- 1School of Economics, Faculty of Economics and Management, East China Normal University, Shanghai, China
- 2School of Economics, Faculty of Economics, Renmin University of China, Beijing, China
- 3School of Economics and Finance, South China University of Technology, Guangzhou, China
The outbreak of COVID-19 had a huge impact on the financial market. As a new growth point in China, it’s necessary to study how SMEs (small and medium-sized enterprises) represented by listed companies on the GEM (growth enterprise market) can withstand sudden shocks. This paper examines the impact of Wuhan’s COVID-19 lockdown on the financial markets based on the data of GEM listed companies and the method of event analysis. The results show that investors had a great response to epidemic related news. Compared with the interest rate cut policy, the targeted RRR reduction policy had a more significant positive influence on the financial markets. Furthermore, in the early stages of COVID-19, there was not a significant effect of distance on the firms’ CARs (cumulative abnormal returns). In an improving epidemic environment, the farther the firms were from Wuhan City, the more positive the impact on their CARs would be. This paper provides new evidence and important enlightenment for preventing the impact of public health emergencies on the GEM market and highlights the significance of developing digital inclusive finance, which can mitigate regional risk and financing issues.
1 Introduction
The COVID-19 epidemic broke out in Wuhan at the beginning of 2020. China managed to control the epidemic with its 2-month economic stagnation. However, enterprise, a key pillar of the national economy, had weathered tough times. Despite the medical pharmaceutical industry, which was directly related to epidemic prevention and control, the vast majority of enterprises could not operate normally. China’s GDP fell 6.8% year-on-year in the first quarter of 2020, according to the National Bureau of Statistics. Figure 1 illustrates the annual growth rate of China’s sub-industrial GDP from March 2018 to September 2020, and the changes of China’s macroeconomic indicators from November 2019 to October 2020. As can be seen from Figure 1, the COVID-19 epidemic had a huge impact on a variety of industries in China. A sharp drop in investment in real estate, fixed assets, and social consumer goods retail was also observed with the increase in newly confirmed COVID-19 cases.
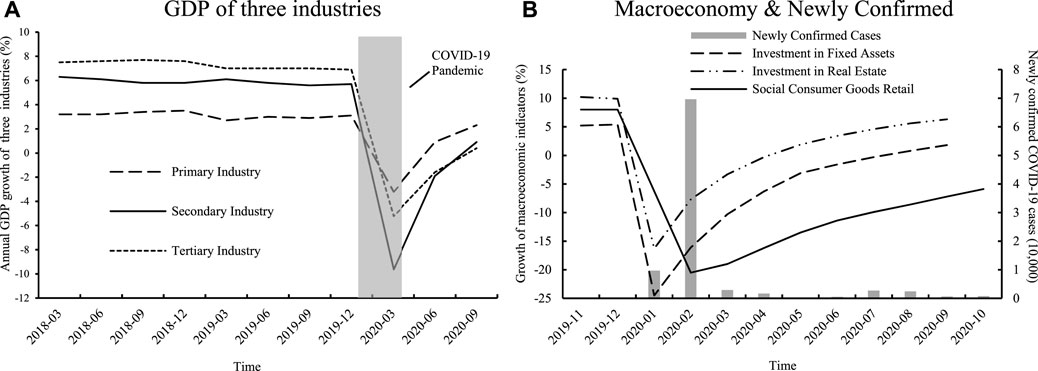
FIGURE 1. The situation of the national economy during COVID-19. Notes: In this figure, the vertical axis indicates annual growth rate of GDP in three industries (including primary industry, secondary industry and tertiary industry), annual growth rate of macroeconomic indicators (including investment in fixed assets, investment in real estate and social consumer goods retail) and newly confirmed cases of COVID-19. The horizontal axis presents the time from March 2018 to September 2020 and the time from November 2019 to October 2020. (A) GDP of three industries (B) Macroeconomy and Newly Confirmed.
A number of policies were issued by the State Council and People’s Bank of China. These include tax reductions and exemptions, reductions of reserve ratios and interest rates, all aimed at encouraging enterprises to resume work and production and boost economic growth. At the same time, fiscal and monetary policies continued to make efforts, and domestic enterprises’ resumption of work and production achieved remarkable results. According to the National Bureau of Statistics, China’s GDP grew by 3.2% year-on-year and 11.5% quarter-on-quarter in the second quarter of 2020, with significant economic growth in high-tech manufacturing, modern services and Internet retail. China is reported to be the only major economy in the world to achieve positive growth in 2020, with a growth rate of 2.3 percent.1
It is no denying that contagion effects have always been the focus of human disease and health network research [1-2] empirically analyzed three types networks of disease transmission, namely, temporary networks, static networks, and a fully connected topology. Results showed that time structure can affect disease transmission more than static network structure. Also, complex networks are always used to describe the spread of major disease in populations of interacting individuals [3]. Furthermore, considering that social activities and infectivity are time phenomena, [4]. Believed that when these two processes are synchronized, diseases are most likely to spread. It can be seen that the existence of contagion networks and social networks will aggravate the impact of epidemics on the whole society. What’s more, maintaining stable economic operation and active capital market is an important issue for a country to be concerned about, so the economic consequences of the epidemic need to be studied. Especially in the context of highly contagion during the incubation period, if there was no interference with any physical restrictions, the embedding of social network into life and business would lead to the spread of the contagion network, causing secondary damage.
