- 1Complex Systems Research Center, Shanxi University, Taiyuan, China
- 2School of Computer and Information Technology, Shanxi University, Taiyuan, China
- 3College of Physics Science and Technology, Yangzhou University, Yangzhou, China
- 4Laboratory for Climate Studies, National Climate Center, China Meteorological Administration, Beijing, China
- 5Department of Mathematics, North University of China, Taiyuan, China
Intensifying global climate change has a significant influence on the vegetation, which is the basis of most of Earth’s ecosystems. It is urgent to identify the critical meteorological elements of vegetation coverage changes to address the problems induced by climate change. Many studies, ranging from theoretical advances to data-driven analyses, have been devoted to investigating meteorological elements’ roles in changing vegetation coverage. However, little has been considered in the aspect of the meteorological elements’ seasonal scale in data-driven studies. Herein, taking China as an example, we collected satellite-derived vegetation coverage data from 2000 to 2020. We then analyzed the meteorological elements, on a seasonal scale, that affect the vegetation coverage change in terms of temperature, precipitation, and solar radiation. We revealed that the critical meteorological elements facilitating vegetation coverage area change differ in both time and space and gave a detailed analysis in line with such findings. Moreover, an apparent seasonal delay effect of meteorological elements on the vegetation coverage change is uncovered.
1 Introduction
Changes in ecosystem structure and function are caused by climate change, topography, and human activities, such as afforestation [1, 2]. Vegetation is the medium of land–air interaction, and it purifies the air and provides food [3–7]. Vegetation growth requires three processes: photosynthesis is a process in which green plants absorb water and CO2 through leaf stomata, produce organic matter, and release O2 under the action of visible light and enzyme catalysis; transpiration is a process in which plants absorb water from roots, only 1%–5% of which is used for photosynthesis, and the remaining is emitted into the air through leaf stomata; respiration is the process of oxidative decomposition of plants to release energy, water, and CO2 [8, 9]. The vegetation mainly interacts with the outside world through leaf stomata; the leaf stomatal state and conductance has remarkable difference under different climatic conditions [10, 11]. Most vegetation only opens stomata under light and interacts with the external environment; stomatal conductance is determined by temperature, moisture, humidity, and CO2 concentration [12–15]. Thus, temperature, precipitation, humidity, solar radiation, and CO2 concentration will affect vegetation growth [16–20].
The scale has always been a research hot spot in the field of ecology [1]. In recent years, most scholars have been carrying out the influence of climate change on vegetation in different temporal and spatial scales based on remote sensing data [21–25]. On the global scale, it is proposed that the growing season vegetation change in the high latitudes of the northern hemisphere is governed by temperature, the arid and semi-arid areas are dominated by precipitation, and Amazon and South and East Asia are dominated by solar radiation [16, 17]. Chen et al. [26] studied the impact of different climatic periods on vegetation change in the Northern Hemisphere from 1982 to 2013 and found that the impact of temperature on vegetation gradually decreased from spring to autumn. Conversely, the impact of solar radiation on vegetation increased. On the regional scale, Qu et al. [3] studied the key meteorological factors affecting vegetation growth during the growing season in China, indicating that northern China is mainly affected by precipitation, and other regions are affected by temperature. Piao et al. [21] studied the correlation between NDVI and climate variables for temperate grassland in China from 1982 to 1999, suggesting that the trend change of the NDVI caused by climate change is different between different vegetation types and seasons. Zhou et al. [27] analyzed the relationship between climate variability and NDVI in eastern China through correlation analysis, which showed that the NDVI in the arid area was negatively correlated with temperature and positively correlated with precipitation. The dominant factor of vegetation change is the temperature in southern China [23].
We know that the critical meteorological factors affecting vegetation change are different among different regions, decades, seasons, and vegetation types. The previous research mainly studies how temperature and precipitation affect vegetation change through correlation and trend analyses. Still, little is known about how vegetation growth responds to solar radiation change and quantifies the impact of seasonal meteorological factors on vegetation change in China. In addition, studies have shown that the impact of meteorological elements on vegetation growth has a delayed effect [28–30], Saatchi et al. [31] investigated that low-frequency drought events in the Amazon cause continuous change of forest canopy. Vegetation growth is driven by the current climate conditions and depends on early climate conditions. Therefore, the delay effect must be considered when studying the impact of meteorological elements on vegetation. The seasonal effect of meteorological elements on vegetation coverage is unclear in China. Understanding how vegetation change responds to meteorological elements is conducive to predicting and evaluating future vegetation changes.
This study aims to identify the critical meteorological element (temperature, precipitation, and solar radiation) periods that influence vegetation growth in different regions of China. Using reanalysis of meteorological elements and satellite-derived vegetation coverage data in China during 2000–2020, we examined the relationship between meteorological elements and vegetation coverage change. We explored the delay effects of meteorological elements on vegetation coverage change on a seasonal scale. More importantly, we analyzed how meteorological elements in different seasons (winter, spring, summer, and autumn) have influenced vegetation coverage in the growing season using least absolute shrinkage and selection operator (Lasso) regression analysis.
2 Materials and Methods
2.1 Data and Preprocessing
Vegetation coverage (VC) is a critical indicator of vegetation growth, which is often used in research fields of ecology, climate, hydrology, and so on [32]. The monthly VC data are calculated from the NDVI data in the 1-km monthly synthetic product of the moderate-resolution imaging spectrometer (MODIS) according to Eq. 1.
where NDVIs represents the pixel value without vegetation coverage, and NDVIv represents the pixel value of complete vegetation coverage. The monthly VC at a spatial resolution of 0.01° × 0.01° was observed during 2000–2020. Vegetation coverage less than 0.05 is considered as non-vegetated areas, which are not considered in the study [33]. VC images of the growing season (April–October) [33–37], spring (April–May), summer (June–August), and autumn (September–October) were obtained by calculating the mean of the corresponding months [21]. The meteorological elements (0.25° monthly 2m temperature (TEM), total precipitation (TPR), and surface net solar radiation (SSR)) for the period 1999–2020 are obtained from ERA Interim Data from the European Centre for Medium Range Weather Forecast (ECMWF).
