- 1General Coordination of Earth Sciences, Division of Impact, Adaptation and Vulnerabilities, National Institute for Spatial Research (INPE), São Paulo, Brazil
- 2Civil Engineering Division, Technological Institute of Aeronautics (ITA), São Paulo, Brazil
The 2030 Agenda framework represents an opportunity for the co-development of local indicators and shares the same principles of cross-sectoral integration and universality as the water–energy–food nexus. This research aimed to develop a quantitative-collaborative methodology for constructing indicators and indexes for the sustainability of the Cerrado and Caatinga Brazilian biomes, considering the connection and synergies between the Nexus perspective and the 2030 Agenda’s SDG–target framework. The study was applied in 2,512 municipalities that make up the total of the mentioned biomes. The methodology consists of four major blocks: i) identification and construction of indicators based on the association of them with one or more 2030 Agenda’s targets, in a collaborative way from expert groups; ii) generation of weights by an indicator considering leverage, according to the number of target assignments, and importance, attributed by experts for each indicator to the target; iii) generation of sustainable development goal indexes, considering the performance of the SDGs; Nexus performance, considering different weights between the SDGs; Nexus synergistic, the standard deviation between the SDGs; and Nexus overall, a combination of the last two; and iv) sensitivity analysis for performance indexes considering the assignment of zero values to the governance indicators. In general, the regions of MATOPIBA, northern and western of Cerrado and the Caatinga, are, on average, the areas of greatest vulnerability. The targets that respond to this inequality are related to eradicating hunger, sustainable and climate-resilient production systems, universal access to water, water quality, efficiency of multiple water uses, access to energy, and the participation of renewable energies. We found that in areas of better average nexus performance, there is also greater inequality between nexus dimensions; therefore, the Nexus overall index is penalized. The federal units and areas with the worst Nexus dimension performances and with insufficient governance measures are north and northwest of Bahia and municipalities bordering the State of Tocantins—food; Maranhão and Piauí, northeast of Mato Grosso, northwest of Goiás, Rio Grande do Norte and Paraíba—water; and Maranhão, extreme southeast of Pará, and more sparsely in Paraíba—energy.
1 Introduction
Global environmental changes have proportions never experienced by humanity, and they are increasingly affecting critical resources such as water, energy, and food. The discussion around the Anthropocene idea [1, 2] put in perspective the global linkages between environmental changes and their associated food, water, and energy securities [3, 4].
The Nexus agenda focuses on how to “deliver water, energy, and food for all, and manage the synergies and trade-offs among them, by understanding how these interactions are shaped by environmental, economic, social, and political changes” [5, 6]. It is an approach that interconnects many geographic scales and is the key to understanding national sustainable development strategies, including human, economic, and environmental sustainability dimensions [7]. Therefore, Nexus could be understood as a sustainability lens to prevent potential future risks [8].
There has been an increase in publications of Nexus research, presenting case studies [3, 9, 10], methodological frameworks [11–14], and a Nexus governance approach [6, 7, 15]. However, the lack of data systematization culture and stakeholder engagement toward policy planning and implementation demands inter-multi-transdisciplinary strategies to comprehend and synthesize the inherent complexity of socio-environmental systems and their sustainability [16–18].
Since 2016, the 2030 Agenda has represented a milestone in international sustainability governance. For the first time, the international community has agreed on a comprehensive, binding, and concrete system of goals and targets to guide global transformation toward sustainability [19]. Although implementing the 17 SDGs has many challenges (e.g., high diversity and complexity of SDG goals [20], lack of governing capacity associated with policymakers, and lack of tools and indicators to assess progress toward goals), there is an opportunity to combine the SDG with Nexus approaches.
Integrating SDGs with the Nexus approach allows for co-development of actionable knowledge toward problem-solving while achieving multiple sustainable development goals (SDGs) [14]. The Nexus approach and SDGs share the same principles of cross-sectoral integration and universality, as achieving a target cannot occur at the expense of another [21, 22].
Although Brazilian research has leadership with Nexus approach studies and SDGs, contributions to social sciences remain marginal as it prioritizes other topics, such as hydroelectric energy and biofuels, with works on efficiency, optimization, modeling, and technological innovation [23–25].
The literature highlights the necessity to use participatory and collaborative methodologies between local knowledge, experts, stakeholders, and decision-makers [18, 26–29]. All relevant stakeholders must work together to manage the synergies and trade-offs among different management and governance sectors (e.g., social, economic, environmental, and institutional), while traditional “silo” approaches cannot effectively address the linked challenges [14].
Brazil is one of the most vulnerable countries to climate change as it heavily depends on agricultural and mineral commodities. The implementation of SDGs and Nexus approaches is particularly demanding in Brazil, where “insufficiently understood interactions within the Nexus are contributing to large-scale deforestation and land-use change, water and energy scarcity, and increased vulnerability to climate change” [30].
Brazilian Cerrado and Caatinga biomes are the ones that have suffered the most pressure associated with fewer conservation policies, such as, respectively, speculation on agricultural frontiers, especially industrial agriculture and cattle-intensive livestock farming [31, 32], and countryside human density, lash-and-burn agriculture, overgrazing by livestock, and firewood collection [33, 34]. The climate change scenarios point to the replacement of tropical forests by tropical savannas, expansion of the Cerrado, and aridization of parts of Northeast Brazil, a region mainly represented by the Caatinga biome.
This study aims to contribute to the literature by presenting a quantitative-collaborative methodology for constructing indicators and indexes for sustainability of the Brazilian Cerrado and Caatinga biomes, considering the connection and synergies between the Nexus perspective and the 2030 Agenda’s SDG–target framework. This study is part of a scientific project called Nexus [35].
2 Materials and methods
2.1 Geographical study area
Biomes are large-scale ecosystems with uniformity of macroclimate, physiognomy (vegetable and animal), soil, and water, among other aspects [36]. In Brazil, there are six biomes: the Amazon, Caatinga, Cerrado, Atlantic Forest, Pampa, and Pantanal. The Cerrado and Caatinga biomes cover 34% of the country’s total area [37]. In both biomes, the vegetation type is the savannah, with physiognomies that reflect the water conditions of the soil [38].
The Cerrado biome stands out for its modern agricultural practices and extensive livestock farming [39], emphasizing the production of soybeans, maize, and sugarcane [40]. Soybean stands out as Brazil’s most important cash crop, with 48% of its production occurring in the Cerrado biome [41]. Despite the economic importance of large-scale agriculture, studies suggest the negative impacts of this activity, for instance, on water resources and soil [42], as well as on nearby rural communities [43]. Another concern for Cerrado biome sustainability is the irregular use of fire, which reduces above-ground and in-ground carbon and increases biodiversity loss [44]. The Cerrado biome has already lost 50% of its original natural cover [45], with 45% of its use related to agriculture, which increased from 619,957 km2 in 1985 to 901,514 km2 in 2021 [46].
The Caatinga biome is in the Brazilian semi-arid region. It is susceptible to desertification, defined as land degradation in arid, semi-arid, and dry sub-humid regions [47]. This region has larger goat and sheep herds than other regions [40]. Extractive activities, logging, and rainfed and irrigated agriculture are typical activities of this biome [48]. The Brazilian semi-arid region is a territorial delimitation based on climatic criteria [49], which is one of the most populous semi-arid regions in the world, representing almost 12% of the Brazilian population [33, 50]. However, 16.7% of the Brazilian semi-arid region is in a potentially degraded area, while 4% of the region is in a heavily degraded area, resulting in high and very high degradation trajectories [51].
Considering the magnitude of the consequences in the Cerrado and Caatinga, we emphasize some socio-ecological characteristics endangered by those impacts. The Cerrado is an important global biodiversity hotspot with a high rate of endemism [52], and with a significant contribution to the maintenance of water resources in Brazilian hydrographic basins [53]. The Caatinga is essential to the population’s water security [54]. Currently, protected areas cover only 8.6% of the Cerrado and 7.6% of the Caatinga [55].
The study area comprises municipalities totally or partially covered by the Cerrado and Caatinga biomes, considering the 2005 delimitation of the MMA. It represents 2,512 municipalities (45% of the total Brazilian municipalities). These municipalities are the unit of analysis for this work, considering the 2018 municipal grid of the IBGE.
The Cerrado overlaps all states of Brazil’s central-west region and, to some extent, other regions like the northeast and southeast. The Cerrado also contains part of the MATOPIBA region, a new Brazilian agricultural frontier covering parts of Maranhão, Tocantins, Piauí, and Bahia states [56]. The Caatinga biome is present in northeastern Brazil and northern Minas Gerais and is practically inserted in the institutional delimitation of the Brazilian semi-arid region (Figure 1).
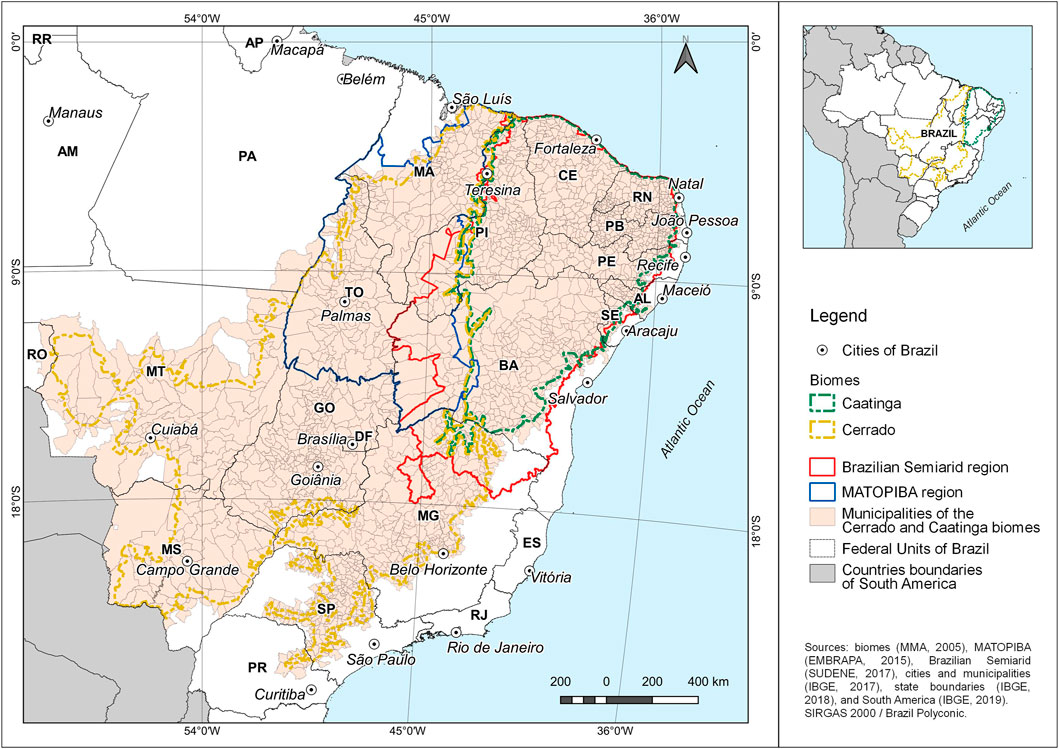
FIGURE 1. Study area, composed of the Cerrado and Caatinga biome municipalities, with emphasis on the Brazilian semi-arid and MATOPIBA regions.