The COVID-19 epidemic had several significant characteristics, such as suddenness, urgency, uncertainty, and unpredictable nature [5], which had a huge negative impact on the economy and social stability, especially the financial market [6]. Many of the existing studies about COVID-19 epidemics followed the ideas of SRAS, which focused on national economy and industrial economy in the long term. Through quantitative and empirical analysis, [7] examined the effects of COVID-19 on SMEs. Nevertheless, the epidemic was a widespread malignant public health emergency, causing a great deal of public concern. They did not examine the huge impact of COVID-19 on investor sentiment and financial markets. A second large group of recently emerging literature focused on the financial markets. And they consistently showed that stock markets around the world have reacted to the epidemic with strong negative yields [8–11]. For instance, [10] examined the data of ten stock markets and found that the market reaction caused by COVID-19 did not seem to be a recession but rather a stagnation. [8] found the share prices of all firms in China declined due to the expected adverse economic outcomes of COVID-19. There is however no special attention given to Chinese growth enterprise market. Against the background of mass entrepreneurship and mass innovation in China, the innovative SMEs represented by GEM’s listed companies are accelerating to become the new economic growth point in China. In addition, the impact of the epidemic on innovative SMEs during the growth period was more severe than the impact on other firms. With the yield on GEM as the starting point, we can show the overall situation of SMEs’ survival under the epidemic.
By using the data of GEM listed companies from 18 June 2019 to 20 June 2020, we examine the performance of the medical and pharmaceutical industries as well as the non-medical and non-pharmaceutical industry stock yields during the epidemic through event analysis. Furthermore, we study how the direct distance between the business location of firms and Wuhan City affects the CARs (cumulative abnormal returns) under four events. This study will answer four questions: Firstly, whether the impact of epidemic-related events and monetary policies on the GEM is consistent with expectations during the outbreak of COVID-19. Secondly, whether there is industry heterogeneity in the above studies. Additionally, in the early stages of an outbreak, whether distances between firms and Wuhan City have an adverse effect on the CAR. Finally, whether digital inclusive finance can contribute to mitigating the effects of the COVID-19 epidemic on the financial markets.
We offer at least three contributions to the existing literature. Firstly, we focus on the growth enterprise market in China. Studies that examined the impact of the COVID-19 epidemic on China’s economy tended to concentrate on long-term macroeconomic effects, and few studies considered the particularities of GEM policy. In addition, research about GEM focused primarily on the prospects of the listed companies in GEM, ignoring their substantial risk-resistance capabilities. In light of the COVID-19 epidemic, we analyze the GEM market’s response and fluctuation. Secondly, we provide a complementary analysis to recent studies that examined government involvement in COVID-19. [9] used the daily data of 77 countries to assess the economic impact of various government interventions, such as social distancing measures, public awareness campaigns, testing policies, etc. We contribute to the existing literature by studying the effects of monetary policy implementation on the financial markets. Furthermore, as the result of the ongoing technological revolution driven by the explosion of information technology, the development of digital inclusive finance contributes to the economic recovery during these difficult times. It is also pertinent for scholars to examine the relationship between the COVID-19 epidemic and digital inclusive finance. We integrate digital inclusive finance into the analysis framework. In this way, it is explored whether digital inclusive finance can contribute to mitigating the negative impact on the financial markets.
2 Literature review
2.1 Public health emergency and macroeconomy
Studies have shown that public emergencies negatively affect a country’s economy over the short and long term [12,13]. Epidemics, a kind of public health emergencies, however, also have unique adverse effects when compared to other public emergencies.
First of all, public health emergencies have a wide range of implications. In general, natural disasters and man-made disasters have local impacts, affecting mostly a few cities, provinces, or regions. However, epidemic diseases have the potential to spread everywhere, impacting the economies of a number of countries and regions. According to [14], the epidemic may cause a self-reinforcing panic and long-term economic weakness. Additionally, evidence from the Ebola virus in West Africa shows that public health emergencies can have a more long-term impact on developing countries [15]. Through the Growth at Risk (GaK), [16] analyzed how financial risk impacts economic growth under COVID-19. The impact of COVID-19 was mainly reflected in the negative impact of short-term economic growth, whereas its long-term impact was relatively small.
