- 1School of Computer and Information Technology, Shanxi University, Taiyuan, Shanxi, China
- 2Complex Systems Research Center, Shanxi University, Taiyuan, Shanxi, China
In recent years, with the abnormal global climate change, the problem of desertification has become more and more serious. The vegetation pattern is accompanied by desertification, and thus, the study of the vegetation pattern is helpful to better understand the causes of desertification. In this work, we reveal the influences of hydrotropism on the vegetation pattern based on a vegetation–water system in the form of reaction–diffusion equations. Parameter ranges for the steady-state mode obtained by analyzing the system show the dynamic behavior near the bifurcation point. Furthermore, we found that vegetation hydrotropism not only induces spatial pattern generation but also promotes the growth of vegetation itself in this area. Therefore, through the study of vegetation patterns, we can take corresponding preventive measures to effectively prevent land desertification and improve the stability of the ecosystem in the region.
1 Introduction
Vegetation is an important part of nature and plays a leading role in the ecosystem, known as the “Ecological Engineer” [1, 2]. As a producer, vegetation can convert carbon dioxide into carbohydrates through photosynthesis and release oxygen and store energy. Moreover, vegetation coverage on the ground can not only reduce water and soil loss and protect slope land but also prevent wind and sand fixation and prevent desertification [3, 4]. In nature, the growth of vegetation will be affected by climatic conditions, geographical environment, and human activities. Nowadays, with the rapid development of irrationality in the human society and global climate change, the vegetation ecosystem has been seriously damaged, and the problem of land desertification is becoming more and more serious [5–7].
In particular, in dry and semi-dry areas, because of its climatic characteristics, the problem of land desertification is particularly prominent. In the process of land desertification, vegetation distribution is uneven, but there are certain rules. We call this uneven and regular spatial distribution of vegetation as the vegetation pattern [8–10]. In addition, different vegetation pattern structures have different significances to the function of the ecosystem, such as the strip pattern can be used as a sign of semi-desert [11]. For the study of vegetation spatial patterns in these areas, many scholars established a series of a dynamic system. Based on ecologically realistic assumptions, Klausmeier established a model with vegetation and water in 1999 and gave suitable parameters, and two pattern types can be found by numerical simulations (regular patterns and irregular patterns). This model helps us understand how rainfall and grazing affect vegetation in semi-arid regions and demonstrates the importance of non-linear mechanisms to the spatial structure of plant community [12]. In 2001, Von Hardenberg proposed a new vegetation–water system, which simulates the competition of vegetation roots for water resources [13]. In 2007, Gilad constructed a mathematical system for the study of the woody plant ecosystem in arid areas and captured various feedback mechanisms between biomass and water resources through this system [14]. Water diffuses freely in soil, and the original Klausmeier system does not consider the diffusion of water. Therefore, Vander Stelt took the diffusion of water into account on the basis of Klausmeier’s system in 2012 [15]. In 2015, Zelnik simplified Gilad’s model and combined with empirical data to study the dynamics of Namibia’s Andromeda ecosystem. The research showed that the pattern trend changes gradually in the spatially expanded ecosystem [16]. Moreover, other scholars have also established relevant systems to study vegetation patterns [17–25].
It is known to all that water is the source of life. Vegetation needs water for photosynthesis and respiration to obtain nutrients needed for vegetation growth. Moreover, when transpiration takes away a lot of heat, water can maintain the normal temperature of vegetation to maintain life activities [26, 27]. Vegetation absorbs water from the soil mainly through the root system to provide water necessary for life activities. In response to a moisture gradient, the roots of vegetation will show the characteristics of hydrotropism [28–31]. In dry and semi-dry areas, soil water distribution is uneven because of less and concentrated rainfall [32, 33]. Therefore, the vegetation root system will absorb the water in other humid areas through the extension of the root system to meet its own growth and development in these areas. At present, it is not clear how the hydrotropism effect of vegetation roots affects the growth and distribution of vegetation. Therefore, to reveal the influence of hydrotropism of vegetation roots on vegetation growth and distribution, we build a spatial system with root hydrotropism effects based on the system of Klausmeier in this paper [34–36].
The following is the content arrangement of this article. In the first place, we propose a reaction–diffusion system with hydrotropism effects and analyze the existence and stability about the equilibrium point. In the next section, we derive the amplitude equation and its coefficients through the method of multi-scale analysis. In the fourth section, on the basis of the theoretical results obtained in the previous section, we conduct a numerical simulation to obtain the dynamic behavior and show the hydrotropism of vegetation’s impact on the vegetation pattern. Finally, the last section gives conclusions and discussions on the effect of hydrotropism on vegetation growth and distribution.
2 Mathematical model and analysis
2.1 Mathematical model
In dry and semi-dry regions, soil water replenishment was mainly derived from rainfall due to their geographical environment. After the rainfall reaches the ground, one part of the rainfall penetrates into the soil and becomes groundwater or surface runoff, and the other part is lost to the atmosphere through the transpiration of vegetation and evaporation of the ground. These areas receive less rainfall because of their climatic conditions. Therefore, soil water distribution in these areas is uneven, forming a moisture gradient. In response to a moisture gradient, the roots of vegetation will show the characteristics of hydrotropism to get more water for basic life activities (Figure 1).
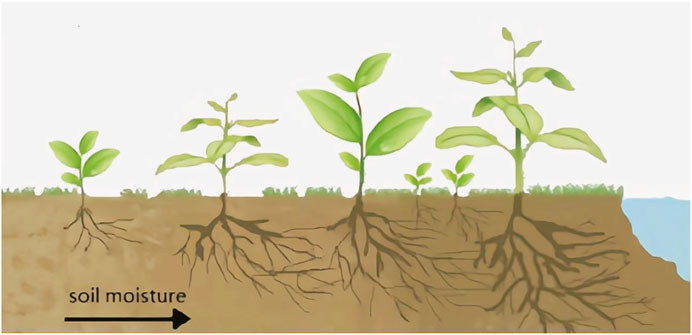
FIGURE 1. Schematic chart of hydrotropism of vegetation roots and the arrow direction indicates that the soil moisture is getting higher and higher. The roots of vegetation grow in areas to obtain more water resources.