2.2 Indicator and index construction for sustainability
In Brazil, there are some national initiatives to bring sustainability indicators. The Brazilian Institute of Geography and Statistics (IBGE) created the National Commission of SDGs, by the Government Secretary of the Republic Presidency, which assigns the role of permanent technical advice to the Applied Economics Research Institute (IPEA). In 2018, the IPEA adapted global goals to Brazilian reality, considering national strategies, plans, and policies [24]. At a sub-national level, there is a third-sector initiative with the Sustainable City Development Index—Brazil (IDSC-BR) [25], which evaluates Brazilian municipality progress and challenges for fulfilling the 2030 Agenda. However, deepening the understanding of the nexus in a broad and synoptic way is still a challenge for the municipality scale in Brazil.
The construction of indicators is one of the main ways of using Nexus analysis to assess progress toward targets, covering 25% of the study cases considered by (11). To this end, the aggregation of qualitative and quantitative analysis, such as the use of surveys for stakeholders, is recommended to provide a broader and deeper understanding of Nexus interactions, but only 19% of this approach has been used. Another issue to consider is the lack of multidimensional data that deal with the Nexus or sustainability. The nations endeavored to achieve Tier 1-type indicators—which are conceptually clear and have a methodology with international standards that are continuously produced—for the 2030 Agenda’s SDGs.
Construction indicators are associated with a measurement process. It requires a normative exercise: i) a robust conceptual definition; ii) a collection of consistent observations; and iii) an analysis of the relationship between the observed data and the concept that the indicator is expected to measure [57]. Indicators have purposes, which differ from variable data purposes, for instance: i) synthesis of information, which is often complex (an indicator always expresses something more significant and not just its value); ii) standardization of communication for a specific or broader audience; and iii) generally, they must have a reference for comparison, whether this is a range of reference values or targets to be pursued.
Indexes are latent variables of reality, and they are not the result of direct observations. Hence, the literature mentions two aspects of latent variables, namely, reflexive and formative [58]. In the reflexive perspective, the index concept determines the choice and the construction of the indicators that generate it. In the formative perspective, the index concept results from the performance of the indicators that form it.
Sustainability indicators must observe the following elements: i) measurable; ii) data availability; iii) clean, transparent, and standardized methodology for their construction; iv) financially viable collection; and v) legitimized by decision-makers [59]. There is a broad application of indices to assess socio-ecological aspects, such as policy analysis and public communication to compare countries [60], ecological quality [61], multidimensional poverty [62], and vulnerability to climate disaster risk [63], to evaluate the Sustainable Development Goals (SDGs) of the 2030 Agenda [64].
In this work, the steps for constructing indicators and their composition in indexes followed parts of the structure and chronology suggested by (66), which were i) theoretical framework, ii) selection variables, iii) imputation of missing data, iv) multivariate analysis, v) normalization of data; vi) weighting and aggregation; vii) robustness and sensitivity; (viii) back to the details; ix) links to other variables; and x) presentation and dissemination. We propose reorganizing these items into specific work packages to better understand the collaborative, qualitative, and quantitative steps. Figure 2 illustrates these work packages and their interconnections, which are detailed below in methodological terms.
Two team structures developed this study in the Nexus Project: Analysis and Development for Sustainability Indicators Laboratory (Ladis-lab) (https://www.ladis-inpe.com/) and the Nexus project’s research team. The first one was composed of eight researchers who were responsible for development of activities and validation of results. The second one was composed of about 30 researchers who were part of the consulting expert body. They have some specialized expertise, and some of them are distributed along the research or educational institutions of the Cerrado and Caatinga.
2.2.1 Conceptual framework
This section presents the definition of concepts that will guide the selection and construction of indicators, semantic values, and weights and their compositions in indexes. It requires an abstraction process that must consider the socio-cultural, geographical, and historical context [58]. Following [58], the conceptual definition process will allow identifying and defining: i) the model for data construction; ii) the spatial and temporal ambit of observation; iii) the aggregation levels (among indicators and/or among observation units); and iv) the models allowing interpretation and evaluation.
This work uses the framework of the 2030 Agenda as the basis for data construction, composed of SGDs, targets, and monitoring indicators. We follow the adapted Brazilian targets by the IPEA [24]. The spatial and temporal scales are the 2,512 municipalities, according to Section 2.1, and the 2010 decade. This time range considers the two more recent Brazilian censuses, the 2010 Demographic Census and the 2017 Agricultural Census. The aggregation of indicators—the composition indexes—follows three levels, namely, targets, SGD, and Nexus. Considering that the 2030 Agenda indicators must meet the pre-established SDGs, this work brought a reflexive approach to assembling indexes.
2.2.2 Variables selection
The search for an initial set of variables concerns the direct relationship of indicators with one or more Brazilian goals adapted from the 2030 Agenda [24]. Starting from the collaborative basis on which we developed this study, we seek to involve a greater number of researchers in the Nexus project to embrace a great diversity of areas of knowledge among the researchers on the Nexus project’s research team.
The variable selection processes start with two meetings to identify Thematic Expert Groups among the Nexus project’s research team. This group identifies the variables that could respond directly to one or more targets, writes the metadata for the variables, and makes them available. Eight Thematic Expert Groups were proposed, namely, water resources, agriculture, forest conservation and biodiversity, land degradation, energy, socioeconomics, greenhouse gases and biogeochemical cycles, and climate risks.
The researchers of the project Nexus produced variables from secondary data or primary sources. The assignment of the targets underwent a review by the Ladis-lab group for conceptual standardization. Each Thematic Group’s experts wrote descriptions and metadata details for each indicator under their responsibility (e.g., construction description, spatial cover and spatial unit, temporal reference, and the source that generates the data). The Ladis-lab group revised the metadata with indicator usefulness for sustainability and information about the type of indicator—whether of state or governance—that will be used for the sensitivity stage.
2.2.3 Indicators datasets
This stage comprises the construction of variables to indicators in a quantitative way. The Ladis-lab team carried out the procedures for the quantitative construction of indicators in this stage and the following stages. For that, some pre-processing of the variables is necessary, such as i) transformation of the value of the variables to the municipal geographical limits; ii) treatment of outliers; iii) transformation of highly skewed variables; and iv) pointing out whether the ordering values are arranged in direct (higher values mean more sustainable) or inverse (lower values mean more sustainable) orderings. The data are from the 2010 decade as a time range, and the municipal limits are from the year 2018, according to the IBGE municipal grid of that year. Thus, the Thematic Expert Groups made the data values available within this grid.
For the treatment of outliers, the winsorization technique [66] was considered. It replaces outliers using two rules: greater outlier values were assigned to upper fence values, while lower outlier values were assigned to lower fence values. The upper fence and the lower fence are defined as the third quartile (
Concerning the normalization procedure, two strategies were applied: one for direct and another for inverse ordering [65]. Eqs 3, 4 present the calculations for the normalizations whose sense of the indicator is direct and inverse concerning sustainability, respectively. After these procedures, we had the indicator dataset, whose values range from 0 to 1.
It is worth mentioning some exceptions: i) binary variables, whose values were only “no” or “yes”; were mapped to values such as 0.3 and 0.7, representing the lowest and highest values for sustainability, respectively; and ii) some outliers were kept in a few variables; otherwise, it would stress information that is far from reality.
2.2.4 Redundancy indicator analysis
Redundancy analysis was assigned in the statistical analysis work package. The set of indicators was analyzed quantitatively and conceptually. The quantitative analysis is the way to evaluate pairs of indicators based on Spearman’s correlations, considering the significance level of Student’s t-test. The redundancy analysis was performed for the indicator dataset of each SDG, following this order: i) identify pairs of indicators with correlations equal to or greater than 0.6 or less than -0.61; ii) check the similar meaning between the pairs of indicators identified within the same SDG; iii) eliminate one of the two similar indicators, also considering the lower consistency of the data that are part of the indicator (e.g., many missing values) and the representativeness concerning the target. Deleting an indicator from one SDG implies removing it from the entire dataset and, consequently, from other SDGs as well.
2.2.5 Indexes composition
This stage focuses on the composition of information levels for construction indexes. The proposed indexes are the following: Target index, SDG index, Performance Nexus index, Synergistic Nexus index, and Overall Nexus index. The composition of indicators to create indexes in this work was performed by assigning weights for each indicator and according to each target. For this purpose, we computed a leverage weight for each indicator and later assigned it for each indicator by targeting a response weight for these specific assignments—target response weights.
The leverage weights were calculated from a frequency matrix of assignments between the indicators and the targets. It can show how important each indicator is for the entire set of indicators. The more targets an indicator is assigned, the greater its importance in terms of its leverage. It means that if their values are high, they tend to affect the performance of more targets or SDGs. Weights from 0 to 0.4 were assigned to this type of importance for each indicator. These leverage weights were given by the normalization between the maximum and minimum frequency values (after treatment of outliers by the winsorization technique) among all indicators and multiplied by 0.4, according to Eq. 5.
The target response weights are associated with the indicator response capacity to what the target proposes. In this case, the Ladis-lab group, in consultation with the Thematic Expert Groups, assigns weights from 0 to 0.6 for each indicator by the target. Last, the total weight (
We produced indexes for each target, SDG, and Nexus based on the total weights. The target indexes were calculated by the weighted average among the municipality values (
The indexes of each SDG (
The performance Nexus index was calculated by a weighted average between the raw SDG indexes, with the current weight values but not normalized, the

TABLE 1. Weights assigned to each SDG2 in the performance Nexus index.