Further, public health emergencies hinder the flow of factors, especially labor factors. The most effective way to deal with the epidemic is to restrict people’s movement and impose physical quarantine. As a result, people and businesses have difficulty getting to work. In particular, the epidemic will be a life-or-death challenge for industries that require a lot of human capital. [17] evaluated the short-term effects of the new H1N1 epidemic on the tourism and aviation industries in Mexico, and concluded that the aviation industry was more vulnerable to high-intensity negative impacts. Due to the COVID-19 epidemic, [18] also believed that the catering, tourism, transportation, entertainment, and manufacturing industries had suffered huge losses. Stagflation in some parts of the manufacturing supply chain caused an asymmetric demand imbalance between upstream and downstream, resulting in serious economic consequences.
2.2 Public health emergency and microeconomy
The impact of public emergencies on the economy is multilevel [19], which can not only have a huge impact on the long-term and stable operation of a country’s economy, but also have a negative impact on enterprises, financial markets and individual behaviors.
From the perspective of enterprises, public health emergencies will affect the normal operation of enterprises in the short term. In comparison to other enterprises, SMEs are more sensitive to public emergencies. Due to the size of SMEs, [20] believed that they were not well equipped to resist risks and to repair themselves. Therefore, public health emergencies have a greater impact on start-ups, which are more likely to be exposed to bankruptcy risk in a crisis [21]. In addition, compared with large enterprises, for a long time, SMEs are often faced with loan difficulties and financing difficulties. In other words, they are more in need of financial support in case of public emergency, but it is also more difficult for them to obtain financial support. Additionally, [22] believed that banks tended to face liquidity crunch and other problems in the context of public emergencies that may lead to economic recession. To adjust their balance sheets, they were more inclined to tighten credit to SMEs, which also increased the operating costs for enterprises in a crisis.
In terms of public emergencies, not only do they depend on objective characteristics of the event, but also on the response of society [23], so the financial market is highly likely to respond to unpredictable emergencies [24]. According to [25] and [26], public emergencies were a systematic risk and could affect the stock yield. [27] and [28] all pointed out that the COVID-19 case could arouse a significant increase in financial market volatility. Moreover, [29] found that overwhelming panic generated by the news media increased equity market volatility. Furthermore, most studies employ the event analysis method to analyze the impact of special events on the financial markets when faced with natural disasters, man-made disasters and public health emergencies. As an example, [30] investigated 43 insurance loss events and found that insurance managers receive abnormal returns on the day of the event. Under some special events, [31], [32], [33], and [34] also used event analysis to investigate share prices, spillover effects, and trade effects in financial markets.
As far as individual behavior is concerned, public health emergencies will likely cause widespread panic, accompanied by a herd effect. According to [35] and [36], vicious public emergencies can easily cause panic among investors and change their trading behavior. During the 2003 SARS epidemic in China, [37] studied the influence of rumors on individual economic behavior. They find that rumors were more frequent in hard-hit areas and triggered a number of knock-on effects, such as herd behavior of buying.
2.3 Summary
It is generally agreed that public emergencies can negatively impact a country’s long-term macroeconomics and short-term financial markets. Among them, public health emergencies are further clarified as being distinct from other kinds of emergency. Furthermore, the emerging literature about the impact of COVID-19 in China is primarily a descriptive summary of the great changes in national economy from a qualitative point of view, as well as suggestions and perspectives for future long-term public governance and policy formulation. Meanwhile, a small number of studies use micro-data to investigate the current state and policy demands of SMEs. Few studies, however, have considered how Chinese growth enterprise market responded to the epidemic news and monetary policies during COVID-19. Also, during the period of the epidemic, the strong development of digital inclusive finance ensures many conveniences and supports economic recovery under the new technological revolution, with artificial intelligence and digital technology at the forefront. In this light, it is also important to consider how to view the relationship between COVID-19 and digital inclusive finance.
3 Basic facts about the epidemic
3.1 Impact of COVID-19 epidemic on Chinese growth enterprise market
In Figure 2, we show the weighted yield on the GEM from 1 July 2019 to 30 June 2020. A shadow region presents the movement of the GEM since the epidemic outbroke. Compared with before the outbreak, the range and volatility of yields are much wider. The yield suffered several large fluctuations, once falling as low as −7.49%.2 On the day Wuhan was officially closed down, the yield dropped from 1% to nearly -8%. It is clear that the outbreak of COVID-19 was a huge blow to China’s growth enterprise market.
3.2 Monetary policies during COVID-19 epidemic
In response to COVID-19, People’s Bank of China issued monetary policies to stabilize the money supply and maintained the country’s economic system. As shown in Figure 3, China’s money supply changed at various levels between November 2019 and September 2020. Before the outbreak, the money supply grew between 2% and 4% annually. When the outbreak occurred, M1’s annual growth rate decreased suddenly, and then gradually picked up. Also, M2’s growth rate became higher than before the epidemic. All evidence shows that the measures taken by People’s Bank of China during the epidemic were successful in recovering the national economy.