The spatial motion of hydrotropism of vegetation roots is to absorb more water. In this sense, it is assumed that the diffusion rate of vegetation hydrotropism is proportional to the diffusion speed of water. Consequently, based on the Klausmeier system [12], we establish a system with water diffusion and vegetation hydrotropism effects as follows:
where P is the vegetation biomass and W is the soil water volume; parameters A, M, L, D1, D2 represent rainfall, plant mortality, evaporation rate, vegetation diffusion rate, and water diffusivity, respectively. The term
For the convenience of mathematical analysis, we reduce the numbers of parameters by dimensionless transformation on the system (2.1) and obtain the following system:
In this paper, we mainly study the flat ground without considering the slope’s influence on the formation of the pattern structure. Therefore, our system is finally simplified as follows:
2.2 Mathematical analysis of the model
System (2.3) without considering diffusion is as follows:
Make the right end of Eq. 2.4 equal to 0, and calculate the equilibrium point. System (2.3) has three equilibrium points, including a semi trivial steady-state solution and two non-trivial steady-state solutions:
(1) E0 = (p0, w0) = (0, a), which corresponds to no vegetation.
(2)
(3)
Because the equilibrium point E1 is always unstable when diffusion is not considered, we will only study the equilibrium point E2 given in the following section. First, linearize system (2.3) at E2 to obtain
where
We set a perturbation to the uniform stationary solution (p2, w2) and expand it in Fourier space:
Substituting Eq. 2.6 variables into Eq. 2.5, one can obtain its following characteristic equation:
It is equivalent to the following equation:
△k = a11a22−a21a12+(a21d−(a11d2+a22d1))k2+d1d2k4.
Tk = (d1+d2)k2−(a11 + a22).
The characteristic value of system (2.3) is as follows:
Then, necessary conditions for system (2.3) to generate bifurcation behavior are as follows:
According to the necessary conditions of Hopf bifurcation (2.9) and Turing bifurcation (2.10), we select a as the control variable, and the system branch diagram of system (2.3) can be drawn, as shown in Figure 2. At the same time, the dispersion relationship of system (2.3) is demonstrated in Figure 3.
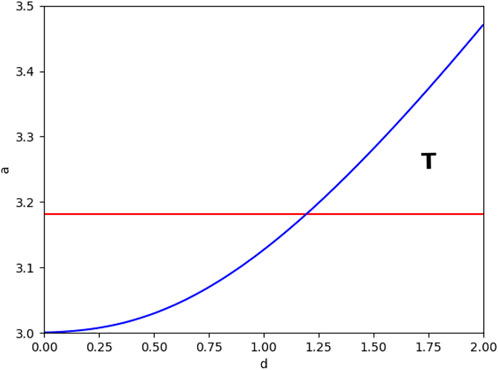
FIGURE 2. Bifurcation diagram of system (2.3) in the parameter space spanned by d and a. Among them, the red line represents the Hopf branch line, and the blue line represents the Turing branch line. The Turing area is marked with T. The parameter values are m = 1.5, d1 = 1, and d2 = 1.8.
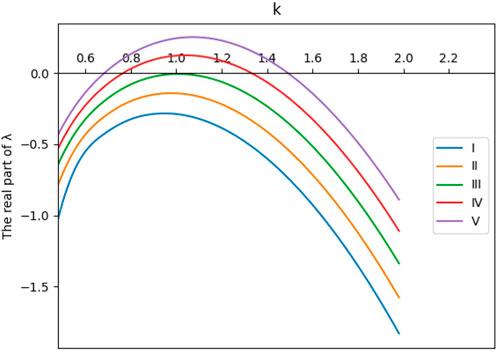
FIGURE 3. Dispersion relation of system (2.3): (I) d = 1, (II) d = 1.2, (III) d = 1.4, (IV) d = 1.6, and (V) d = 1.8. The other parameter values are a = 3.25, m = 1.5, d1 = 1, and d2 = 1.8.
Obviously, Figure 3 shows that within an appropriate parameter range, as the parameter d increases, the real part of the eigenvalue gradually increases and the Turing patterns appear.
3 Amplitude equations
Generally, the pattern structure is influenced by the most active mode of the system. The amplitude equation can describe the system’s dynamic behavior around the most active mode [10]. Therefore, we can use the amplitude equation to research the system’s dynamic behavior around the Turing bifurcation point. The pattern structure is represented by three pairs of resonance modes (νj, − νj), which are 120° angles. For this paper, through the method of multi-scale analysis, the amplitude equation of system (2.3) and its coefficients are derived.
3.1 Multi-scale analysis
First, rewrite system (2.3) at the equilibrium point E2 as follows:
Near a = aT, the solution can be written in the following form:
Making
where
Expand a, c, L, N as follows:
LT, b, h2, h3 are as follows:
Decompose the time scale (T = t, T1 = ɛt, T2 = ɛ2t), then the derivative of the amplitude A with respect to time is
Substituting (3.4) into formula (3.3), we get the equation of different order of ɛ (see Table 1).