We resample the VC data to ensure the same resolution of the meteorological element data, and the linear trend of the VC and meteorological element time series was removed before statistical analysis [26, 38].
2.2 Methodology
2.2.1 Partial Correlation Analysis
We analyze the data for partial correlation to explore the relationship between VC and single meteorological elements (TEM, TPR, and SSR) after controlling the influence of other variables. We calculate the partial correlation coefficient between meteorological elements and VC. Strong partial correlation means that meteorological element exerts strongly impact VC change in the regions. The critical value of the partial correlation coefficient at the 5% significance level is 0.455. We can significantly correlate VC and meteorological elements if the calculated absolute values are more significant than the critical value. The partial correlation coefficient between vegetation coverage and SSR after controlling the two variables (TEM and TPR) is
where
where rSSR,VC,TEM is Pearson’s correlation coefficient. Similarly, the partial correlation coefficient between VC and TEM and TPR can be obtained. The influence of meteorological elements on VC in different seasons is determined by partial correlation analysis. Similarly, partial correlation analysis also explains the seasonal delay effect of meteorological elements on VC.
2.2.2 Lasso Regression
To determine the critical meteorological element periods affecting growing season VC change in China, Lasso regression was used to study the relationship between seasonal meteorological elements and VC change in the growing season [39–41]. Lasso is a classical variable selection model which reduces the regression coefficient of insignificant variables to 0, retains only a few significant variables, and vastly reduces the influence of multicollinearity between variables. Lasso regression is suitable for defining the critical climate stages affecting VC change in the growing season in China. The regression coefficient of Lasso output can effectively interpret the influence of meteorological elements in different seasons on VC in the growing season. The mean vegetation coverage in the growing season is used as the index of annual vegetation growth condition; the meteorological elements (TEM, TPR, and SSR) in four seasons (previous year winter, spring, summer, and autumn) are used as independent variables, and the annual vegetation growth condition is used as dependent variables to construct the regression model. In order to eliminate the influence of different variable dimensions on the vegetation model, the data of the input are standardized at the time.
In addition, to study the changes of meteorological elements affecting vegetation growth in different decades, we divided the data from 2000 to 2020 into two stages: 2000–2010 and 2011–2020.
3 Result
3.1 Basic Characteristics of Vegetation and Meteorological Elements
The VC gradually increases from northwest to southeast from 2000 to 2020 (Figure 1). Growing season average VC is calculated for the periods 2000–2005, 2006–2010, 2011–2015, and 2016–2020. During 2000–2005, the high vegetation coverage (0.6–1.0) area accounts for 41.6%, the low vegetation coverage (0.05–0.3) area accounts for 16.0%, and the medium vegetation coverage (0.3–0.6) area accounts for about 42.4% in China. The proportion of high vegetation coverage area increased to 52.5% during 2016–2020, and the proportion of low and medium coverage area decreased to 14.1% and 33.4% respectively. The high-coverage areas in China gradually increased, and the medium-coverage and low-coverage areas gradually decreased from 2000 to 2020. Low-coverage areas are mainly distributed in Xinjiang, central and western Inner Mongolia, and Tibet. High-coverage areas are mainly distributed in southern China and the northeast, indicating that vegetation coverage has obvious differences in different regions of China.