For the analysis of the nexus approach, in addition to the good general performance of the most relevant themes, represented by
The Nexus Overall index (
2.2.6 Sensitivity analysis
Sensitivity analysis quantitatively investigates assumptions and particular contributions that are attributed to the composition of the indicators, which can significantly change the variation of the final indexes [65, 66]. The following steps are recommended [66]: i) identify the main uncertainties behind the composition of indicators; ii) assess the impact of the uncertainties on the output indexes or ranks to see which assumptions cause the most uncertainty; and iii) explain the reason for the results of the final indexes with changes in the assumptions made. In this work, the analysis of uncertainties related to the presence and absence of certain groups of indicators was prioritized.
According to Section 2.2.2—Variable selection—each variable was assigned by state type, which represents the current situation, or by governance type, which represents the governance measures to improve the current situation. Such improvement measures can have different dimensions, such as economic, social, political, and/or institutional, but all of them, in whole or in part, have governance aspects. We consider that the governance aspect can be decisive in the trajectory to achieve different targets of the SDG, and public policies in Brazil have high volatility, according to interests and candidacy lobbies. Therefore, indicators of this nature bring high uncertainty, and, in this sense, the
For
For
The municipalities with the highest values of these differences are those that would have the greatest loss of performance in their
Sensitivity analyses were performed using the following patterns: i) existence of regional concentration of contribution of governance indicators; ii) which regions have the highest contribution of governance indicators to high-performance indices for an SDG; iii) which regions have the lowest contribution of governance indicators to low-performance indices for an SDG; and iv) non-existence of patterns “i” and “ii.” The first case deals with the verification of the contribution of governance in a regional way. The second case informs about the continuity of governance aspects because the governance indicators are contributing to the
2.2.7 Targets contribution proportions
This topic aims to evaluate the targets that most contributed to the Nexus. For this purpose, we calculate the target contribution for each municipality (
2.2.8 Diagnostics and presentation
This stage is based on how to present the information obtained in a synthetic way and situate the results with indicators and indexes that exist today. Maps were used to visualize the performance of indexes and sensitivity analysis for all municipalities, while graphs visualize the performance of targets and proportionalities, in all municipalities. The comparison of this work was carried out with another work that currently exists in Brazil at the municipal level, the Sustainable City Development Index—Brazil (IDSC) [25]. This analysis was carried out to investigate the main performance differences between the two works and to verify the positive points between the works. This analysis contributed to assess future perspectives and challenges in improving this work.
3 Results
3.1 Indicators dataset
Initially, a total of 137 indicators were identified and built by the eight Thematic Group’s Experts with different quantities, as shown in Figure 3. The groups with few indicators produced them from geoprocessing or biophysical modeling—primary source data—especially the GHG and BGQ cycles, land degradation, climate risks and forest conservation, and biodiversity groups. The Thematic Group’s experts produced 19 indicators as primary sources, and other 118 indicators were built from secondary sources. This result is coherent to the 2030 Agenda’s SDG as most of the targets have social or economic aspects, which are decisive and transversal to advance environmental and institutional dimensions, in addition to the socioeconomics itself. The supplementary material contains all indicators with their list, including name, short description, direct or inverse sense to the sustainability, temporal reference, type indicator (state or governance), targets related, institutional sources, type of source (primary or secondary), list of acronyms, and values of variables and of indicators.
3.2 Redundancy analysis and the 2030 Agenda’s indexes
All 137 indicators have at least one target. Therefore, the redundancy analysis was performed using all of them. Seven pairs of indicators have similar meanings and correlations greater than or equal to 0.6 or less than or equal to -0.6, according to Table 2. In these cases, the main criterion for their removal was their small coverage compared to similar indicators in different targets. The only exception was “installed capacity in energy self-producers,” whose removal was due to its lower assignment of targets—all of SDG 7—than their similar indicators.
After the redundancy analysis, 130 indicators are available to construct indexes. Based on the frequency matrix of indicators by targets, the leverage weights were computed, ranging from 0.06 (only one target assigned) to 0.40 (six targets assigned). The indicators with the highest leverage aspect (0.4) are “scope of the Bolsa Família program,” “coverage of the Brazilian Cisternas program,” “inadequate households,” “women in formal jobs with more than five minimum wages,” and “measures for reduction of school evasion.” It is noticed that government programs related to eradication of hunger, access to water, and maintenance of education, with adequate housing conditions and appreciation of women’s work, are fundamental for sustainability, as they permeate several goals of the 2030 Agenda.
The distribution of indicators in relation to SDGs and targets is shown in Figure 4. No indicators or representative data at the municipal level for SDG 14 (oceans) are available; therefore, this SDG is not considered in this work. SDGs 2 (zero hunger), 3 (health), 1 (no poverty), 11 (cities), and 6 (clean water and sanitation) had the most coverage of indicators in their targets, with around 17%, 13%, 10%, 10%, and 10%, respectively, comprising 60% of the attribution of indicators to SDG targets. The targets with 10 or more indicators associated with them were 2.3, 2.4, 8.4, 11.5, and 13.1 because they are broad targets, associating issues such as socio-environmental resilience, productivity, environmental risks, and public policies to reduce vulnerabilities. According to the methodology, the indexes were processed according to the average among the targets, and thus the number of indicators did not influence the index´s results.
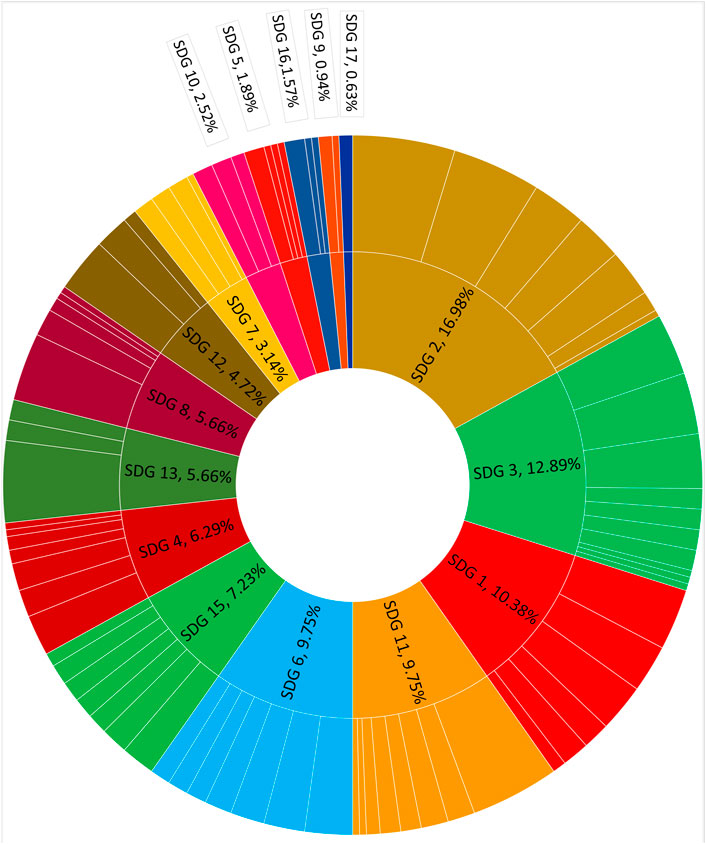
FIGURE 4. Proportion of indicator assignment to 2030 Agenda‘s SDGs (inside circumference) and targets (outside circumference).
3.3 SDG and Nexus indexes
Figure 5 presents the maps of the SDG indexes 2, 6, and 7, which have the highest weight attribution to the Nexus. The maps with other SDG indexes are in Supplementary Material S1. The SDG 2 targets most influenced are 2.1, 2.4, and 2.a, which refer to aspects of eradicating hunger and affordability of food, sustainable and climate-resilient production systems, and increasing investment and technical assistance to family farmers, respectively. The main targets of SDG 6 are 6.1, 6.3, and 6.4, which refer to universal and equitable access to water, water quality, and efficiency of multiple uses of water consumption, respectively. Concerning SDG 7, the Cerrado biome has more favorable energy security, apart from the northern portions of the biome. The targets that present the most similar patterns with this index are 7.1 and 7.2, related to access to energy services and participation of renewable energies in the national energy matrix, respectively.
According to Table 3 and SDG maps (Supplementary Material S1), some patterns have similarities for the other indexes, with the Caatinga and the north and west of the Cerrado being the areas with worse political conditions in the subjects of SDGs 1, 3, 9, 11, 12, 13, and 17. Only SDG 10 (inequality reduction) presents the opposite pattern, although there are many locations in the Caatinga with low values. The highest performance areas of SDG 15 are in mountainous regions or areas with less human occupation, although not necessarily with adequate protection by conservation units. Concerning the other SDGs [4, 5, 8, 16], there is not much uniformity among federative units or biomes.