For the order of ɛ,
where LT is the model’s linear operator at the critical point, and
with
For the order of ɛ2,
According to the solvability condition of Fredholm, to guarantee the existence of equation’s non-trivial solution, the right term of Eq. 3.8 must be the same as the
The zero characteristic value of
Use
where
Then,
It can be found that the second-order term’s coefficient value is more than 0 from all the aforementioned equations, which will cause the amplitude of Wj to diverge, and higher-order terms need to be introduced to saturate it. Therefore, the solution of Equation 3.8 is written in the following form:
Substituting formula (3.11) into Eq. 3.8, we get
For the order of ɛ3,
Similarly, use
where
According to the solvability condition of Fredholm, we get
where
Change the subscript to get the other equation. Then, the amplitude Mi can be expressed as follows:
Combine Eqs 3.10, 3.14, 3.15 to get the following amplitude equation:
where
Change the subscript to get the other equation. Then, the amplitude equation of system (2.3) is
3.2 Stability analysis
Each amplitude equation can be expressed into the product of its mode βi = |Mi| and its corresponding phase angle ψi, which is Mi = βi exp (iψi). Substituting it into the amplitude Eq. 3.17, we get
where ψ = ψ1+ψ2+ψ3.
The aforementioned system has four solutions, corresponding to four different pattern structures. Table 2 summarizes the stability of the four pattern structures.
4 Main results
For the study of the spatial model, we cannot use analytical methods to obtain its dynamic behavior. Therefore, in this section, we simulate system (2.3) using the method of numerical simulation and reveal the influence of vegetation hydrotropism on vegetation growth and distribution. The selected area is M × N, where M = N = 100. Its boundary conditions meet Neumann boundary conditions, that is, the study area is not connected with its surrounding environment. We set the time zone to [0,10000], time step to Δt = 0.1, and spatial step to Δh = 1. The initial value is the random disturbance at the equilibrium point E2.
4.1 Basic pattern structures
In this part, the theoretical analysis of the third part is verified by numerical simulation. Choose the values of different parameters a, m, d, d1, and d2. We can calculate the values of h, g1, g2, μ1, μ2, μ3, and μ4, according to the expression of the amplitude equation coefficients in section 3. In order to observe the simulation results, we have selected three sets of parameter values in Table 3, and the corresponding results are shown in Figure 4; among them, Figure 4 (a1)-(c1) is a water pattern and Figure 4 (a2)-(c2) is a vegetation pattern. When the first set of parameters is selected, the value of μ is between μ2 and μ3, and system (2.3) has a dot pattern, as shown in Figure 4 (a1) and Figure 4 (a2); when the second set of parameters is selected, the value of μ is between μ3 and μ4, and system (2.3) has a mixed pattern, as shown in Figure 4 (b1) and Figure 4 (b2); when the third set of parameters is selected, the value of μ is greater than μ4, and system (2.3) has a stripe pattern, as shown in Figure 4 (c1) and Figure 4 (c2).
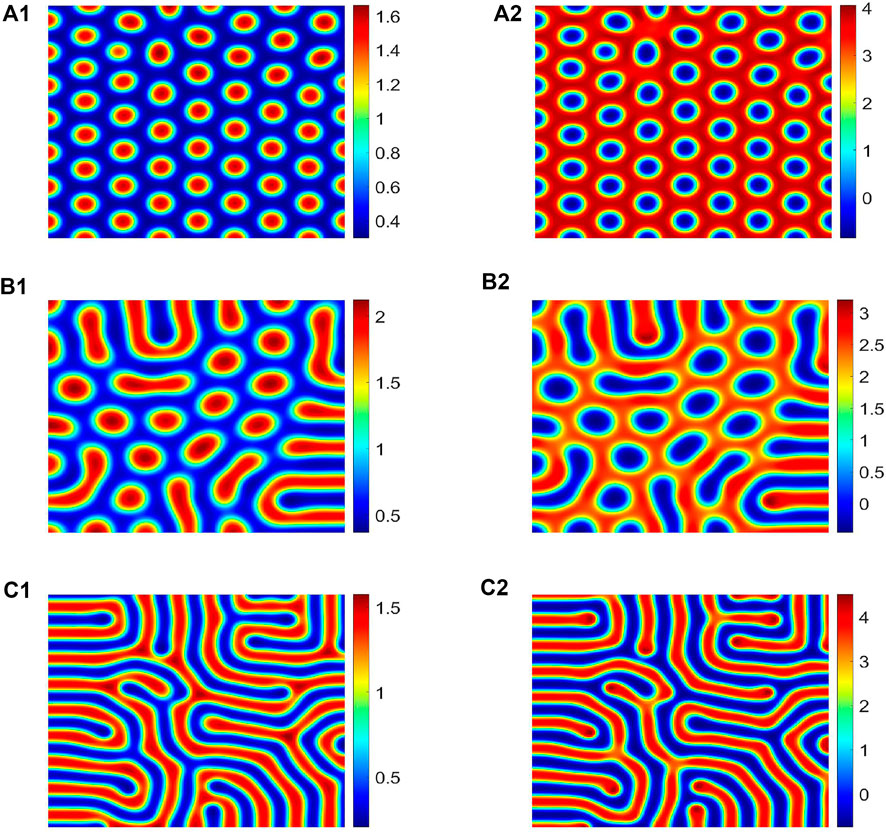
FIGURE 4. For different pattern structures, the parameter values are shown in Table 3. (a1)-(c1) is the water pattern, and (a2)-(c2) is the vegetation pattern. Among them, (a1) and (a2) are spot patterns, (b1) and (b2) are mixed patterns, and (c1) and (c2) are strip patterns.
4.2. Pattern phase transition induced by soil water diffusion
In this section, we will study the influence of soil water diffusion on vegetation in dry and semi-dry places. In these areas, the lack of water resources causes the diffusion of soil water mainly caused by concentration differences, that is, absorption water feedback. For different vegetation, the ability to absorb water is different, which will lead to the concentration difference of soil water. It is reflected in the parameter d2 in our model; the greater the concentration difference of soil water, the greater is the parameter d2. In order to better reveal the effects of different water diffusion intensities on the vegetation pattern, we do not consider the hydrotropism of vegetation and fix the parameters d = 0 and d1 = 1; then, we can change the parameter d2 to reflect different water diffusion intensities.