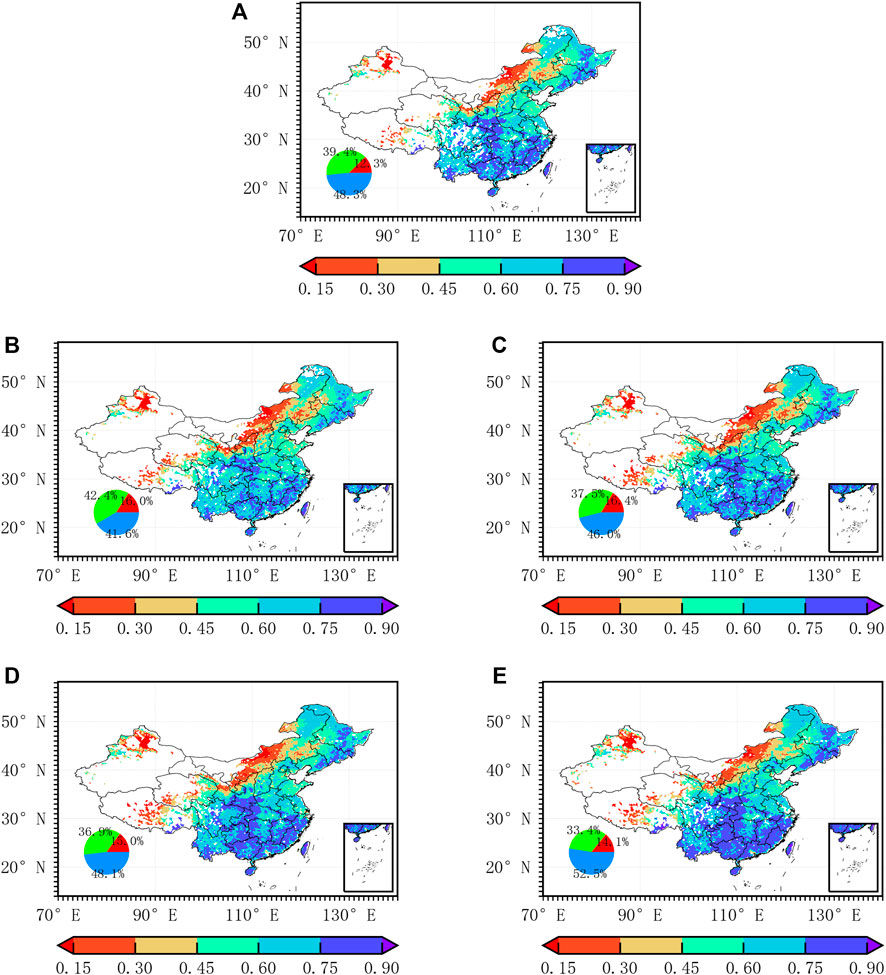
FIGURE 1. Mean value of vegetation coverage in the growing season for different periods; areas with mean value
The annual average spatial distribution of meteorological elements, TEM and TPR, gradually increase from the northwest to southeast (Figures 2A1–C1). The spatial distribution of TEM and TPR is consistent with the VC characteristics; that is, the area with appropriate TEM and sufficient TPR has higher vegetation coverage. The annual average TEM in China is about −12–24°C, the low-temperature area is mainly distributed in southern Xinjiang and Tibet, and the high-temperature area is distributed in South China. In northern China, the mean annual TPR is 0–200 mm, which belongs to arid and semi-arid area [42]. In southern China, the mean annual TPR is about 600 mm, which pertains to humid areas. The SSR remains in the range of (1000–1400) × 104J/m2 in other areas, except for Xizang and Yunnan during 2000–2020. The TEM shows a warming trend, but the Tarim Basin in Xinjiang tends to get colder during 2000–2020. Except for northeast, Qinghai, and Sichuan, the TPR of other provinces generally shows a downward trend. The SSR increased in the northwest and northeast and decreased in the middle (Figures 2A2–C2).
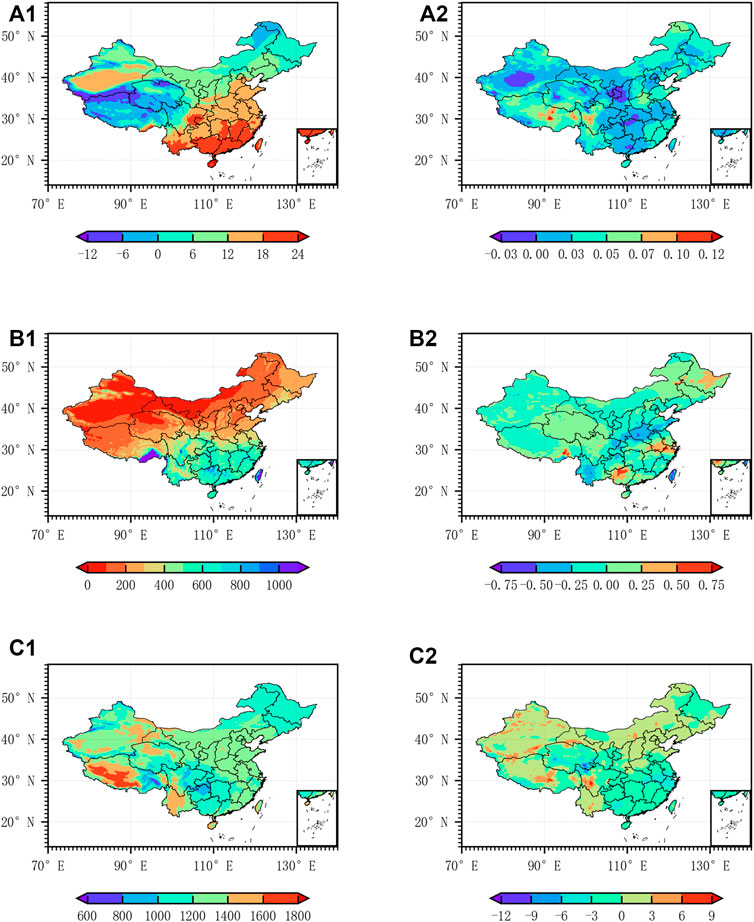
FIGURE 2. (A1–C1) Mean value of interannual TEM (°C), TPR (mm) and SSR (×104J/m2) during 2000–2020 in China. (A2–C2) Linear trend of interannual TEM (°C/Year), TPR (mm / Year), and SSR (×104J/m2 × Year) during 2000–2020 in China.
3.2 Change in Meteorological Elements Constrains Vegetation Coverage Between Seasons
Figure 3 and Table 1 show the partial correlation coefficients between VC change and meteorological elements (TEM, TPR, and SSR) in spring, summer, and autumn. Meteorological elements have obvious temporal and spatial heterogeneity on VC change [43, 44]. During spring, significant correlations between VC change and TEM (TPR and SSR) were observed across 40.29% (33.72% and 28.48%) of the total vegetation regions of China. The significant positive effects of TEM on vegetation are concentrated in northeast, Tibet, and Yunnan. The significant positive effects of TPR on vegetation are mainly distributed in arid and semi-arid areas, such as northwest and North China Plain. The significant positive effects of SSR on vegetation are mainly distributed in Chongqing, Hubei. During summer, the significantly positively affected areas by TEM decreased from 17.02% to 7.16%, especially in southern and northeast China. In arid and semi-arid areas, such as Inner Mongolia and North China Plain, VC change is negatively correlated with TEM and positively correlated with TPR. Warming in summer can enhance the activity of photosynthetic enzymes and postpone the date of frost in autumn. However, in arid and semi-arid areas, TPR increase can promote vegetation growth and warming will aggravate water loss and inhibit vegetation growth [45, 46]. The effect of SSR on VC is basically consistent with TEM. During autumn, significant positive correlations between VC and TEM were observed in southern China; SSR has little effect on VC. No matter which season, VC is sensitive to TPR in northern China, such as Inner Mongolia, Xinjiang, and North China. In the humid area of southern China, vegetation growth is susceptible to TEM. A similar result was also obtained in climate–vegetation studies [23].
