/chest.10-3147
3. Sodhi KS, Khandelwal N, Saxena AK, Singh M, Agarwal R, Bhatia A, et al. Rapid Lung Mri in Children with Pulmonary Infections: Time to Change Our Diagnostic Algorithms. J Magn Reson Imaging (2016) 43:1196–206. doi:10.1002/jmri.25082
4. Sim AJ, Kaza E, Singer L, Rosenberg SA. A Review of the Role of Mri in Diagnosis and Treatment of Early Stage Lung Cancer. Clin Translational Radiat Oncol (2020) 24:16–22. doi:10.1016/j.ctro.2020.06.002
5. Kirby M, Pike D, McCormack D, Sin D, Lam S, Coxson H, et al. Longitudinal Computed Tomography and Magnetic Resonance Imaging of Copd: Thoracic Imaging Network of canada (Tincan) Study Objectives. J Copd F (2014) 1:200–11. doi:10.15326/jcopdf.1.2.2014.0136
6. Holmes JE, Bydder GM. Mr Imaging with Ultrashort Te (Ute) Pulse Sequences: Basic Principles. Radiography (2005) 11:163+174. doi:10.1016/j.radi.2004.07.007
7. Weiger M, Brunner DO, Dietrich BE, Müller CF, Pruessmann KP. Zte Imaging in Humans. Magn Reson Med (2013) 70:328–32. doi:10.1002/mrm.24816
8. Bae K, Jeon KN, Hwang MJ, Lee JS, Ha JY, Ryu KH, et al. Comparison of Lung Imaging Using Three-Dimensional Ultrashort echo Time and Zero echo Time Sequences: Preliminary Study. Eur Radiol (2019) 29:2253–62. doi:10.1007/s00330-018-5889-x
9. Bae K, Jeon KN, Hwang MJ, Lee JS, Park SE, Kim HC, et al. Respiratory Motion-Resolved Four-Dimensional Zero echo Time (4d Zte) Lung Mri Using Retrospective Soft Gating: Feasibility and Image Quality Compared with 3d Zte. Eur Radiol (2020) 30(9):5130–8. doi:10.1007/s00330-020-06890-x
10. Burris NS, Johnson KM, Larson PE, Hope MD, Nagle SK, Behr SC, et al. Detection of Small Pulmonary Nodules with Ultrashort echo Time Sequences in Oncology Patients by Using a Pet/mr System. Radiology (2016) 278:239–46. doi:10.1148/radiol.2015150489
11. Zucker EJ, Cheng JY, Haldipur A, Carl M, Vasanawala SS. Free-breathing Pediatric Chest Mri: Performance of Self-Navigated golden-angle Ordered Conical Ultrashort echo Time Acquisition. J Magn Reson Imaging (2018) 47:200–9. doi:10.1002/jmri.25776
12. Tibiletti M, Paul J, Bianchi A, Wundrak S, Rottbauer W, Stiller D, et al. Multistage Three-Dimensional Ute Lung Imaging by Image-Based Self-Gating. Magn Reson Med (2016) 75:1324–32. doi:10.1002/mrm.25673
13. Wild JM, Marshall H, Bock M, Schad LR, Jakob PM, Puderbach M, et al. Mri of the Lung (1/3): Methods. Insights Imaging (2012) 3:345–53. doi:10.1007/s13244-012-0176-x
14. Biederer J, Beer M, Hirsch W, Wild J, Fabel M, Puderbach M, Van Beek EJ. MRI of the Lung (2/3). Why … when … How? Insights Imaging (2012) 3:355–71. doi:10.1007/s13244-011-0146-8
15. Lederlin M, Crémillieux Y. Three-dimensional Assessment of Lung Tissue Density Using a Clinical Ultrashort echo Time at 3 Tesla: a Feasibility Study in Healthy Subjects. J Magn Reson Imaging (2014) 40:839–47. doi:10.1002/jmri.24429
16. Zhu X, Chan M, Lustig M, Johnson KM, Larson PEZ. Iterative Motion-Compensation Reconstruction Ultra-short Te (Imoco Ute) for High-Resolution Free-Breathing Pulmonary Mri. Magn Reson Med (2020) 83:1208–21. doi:10.1002/mrm.27998
17. Ehman RL, McNamara MT, Pallack M, Hricak H, Higgins CB. Magnetic Resonance Imaging with Respiratory Gating: Techniques and Advantages. AJR Am J Roentgenol (1984) 143:1175–82. doi:10.2214/ajr.143.6.1175
18. Pang J, Bhat H, Sharif B, Fan Z, Thomson LE, LaBounty T, et al. Whole-heart Coronary Mra with 100% Respiratory Gating Efficiency: Self-Navigated Three-Dimensional Retrospective Image-Based Motion Correction (Trim). Magn Reson Med (2014) 71:67–74. doi:10.1002/mrm.24628
19. Weick S, Breuer FA, Ehses P, Völker M, Hintze C, Biederer J, et al. Dc-gated High Resolution Three-Dimensional Lung Imaging during Free-Breathing. J Magn Reson Imaging (2013) 37:727–32. doi:10.1002/jmri.23798
20. Higano NS, Hahn AD, Tkach JA, Cao X, Walkup LL, Thomen RP, et al. Retrospective Respiratory Self-Gating and Removal of Bulk Motion in Pulmonary Ute Mri of Neonates and Adults. Magn Reson Med (2017) 77:1284–95. doi:10.1002/mrm.26212
21. Richter JAJ, Wech T, Weng AM, Stich M, Weick S, Breuer K, et al. Free-breathing Self-Gated 4d Lung Mri Using Wave-Caipi. Magn Reson Med (2020) 84:3223–33. doi:10.1002/mrm.28383
22. Balasch A, Metze P, Stumpf K, Beer M, Büttner SM, Rottbauer W, et al. 2d Ultrashort echo-time Functional Lung Imaging. J Magn Reson Imaging (2020) 52:1637–44. doi:10.1002/jmri.27269