Figure 5 shows the change in the corresponding vegetation pattern with the change in the parameter d2. With the increase in d2, the vegetation pattern structures change in the following sequence, gap pattern, mixed pattern, strip pattern, and spot pattern. Moreover, we also find that when d2 ≤ d1, no vegetation pattern is generated; when d2 > d1, with the increase in the parameter d2, the gap between the pattern becomes larger and larger. In fact, the difference in the soil water concentration reflects the difference in the water absorption capacity among vegetation. The greater the diffusion intensity of the water beside the vegetation, the stronger is the absorption capacity of the vegetation for water, which will promote its own growth more. Conversely, as the intensity of diffusion of water next to the vegetation increases, more water will flow to its location, which will suppress the growth of the nearby vegetation. It shows that the vegetation pattern is formed through the long-range competition and short-range promotion mechanism.
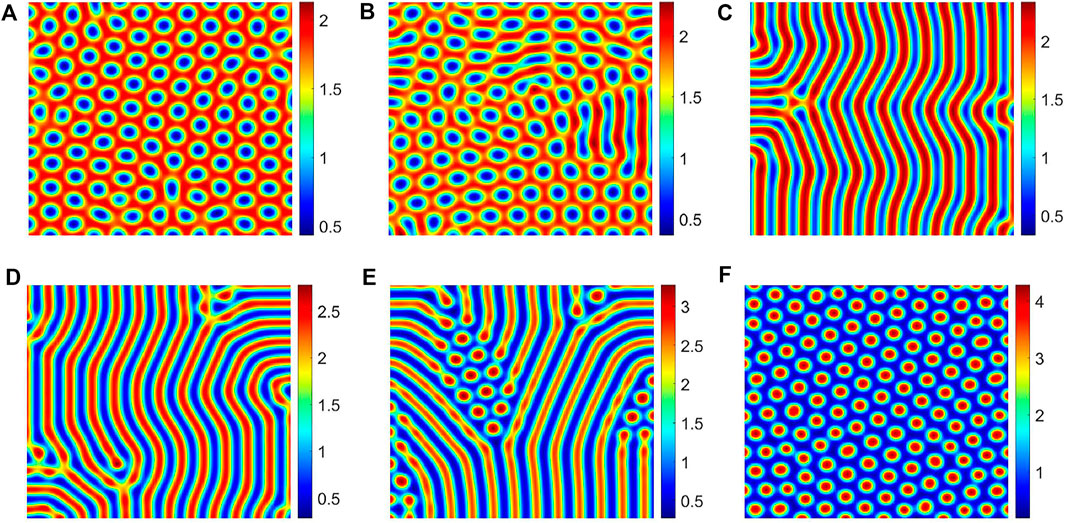
FIGURE 5. Different values of the parameter d2 correspond to different vegetation patterns, (A) d2 = 7.80, (B) d2 = 8.21, (C) d2 = 9.00, (D) d2 = 10.20, (E) d2 = 12.80, and (F) d2 = 15.48. The other parameter values are a = 3.25, m = 1.50, and d1 = 1.00.
4.3 Influence of hydrotropism on the vegetation pattern
Due to the climatic conditions and geographical environment in dry and semi-dry regions, the soil water resource distribution is uneven, and a water gradient is formed. Because of the presence of the moisture gradient, roots exhibit hydrotropism characteristics. For different vegetation, the intensity of the hydrotropism of the vegetation root is different. Some vegetation roots are sensitive to soil moisture, but some vegetation roots are relatively weak. Therefore, in this section, we study the influence of different vegetation growth abilities of water on the vegetation in the area. In our spatial model, we fixed other diffusion parameters, that is, d1 = 1, d2 = 1, and observed the influence of hydrotropism on the vegetation pattern structures by changing the parameter d. Figure 6 shows the pattern structures of vegetation under different root hydrotropism intensities. Through theoretical analysis and numerical simulation, we know that when we do not consider the hydrotropism of vegetation roots in our model, let d1 = d2, in which no vegetation pattern will be generated. Therefore, the hydrotropism of vegetation roots can induce the generation of vegetation patterns.
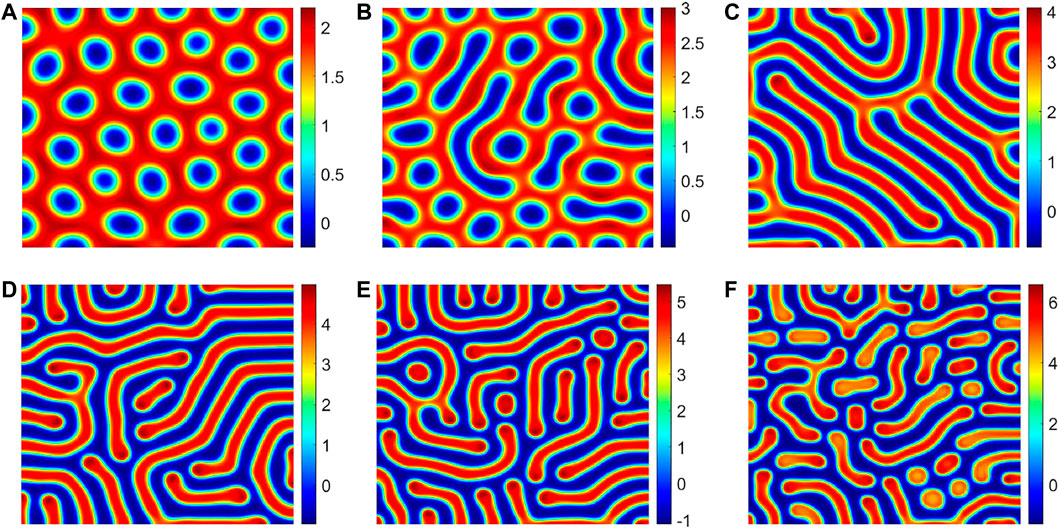
FIGURE 6. Different values of the parameter d correspond to different vegetation patterns, (A) d = 1.52, (B) d = 2.34, (C) d = 3.81, (D) d = 5.86, (E) d = 7.23, and (F) d = 9.56. The other parameter values are a = 3.25, m = 1.5, d1 = 1, and d2 = 1.