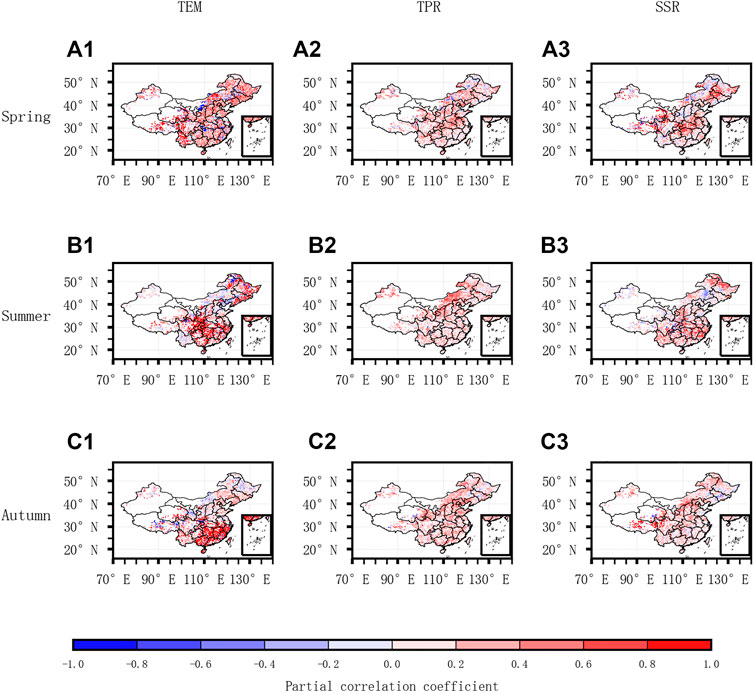
FIGURE 3. Partial correlation coefficient between vegetation coverage and meteorological elements (TEM, TPR, and SSR) in different seasons (spring, summer, and autumn), the absolute value of the partial correlation coefficient ≥0.455; it passes the 95% significance test.

TABLE 1. Proportion of vegetated areas with vegetation coverage significantly related to meteorological elements in China.
Early climate change will affect the current vegetation growth, so meteorological factors have delayed effect on vegetation growth. The partial correlations between VC and previous-season TEM, TPR, and SSR are provided in Figure 4. The strong relationship between spring VC and previous-year winter TPR and SSR was observed in Inner Mongolia. A strong positive relationship between spring TEM and summer VC change was observed in northeast and southern China. In North China, summer VC change is negatively correlated with spring TEM and positively correlated with spring TPR; spring SSR has little effect on summer VC. The autumn VC change in central and southern China is positively correlated with summer TEM and negatively correlated in other regions; summer TPR has a significant positive correlation with autumn VC. The correlation between summer SSR and TEM on vegetation change is basically the same. Suggesting that the meteorological elements have a strong seasonal effect on the VC change, significant correlations between spring VC change and previous-winter TEM (TPR and SSR) were observed across 19.62% (25.06% and 23.28%) of the total vegetation regions of China (Table 2). It shows that the seasonal effect of previous-winter TPR and SSR on spring VC change is stronger than that of TEM. However, the influence of TEM and SSR in spring and summer on VC change in summer and autumn is stronger than that of TPR.
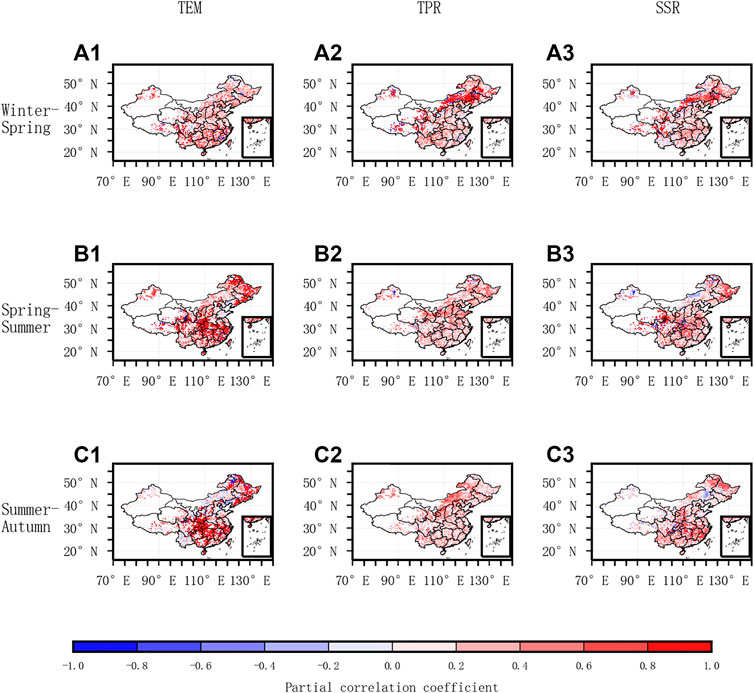
FIGURE 4. Partial correlation coefficient between vegetation coverage and meteorological elements (TEM, TPR, and SSR) of the previous seasons, the absolute value of the partial correlation coefficient ≥0.455; it passes the 95% significance test.