4 The GEM’s response to the epidemic
4.1 Empirical strategy
According to [34], we use event analysis to study the impact of COVID-19 epidemic on the GEM listed companies. ARs (abnormal returns) and CARs (cumulative abnormal returns) of the GEM listed companies were also calculated in accordance with [38]. For calculating ARs of stock
First of all, using the daily trading data of individual stocks, we calculate the daily stock yields according to Eq. 1:
Where
Secondly, we estimate the model parameters
Among them,
Finally, Eq. 3 is used to calculate
In order to examine the GEM’s responds to the epidemic news and monetary policies, four event days are selected. Event dates are determined on the basis of official media bulletins and reports from the official website of People’s Bank of China, the Chinese government website and XINHUANET. In the case of the lockdown of Wuhan City, 23 January 2020 is taken as the first event. Table 1 shows the description of four event days.3
[39] pointed out that choosing an estimate window with a time span of more than 100 days can guarantee the accuracy of parameter estimation. According to previous studies, the estimated window spans 100–300 days. Therefore, we select the time span from the first 150 days to the first 30 days of the event, a total of 120 days for the estimation window. Four event windows are included, that is,
4.2 Responds to epidemic events
In Table 2 shows the GEM’s respond to the “Bad News” (lockdown of Wuhan City). There was a significant negative impact on GEM’s share price due to “Bad News”. The reason is that measures like city closures, market strikes, household closures, and gathering bans were widely used to prevent the spread of COVID-19. Consequently, most industries in China suffered a huge blow as these measures increased transportation costs and reduced consumer demand. As for the medical and pharmaceutical industries, the CARs of GEM listed companies were significantly positive. In the epidemic, medical and pharmaceutical companies contributed greatly to the production of epidemic prevention products. They tried their best to meet consumers’ demands for medical and pharmaceutical products. This gave investors a positive signal that this industry met an excellent opportunity for growth during the recession.
Table 3 presents the GEM’s respond to the “Good News” (improving situation). A significant positive effect of “Good News” on the share price of GEM can be seen in the results. In the non-medical and non-pharmaceutical industries, the positive CARs all passed the significance level test of 1%. “Good News” indicated that the situation of the epidemic was gradually improving, and investors’ confidence in the stock market was gradually increasing, which was why funds flowed into the GEM. As for the medical and pharmaceutical industries, “Good News” had a significant negative impact on share prices. Considering that investors’ mood toward the medical pharmaceutical industry had been on the rise since the outbreak, “Good News” could release increased demand for medical products, leading investors to sell some of the medical pharmaceutical stocks, resulting in a decline in the share prices of medical pharmaceutical companies.
Table 4 shows the GEM’s respond to the “Rate Cut”. Surprisingly, “Rate Cut” had a negative impact on the GEM overall. However, this policy could lead positive effects to the GEM medical and pharmaceutical industries to some degree. To sum up, we find that the interest rate cut policy had not much positive impact on innovative SMEs. In fact, this problem was homogenous with SMEs’ financing difficulty. The SMEs were not capable of dealing with bank financing evaluations and were unable to effectively enjoy the policy support of People’s Bank of China. Due to this, the interest rate cut policy were difficult to apply to all types of small businesses.
Table 5 shows the GEM’s respond to the “RRR Reduction”. Within 1 week of implementing the RRR reduction policy, except for the event window [0,3], the CARs passed the significance level test of 5%, indicating that the targeted RRR reduction policy for inclusive finance had a positive impact on GEM. Compared with the interest rate cut policy, the RRR reduction policy was more effective for innovative SMEs. SMEs were more likely to raise funds and get financial support to tide over the epidemic because of the RRR reduction policy, which expanded the credit scale of commercial banks.
5 Distance from Wuhan City and market responds
As the epidemic was highly contagious and physical prevention measures were more effective than others, the distance from the epidemic center would seriously affect the development of enterprises. Wuhan lockdown would cause the enterprise upstream and downstream supply chain fracture and other serious economic consequences. Therefore, this section further studies the impact of distance from Wuhan on the response of GEM listed companies. Considering that the monetary policies were issued by the People’s Bank of China, which was radiated throughout the country and less restricted by geographical location, this section only discusses the epidemic events, namely Event 1 (“Bad News”) and Event 2 (“Good News”).
5.1 Empirical strategy
5.1.1 Model
We establish the following regression model:
Where explained variable
5.1.2 Data
This paper focuses on Growth Enterprise Market listed companies. Basic information and financial data are retrieved from the CSMAR database, while share price data is gathered from the Wind database. Data is collected from 18 June 2019 to 7 April 2020. Also, the GEM listed companies with insufficient stock data, suspended for more than 3 months, with updated listings, or suspended longer than 3 months are excluded. A total of 764 GEM listed companies are obtained. According to the latitude and longitude data, we manually calculate the distance between firms and Wuhan City.
Following the Guidelines on Industry Classification of Listed Companies (Revised in 2012), we initially classify pharmaceutical manufacturing, scientific research, and health industry firms as medical and pharmaceutical industries. Furthermore, firms are manually divided according to the scope of their business, with 84 in the medical and pharmaceutical industry and 680 in the non-medical and non-pharmaceutical industry.