In Figure 6, we show the influence of the parameter d on the vegetation pattern structures. When the parameter d is small, the gap pattern appears (Figure 6A). When the parameter d increases slightly, the gap pattern begins to disappear, the strip pattern appears, and the vegetation pattern structure becomes mixed gap and strip pattern structures (Figure 6B). When the parameter d continues to increase, the gap pattern completely disappears, showing the strip pattern structure (Figure 6C). Subsequently, with the increase in the d parameter, the spot pattern gradually appears (Figures 6D,F). To sum up, gradually enhance the hydrotropism effect of roots, then the vegetation pattern’s structure changes in the following sequence: the gap pattern, mixed pattern, strip pattern, and spot pattern. In Figure 7, we show the realistic vegetation distribution corresponding to numerical simulation pattern structures.
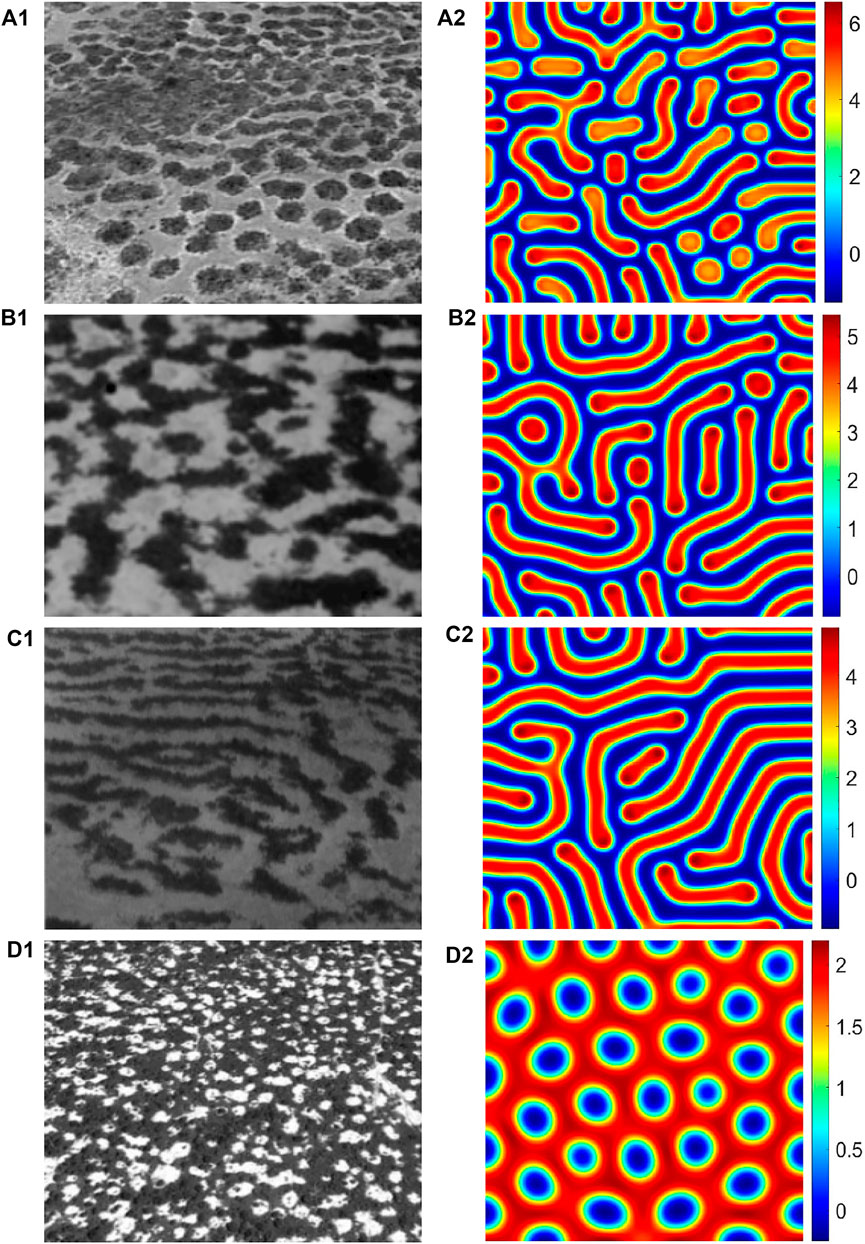
FIGURE 7. Vegetation structure observed in the nature and pattern structure obtained by numerical simulation. Fig (a1-d1) shows realistic vegetation pattern structures: (a1) Zambia, spot vegetation structure [4]; (b1) Niger, mixed vegetation structure [27]; (c1) Niamey and Niger, stripe vegetation structure [12]; and (d1) SW Niger, gap vegetation structure [4]. Fig (a2-d2) shows corresponding numerical simulation patterns.
Moreover, in Figure 8, we show the relationship between the root hydrotropism effect and average vegetation biomass. We can find that with the increasing intensity of the hydrotropism of the vegetation root, the average density of vegetation increases. However, as the intensity of vegetation to grow toward water increases, the interval of plants also becomes larger, which means that the stability of the ecosystem in the region is also reduced.