TABLE 2. Proportion of vegetation areas with vegetation coverage significantly related to previous-season meteorological elements in China.
3.3 Drivers of Growing Season Vegetation Cover Change
Meteorological element change is thought to affect growing season VC change, including TEM, TPR, and SSR in four seasons (previous winter, spring, summer, and autumn). We study the key drivers of vegetation coverage change in different regions of China and efforts to quantitatively analyze the contribution of each variable to vegetation coverage increase in a different decade. Precipitation-driven VC increase is most evident in northwest China and Inner Mongolia. The regions belong to arid and semi-arid areas and lack water. Therefore, the increase of precipitation can promote VC increase. SSR is a necessary condition for photosynthesis, and the increase of solar radiation in an appropriate range is conducive to vegetation growth. As the SSR in North China and central China is low, the increase of solar radiation is conducive to vegetation growth. In Yunnan, the SSR positively impacted VC change in the 2000s, but the impact of SSR gradually decreases and the impact of precipitation gradually increases in the 2010s. In the coastal area of South China, it is mainly affected by temperature in the 2000s, but the influence of temperature on it gradually weakens and precipitation gradually increases in the 2010s. From the 2000s to 2010s, the impact of precipitation on the change of vegetation coverage in China gradually increased. The effects of radiation and temperature gradually weakened. It is also found that the vital meteorological factors promoting vegetation growth are different in different decade periods. Among all meteorological elements, the driving factors of vegetation change in the growing season in China are mainly precipitation in spring and summer, followed by radiation in spring. In 2000s, 35% of Chinese vegetation coverage was dominated by precipitation, mainly concentrated in northern China. From the 2000s to 2010s, the area dominated by temperature increased from 32% to 36%, but the radiation decreased from 32% to 29% (Figure 5). There are apparent inter-decadal changes in temperature and radiation.
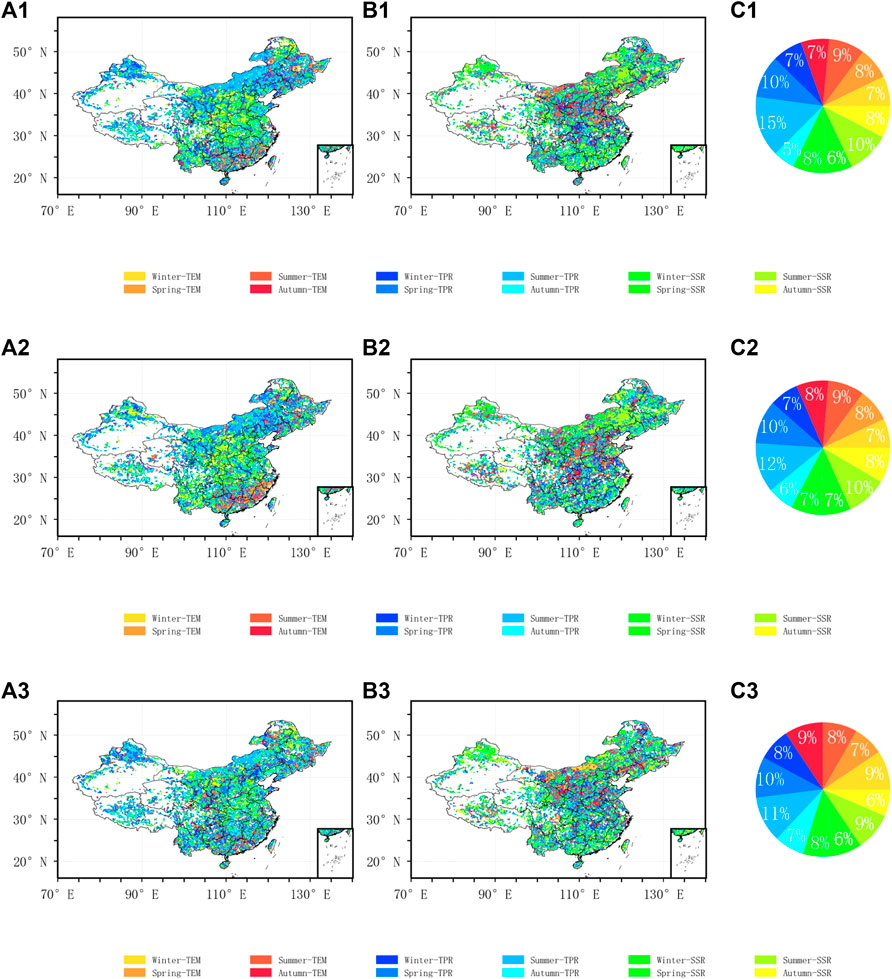
FIGURE 5. Regression coefficient: (A1–A3) Positive effect of meteorological elements on vegetation coverage change. (B1–B3) Negative effect of meteorological elements on vegetation coverage change. (C1–C3) Proportion of vegetation area in China governed by meteorological elements in different seasons, where (A1–C1) indicate 2000–2020, (A2–C2) indicate 2000s, and (A3–C3) indicate 2010s.
4 Discussion and Conclusion
This article analyzes the relationship between meteorological elements and vegetation on a seasonal scale in China. The influence of meteorological elements on growing season vegetation change has obvious seasonal shift and inter-decadal difference. The conclusions are as follows:
In China, the high-coverage areas gradually increased and the medium- and low-coverage areas gradually decreased from 2000–2005 to 2016–2020. Low-coverage areas are mainly distributed in Xinjiang and Inner Mongolia as well as Tibet, and high-coverage areas are in southern and northeast China; TEM and TPR gradually increase from the northwest to southeast (Figures 2A1–C1). The spatial distribution of TEM and TPR is basically consistent with the VC characteristics.