We determine the level of digital inclusive finance in each firm’s business location using the 2018 Digital Financial Index. Following that, we divide the firms by their median index into two groups. A total of 398 companies are classified as having a digital inclusive finance level above the median level, and 341 companies are classified as having a digital inclusive finance level below the median level.
The business scope information of all GEM listed companies is manually gathered. Businesses involved in trade business are classified as those with the word “import” “export”, “trade” or other related words in their business scope. The total number of firms involved in trade business is 380, and 359 are not.
The descriptive statistics of the main variables are shown in Table 6.
5.2 Baseline regression results
The baseline regression results are presented in Table 7. In Columns (1) and (2), the dependent variables are the CARs from Event 1, the lockdown of Wuhan City. The effect of distance on the CARs from Event 1 is not significant. This finding contradicts the view that companies at the epicenter of the outbreak were hard hit by COVID-19. This may be because, as a major public health emergency, the COVID-19 epidemic had a wide radiation range. The outbreak of epidemic had a serious impact on the overall economic trend of the whole country, causing investors to be indiscriminately negative about firms across the whole country. Therefore, in the early stage of the outbreak, whether the firm is located far away from Wuhan City or not, it suffered a severe economic impact.
Columns (3) and (4) examine the CARs from Event 2, the announcement that the epidemic situation was improving. The longer the distance between firms’ business locations and Wuhan City, the greater the positive impact on CARs from Event 2. This finding is consistent with investors’ belief that firms at the epicenter of the outbreak were more difficult to resume production and operations. Therefore, investors remain pessimistic about firms close to Wuhan City.
The epidemic had severely restricted the movement of factors such as labor. One of the most important ways to deal with the epidemic is to impose physical quarantines and restrict the movement of people, which would prevent employees from coming to work, greatly hinder the process of logistics, and disrupt firms’ supply chains. Wuhan City was the epicenter of the epidemic, and restricting and sealing the city had become an important measure to stop the further spread of the epidemic. As a result, firms near Wuhan City were more likely to have employees unable to get to work. At the same time, considering the necessary transaction costs in business activities, such as distance cost, firms often choose to conduct business with those close to their business location. Therefore, distance also affected the smooth logistics and the integrity of the supply chain. All these would cause difficulties in enterprise operation and management, and then affected firm performance. In the short term, investors were more inclined to invest in firms further away from Wuhan City.
In addition, the impact of the epidemic could also bring about uncertainty and negative mood. On the one hand, investors might be more optimistic about the recovery of firms farther from Wuhan City. On the other hand, the confidence of entrepreneurs was crucial to restoring in production. Entrepreneurs at the center of the epidemic would have more negative moods to the resumption of production, which was not conducive to the normal operation of the firm from a subjective perspective. And investors would take a wait-and-see attitude towards such firms in anticipation of this phenomenon. To sum up, distance indeed matters firms’ CARs a lot.
5.3 Heterogeneity
We also explore some potential heterogeneity to see if distance between firms’ business locations and Wuhan City impacts CARs across some key firm or industry characteristics. Heterogeneity regression results are presented in Table 8.
We first analyze an industrial heterogeneity. The interaction term between the pharmaceutical industry dummy and the distance is added to the regressions. As shown in Columns (1) and (2) of Table 8, the interaction term is significantly positive in Event 1, and significantly negative in Event 2. Due to the lockdown, raw materials for firms were limited, and the Spring Festival holiday made it difficult for firms to resume operations on time. However, firms in the medical and pharmaceutical industries restarted their operations immediately when faced with difficulties. They even continued on to work overtime to create medical products, resulting in the industry’s recovery. Therefore, investors’ expectations for the pharmaceutical and medical industry were raised, thereby alleviating the bad situation. Then, when the epidemic situation improved, companies in the medical and pharmaceutical industries no longer had higher market expectations than other industries, which provided more firms with good development prospects.
Columns (3) and (4) explore the heterogeneity of financial development. The regressions include the interaction term between the distance and the digital inclusive finance dummy. The coefficient of the interaction term is significantly positive during Event 1, but significantly negative during Event 2. This indicates that digital inclusive finance released the negative impact of the epidemic and slowed down the positive effects of good news on CARs. This may be due to the fact that digital inclusive finance reflects China’s digital economy, which can alleviate regional inequalities more effectively. During a severe epidemic, when factors flowed relatively slowly, digital inclusive finance greatly facilitated the economic behaviors of individuals, enterprises, and governments, and could counteract the distance-related difference in CARs. In addition, digital inclusive finance can increase the coverage of financial services and reduce the cost of financial services [40], as well as make financial behaviors more accessible and convenient [41]. Particularly for SMEs with financing difficulties, digital inclusive finance reduced the likelihood of financial constraints [42,43] and allows SMEs to handle business shocks more effectively. This would make sure that SMEs of all sizes and regions could benefit more evenly from the policy, given the improving epidemic situation and looser monetary policy.