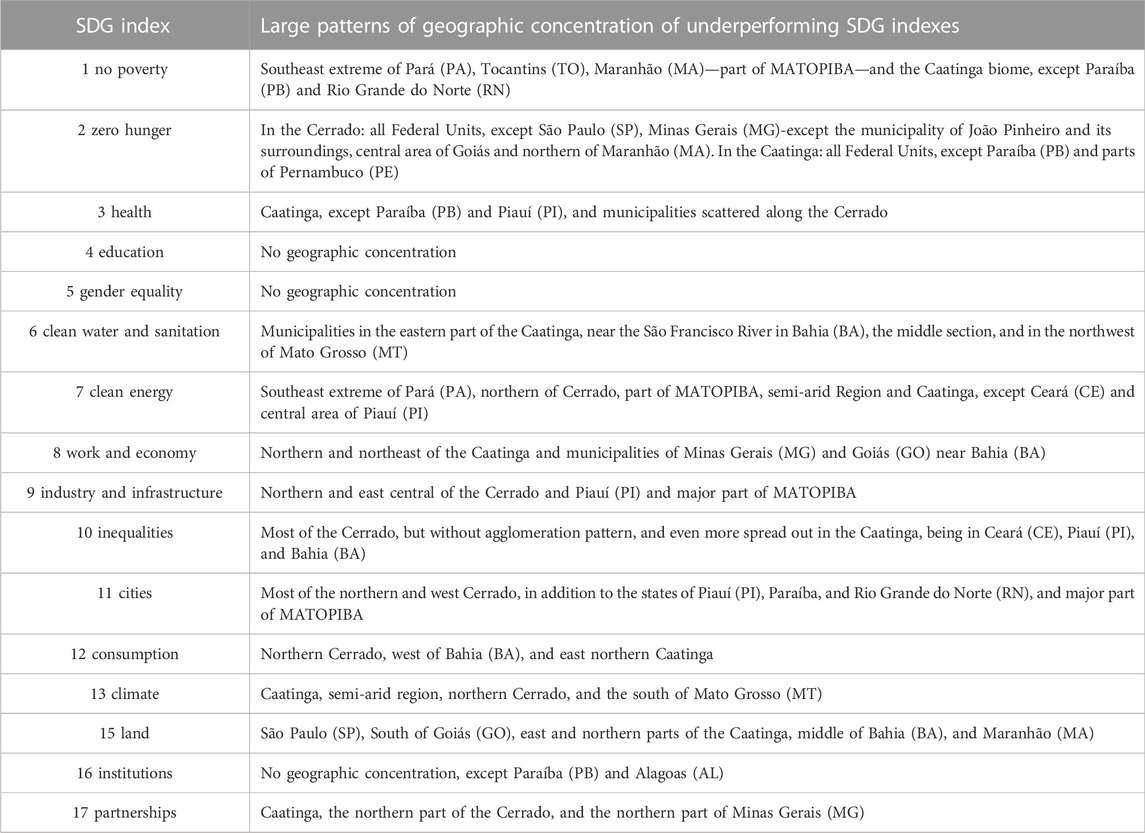
TABLE 3. presents the patterns of geographic concentration of underperforming SDG indexes. The supplementary material contains all figures as maps of SDG indexes, Nexus indexes, and sensitivity analysis.
The
Figure 6 presents the
The
We also performed a comparative analysis by the biome, considering the performance ranges in each of the maps produced (Figure 7). It is noteworthy that mostly the lowest performances are found in the Caatinga. Only in SDGs 2, 5, 10, and 15, this relationship is the opposite, and in SDG 4 and 16, the differences are minimal. We can associate the lower performances of Caatinga’s SDGs and Nexus mentioned according to socioeconomic and climatic issues. The Caatinga is located almost entirely in the northeast region of Brazil, with some portions in the north of Minas Gerais state. The lowest human development indexes (HDIs) are in the northern and the northeast Brazil. The northeast region has predominantly HDIs ranging from low (from 0.50) to medium (up to 0.69), while in the rest of the country—Center-South—the predominance is from medium to high (up to 0.79) [67]. The Caatinga is in a semi-arid climate region and, therefore, demands actions and adaptations to live with this type of climate. The SDG 13 presents targets to combat climate change and its impacts, and according to the variables and indicators constructed for these targets, the region has less capacity for action to reach these targets in relation to the Cerrado.
Conversely, the Cerrado presented lower performances related to the SDGs corresponding to food security, gender equality, reduction of inequality, and the terrestrial environment. The targets of SDG 2 recommend the production of food in a sustainable way, and despite the Cerrado having a high agricultural production, this characteristic has not been evidenced among the indicators that meet such targets. The Cerrado is home to one of the largest agricultural commodities in the world, soybean, in addition to others, such as beef, maize, cotton, sorghum, and sugarcane. Publications have emphasized that the expansion of agriculture in the Cerrado has been highly mechanized and accelerated in recent years, especially in the MATOPIBA region, and in addition to that, 60% of the pastures are in degradation, systematically harming ecosystem services that ensure proper maintenance of agriculture [31, 68, 69]. This land use expansion pattern justifies the lower performance of SDG 15, when compared to the Caatinga, whose average annual deforestation has been 8.6 thousand km2 between 2010 and 2021 [70].
Concerning reducing inequality issues, the Cerrado biome is more unequal, considering the questions of gender (SDG 5) and socioeconomics (SDG 10). For SDG 5, all UFs in the Cerrado, except for MATOPIBA and São Paulo, show a predominance of values from 0 to 0.4. The indicator that most contributed to this result is “violence against the LGBTQI+ population,” meaning the number of violence notifications against the LGBTQI+ population for one hundred residents, between 2015 and 2020. In relation to SDG 10, the Bolsa Família public policy indicator [71], “scope of the Bolsa Família program,” is the one that most contributes to the lower performance in the Cerrado, and this indicator considers the total number of households with a monthly household income of up to half the minimum wage between 2012 and 2016. It is worth noting that despite the greater need for the Bolsa Família program application in the most vulnerable regions of the northeast of the country, this public policy could be more comprehensive for other areas of the country, especially in Minas Gerais, at least within the temporality of the data. Conversely, the Gini index indicator “Gini index variation,” which considers the percentage of variation between the Gini index between the years 2000 and 2010, the Caatinga and the northeast have a predominance of distribution of low intervals (0–0.4), that is, there is greater income inequality.
3.4 Sensitivity analysis
Table 4 presents an overview of sensitivity analysis results for each SDG.
According to Table 4 and the maps (see Supplementary Material for all maps), SDGs 5 and 16 do not present patterns of geographic concentration, which can be inferred that such SDGs have governance capabilities that are more closely linked to the municipality than federative units or Brazilian regions. Also, SDGs 9, 13, and 15 do not present patterns of relationship between the performance of the SDG index and the sensitivity based on the exclusion of governance (
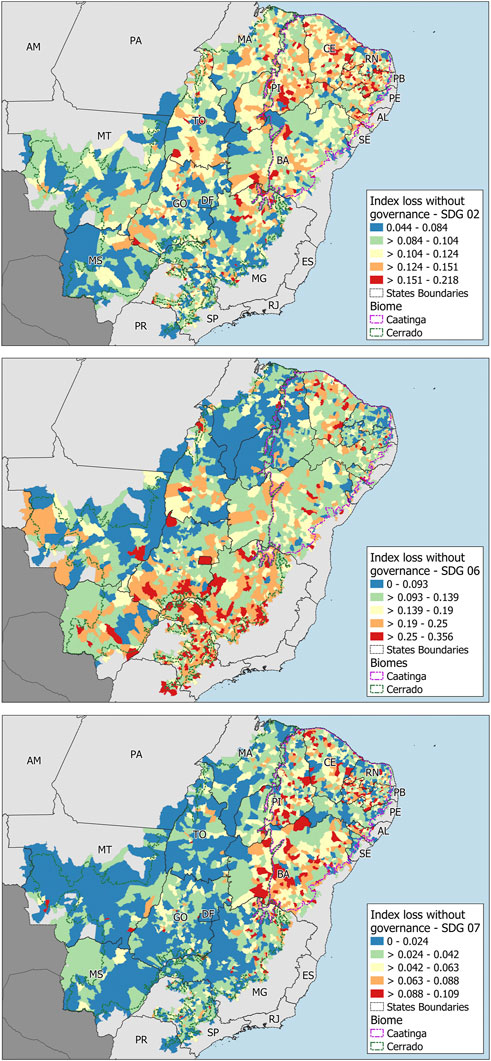
FIGURE 8. (A) Map of sensitivity analysis to governance indicators for SDG 2, (B) map of sensitivity analysis to governance indicators for SDG 6, and (C) map of sensitivity analysis to governance indicators for SDG 7.
For SDG 6, the areas with the most investment in governance mechanisms, improving this SDG, are in the Cerrado biome, especially in the states of São Paulo, Minas Gerais, and Goiás, in addition to the Federal District. In Caatinga, the State of Ceará, and in a more distributed and less evident way in Bahia, positive governance contributions have been made to the improvement of this SDG. On the other hand, the portions that have fewer governance mechanisms together with low sustainability values in this SDG and therefore would need more governmental attention are the north of the Cerrado, the states of Maranhão and Piauí, northeast of Mato Grosso, and the northwest part of Goiás; and in the Caatinga, the states of Rio Grande do Norte and Paraíba.
For SDG 7, the areas with the most investments in governance mechanisms to contribute to the improvement of performance in this SDG are in the Caatinga biome, in Ceará, and in the central-eastern area of Piauí. On the other hand, the portions that have fewer governance mechanisms along with low sustainability values in this SDG and therefore would need more governmental attention are in the State of Maranhão, extreme southeast of Pará, and more sparsely in Paraíba. It is interesting to note that the low values in the north and center of Bahia are not associated with governance indicators. However, it is worth noting that for this SDG, only one governance indicator is presented for target 7.b, the “percentage of public investment in infrastructure in relation to GDP.”
In relation to the sensitivity analysis of governance indicators to the
3.5 Target contribution proportion analysis
This work found indicators associated with 82 targets of the 2030 Agenda. After calculating the proportion of targets to the Nexus of each municipality,
4 Discussion
4.1 Nexus water–energy–food on the 2030 Agenda
The Nexus is an approach capable of supporting sustainable development and efficiently implementing the various SDGs and their synergies [72, 73]. However, few works focus on the challenges of sustainable development at the local level, with much of the Nexus analysis being on national or global scales, which underestimates the most significant challenges that occur at minor scales [74]. The present work promotes the discussion of the nexus in a more localized way, focusing on the municipalities that embed the two most climate vulnerable and susceptible to degradation Brazilian biomes. The evaluation of collaboratively built indicators considers the targets and SDGs of the 2030 Agenda framework. Such an approach becomes especially relevant considering the Brazilian context of population growth combined with factors such as lack of infrastructure and investments, unemployment, and human pressure on ecosystems, which restrict the paths and political actions capable of guaranteeing sustainability under scenarios of strong demand for natural resources. The SDGs 2, 6, and 7 are the ones that most directly address this issue in the Nexus approach and, therefore, received greater weight in the present analysis.
SDG 2 represents the duality of the Brazilian development model, in which the conversion of vegetation cover to agriculture (crops or pasture) on a large scale is synonymous with economic and social progress. However, this type of conversion is not often reflected in better socioeconomic indicators [75, 76]. It is interesting to observe the spatial distribution of SDG 2, pointing out that the areas of agricultural expansion, especially cattle and monoculture agriculture for export, such as soy, cotton, and maize, are also the least sustainable and perform worst in terms of food security, nutrition, and hunger (northern and western parts of the study region). MATOPIBA is the most recent frontier of intensive agriculture in the Cerrado, which was promoted by federal programs, projects, and actions based on the Agricultural Development Plan for the region, pursuant to Decree Nº. 8,447/2015 [77] and is a great exponent of contradictions. A study on inequality and wealth in MATOPIBA between 2011 and 2018 analyzes indicators related to demography, GDP, income, inequality, poverty, life expectancy, and infant mortality to define four types of municipalities. This study shows that of the 337 components of MATOPIBA, only 45 made up group A, in which high agricultural production was accompanied by an expansion of wellbeing [78]. Group D is the most numerous—196 municipalities have low production and social indicators. In addition to the MATOPIBA region, Mato Grosso and west and north of Bahia also have the worst performance values despite having a high agricultural production.