The results show that the key meteorological factors affecting vegetation change are different in different regions and seasons. In arid and semi-arid area, especially Inner Mongolia and Xinjiang [47, 48], the VC change mainly depends on TEM, whereas the relationship between VC change and TPR and SSR is weak during spring. There is a strong positive correlation between VC change and TPR during summer and autumn, but the relationship between VC change and TEM is negatively correlated (Figure 3). TPR increase can promote vegetation growth and warming will aggravate water loss and inhibit vegetation growth [45, 46]. These findings are consistent with the response of vegetation to climate in arid and semi-arid areas [3, 16, 17]. The vegetation growth in spring mainly depends on the previous-winter TPR and SSR, and the vegetation growth in autumn depends on the summer TPR (Figure 4). In addition, our study also shows that the increase of TPR in spring and summer is conducive to the increase of annual vegetation coverage (Figures 5C1–C3). In northeast China, spring TEM and summer VC change has a strong relationship. In the North China Plain, the VC change during spring is significantly positively correlated with TEM and TPR. During summer and autumn, it is significantly positively correlated with TPR, but the correlation with TEM is weak. Vegetation growth in summer mainly depends on spring TPR; spring SSR has little effect on summer VC. This is consistent with previous studies [3, 22, 49]. The increase of autumn SSR and winter and spring TPR is conducive to the increase of VC. In humid areas, such as South China and East China, the VC change mainly depends on the change of TEM in every season, and there is a statistically significant correlation between VC change and SSR (Figure 3). These findings are consistent with those of previous studies [3, 23]. TEM has a strong seasonal delay effect on VC change, but TPR is relatively weak (Figure 4).
Through the research, we can know the critical meteorological elements affecting vegetation change in different regions of China. Critical meteorological element modeling can more accurately predict future vegetation coverage. In the course of this study, the scale of vegetation and climate data does not match, and interpolation may cause errors in the data set. On the other hand, the study ignored the impact of human activities, such as ecological engineering and crop irrigation.
Data Availability Statement
The original contributions presented in the study are included in the article/Supplementary Material; further inquiries can be directed to the corresponding author.
Author Contributions
HB is responsible for data processing, drawing, and article writing. GS, LL, and YW are responsible for raising scientific issues. ZG and GF provided data.
Funding
The project is funded by the National Key Research and Development Program of China (Grant no. 2018YFE0109600), the National Natural Science Foundation of China under Grant no. 42 075 029, the Program for the Outstanding Innovative Teams (OITs) of Higher Learning Institutions of Shanxi, and the Outstanding Young Talents Support Plan of Shanxi province.
Conflict of Interest
The authors declare that the research was conducted in the absence of any commercial or financial relationships that could be construed as a potential conflict of interest.
Publisher’s Note
All claims expressed in this article are solely those of the authors and do not necessarily represent those of their affiliated organizations, or those of the publisher, the editors, and the reviewers. Any product that may be evaluated in this article, or claim that may be made by its manufacturer, is not guaranteed or endorsed by the publisher.
References
1. Wu J, Hobbs R. Key Issues and Research Priorities in Landscape Ecology: An Idiosyncratic Synthesis. Landscape Ecol (2002) 17:355–65. doi:10.1023/A:1020561630963
2. Wang F, Wang X, Zhao Y, Yang Z. Temporal Variations of Ndvi and Correlations between Ndvi and Hydro-Climatological Variables at lake Baiyangdian, china. Int J Biometeorol (2014) 58:1531–43. doi:10.1007/s00484-013-0758-4
3. Qu B, Zhu W, Jia S, Lv A. Spatio-temporal Changes in Vegetation Activity and its Driving Factors during the Growing Season in china from 1982 to 2011. Remote Sensing (2015) 7:13729–52. doi:10.3390/rs71013729
4. Wang W, Anderson BT, Phillips N, Kaufmann RK, Potter C, Myneni RB. Feedbacks of Vegetation on Summertime Climate Variability over the north American Grasslands. Part I: Statistical Analysis. Earth Interactions (2006) 10:1–27. doi:10.1175/EI196.1
5. Wang X, Piao S, Ciais P, Li J, Friedlingstein P, Koven C, et al. Spring Temperature Change and its Implication in the Change of Vegetation Growth in north america from 1982 to 2006. Proc Natl Acad Sci (2011) 108:1240–5. doi:10.1073/pnas.1014425108
6. Bonan GB, Pollard D, Thompson SL. Effects of Boreal forest Vegetation on Global Climate. Nature (1992) 359:716–8. doi:10.1038/359716a0
7. Piao S, Wang X, Park T, Chen C, Lian X, He Y, et al. Characteristics, Drivers and Feedbacks of Global Greening. Nat Rev Earth Environ (2020) 1:14–27. doi:10.1038/s43017-019-0001-x
8. Dusenge ME, Duarte AG, Way DA. Plant Carbon Metabolism and Climate Change: Elevated Co2 and Temperature Impacts on Photosynthesis, Photorespiration and Respiration. New Phytol (2019) 221:32–49. doi:10.1111/nph.15283
9. Smith NG, Dukes JS. Plant Respiration and Photosynthesis in Global-Scale Models: Incorporating Acclimation to Temperature and Co2. Glob Change Biol (2013) 19:45–63. doi:10.1111/j.1365-2486.2012.02797.x
10. Monje O, Bugbee B. Radiometric Method for Determining Canopy Stomatal Conductance in Controlled Environments. Agronomy (2019) 9:114. doi:10.3390/agronomy9030114
11. Yu Q, Xie X, Sun S. Andances in Simulation of Plant Photosynthetic Productivity and Canopy Evapotranspiration. Acta Ecologica sinica (in Chinese) (1999) 19:10.