23. Wundrak S, Paul J, Ulrici J, Hell E, Geibel MA, Bernhardt P, et al. A Self-Gating Method for Time-Resolved Imaging of Nonuniform Motion. Magn Reson Med (2016) 76:919–25. doi:10.1002/mrm.26000
24. Veldhoen S, Weng AM, Knapp J, Kunz AS, Stäb D, Wirth C, et al. Self-gated Non-contrast-enhanced Functional Lung MR Imaging for Quantitative Ventilation Assessment in Patients with Cystic Fibrosis. Radiology (2017) 283:242–51. doi:10.1148/radiol.2016160355
25. Bauman G, Puderbach M, Deimling M, Jellus V, Chefd'hotel C, Dinkel J, et al. Non-contrast-enhanced Perfusion and Ventilation Assessment of the Human Lung by Means of Fourier Decomposition in Proton Mri. Magn Reson Med (2009) 62:656–64. doi:10.1002/mrm.22031
26. Wundrak S, Paul J, Ulrici J, Hell E, Rasche V. A Small Surrogate for the golden Angle in Time-Resolved Radial Mri Based on Generalized Fibonacci Sequences. IEEE Trans Med Imaging (2014) 34(6):28. doi:10.1109/TMI.2014.2382572
27. Wundrak S, Paul J, Ulrici J, Hell E, Geibel MA, Bernhardt P, et al. Golden Ratio Sparse Mri Using Tiny golden Angles. Magn Reson Med (2016) 75:2372–8. doi:10.1002/mrm.25831
28. Kass M, Witkin A, Terzopoulos D. Snakes: Active Contour Models. Int J Comput Vis (1988) 1:321+331. doi:10.1007/bf00133570
29. Larson AC, Kellman P, Arai A, Hirsch GA, McVeigh E, Li D, et al. Preliminary Investigation of Respiratory Self-Gating for Free-Breathing Segmented Cine Mri. Magn Reson Med (2005) 53:159–68. doi:10.1002/mrm.20331
30. Constantinides CD, Atalar E, McVeigh ER. Signal-to-noise Measurements in Magnitude Images from Nmr Phased Arrays. Magn Reson Med (1997) 38:852–7. doi:10.1002/mrm.1910380524
31. Dietrich O, Raya JG, Reeder SB, Reiser MF, Schoenberg SO. Measurement of Signal-To-Noise Ratios in Mr Images: Influence of Multichannel Coils, Parallel Imaging, and Reconstruction Filters. J Magn Reson Imaging (2007) 26:375–85. doi:10.1002/jmri.20969
32. Hatabu H, Alsop DC, Listerud J, Bonnet M, Gefter WB. T2* and Proton Density Measurement of normal Human Lung Parenchyma Using Submillisecond echo Time Gradient echo Magnetic Resonance Imaging. Eur J Radiol (1999) 29:245–52. doi:10.1016/s0720-048x(98)00169-7
33. Yu J, Xue Y, Song HK. Comparison of Lung T2* during Free-Breathing at 1.5 T and 3.0 T with Ultrashort echo Time Imaging. Magn Reson Med (2011) 66:248–54. doi:10.1002/mrm.22829
34. Henkelman RM. Measurement of Signal Intensities in the Presence of Noise in Mr Images. Med Phys (1985) 12:232–3. doi:10.1118/1.595711
35. Myronenko A. Mirt — Medical Image Registration Toolbox for Matlab. available at: https://sites.google.com/site/myronenko/research/mirt. (Febuary 14, 2022). (2010).
36. Zapke M, Topf H-G, Zenker M, Kuth R, Deimling M, Kreisler P, et al. Magnetic Resonance Lung Function - a Breakthrough for Lung Imaging and Functional Assessment? A Phantom Study and Clinical Trial. Respir Res (2006) 7:106. doi:10.1186/1465-9921-7-106
Keywords: lung MRI, self-gating, free breathing, pulmonary imaging, UTE imaging
Citation: Metze P, Frantz H, Straubmüller F, Speidel T, Stumpf K, Beer M, Rottbauer W and Rasche V (2022) Non-Uniform Self-Gating in 2D Lung Imaging. Front. Phys. 10:836178. doi: 10.3389/fphy.2022.836178
Received: 15 December 2021; Accepted: 03 February 2022;
Published: 04 March 2022.
Edited by:
Ciprian Catana, Massachusetts General Hospital and Harvard Medical School, United StatesReviewed by:
Christian Herbert Ziener, German Cancer Research Center (DKFZ), GermanyChristoph Stefan Aigner, Physikalisch-Technische Bundesanstalt, Germany
Peter Niedbalski, University of Kansas Medical Center, United States
Matthew Willmering, Cincinnati Children’s Hospital Medical Center, United States
Copyright © 2022 Metze, Frantz, Straubmüller, Speidel, Stumpf, Beer, Rottbauer and Rasche. This is an open-access article distributed under the terms of the Creative Commons Attribution License (CC BY). The use, distribution or reproduction in other forums is permitted, provided the original author(s) and the copyright owner(s) are credited and that the original publication in this journal is cited, in accordance with accepted academic practice. No use, distribution or reproduction is permitted which does not comply with these terms.
*Correspondence: Patrick Metze, patrick.metze@uni-ulm.de