Columns (5) and (6) examine the heterogeneity based on the type of firm’s business. The interaction term between the distance and the trade business dummy is included in the regression. Under Event 1, the interaction term is insignificant. The epidemic was just breaking out, and the international environment was stable, so CARs did not suffer any effects from the trade business. Under Event 2, the interaction term is significantly negative. This may because that Event 2 coincided with the time node when the overseas epidemic worsened. Many SMEs involved in import and export business were affected by the global spread of COVID-19.
6 Conclusion
Early 2020 marks the beginning of the COVID-19 epidemic. SMEs had a low level of risk resistance and self-healing ability, so public emergencies affected them more severely. Moreover, innovative firms are more likely to go bankrupt during crises [21]. As representative of innovative SMEs, GEM listed companies are contributing to China’s economic growth. Thus, this paper uses event analysis to investigate the stock yield performance of GEM listed companies during the epidemic. Furthermore, this paper examines the effects of the straight-line distance between a firm’s business location and Wuhan City on the CARs.
The empirical results indicate that: Firstly, “Good News” of epidemic made the GEM more optimistic, while “Bad News” of epidemic had a significantly negative impact on the GEM. Secondly, a targeted RRR reduction policy was more beneficial to GEM listed companies than an interest rate reduction policy. Thirdly, the distance between the firms’ business locations and Wuhan City did not significantly affect the CARs in the early stages of the outbreak. When the epidemic situation improves, the farther the firms’ business locations are from Wuhan City, the more positive the impact on CARs. Finally, there were heterogeneous effects based on industry, inclusive finance, and business type. COVID-19’s negative impact on CARs can be released by digital inclusive finance.
Based on these findings, this paper provides some recommendations on how to mitigate the negative impacts of public health emergencies on SMEs.
Firstly, the central government needs to grasp the benefits of policies and assist SMEs that are suffering large losses. In order to meet SMEs’ needs, local governments should accelerate the implementation of policies. Additionally, they should provide loan guarantees for qualified SMEs to ease their lending and financing difficulties.
Secondly, the government must take action to stabilize the stock market during the epidemic, to ease investors’ panic and to ensure the survival and development of GEM listed companies. The government could prioritize the stable shutdown of the stock market in case of a public emergency in order to stabilize the securities market. Moreover, government should ensure that information disclosure is timely and effective and the content of information is true and reliable. Securities regulatory authorities are supposed to send positive and correct signals to investors and listed companies, guiding market participants to invest rationally.
Thirdly, the central bank can prioritize RRR reduction policy over interest rate cuts. Based on the epidemic situation, the two policies could be implemented alternately, creating a superposition effect to complement one another. In addition, the central bank must pay attention to the tilt of innovative SMEs, deepening inclusive finance activities.
Lastly, the government should fully consider the effect of the distance factor on the production and operation activities of SMEs, as far as possible to achieve “one-industry, one-way” and “one firm, one policy”. The government should pay close attention to the medical and pharmaceutical industry, which is overheating in the early stages of the epidemic. Thus, overproduction would be avoided, and its steady and healthy development would be ensured.
There are limitations in this study. At the initial stage of the epidemic, various epidemic news and policies were released frequently. It is difficult for us to accurately test the impact of all events on the market and obtain a pure effect. Therefore, only four representative and comparative epidemic news and monetary policies are the main research objects. In addition, this paper only examines the short-term market performance within half a year after the outbreak of the epidemic, but did not track and study the medium and long-term market changes and economic conditions within 3 years since the outbreak. How to develop the capital market in the post epidemic era is an important future research direction. Finally, considering the high infectivity of the epidemic, the contagion network will be further expanded in business and interpersonal communication. On the basis of this fact, how various economic networks, such as production networks, will change is also a research direction worthy of attention.
Data availability statement
The raw data supporting the conclusion of this article will be made available by the authors, without undue reservation.
Author contributions
All authors listed have made a substantial, direct, and intellectual contribution to the work. LW and YW contributed to conception and design of the study. LW organized the database. YW performed the statistical analysis. ZH wrote the first draft of the manuscript. All authors contributed to manuscript revision, read, and approved the submitted version.
Funding
This work is supported by Humanities and Social Sciences Project of the Ministry of Education of China (Grant numbers 22YJA790055, Grant numbers 21YJC790107), Guangdong Philosophy and Social Science Planning Project (Grant numbers GD22YYJ06), National Natural Science Foundation of China (Grant numbers 72073142).
Conflict of interest
The authors declare that the research was conducted in the absence of any commercial or financial relationships that could be construed as a potential conflict of interest.
Publisher’s note
All claims expressed in this article are solely those of the authors and do not necessarily represent those of their affiliated organizations, or those of the publisher, the editors and the reviewers. Any product that may be evaluated in this article, or claim that may be made by its manufacturer, is not guaranteed or endorsed by the publisher.
Footnotes
1Source:https://news.china.com/focus/2021quanguolianghui/13004116/20210305/39349372.html.
2Source: CSMAR database.