12. Ma X, Song L, Yu W, Hu Y, Liu Y, Wu J, et al. Growth, Physiological, and Biochemical Responses of Camptotheca Acuminata Seedlings to Different Light Environments. Front Plant Sci (2015) 6:321. doi:10.3389/fpls.2015.00321
13. Si J, Chang Z, Su Y, Xi H, Feng Q. Stomatal Conductance Characteristics of Populus Euphrat Ica Leaves and Response to Environmental Factors in the Extreme Arid Region. Acta Botanica Boreali-Occidentalia Sinica (2008) 28:125–30. doi:10.3321/j.issn:1000-4025.2008.01.023
14. von Caemmerer S, Farquhar GD. Some Relationships between the Biochemistry of Photosynthesis and the Gas Exchange of Leaves. Planta (1981) 153:376–87. doi:10.1007/BF00384257
15. Buckley TN, Mott KA. Modelling Stomatal Conductance in Response to Environmental Factors. Plant Cel Environ (2013) 36:1691–9. doi:10.1111/pce.12140
16. Nemani RR, Keeling CD, Hashimoto H, Jolly WM, Piper SC, Tucker CJ, et al. Climate-driven Increases in Global Terrestrial Net Primary Production from 1982 to 1999. science (2003) 300:1560–3. doi:10.1126/science.1082750
17. Zhao L, Dai A, Dong B. Changes in Global Vegetation Activity and its Driving Factors during 1982-2013. Agric For Meteorology (2018) 249:198–209. doi:10.1016/j.agrformet.2017.11.013
18. Hou M, Zhao H, Wang Z. Vegetation Responses to Climate Change by Using the Satellite-Derive Normalized Difference Vegetation index: A Review. Climatic Environ Res (in Chinese) (2013) 18:353–64. doi:10.3878/j.issn.1006-9585.2012.11137
19. Sun G-Q, Zhang H-T, Wang J-S, Li J, Wang Y, Li L, et al. Mathematical Modeling and Mechanisms of Pattern Formation in Ecological Systems: a Review. Nonlinear Dyn (2021) 104:1677–96. doi:10.1007/s11071-021-06314-5
20. Xue Q, Liu C, Li L, Sun G-Q, Wang Z. Interactions of Diffusion and Nonlocal Delay Give Rise to Vegetation Patterns in Semi-arid Environments. Appl Maths Comput (2021) 399:126038. doi:10.1016/j.amc.2021.126038
21. Piao S, Mohammat A, Fang J, Cai Q, Feng J. Ndvi-based Increase in Growth of Temperate Grasslands and its Responses to Climate Changes in china. Glob Environ Change (2006) 16:340–8. doi:10.1016/j.gloenvcha.2006.02.002
22. Duo A, Zhao W, Qu X, Jing R, Xiong K. Spatio-temporal Variation of Vegetation Coverage and its Response to Climate Change in north china plain in the Last 33 Years. Int J Appl Earth Observation Geoinformation (2016) 53:103–17. doi:10.1016/j.jag.2016.08.008
23. Gao J, Jiao K, Wu S, Ma D, Zhao D, Yin Y, et al. Past and Future Influence of Climate Change on Spatially Heterogeneous Vegetation Activity in china. Earth Syst Dyn Discuss (2017) 2017:1–20. doi:10.5194/esd-2017-13
24. Piao S, Fang J, Zhou L, Guo Q, Henderson M, Ji W, et al. Interannual Variations of Monthly and Seasonal Normalized Difference Vegetation index (Ndvi) in china from 1982 to 1999. J Geophys Res (2003) 108:4401. doi:10.1029/2002JD002848
25. Zhou L, Kaufmann RK, Tian Y, Myneni RB, Tucker CJ. Relation between Interannual Variations in Satellite Measures of Northern forest Greenness and Climate between 1982 and 1999. J Geophys Res (2003) 108:4004. ACL 3–1–ACL 3–16. doi:10.1029/2002JD002510
26. Chen C, He B, Guo L, Zhang Y, Xie X, Chen Z. Identifying Critical Climate Periods for Vegetation Growth in the Northern Hemisphere. J Geophys Res Biogeosci (2018) 123:2541–52. doi:10.1029/2018JG004443
27. Zhou C, Shi R, Zhang C, Liu C, Gao W. Spatio-temporal Distribution of Ndvi and its Correlation with Climatic Factors in Eastern china during 1998-2008. In: W Gao, NB Chang, and J Wang, editors. Remote Sensing And Modeling Of Ecosystems For Sustainability XI (SPIE). San Diego, CA: SPIE Optical Engineering + Applications (2014). p. 96–105. doi:10.1117/12.2060768
28. Davis MB. Lags in Vegetation Response to Greenhouse Warming. Climatic Change (1989) 15:75–82. doi:10.1007/BF0013884610.1007/bf00138846
29. Wu D, Zhao X, Liang S, Zhou T, Huang K, Tang B, et al. Time-lag Effects of Global Vegetation Responses to Climate Change. Glob Change Biol (2015) 21:3520–31. doi:10.1111/gcb.12945
30. Kuzyakov Y, Gavrichkova O. Review: Time Lag between Photosynthesis and Carbon Dioxide Efflux from Soil: a Review of Mechanisms and Controls. Glob Change Biol (2010) 16:3386–406. doi:10.1111/j.1365-2486.2010.02179.x
31. Saatchi S, Asefi-Najafabady S, Malhi Y, Aragao LEOC, Anderson LO, Myneni RB, et al. Persistent Effects of a Severe Drought on Amazonian forest Canopy. Proc Natl Acad Sci (2013) 110:565–70. doi:10.1073/pnas.1204651110