An important cause for these geographical patterns according to the 2030 Agenda, which in this study has greater adherence to targets 2.1, 2.4, and 2.a, is the issues of affordability to food for the most vulnerable people and food production in a more sustainable, diverse, productive, and sustainable way. Its characteristics cover mostly small properties and often family farms. The SDG 2 presents more closely related patterns with SDGs 1, 9, 11, and 12, which means these regions also have similar problems of poverty, infrastructure, and value addition in production, adequate urban conditions, and sustainable production. In areas where the performance of the SDG is low and at the same time there is little input from governance, greater attention from public policies is needed for these, as presented in the results (north and northwest of Bahia, Mato Grosso, some parts of Tocantins, northern Goiás, and extreme southeast of Pará).
Despite the recognition of Brazil as having significant water availability, this distribution is very unequal, either because of the very particular environmental and social conditions or because of the inefficiency of access in more remote regions or the lack of sewage treatment or losses in water distribution in urban centers [79]. Although the rate of water consumption in Brazil has decreased in the last 2 decades, its increase is still constant, especially for water consumptive uses when there is no guarantee of returning the water to be used again [79]. Water consumption that has grown the most is for irrigated agriculture and, more recently, the use of thermoelectric plants. Consumptive use for livestock, which is associated with irrigated agriculture, is also very significant and represents 80% of the total consumptive use in the country. The increase in human supply, especially in basic sanitation, has not grown in the same proportion as the agricultural, energy, and industrial sectors [79, 80].
The SDG 6 index presents a more uniform map than the SDG 2, with most of the study area at average performance (0.4–0.6), but it is still possible to verify the coincidence of areas with low performance. SDG 6 index with areas where the access to the water supply system and basic sanitation still need to improve, such as the regions of the northeast of the country (including the major part of the Caatinga, semi-arid region, and MATOPIBA) and areas to the west of the Cerrado. The SDG 6 presents more closely related patterns with SDGs 1, 3, 4, 11, and 12, which means the SDG 6’s vulnerable regions also have similar problems of poverty; health and wellbeing; inclusive, equitable, and quality education; adequate urban conditions; and sustainable production. In the northeast region, especially in the northern portions, they present a high water risk, especially due to the low water recharge of reservoirs due to long periods of drought—a fact that between 2003 and 2017, 78.5% of the municipalities declared a state of emergency [80]. The 2010s were especially severe, with extensive drought from 2011 to 2017 [81]. It is worth mentioning that this region has been one of the most affected by climate change in the country, with trends of increase in dryness, temperature, and dry days that will be prevalent in the second half of the 21st century [82]. Also, south of the Amazon, where the Cerrado is predominant, is one of the areas that tend to suffer more climatic changes in Brazil, especially in terms of rainfall regime. The northeast region had high investments in the 2010s to mitigate water-related risks with consequences of social and economic impacts [83], and there are expectations that there will be a greater water support capacity for the urban concentrations as parts of the transposition of the São Francisco River are completed [84]. This region, together with the north region, has greater deficits in sanitation in urban areas and, therefore, a greater need for investments and governance measures [80, 83]. The targets 6.1, 6.3, and 6.4 are the ones that most represent the SDG 6 index pattern, which confirms the aspects mentioned.
Concerning Brazilian energy, it is heavily dependent on water resources, with 63.8% of the electricity generated for the National Integrated System by hydraulic sources in 2020 [85]. It should be noted that in this study, a major part of the indicators corresponds to the generation of electric energy, except for the indicators “extraction of charcoal and firewood” and “energy intensity.” It is worth mentioning that in SDG 7, there is no list of associated targets only for renewable and clean energy, such as solar and wind energy (13.6% of the total in Brazil) [85], but only renewable energy. The target 7.a mentions an international cooperation aspect: “enhance international cooperation to facilitate access to clean energy research and technology, including renewable energy and energy efficiency (...),” but we do not consider international targets that could not be applied at the sub-national level. If target 7.2, “renewable energy share in the total final energy consumption,” had been complemented with clean energy, probably some parts of northeast Brazil would have performed better in the index, given the wide existence of wind and solar parks and their 82.1% participation in Brazil [85]. However, the generation of distributed mini and micro energy, which is largely from photovoltaic energy [86], provides some contribution in this regard to the index.
In relation to the SDG 7 index, the most vulnerable areas are in northeast, especially in central and northern Bahia, Maranhão, southwest of Piauí, and eastern Caatinga. This SDG does not have many similarities with the others because its composite indicators generally had spatial resolutions by federal units and not by the municipality, resulting in coarser gradients. However, if we ponder this question, the most similar SDGs are 6, 9, 12, 13, and 17, which means the SDG 7’s vulnerable regions also have similar problems of access and sustainable management of water, infrastructure, and value addition in production, sustainable production, combating climate change and its impacts, and centralized financial resources. Regarding the 7.1 and 7.2 targets having greater adherence to the SDG 7 index, the indicators that most contribute to target 7.1 are “percentage of households without electricity” and “percentage of agricultural establishments that do not use electricity,” and the indicator that most contributes to the target 7.2 is “installed capacity of mini- and micro-distributed energy generation.” These indicators concern aspects of access to energy services and the participation of renewable energies in the national energy matrix, representing 7.1 and 7.2 targets, respectively.
Concerning the mentioned SDGs 2, 6, and 7, the SDGs 1, 9, 11, and 12 are more transversal in relation to those. It means that egalitarian economic growth and social responsibility measures in these SDGs will help those SDGs. If we consider a weighted analysis for the nexus with the 20 most important targets, as shown in Figure 10, it does not show only targets for SDGs 2, 6, and 7, although these are predominant, but it also shows other relevant targets to the Nexus, such as 15.1.2 br, 12.4, and 12.2, which refer, respectively, to conservation of continental aquatic systems; environmentally sound management of chemicals and all wastes, reducing their release to air, water, and soil; and sustainable management and efficient use of natural resources. These targets are related to the environmental dimension, which are important as maintenance of ecosystem services to the food, water, and energy securities. The six targets that stand out in the boxplot of this figure are connected to aspects of water and energy access, water quality, efficient and sustainable use of water, basic sanitation, and biological diversity for human nutrition.
Regarding the comparative analysis between the Cerrado and Caatinga, we can list some considerations, one related to the historical–structural aspects of the Caatinga and another related to data recording. Regarding the historical-structural aspects, the formation of the post-slavery Northeast (19th century) can explain the current lower performances in the Caatinga with most of the ODS and Nexus indices, which there were some economic and political characteristics: pre-capitalist labor relations; periphery dependent on sugar production; surpluses consumed by the elite and not invested, and; government stimulus for a relationship of primary producer and importer of industrial goods with high prices in the Center-South region of Brazil [87, 88]. The second case is related to the results that indicated that the Caatinga is less unequal than the Cerrado (SDGs 5 and 10). The “violence against the LGBTQI+ population” contributed for the mentioned pattern; however, these data come from a record of violence against this population assisted in health services and are subject to underreporting of the event, in which errors may occur in identifying this population by health professionals [89].
4.2 Governance and the Nexus
“Governance of the Nexus refers here to a broad understanding of governance that embraces political, social, economic, and administrative systems that determine the use of resources and delivery of goods and services related to water, energy, and food” (p. 357 [6]). As a result, in contexts with governance measures, it is expected to perform better than in contexts without governance measures. This is what can be deduced from examining the sensitivity of SDGs 2, 6, and 7 to governance measures. Although developmental measures considered have mostly a governmental, public, and top–down nature, they have been able to minimally address institutional issues of great urgency for the Cerrado and Caatinga biomes. However, this configuration does not minimize the need to consider complementary structures of vertical and horizontal governance that are more effective and capable of overcoming the challenge of integrating environmental, economic, and social priorities into policies for sustainable development policies [23]. This places the socio-political dimension of Nexus governance in a central field of discussion, often neglected by technocratic approaches. The reality of developing countries requires a focus on more fundamental aspects of the Nexus, such as water over hygiene and energy over clean energy, as these are still unbalanced priorities for countries in the Global South [23, 90].
Sensitivity analysis shows that some regions have more responses to governance measures than others, which helps improve the overall SDG performance. Regions such as Tocantins, Goiás and Matro Grosso frontiers, municipalities in the southeast and southwest of Mato Grosso do Sul, southeast of the semi-arid region in Minas Gerais, and northeast of Maranhão showed low performance to SDG 2 (Figure 5) and a favorable response to governance indicators (Figure 7) and emphasis on governance in meeting the 2030 Agenda. Governance indicators with most weight to SDG 2, respectively, was “scope of the Bolsa Família program” (weight 11), one of the most important social policies of the last 2 decades; “coverage of the Brazil’s Cisternas program,” a reference program to water collection by cisterns in taking rain water to Brazilian semi-arid region (weights 0,95 and 0,8, respectively) and was relevant to two targets of SDG; “scope of PRONAF in terms of land distribution” (weight 0,8) and “Food Safety Planning and Management Instruments” (weight 0,7). Through the sensitivity analysis, the municipalities of the Caatinga with a greater extent from these governance measures have great capillarity in the areas of family farming. This is considered the predominant form of production in the region and plays an important socioeconomic role in the regional context, which confirms the importance of government public policies to leverage the achievement of goals related to SDG 2. In addition to the previously mentioned governance actions, the rural social security program and the Bolsa Família program are added, which configure a mosaic of policies to support production and social protection that, through their financial resources, generate social stability in a region historically marked by poverty and recurrent drought [91, 92].