3On 3 Feb 2020, People’s Bank of China launched an open market reverse repurchase operation with 1.2 trillion yuan. It was the monetary policy closest to the outbreak. Considering that the Chinese stock market is closed during the Spring Festival holiday, it was only one trading day between the announce of this policy and the lockdown. The pure effect of this monetary policy is hard to come by, so we have not included it in our event day list.
References
1. Xu R. Statistical methods for the estimation of contagion effects in human disease and health networks. Comput Struct Biotechnol J (2020) 18:1754–60. doi:10.1016/j.csbj.2020.06.027
2. Holme P. Temporal network structures controlling disease spreading. Phys Rev E (2016) 94:022305. doi:10.1103/PhysRevE.94.022305
3. Iacopini I, Petri G, Barrat A, Latora V. Simplicial models of social contagion. Nat Commun (2019) 10:2485. doi:10.1038/s41467-019-10431-6
4. Colman E, Spies K, Bansal S. The reachability of contagion in temporal contact networks: How disease latency can exploit the rhythm of human behavior. BMC Infect Dis (2018) 18:219. doi:10.1186/s12879-018-3117-6
5. Yang Z, Chen Y, Zhang P. Macroeconomic shocks, financial risk transmission and governance response in major public emergencies. Manag World (2020) 36(5):13–35+7. (in Chinese). doi:10.19744/j.cnki.11-1235/f.2020.0067
6. Gourio F. Disaster risk and business cycles. Am Econ Rev (2012) 102(6):2734–66. doi:10.1257/aer.102.6.2734
7. Zhu W, Zhang P, Li P, Wang Z, Kong F, Fu P, et al. Novel macrolactams from a deep-sea-derived streptomyces species. Manag World (2020) 36(4):13–26. (in Chinese). doi:10.3390/md19010013
8. Al-Awadhi AM, Alsaifi K, Al-Awadhi A, Alhamadi S. Death and contagious infectious diseases: Impact of the COVID-19 virus on stock market returns. J Behav Exp Finance (2020) 27:100326. doi:10.1016/j.jbef.2020.100326
9. Ashraf BN. Economic impact of government interventions during the COVID-19 pandemic: International evidence from financial markets. J Behav Exp Finance (2020) 27:100371. doi:10.1016/j.jbef.2020.100371
10. Estrada M, Koutronas E, Lee M. Stagpression: The economic and financial impact of the COVID-19 pandemic. Contemp Econ (2020) 15(1):19–33. doi:10.5709/ce.1897-9254.433
11. Zhang D, Hu M, Ji Q. Financial markets under the global pandemic of COVID-19. Finance Res Lett (2020) 36:101528. doi:10.1016/j.frl.2020.101528
12. Jackson EL. Response to earthquake hazard: The west coast of North America. Environ Behav (1981) 13(4):387–416. doi:10.1177/0013916581134001
13. Dessaint O, Matray A. Do managers overreact to salient risks? Evidence from hurricane strikes. J Financial Econ (2017) 126(1):97–121. doi:10.1016/j.jfineco.2017.07.002
14. Misici L, Filippo S. Epidemic propagation: An automaton model as the continuous SIR model. Appl Math (2013) 4(10C):84–9. doi:10.4236/am.2013.410A3011
15. Bausch DG, Schwarz L. Outbreak of Ebola virus disease in Guinea: Where ecology meets economy. Plos Negl Trop Dis (2014) 8(7):e3056. doi:10.1371/journal.pntd.0003056
16. Zhang X, Liu L, Ge Z, Zhao H, Miao L, Pan Y. The dimension and morphology of alveolar bone at maxillary anterior teeth in periodontitis: A retrospective analysis-using CBCT. Econ Res J (2020) 55(6):4–21. (in Chinese). doi:10.1038/s41368-019-0071-0
17. Rassy D, Smith RD. The economic impact of H1N1 on Mexico’s tourist and pork sectors. Health Econ (2013) 22(7):824–34. doi:10.1002/hec.2862
18. He C, Wen Y, Chang Y, Geng X. Measurement analysis of the impact of the new coronavirus pneumonia outbreak on China’s economy. J Quantitative Tech Econ (2020) 37(5):3–22. (in Chinese). doi:10.13653/j.cnki.jqte.2020.05.001
19. Worthington A, Valadkhani A. Measuring the impact of natural disasters on capital markets: An empirical application using intervention analysis. Appl Econ (2004) 36(19):2177–86. doi:10.1080/0003684042000282489
20. Mel S, McKenzie D, Woodruff C. Enterprise recovery following natural disasters. Econ J (2012) 122(559):64–91. doi:10.1111/j.1468-0297.2011.02475.x
21. Lee N, Sameen H, Cowling M. Access to finance for innovative smes since the financial crisis. Res Pol (2015) 44(2):370–80. doi:10.1016/j.respol.2014.09.008
22. Sannajust A. Impact of the world financial crisis to SMEs: The determinants of bank loan rejection in Europe and USA. No 2014-327, Working Papers. France: Department of Research, Ipag Business School (2014).