32. Gong Z, Zhao S, Gu J. Correlation Analysis between Vegetation Coverage and Climate Drought Conditions in north china during 2001-2013. J Geogr Sci (2017) 27:143–60. doi:10.1007/s11442-017-1369-5
33. He B, Chen A, Jiang W, Chen Z. The Response of Vegetation Growth to Shifts in Trend of Temperature in china. J Geogr Sci (2017) 27:801–16. doi:10.1007/s11442-017-1407-3
34. Peng S, Chen A, Xu L, Cao C, Fang J, Myneni RB, et al. Recent Change of Vegetation Growth Trend in china. Environ Res Lett (2011) 6:044027. doi:10.1088/1748-9326/6/4/044027
35. Piao S, Cui M, Chen A, Wang X, Ciais P, Liu J, et al. Altitude and Temperature Dependence of Change in the spring Vegetation green-up Date from 1982 to 2006 in the Qinghai-Xizang Plateau. Agric For Meteorology (2011) 151:1599–608. doi:10.1016/j.agrformet.2011.06.016
36. Tucker CJ, Pinzon JE, Brown ME, Slayback DA, Pak EW, Mahoney R, et al. An Extended AVHRR 8‐km NDVI Dataset Compatible with MODIS and SPOT Vegetation NDVI Data. Int J Remote Sensing (2005) 26:4485–98. doi:10.1080/01431160500168686
37. Zhou L, Tucker CJ, Kaufmann RK, Slayback D, Shabanov NV, Myneni RB. Variations in Northern Vegetation Activity Inferred from Satellite Data of Vegetation index during 1981 to 1999. J Geophys Res (2001) 106:20069–83. doi:10.1029/2000JD000115
38. Chen C, He B, Yuan W, Guo L, Zhang Y. Increasing Interannual Variability of Global Vegetation Greenness. Environ Res Lett (2019) 14:124005. doi:10.1088/1748-9326/ab4ffc
39. Tibshirani R. Regression Shrinkage and Selection via the Lasso. J R Stat Soc Ser B (Methodological) (1996) 58:267–88. doi:10.1111/j.2517-6161.1996.tb02080.x
40. Adak A, Murray SC, Božinović S, Lindsey R, Nakasagga S, Chatterjee S, et al. Temporal Vegetation Indices and Plant Height from Remotely Sensed Imagery Can Predict Grain Yield and Flowering Time Breeding Value in maize via Machine Learning Regression. Remote Sensing (2021) 13:2141. doi:10.3390/rs13112141
41. Wang S, Liu Q, Huang C. Vegetation Change and its Response to Climate Extremes in the Arid Region of Northwest china. Remote Sensing (2021) 13:1230. doi:10.3390/rs13071230
42. Huang J, Ma J, Guan X, Li Y, He Y. Progress in Semi-arid Climate Change Studies in china. Adv Atmos Sci (2019) 36:922–37. doi:10.1007/s00376-018-8200-9
43. Jiao K-W, Gao J-B, Liu Z-H, Wu S-H, Fletcher TL. Revealing Climatic Impacts on the Temporal and Spatial Variation in Vegetation Activity across china: Sensitivity and Contribution. Adv Clim Change Res (2021) 12:409–20. doi:10.1016/j.accre.2021.04.006
44. Liu H, Zhang M, Lin Z, Xu X. Spatial Heterogeneity of the Relationship between Vegetation Dynamics and Climate Change and Their Driving Forces at Multiple Time Scales in Southwest china. Agric For Meteorology (2018) 256-257:10–21. doi:10.1016/j.agrformet.2018.02.015
45. Shen M, Piao S, Jeong S-J, Zhou L, Zeng Z, Ciais P, et al. Evaporative Cooling over the Tibetan Plateau Induced by Vegetation Growth. Proc Natl Acad Sci USA (2015) 112:9299–304. doi:10.1073/pnas.1504418112
46. Fracheboud Y, Luquez V, Björkén L, Sjödin A, Tuominen H, Jansson S. The Control of Autumn Senescence in European Aspen. Plant Physiol (2009) 149:1982–91. doi:10.1104/pp.108.133249
47. Zhuang Q, Wu S, Feng X, Niu Y. Analysis and Prediction of Vegetation Dynamics under the Background of Climate Change in Xinjiang, china. PeerJ (2020) 8:e8282. doi:10.7717/peerj.8282
48. Chuai XW, Huang XJ, Wang WJ, Bao G. NDVI, Temperature and Precipitation Changes and Their Relationships with Different Vegetation Types during 1998-2007 in Inner Mongolia, China. Int J Climatol (2013) 33:1696–706. doi:10.1002/joc.3543
Keywords: vegetation coverage, temperature, precipitation, solar radiation, China, Lasso regression
Citation: Bai H, Li L, Wu Y, Feng G, Gong Z and Sun G (2022) Identifying Critical Meteorological Elements for Vegetation Coverage Change in China. Front. Phys. 10:834094. doi: 10.3389/fphy.2022.834094
Received: 13 December 2021; Accepted: 03 January 2022;
Published: 21 January 2022.
Edited by:
Peican Zhu, Northwestern Polytechnical University, ChinaCopyright © 2022 Bai, Li, Wu, Feng, Gong and Sun. This is an open-access article distributed under the terms of the Creative Commons Attribution License (CC BY). The use, distribution or reproduction in other forums is permitted, provided the original author(s) and the copyright owner(s) are credited and that the original publication in this journal is cited, in accordance with accepted academic practice. No use, distribution or reproduction is permitted which does not comply with these terms.
*Correspondence: Guiquan Sun, gquansun@126.com