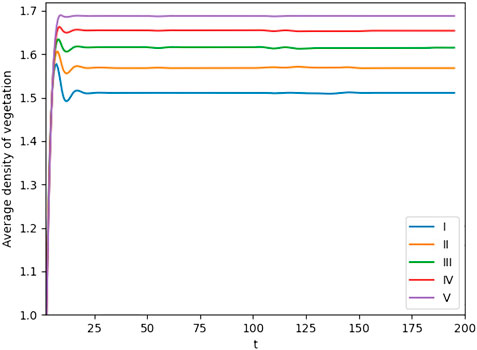
FIGURE 8. Average density of vegetation changes with time t corresponding to different values of the parameter d (I) d = 3.52, (II) d = 4.52, (III) d = 5.52, (IV) d = 6.52, and (V) d = 7.52. The other parameter values are a = 3.25, m = 1.50, d1 = 1.00, and d2 = 1.00.
Owing to the fact that water at the location of the vegetation itself is not enough to meet the needs of its own growth, the vegetation has to extend to the humid area to obtain water resources through the roots, which makes the root system of the vegetation exhibit hydrotropism. For different vegetation, the degree of development of the root system is different. When the root system of vegetation is developed, vegetation has higher hydrotropism effects, which means it can absorb more water and other vegetation cannot obtain enough water resources because of limited water resources in these dry regions. A large amount of vegetation died due to the lack of water, resulting in vegetation degradation. In other words, increasing the intensity of the hydrotropism of roots in a range can increase the average biomass of vegetation. However, excessively increasing the intensity of the hydrotropism of roots will lead to desertification.
5 Conclusion
In our paper, we mainly analyze spatial dynamics with hydrotropism effects. First, on the basis of Klausmeier’s system, a spatial system is established considering the hydrophilic effect. Then, the existence and stability of the system equilibrium point are analyzed. Second, through the method of multi-scale analysis, the amplitude equation of the system and its coefficients are derived [38–40]. Finally, we numerically simulated the system to show the influence of vegetation root hydrotropism on vegetation growth distribution.
Bases on the numerical results, we find that the hydrotropism of vegetation roots can induce pattern generation. Furthermore, with the enhancement of the root hydrotropism effect, pattern structures change as follows: the spot pattern, mixed pattern, strip pattern, and gap pattern. Moreover, we also study the relationship between the root hydrotropism effects and average vegetation biomass. We find that increasing the intensity of the hydrotropism of roots in a range can increase the average vegetation biomass which is consistent with the results in [35, 41]. However, excessively increasing the strength of root hydrotropism, the spacing between the vegetation pattern also increases, which means that the ecosystem stability is reduced and prone to land desertification.
We all know that many factors will affect vegetation distribution in the real world, including climatic conditions (such as temperature, light, and carbon dioxide concentration), topographic conditions (such as mountains and plains), and human activities (such as deforestation and grazing) [41–50]. However, in our system, we only consider rainfall and vegetation root hydrotropism effects. Therefore, in order to better protect vegetation, prevent land desertification, and improve the stability of the ecosystem, we hope to establish a more realistic vegetation dynamic system, including climatic conditions, internal growth mechanism of vegetation, human activities, and other factors, to better study the dynamic mechanism of spatial vegetation and reveal relevant factors affecting the structure of the vegetation pattern.
Data availability statement
The original contributions presented in the study are included in the article/Supplementary Material; further inquiries can be directed to the corresponding author.
Author contributions
All authors have made great contributions to the writing of the study and approved the submitted version. LL, F-LW, and L-FH established dynamical modeling, participated in the program design, and provided valuable comments on the manuscript writing.
Funding
The research was supported by the National Key Research and Development Program of China (2018YFE0109600) and the National Natural Science Foundation of China (Nos. 42275034 and 42075029).
Conflict of interest
The authors declare that the research was conducted in the absence of any commercial or financial relationships that could be construed as a potential conflict of interest.
Publisher’s note
All claims expressed in this article are solely those of the authors and do not necessarily represent those of their affiliated organizations, or those of the publisher, the editors, and the reviewers. Any product that may be evaluated in this article, or claim that may be made by its manufacturer, is not guaranteed or endorsed by the publisher.
References
1. Jones CG, Lawton JH, Shachak M. Organisms as ecosystem engineers. In: Ecosystem management. Berlin, Germany: Springer (1994). p. 130–47. doi:10.1007/978-1-4612-4018-1_14
2. Jones CG, Lawton JH, Shachak M. Positive and negative effects of organisms as physical ecosystem engineers. Ecology (1997) 78(7):1946–57.
3. Shi C, Zhou Y, Fan X, Shao W. A study on the annual runoff change and its relationship with water and soil conservation practices and climate change in the middle yellow river basin. Catena (2013) 100:31–41.
4. Wright JP, Jones CG. The concept of organisms as ecosystem engineers ten years on: Progress, limitations, and challenges. BioScience (2006) 56(3):203–9. doi:10.1641/0006-3568(2006)056[0203:tcooae]2.0.co;2
5. D’Odorico P, Bhattachan A, Davis KF, Ravi S, Runyan CW. Global desertification: Drivers and feedbacks. Adv Water Resour (2013) 51:326–44.