23. Zhang H, Tong X. Structural change in China’s emergency management: Theoretical generalizations. Social Sci China (2016) 37(2):77–98. doi:10.1080/02529203.2016.1162010
24. Noy I. The macroeconomic consequences of disasters. J Dev Econ (2009) 88(2):221–31. doi:10.1016/j.jdeveco.2008.02.005
25. Hartmann P, Straetmans S, Vries CG. Asset market linkages in crisis periods. Rev Econ Stat (2004) 86(1):313–26. doi:10.1162/003465304323023831
26. Barunik J, Krehlik T. Measuring the frequency dynamics of financial connectedness and systemic risk. J Financial Econom (2018) 16(2):271–96. doi:10.1093/jjfinec/nby001
27. Albulescu CT. COVID-19 and the United States financial markets’ volatility. Finance Res Lett (2021) 38:101699. doi:10.1016/j.frl.2020.101699
28. Baig AS, Butt HA, Haroon O, Rizvi SAR. Deaths, panic, lockdowns and US equity markets: The case of COVID-19 pandemic. Finance Res Lett (2021) 38:101701. doi:10.1016/j.frl.2020.101701
29. Haroon O, Rizvi SAR. COVID-19: Media Coverage and financial markets behavior-A sectoral inquiry. J Behav Exp Finance (2020) 27:100343. doi:10.1016/j.jbef.2020.100343
30. Ragin MA, Halek M. Market expectations following catastrophes: An examination of insurance broker returns. J Risk Insurance (2016) 83:849–76. doi:10.1111/jori.12069
31. Shelor RM, Anderson DC, Cross ML. Gaining from loss: Property liability insurer stock values in the aftermath of the 1989 California earthquake. J Risk Insurance (1992) 59(3):476–88. doi:10.2307/253059
32. Lamb RP. An exposure-based analysis of property-liability insurer stock values around Hurricane Andrew. J Risk Insurance (1995) 62(1):111–20. doi:10.2307/253695
33. Lanfear MG, Lioui A, Siebert MG. Market anomalies and disaster risk: Evidence from Extreme weather events. J Financial Markets (2019) 46:100477. doi:10.1016/j.finmar.2018.10.003
34. Xie H, Jiang T, Zhang W. Trade effects of political shocks - analysis of events with the election of trump. China Econ Q (2020) 19(1):233–60. (in Chinese). doi:10.13821/j.cnki.ceq.2019.04.11
36. Hood M, Kamesak a A, Nofsinger J, Tamura T. Investor response to a natural disaster: Evidence from Japan’s 2011 earthquake. Pacific-Basin Finance J (2013) 25:240–52. doi:10.1016/j.pacfin.2013.09.006
37. Tai Z, Sun T. The rumouring of SARS during the 2003 epidemic in China. Sociol Health Illness (2011) 33(5):677–93. doi:10.1111/j.1467-9566.2011.01329.x
38. Campbell JY, Lo AWC, Mackinlay AC. The econometrics of financial markets. Princeton, New Jersey: Princeton University Press (1997).
39. Corrado CJ, Zivney TL. The specification and power of the sign test in event study hypothesis tests using daily stock returns. J Financial Quantitative Anal (1992) 27(3):465–78. doi:10.2307/2331331
40. Sutherland A. Does credit reporting lead to a decline in relationship lending? Evidence from information sharing technology. J Account Econ (2018) 66(1):123–41. doi:10.1016/j.jacceco.2018.03.002
41. Jaksic M, Marinc M. Relationship banking and information technology: The role of artificial intelligence and fintech. Risk Management-an Int J (2019) 21(1):1–18. doi:10.1057/s41283-018-0039-y
42. Mollick E. The dynamics of crowdfunding: An exploratory study. J Business Venturing (2014) 29(1):1–16. doi:10.1016/j.jbusvent.2013.06.005
Keywords: COVID-19 pandemic, Wuhan’s lockdown, growth enterprise market, monetary policy, digital inclusive finance
Citation: Wang L, Huang Z and Wang Y (2023) Measuring the impact of Wuhan’s COVID-19 lockdown on the growth enterprise market in China. Front. Phys. 10:1081615. doi: 10.3389/fphy.2022.1081615
Received: 27 October 2022; Accepted: 14 December 2022;
Published: 06 January 2023.
Edited by:
Jianbo Wang, Southwest Petroleum University, ChinaReviewed by:
Zhiwu Hong, China University of Political Science and Law, ChinaLiyun Zhou, South China Agricultural University, China
Xian Fang, Institute of industrial economics, Jinan University, China
Copyright © 2023 Wang, Huang and Wang. This is an open-access article distributed under the terms of the Creative Commons Attribution License (CC BY). The use, distribution or reproduction in other forums is permitted, provided the original author(s) and the copyright owner(s) are credited and that the original publication in this journal is cited, in accordance with accepted academic practice. No use, distribution or reproduction is permitted which does not comply with these terms.
*Correspondence: Yanan Wang, d2FuZ3luNTE4QHNpbmEuY29t