Index SDG 6 has a different spatial pattern from SDG 2, a better performance, and the most relevant response to governance indicators (Figure 7). Indicators of “coverage of the Brazil’s Cisternas program” and “basic sanitation plan” are considered the most important for the SDG 6 composition in governance terms. The first is a public policy of water access in the northeast region, which has been crucial for coexistence with the semi-arid regions in rural areas, and the second one is a legal management instrument of water and sanitation services, drainage, and waste management provided for by federal law. Thus, the water access issue is the most important indicator for SDGs 2 and 6 because water is an important input to agriculture production, and the major target of SDG 6, and the response of this policy gains strength from demand, given the water scarcity of the Brazilian semi-arid region [91]. The region has high socio-ecological vulnerability associated with conditions of rural poverty and access to water resources, which has historically been remedied by emergency measures and top–down approaches [18]. Federal social protection actions have been implemented since the 2000s with a new paradigm of coexistence rather than combating drought, in which the focus is on “the decentralization of access to water through local solutions, experiments, adaptations, and use of technologies appropriate to the Caatinga biome” [93]. Thus, the main paradigm is the capture and storage of rainwater for later use, converging toward the concept of sustainable development [94]. That said, governance related to the water silo requires considering socio-ecological inequities in a territorialized manner, with community and social participation playing strategic roles in the engagement of multilevel governance systems—polycentric systems (decentralized and coordinated)—that present better management and development of water resources than centralized arrangements as they promote more constructive forms of institutional interaction [18, 95–97].
In this sense, the “Cisternas” program, one of the governance indicators used for sensitivity analysis, demonstrates the importance of vertical relationships between actors acting at different administrative levels for SDG 6, with synergies for SDG 2. The Brazilian Semi-Arid Articulation (ASA), an entity that aggregates hundreds of non-governmental organizations (NGOs), churches, and social movements in the semi-arid region, was the one who first promoted the construction of cisterns in the region as a social technology for coexistence with droughts in 1990, which later was gradually incorporated by the state in its public policy list [94]. The program focuses on low-income rural families affected by drought or regular water shortages, with priority given to traditional peoples and communities, which allowed that in 2021, 963,985 cisterns had been built for consumption, 165,548 for production, and 7,592 for school cisterns. According to [94], the program’s contribution is more evident for goal 6.1 (universal and equitable access to water), a fact also observed by the present study, since the population, which is mainly rural, is dispersed throughout the territory. This fact, associated with the low water availability associated with the climatic conditions of the semi-arid region and the Caatinga biome, justifies the investment in cisterns as a social technology for water storage, whose cost is reduced and clearly contributes to the achievement of water SDG target 6.1. Positive impacts are also observed for food security in the case of production cisterns, as they guarantee the watering of animals and the irrigation of small lands, assets that have significant patrimonial relevance for the rural families of the semi-arid region [94].
The SDG 7 pattern has different conduct than the other two dimensions of Nexus (water and food), both in the performance of the index—more important in the Caatinga and semi-arid region and with better response to governance indicators in the State of Maranhão, extreme southeast of Pará, and more sparsely in Paraíba (Figure 7). SDG 7 has one governance indicator “public investment in infrastructure in relation to GDP.” In addition to the differences between SDG 7, in all three extremes, southeast of Pará presents low performance to the Nexus index and is also an important response to governance (Figure 7). It is important to note that for SDG 7, the municipalities of the Caatinga continue to predominate among the most dependent in terms of governance to achieve the goals, together with the state of Maranhão. In these places, the values of the SDG 7 index were the lowest, and even so, they were the ones that least benefited from investments in infrastructure to guarantee access to energy. Although Brazil has evolved a lot in the universalization of access to electric energy, especially after the “Luz para Todos”—Light for all in English—program in 2003, there are still few communities located in areas further away from the distribution networks, which still do not have access to the public electric energy service, mainly in rural areas [98].
Brazil still needs to face the challenge of increasing available alternatives and access to renewable energy, expanding, and strengthening federal public policies to increase access to and use of modern energy sources [99, 100]. It is common sense that this scope could help reduce social and regional inequalities since the existence of a proper solution for energy supply, or even access to energy from the distribution company, has not, by itself, provided adequate conditions to promote regional development [99, 100]. About the energy transition, a study that proposed a qualitative model of analysis of the Nexus including the land in the Nexus (water–energy–food–land) presents the relevance of the nexus approach to “integrate the development of policies, governance, and stakeholder actions to support cost-effective decisions for optimal resource management and regulatory processes, while enabling better integration of scientific insight and policy-making” [101]. In this sense, a series of barriers are pointed out for the universalization of access to renewable energies that are urgent in the country, such as regulatory obstacles, high costs of new technologies, and limited availability of infrastructure for these sources, the latter being pointed out by the sensitivity analysis of the present study [100].
4.3 Comparative analysis with the Sustainable City Development Index (IDSC)
In this section, we performed a comparative analysis with the IDSC-BR initiative as a step of validation expected in our methodology. The IDSC-BR initiative, focused on the 2030 Agenda, combines composite measures and panels to inform policies for achieving integrated goals, a necessary condition for assessing the SDGs [64].
Although IDSC-BR is the closest initiative for comparing performance and results for Brazil, the methodology has differences that require a parsimonious comparison with Nexus, mainly regarding the indicators’ calculation process and the number/type of indicators used to assess each SDG.
We highlight the differences between the calculation of the indicator; the frequency of indicators in SDGs 2, 6, and 7; and spatial distribution in SDGs 2, 6, and 7. Conversely, there are similarities in SDG indexes 1, 7, and 17. We further mention some peculiarities and technical information that may contribute to the results of this paper.
The IDSC-BR uses 100 indicators to assess the distance to achieving the targets of the SDG objectives in the 5,570 Brazilian municipalities [25]. These data come from national public and official sources [25].
For the calculation of indicators, IDSC-BR used specific values to not only truncate the upper limits of each indicator, mainly absolute target limits, but also the average of the five best performances depending on the indicator [25, 64]. For lower bounds, data truncation was carried out by removing the lower 2.5 percentiles from the distribution; for persisting outliers, an intermediate value between the outlier and the normal distribution was chosen [25]. The index result is then the arithmetic mean of the targets for each of the SDGs.
This calculation process mentioned previously shows that the values presented by the IDSC-BR do not vary between the minimum and maximum possible, making possible comparisons between the indexes of each SDG, the regions, and our products difficult.
In the analysis of the indicators, the IDSC-BR used ascending and descending terms to describe the behavior of the indicators in each municipality based on a historical series, while the Nexus works with the average or rates of historical values to produce indicators referring to time series.
All these methodological choices lead to a different presentation and interpretation of the results. The colors of the IDSC-BR maps show how far each Brazilian municipality is from reaching the SDG goal, as the goals’ limits are considered in the evaluation. Conversely, the SDG index of this work represents the municipality’s performance during the historical series, reflecting its position relative to the others in the theme of each SDG.
Regarding the frequency differences of indicators and themes for SDG 2, the IDSC-BR has five indicators, while the Nexus compiles 40 focused on the environmental dimension related to eradicating hunger and sustainable agriculture, such as land degradation, poor pasture conditions, use of pesticides, and drought-related agricultural losses.
In SDG 6, there are five indicators used in IDSC-BR and 25 in the Nexus. The first focus is mainly on basic sanitation (i.e., water, sewage, and garbage collection), while the present work also includes water quality indicators, environmental aspects related to the maintenance of water resources, and community participation.
Finally, for SDG 7, IDSC-BR utilizes two indicators and Nexus 9, focusing on the first being accessible energy. Our index also evaluates clean energy when working with indicators such as charcoal and firewood extraction and electricity production from different sources.
Comparing the spatial distribution of the SDG index and the IDSC-BR referring to SDG 2, one sees a greater convergence between both for positive results for the Caatinga biome, in which the eradication of hunger and family farming receive strong support from state public policies. For the Cerrado biome, the discrepancies are greater, as the IDSC-BR indicates greater difficulty for municipalities in this region to meet SDG 2, which presents convergence to the mapping of food and nutrition insecurity in Brazil [102], while the SDG index presents counties with high values. The contradiction can be explained by the scope of the indicators used in the Nexus, and the frequency of indicators related to sustainable agricultural production and public governance mechanisms in agriculture is higher than that of indicators related to food security and policies to eradicate hunger.
Analyzing the SDG index and the IDSC-BR for the SDG 6, the similarities are concentrated in the southeast region, where access to clean water and sanitation is greater than in the IDSC-BR. Marked differences can be observed between the indexes for the Caatinga and the northern portion of the Cerrado biome, in which the IDSC-BR presents many red municipalities with challenges to reach SDG 6 fullness. However, the SDG index shows a heterogeneous distribution of values.
Once again, we punctuate that differences are related to the more holistic view of the nexus approach against the IDSC-BR one, mainly related to the protection and use of water resources, which is absent in the IDSC-BR.
Finally, the indices for the SDG 7 showed agreement, where municipalities with the lowest performance in the Caatinga and the border regions between the two biomes show a predominance of access to electricity in the southeast of the country.
The following factors explain the discrepancy in the use of indicators, which also reflect on the following possible evaluations:
1) the calculation method of the SDG indexes in this work is based on the adherence of the indicators to the goals and SDGs, allowing an indicator to be considered relevant to more than one goal or SDG;
2) the conceptual/theoretical model that supports the choice and construction of indicators differs between projects—while the IDSC-BR intends to equip municipal public management to comply with the 2030 Agenda—choosing one indicator per target—the NIP is based on the transition to sustainability anchored in the water–energy–food nexus, with adherence to the SDG indexes built on these pillars;
3) we propose the NOI that integrates synergistic information from the ODS indexes, the NSI, in addition to the NPI, as the Nexus cannot be seen only as the sum of performances. This methodological perspective enables the Nexus to assess the scope of the 2030 Agenda for the municipalities studied with thematic foci more relevant to a chain of impacts in the water–energy–food triad, which needs to be more deeply analyzed or the focus of the IDSC-BR.
We also highlight some similarities between the results. The IDSC-SDGs, most likely the SDG indexes, were, in order, 17, 1, and 7. The IDSC-BR is close to the NPI, but the lowest values are concentrated in all northeast states, especially in almost all Maranhão, Piauí, and western Bahia.
In addition to Tocantins, and moderately in Mato Grosso, while in the NPI the lowest values are also in the northeast, the concentration of the worst values is present in smaller areas, in the central area of Maranhão and Bahia, in the sub-middle stretch region of the São Francisco River, and more moderately in Tocantins, northern Minas Gerais, and Mato Grosso.