6. Sun GQ, Li L, Li L, Liu C, Wu YP, Gao S, et al. Impacts of climate change on vegetation pattern: Mathematical modeling and data analysis. Phys Life Rev (2022) 43:239–70. doi:10.1016/j.plrev.2022.09.005
7. Wang Q, Takahashi H. A land surface water deficit model for an arid and semiarid region: Impact of desertification on the water deficit status in the loess plateau, China. J Clim (1999) 12(1):244–57. doi:10.1175/1520-0442-12.1.244
8. Saco PM, Willgoose GR, Hancock GR. Eco-geomorphology of banded vegetation patterns in arid and semi-arid regions. Hydrol Earth Syst Sci (2007) 11(6):1717–30. doi:10.5194/hess-11-1717-2007
9. Sherratt JA. An analysis of vegetation stripe formation in semi-arid landscapes. J Math Biol (2005) 51(2):183–97. doi:10.1007/s00285-005-0319-5
10. Sun GQ, Wang CH, Chang LL, Wu YP, Li L, Jin Z. Effects of feedback regulation on vegetation patterns in semi-arid environments. Appl Math Model (2018) 61:200–15.
11. Sherratt JA, Synodinos AD. Vegetation patterns and desertification waves in semi-arid environments: Mathematical models based on local facilitation in plants. DCDS-B (2012) 17(8):2815–27. doi:10.3934/dcdsb.2012.17.2815
12. Klausmeier CA. Regular and irregular patterns in semiarid vegetation. Science (1999) 284(5421):1826–8. doi:10.1126/science.284.5421.1826
13. von Hardenberg J, Meron E, Shachak M, Zarmi Y. Diversity of vegetation patterns and desertification. Phys Rev Lett (2001) 87(19):198101. doi:10.1103/physrevlett.87.198101
14. Gilad E, von Hardenberg J, Provenzale A, Shachak M, Meron E. A mathematical model of plants as ecosystem engineers. J Theor Biol (2007) 244(4):680–91. doi:10.1016/j.jtbi.2006.08.006
15. van der Stelt S, Doelman A, Geertje H, Jens Rademacher DM. Rise and fall of periodic patterns for a generalized klausmeier–gray–scott model. J Nonlinear Sci (2013) 23(1):39–95.
16. Zelnik YR, Meron E, Bel G. Gradual regime shifts in fairy circles. Proc Natl Acad Sci U.S.A (2015) 112(40):12327–31. doi:10.1073/pnas.1504289112
17. Bo TL, Fu LT, Zheng XJ. Modeling the impact of overgrazing on evolution process of grassland desertification. Aeolian Res (2013) 9:183–9. doi:10.1016/j.aeolia.2013.01.001
18. Chen Z, Wu YP, Feng GL, Qian ZH, Sun GQ. Effects of global warming on pattern dynamics of vegetation: Wuwei in China as a case. Appl Maths Comput (2021) 390:125666. doi:10.1016/j.amc.2020.125666
19. Li L, Sun GQ, Guo ZG. Bifurcation analysis of an extended Klausmeier-Gray-Scott model with infiltration delay. Stud Appl Math (2022) 148:1519–42. doi:10.1111/sapm.12482
20. Li L, Sun GQ, Jin Z. Interactions of time delay and spatial diffusion induce the periodic oscillation of the vegetation system. DCDS-B (2022) 27:2147–72. doi:10.3934/dcdsb.2021127
21. Liu C, Li L, Wang Z, Wang R. Pattern transitions in a vegetation system with cross-diffusion. Appl Maths Comput (2019) 342:255–62. doi:10.1016/j.amc.2018.09.039
22. Sherratt JA, Lord GJ. Nonlinear dynamics and pattern bifurcations in a model for vegetation stripes in semi-arid environments. Theor Popul Biol (2007) 71(1):1–11. doi:10.1016/j.tpb.2006.07.009
23. Sun GQ, Zhang HT, Song YL, Li L, Jin Z. Dynamic analysis of a plant-water model with spatial diffusion. J Differential Equations (2022) 329:395–430. doi:10.1016/j.jde.2022.05.009
24. Tarnita C, Bonachela JA, Sheffer E, Guyton JA, Coverdale TC, Long RA, et al. A theoretical foundation for multi-scale regular vegetation patterns. Nature (2017) 541(7637):398–401. doi:10.1038/nature20801
25. Xue Q, Sun GQ, Liu C, Guo Z, Jin Z, Wu YP, et al. Spatiotemporal dynamics of a vegetation model with nonlocal delay in semi-arid environment. Nonlinear Dyn (2020) 99(4):3407–20. doi:10.1007/s11071-020-05486-w
26. Chen S, Li J, Wang S, Fritz E, Hüttermann A, Altman A. Effects of nacl on shoot growth, transpiration, ion compartmentation, and transport in regenerated plants of populus euphratica and populus tomentosa. Can J For Res (2003) 33(6):967–75. doi:10.1139/x03-066
27. Lin H, Chen Y, Zhang H, Fu P, Fan Z. Stronger cooling effects of transpiration and leaf physical traits of plants from a hot dry habitat than from a hot wet habitat. Funct Ecol (2017) 31(12):2202–11. doi:10.1111/1365-2435.12923
28. Hille Ris Lambers R, Rietkerk M, van den Bosch F, Prins HHT, de Kroon H. Vegetation pattern formation in semi-arid grazing systems. Ecology (2001) 82(1):50–61. doi:10.1890/0012-9658(2001)082[0050:vpfisa]2.0.co;2
29. Takahashi H. Hydrotropism: The current state of our knowledge. J Plant Res (1997) 110(2):163–9. doi:10.1007/bf02509304
30. Takahashi H, Miyazawa Y, Fujii N. Hormonal interactions during root tropic growth: Hydrotropism versus gravitropism. Plant Mol Biol (2009) 69(4):489–502. doi:10.1007/s11103-008-9438-x
31. Takahashi N, Yamazaki Y, Kobayashi A, Higashitani A, Takahashi H. Hydrotropism interacts with gravitropism by degrading amyloplasts in seedling roots of arabidopsis and radish. Plant Physiol (2003) 132(2):805–10. doi:10.1104/pp.018853
32. Gómez-Plaza A, Martınez-Mena M, Albaladejo J, Castillo VM. Factors regulating spatial distribution of soil water content in small semiarid catchments. J Hydrol (2001) 253(1-4):211–26. doi:10.1016/s0022-1694(01)00483-8
33. Wu GL, Zhang ZN, Wang D, Shi ZH, Zhu YJ. Interactions of soil water content heterogeneity and species diversity patterns in semi-arid steppes on the loess plateau of China. J Hydrol (2014) 519:1362–7. doi:10.1016/j.jhydrol.2014.09.012
34. Dietrich D. Hydrotropism: How roots search for water. J Exp Bot (2018) 69(11):2759–71. doi:10.1093/jxb/ery034
35. Liu C, Wang FG, Xue Q, Li L, Wang Z. Pattern formation of a spatial vegetation system with root hydrotropism. Appl Maths Comput (2022) 420:126913. doi:10.1016/j.amc.2021.126913
36. Miyazawa Y, Takahashi A, Kobayashi A, Kaneyasu T, Fujii N, Takahashi H. GNOM-mediated vesicular trafficking plays an essential role in hydrotropism of arabidopsis roots. Plant Physiol (2009) 149(2):835–40. doi:10.1104/pp.108.131003
37. Wang X, Zhang G. Vegetation pattern formation in seminal systems due to internal competition reaction between plants. J Theor Biol (2018) 458:10–4. doi:10.1016/j.jtbi.2018.08.043
38. Guo ZG, Sun GQ, Wang Z, Jin Z, Li L, Li C. Spatial dynamics of an epidemic model with nonlocal infection. Appl Maths Comput (2020) 377:125158. doi:10.1016/j.amc.2020.125158
39. Liang J, Liu C, Sun GQ, Li L, Zhang L, Hou M, et al. Nonlocal interactions between vegetation induce spatial patterning. Appl Maths Comput (2022) 428:127061. doi:10.1016/j.amc.2022.127061
40. Liu QX, Herman MJ, Mooij WM, Huisman J, Scheffer M, Olff O, et al. Pattern formation at multiple spatial scales drives the resilience of mussel bed ecosystems. Nat Commun (2014) 5(1):5234–7. doi:10.1038/ncomms6234
41. Xue Q, Liu C, Li L, Sun GQ, Wang Z. Interactions of diffusion and nonlocal delay give rise to vegetation patterns in semi-arid environments. Appl Maths Comput (2021) 399:126038. doi:10.1016/j.amc.2021.126038
42. Baldi G, Verón SR, Jobbágy EG. The imprint of humans on landscape patterns and vegetation functioning in the dry subtropics. Glob Change Biol (2013) 19(2):441–58. doi:10.1111/gcb.12060
43. Bonachela JA, Pringle RM, Sheffer E, Coverdale TC, Guyton JA, Caylor K, et al. Termite mounds can increase the robustness of dryland ecosystems to climatic change. Science (2015) 347(6222):651–5. doi:10.1126/science.1261487
44. Kéfi S, Rietkerk M, Alados AL, Pueyo Y, Papanastasis VP, ElAich E, et al. Spatial vegetation patterns and imminent desertification in mediterranean arid ecosystems. Nature (2007) 449(7159):213–7. doi:10.1038/nature06111
45. Kefi S, Rietkerk M, Katul GG. Vegetation pattern shift as a result of rising atmospheric co2 in arid ecosystems. Theor Popul Biol (2008) 74(4):332–44. doi:10.1016/j.tpb.2008.09.004
46. Lawley V, Parrott L, Lewis MR, Sinclair B, Ostendorf B. Self-organization and complex dynamics of regenerating vegetation in an arid ecosystem: 82 years of recovery after grazing. J Arid Environments (2013) 88:156–64. doi:10.1016/j.jaridenv.2012.08.014
47. Marasco A, Iuorio A, Cartení F, Bonanomi G, Tartakovsky MT, Mazzoleni S, et al. Vegetation pattern formation due to interactions between water availability and toxicity in plant-soil feedback. Bull Math Biol (2014) 76(11):2866–83. doi:10.1007/s11538-014-0036-6
48. Meron E, Gilad E, von Hardenberg J, Shachak M, Zarmi Y. Vegetation patterns along a rainfall gradient. Chaos, Solitons & Fractals (2004) 19(2):367–76. doi:10.1016/s0960-0779(03)00049-3
49. Sun GQ, Zhang HT, Wang JS, Li L, Wang Y, Li L, et al. Mathematical modeling and mechanisms of pattern formation in ecological systems: A review. Nonlinear Dyn (2021) 104(2):1677–96. doi:10.1007/s11071-021-06314-5
Keywords: vegetation–water model, Turing pattern, multi-scale analysis, vegetation hydrotropism, desertification
Citation: Li L, Wang F-G and Hou L-F (2023) Rich dynamics of a vegetation–water system with the hydrotropism effect. Front. Phys. 10:1084142. doi: 10.3389/fphy.2022.1084142
Received: 30 October 2022; Accepted: 28 November 2022;
Published: 04 January 2023.
Edited by:
Ling Xue, Harbin Engineering University, ChinaReviewed by:
Sanling Yuan, University of Shanghai for Science and Technology, ChinaJie Lou, Shanghai University, China
Copyright © 2023 Li, Wang and Hou. This is an open-access article distributed under the terms of the Creative Commons Attribution License (CC BY). The use, distribution or reproduction in other forums is permitted, provided the original author(s) and the copyright owner(s) are credited and that the original publication in this journal is cited, in accordance with accepted academic practice. No use, distribution or reproduction is permitted which does not comply with these terms.
*Correspondence: Li Li, bGlsaTgzMTExM0BzeHUuZWR1LmNu