5 Conclusion
The SDGs have many transversal targets, and therefore, there are repetitions of indicators among them, in addition to having greater availability of data from secondary sources for the Cerrado and Caatinga biomes; for instance, from IBGE, ANA, and DataSUS [103], it was affirmed that some challenges faced in Brazil to produce the SDG environmental indicators are
1. Institutional fragility in the production of part of the primary environmental information because, depending on resources, some of the information may not have continuity assured.
2. The spreading of information by many institutions implies the expenditure of time in obtaining and gathering information.
3. Some statistics depend on the effort spent obtaining the information and the intensity and scope of the surveys, such as those dependent on the efforts made by inspecting environmental and policy agencies.
4. Part of the environmental information produced is point and “snapshot” values, which raises the question of how to transform them into national indicators produced by state and municipal environmental agencies, whose objective is to provide data for monitoring.
5. Irregularity in producing environmental information, that is, surveys without defined periodicity, is very dependent on budgetary issues, making it challenging to create time series.
Achieving the SDGs depends on using relevant, reliable, and timely data to measure progress, inform policy, and target areas needing improvement. Traditional data sources have administrative and technical limitations in terms of access; standardization and quality of data; lack of awareness of the benefits of current technologies; lack of financial resources, technology, and skill gaps; geographic constraints (in terms of the development of spatial projects); and gaps in coverage in space and time [104].
The methodology presented is also situated in the field of socio-environmental perception studies. Therefore, uncertainty is pertinent because there are inherent value judgments and because many targets are broad meaning and their interpretation can be compatible with many indicators, even in a direct sense. Consequently, there is a difficulty in selecting and qualifying indicators in relation to broad targets. Thus, apart from the values of the variables, the result of this study, mainly the target response weights, reflects the perception of the group of experts in the years 2020–2021. However, it is worth noting that the group consulted is made up of researchers who deal with the social and environmental issues of the Nexus Project.
Despite the uncertainties, the use of indicators considering only normalization techniques leaves aside issues of target interpretation. The trajectory to achieve the SDGs goes through the interpretation of these by managers, which are in some way monitored by indicators. Therefore, this methodology can also be seen as a way for a collective, or decision-makers, to understand which indicators best respond to assess the sustainability trajectory, from the point of view of the 2030 Agenda and the Nexus.
The next challenge for this workgroup is i) to expand the scope to the national level; ii) encompass two historical moments because the 2022 Brazilian demographic census will be launched; iii) include geographic clusters for different normalization by indicator categories; iv) include target reference measures, as the IDSC did; v) include more experts and regional stakeholders for the selection and weighting indicators; and vi) research possible validations with real situation.
Data availability statement
The original contributions presented in the study are included in the article/Supplementary Material; further inquiries can be directed to the corresponding author.
Author contributions
GA contributed to the conceptualization, methodology, investigation, indicators construction, formal analysis, supervision, writing—original draft and review. JM contributed to the Investigation, data curation, metadata, validation, writing—original draft and review. MP contributed to the Investigation, data curation, validation, visualization, writing—original draft and review. JB contributed to the data curation, visualization, especially writing the geographical area of study section. JF contributed to the data curation, formal analysis and some writing. EB contributed to the conceptualization, writing discussion and review of the manuscript. PD contributed to the review of the manuscript. SP contributed to the review of the manuscript. JO contributed to the review of the manuscript, funding acquisition and project administration.
Funding
The funding was provided by “FAPESP,” project number: 2017/22269-2.
Acknowledgments
This study is part of a scientific project called Nexus, involving distributed research institutions along the country, whose objective is “to propose solutions using a novel participative approach, integrating quantitative and qualitative methods of natural and social sciences at multiple scales, to support the transformation to sustainability in the Cerrado and Caatinga biomes by proposing pathways, scenarios, and indicators reconciling the three pillars of sustainability for the use of natural resources and production of food and energy” [37]. This study was made possible, thanks to the participation of the Thematic Group’s experts of the Nexus Project, Ladis-lab team (https://www.ladis-inpe.com/), and funding from the Research Support Foundation of the State of São Paulo (FAPESP) for the Nexus Project (Transition to sustainability and the water–agriculture–energy nexus: exploring an integrative approach Cerrado e Caatinga biomes study cases) (Project number FAPESP 2017/22269-2).
Conflict of interest
The authors declare that the research was conducted in the absence of any commercial or financial relationships that could be construed as a potential conflict of interest.
Publisher’s note
All claims expressed in this article are solely those of the authors and do not necessarily represent those of their affiliated organizations, or those of the publisher, the editors, and the reviewers. Any product that may be evaluated in this article, or claim that may be made by its manufacturer, is not guaranteed or endorsed by the publisher.
Supplementary material
The Supplementary Material for this article can be found online at: https://www.frontiersin.org/articles/10.3389/fphy.2022.1060182/full#supplementary-material
Footnotes
1This range was determined from observations of cutoff points with the number of indicators that would be excluded, indicating that there should not be many exclusions related to this factor.
2Short SDG descriptions: Goal 1: Poverty eradication—end poverty in all its forms everywhere. Goal 2: Zero hunger—end hunger, achieve food security and improved nutrition, and promote sustainable agriculture. Goal 3: Good health and wellbeing—ensure healthy lives and promote wellbeing for all at all ages. Goal 4: ensure inclusive and equitable quality education and promote lifelong learning opportunities for all. Goal 5: Gender equality—achieve gender equality and empower all women and girls. Goal 6: Clean water and sanitation—ensure the availability and sustainable management of water and sanitation for all. Goal 7: Affordable and clean energy—ensure access to affordable, reliable, sustainable, and modern energy for all. Goal 8: Decent work and economic growth—promote sustained, inclusive, and sustainable economic growth, full and productive employment, and decent work for all. Goal 9: Industry, innovation, and infrastructure—build resilient infrastructure, promote inclusive and sustainable industrialization, and foster innovation. Goal 10: Reduced inequalities—reduce inequality within and among countries. Goal 11: Sustainable cities and communities—make cities and human settlements inclusive, safe, resilient, and sustainable. Goal 12: Responsible consumption and production—ensure sustainable consumption and production patterns. Goal 13: Climate action—take urgent action to combat climate change and its impacts. Goal 14: Life below water—conserve and sustainably use the oceans, seas, and marine resources for sustainable development. Goal 15: Life on land—protect, restore, and promote sustainable use of terrestrial ecosystems, sustainably manage forests, combat desertification, and halt and reverse land degradation, and halt biodiversity loss. Goal 16: Peace, justice, and strong institutions—promote peaceful and inclusive societies for sustainable development; provide access to justice for all; and build effective, accountable, and inclusive institutions at all levels. Goal 17: Partnerships—strengthen the means of implementation and revitalize the Global Partnership for Sustainable Development.
References
1. Rockström J, Steffen W, Noone K, Persson Å, Chapin FS, Lambin E, et al. Planetary boundaries: Exploring the safe operating space for humanity. E&S (2009) 14(2):art32. doi:10.5751/es-03180-140232
2. Crutzen PJ. “The Anthropocene,” in Earth System Science in the Anthropocene. Editors E. Ehlers, and T. Krafft (Berlin: Springer), (2006) 13–8. doi:10.1007/3-540-26590-2_3
3. Simpson GB, Jewitt GPW. The development of the water-energy-food nexus as a framework for achieving resource security: A review. Front Environ Sci (2019) 7:8. doi:10.3389/fenvs.2019.00008
4. Scott CA, Kurian M, Wescoat JL. The water-energy-food nexus: Enhancing adaptive capacity to complex global challenges. In: Governing the nexus. Cham: Springer International Publishing (2015). p. 15–38.
6. Pahl-Wostl C. Governance of the water-energy-food security nexus: A multi-level coordination challenge. Environ Sci Pol (2019) 92:356–67. doi:10.1016/j.envsci.2017.07.017
7. Bhaduri A, Ringler C, Dombrowski I, Mohtar R, Scheumann W. Sustainability in the water–energy–food nexus. Water Int (2015) 40(5–6):723–32. doi:10.1080/02508060.2015.1096110
8. Biggs EM, Bruce E, Boruff B, Duncan JMA, Horsley J, Pauli N, et al. Sustainable development and the water-energy-food nexus: A perspective on livelihoods. Environ Sci Pol (2015) 54:389–97. doi:10.1016/j.envsci.2015.08.002
9. Carvalho PN, Finger DC, Masi F, Cipolletta G, Oral HV, Tóth A, et al. Nature-based solutions addressing the water-energy-food nexus: Review of theoretical concepts and urban case studies. J Clean Prod (2022) 338:130652. doi:10.1016/j.jclepro.2022.130652
10. Liu Q. Interlinking climate change with water-energy-food nexus and related ecosystem processes in California case studies. Ecol Process (2016) 5(1):14. doi:10.1186/s13717-016-0058-0
11. Albrecht TR, Crootof A, Scott CA. The water-energy-food nexus: A systematic review of methods for nexus assessment. Environ Res Lett (2018) 13(4):043002. doi:10.1088/1748-9326/aaa9c6
12. Karnib A. A quantitative nexus approach to analyze the interlinkages across the sustainable development goals. J Sustain Dev (2017) 10(5):173. doi:10.5539/jsd.v10n5p173
13. Endo A, Burnett K, Orencio PM, Kumazawa T, Wada CA, Ishii A, et al. Methods of the water-energy-food nexus. Water (Basel) (2015) 7(10):5806–30. doi:10.3390/w7105806
14. Liu J, Hull V, Godfray HCJ, Tilman D, Gleick P, Hoff H, et al. Nexus approaches to global sustainable development. Nat Sustain (2018) 1(9):466–76. doi:10.1038/s41893-018-0135-8
15. Urbinatti AM, Benites-Lazaro LL, Carvalho CM, Giatti LL. The conceptual basis of water-energy-food nexus governance: Systematic literature review using network and discourse analysis. J Integr Environ Sci (2020) 17(2):21–43. doi:10.1080/1943815x.2020.1749086
16. Martin MA, Sendra OA, Bastos A, Bauer N, Bertram C, Blenckner T, et al. Ten new insights in climate science 2021: A horizon scan. Glob Sustain (2021) 4:e25. doi:10.1017/sus.2021.25
17. Steffen W, Richardson K, Rockström J, Schellnhuber HJ, Dube OP, Dutreuil S, et al. The emergence and evolution of earth system science. Nat Rev Earth Environ (2020) 1(1):54–63. doi:10.1038/s43017-019-0005-6
18. Araujo M, Ometto J, Rodrigues-Filho S, Bursztyn M, Lindoso DP, Litre G, et al. The socio-ecological Nexus+ approach used by the Brazilian research network on global climate change. Curr Opin Environ Sustainability (2019) 39:62–70. Elsevier B.V.
19. Bornemann B, Weiland S. The un 2030 agenda and the quest for policy integration: A literature review. PaG (2021) 9(1):96–107. doi:10.17645/pag.v9i1.3654
20. Xue L, Weng L, Yu H. Addressing policy challenges in implementing sustainable development goals through an adaptive governance approach: A view from transitional China. Sust Develop (2018) 26(2):150–8. doi:10.1002/sd.1726
21. Boas I, Biermann F, Kanie N. Cross-sectoral strategies in global sustainability governance : Towards a nexus approach. Int Environ Agreements (2016) 16(3):449–64. doi:10.1007/s10784-016-9321-1
22. Nilsson M, Griggs D, Visbeck M. Policy: Map the interactions between sustainable development goals. Nature (2016) 534(7607):320–2. doi:10.1038/534320a
23. Dalla Fontana M, Moreira Fde A, di Giulio GM, Malheiros TF. The water-energy-food nexus research in the Brazilian context: What are we missing? Environ Sci Pol (2020) 112:172–80. doi:10.1016/j.envsci.2020.06.021
24.IPEA. Agenda 2030: Ods. In: DS ERA, AM Peliano, and JV Chaves, editors. Metas nacionais dos objetivos de desenvolvimento sustentável [Internet]. Brazil: Instituto de Pesquisa Econômica Aplicada (2018). [cited 2022 Sep 30]. Available from: http://repositorio.ipea.gov.br/bitstream/11058/8855/1/Agenda_2030_ods_metas_nac_dos_obj_de_desenv_susten_propos_de_adequa.pdf. (Accessed April 23, 2022).
25.IDSC. UN Sustainable Development Solution Network - SDSN. Índice de Desenvolvimento Sustentável das Cidades (IDSC) (2022). [Internet]. 2022. Available from: https://idsc.cidadessustentaveis.org.br/. (Accessed June 15, 2022).
26. Hoff H. Understanding the nexus: Background Paper for the Bonn 2011. In: The Water, Energy and Food Security Nexus, Stockholm (Bonn, Germany: Stockholm Environment Institute)(2011).
27. Ometto JPHB, Paz MG, Branco EA, Arcoverde GFB, Pulice SMP, Barros JD, et al. Collaborative methodological approaches towards water-energy-food nexus. In: Fde A Moreira, MD Fontana, TF Malheiros, and GM di Giulio, editors. The water-energy-food nexus: What the Brazilian research has to say. 1st ed. São Paulo: Faculdade de Saúde Pública da USP (2022). p. 213–27.
28. Benites-Lazaro LL, Giatti LL, Sousa Junior WC, Giarolla A. Land-water-food nexus of biofuels: Discourse and policy debates in Brazil. Environ Dev (2020) 33:100491. doi:10.1016/j.envdev.2019.100491
29. Funtowicz SO, Ravetz JR. Science for the post-normal age. Futures (1993) 25(7):739–55. doi:10.1016/0016-3287(93)90022-l
30. Mercure JF, Knobloch F, Pollitt H, Paroussos L, Scrieciu SS, Lewney R. Modelling innovation and the macroeconomics of low-carbon transitions: Theory, perspectives and practical use. Clim Pol (2019) 19(8):1019–37. doi:10.1080/14693062.2019.1617665
31. Lahsen M, Bustamante MMC, Dalla-Nora EL. Undervaluing and overexploiting the Brazilian Cerrado at our peril. Environment. Sci Pol Sust Develop (2016) 58(6):4–15. doi:10.1080/00139157.2016.1229537
32. Klink CA, Machado RB. Conservation of the Brazilian Cerrado. Conservation Biol (2005) 19(3):707–13. doi:10.1111/j.1523-1739.2005.00702.x
33. Medeiros Sde S, Cavalcante Ade MB, Marin AMP, Tinôco LBde M, Salcedo IH, Pinto TF. Sinopse do Censo Demográfico para o Semiárido Brasileiro. Campina Grande: IBICT (2012).
34. Ribeiro EMS, Arroyo-Rodríguez V, Santos BA, Tabarelli M, Leal IR. Chronic anthropogenic disturbance drives the biological impoverishment of the Brazilian Caatinga vegetation. J Appl Ecol (2015) 52(3):611–20. doi:10.1111/1365-2664.12420
35. Ometto JPHB, da Paz MGA, Branco EA, Arcoverde GFB, Sérgio M, Pulice P, et al. Collaborative methodological approaches towards Water-Energy-Food nexus. In: F de Araujo Moreira, MD Fontana, T Fabrício Malheiros, and G Marques Di Giulio, editors. The water-energy-food nexus - what the Brazilian research has to say. 1st ed. São Paulo: Universidade de São Paulo-Faculdade de Saúde Pública (2022). p. 213–27.
36. Mucina L. Biome: Evolution of a crucial ecological and biogeographical concept. New Phytol (2019) 222(1):97–114. doi:10.1111/nph.15609
37.IBGE. Brasil em síntese (2021). [Internet]. [cited 2021 Jul 28]. Available from: https://brasilemsintese.ibge.gov.br/ (Accessed June 28, 2021).
39. Rangel TFLVB, Bini LM, Diniz-Filho JAF, Pinto MP, Carvalho P, Bastos RP. Human development and biodiversity conservation in Brazilian Cerrado. Appl Geogr (2007) 27(1):14–27. doi:10.1016/j.apgeog.2006.09.009
40.IBGE. Mapas da produção agropecuária (2020) [Internet]. 2020 [cited 2022 Aug 23]. Available from: Mapas da produção agropecuária.
41. Soterroni AC, Ramos FM, Mosnier A, Fargione J, Andrade PR, Baumgarten L, et al. Expanding the soy moratorium to Brazil’s Cerrado. Sci Adv (2019) 5(7):eaav7336. doi:10.1126/sciadv.aav7336
42. Rekow L. Socio-ecological implications of soy in the Brazilian Cerrado. Challenges in Sustainability (2019) 7(1):7–29. doi:10.12924/cis2019.07010007
43. Lopes GR, Lima MGB, Reis TNP. Maldevelopment revisited: Inclusiveness and social impacts of soy expansion over Brazil’s Cerrado in Matopiba. World Develop (2021) 139:105316. doi:10.1016/j.worlddev.2020.105316
44. Abreu RCR, Hoffmann WA, Vasconcelos HL, Pilon NA, Rossatto DR, Durigan G. The biodiversity cost of carbon sequestration in tropical savanna. Sci Adv (2017) 3(8):e1701284. doi:10.1126/sciadv.1701284
45. Almeida CA, Valeriano DM, Maurano L, Vinhas L, Fonseca LMG, Silva D, et al. Deforestation monitoring in different Brazilian biomes: Challenges and lessons. In: 2020 IEEE Latin American GRSS & ISPRS Remote Sensing Conference (LAGIRS); 22-26 March 2020; Santiago, Chile. IEEE (2020). p. 357–62.
47.UNCCD. Intergovernmental Negotiating Committee for the elaboration of an International Convention to Combat Desertification in those countries experiencing serious drought and/or desertification, particularly in Africa. Assembly. (1994).
48. Maia JM, Sousa V, Lira E, Lucena A. Motivações socioeconômicas para a conservação e exploração sustentável do bioma Caatinga. Desenvolv Meio Ambiente (2017) 41:41. doi:10.5380/dma.v41i0.49254
49.MDR - Ministério do Desenvolvimento Regional. Resolução condel/sudene No 150, de 13 de dezembro de 2021. Delimitação do semiárido (2021). [Internet]. 2021. Available from: https://www.gov.br/sudene/pt-br/centrais-de-conteudo/resolucao1502021.pdf (Accessed February 15, 2022).
50. Rufino IAA, Silva ST. Análise das relações entre dinâmica populacional, clima e vetores de mudança no semiárido brasileiro: Uma abordagem metodológica. Bol Cienc Geod (2017) 23(1):166–81. doi:10.1590/s1982-21702017000100011
51. Bezerra FGS, Aguiar APD, Alvalá RCS, Giarolla A, Bezerra KRA, Lima PVPS, et al. Analysis of areas undergoing desertification, using EVI2 multi-temporal data based on MODIS imagery as indicator. Ecol Indic (2020) 117:106579. doi:10.1016/j.ecolind.2020.106579
52. Strassburg BBN, Brooks T, Feltran-Barbieri R, Iribarrem A, Crouzeilles R, Loyola R, et al. Moment of truth for the Cerrado hotspot. Nat Ecol Evol (2017) 1(4):0099. doi:10.1038/s41559-017-0099
53. Lima JEFW, Silva E, Oliveira-Filho EC, Martins ES, Reatto A. The relevance of the cerrado’s water resources to the Brazilian development. In: XIVth IWRA World Water Congress. Porto de Galinhas: International Water Resources Association; September 25-29, 2011; Porto de Galinhas, Pernambuco (2011).
54. Pinheiro EAR, Lier Q, Bezerra AHF. Hydrology of a water-limited forest under climate change scenarios: The case of the caatinga biome, Brazil. Forests (2017) 8(3):62. doi:10.3390/f8030062
55. Santos WA, Erthal Junior M, Barcellos RGS. Biocapacity of Brazilian biomes using emergy ecological footprint concepts. Ambient Soc (2021) 24:1–23. doi:10.1590/1809-4422asoc20180134r2vu2021l5ao
56. Miranda EE, Magalhães LA, Carvalho CA. Proposta de delimitação territorial do Matopiba (2014). [Internet]. 2014 [cited 2022 Aug 24]. Available from: https://www.infoteca.cnptia.embrapa.br/infoteca/bitstream/doc/1037313/1/NT1DelimitacaoMatopiba.pdf (Accessed August 24, 2022).
57. Maggino F. Developing indicators and managing the complexity. In: Complexity in society: From indicators construction to their synthesis. New York, United States: Springer (2017). p. 87–114.
58. Finn A, Wang L. Formative vs. reflective measures: Facets of variation. J Business Res (2014) 67(1):2821–6. doi:10.1016/j.jbusres.2012.08.001
59. Gallopin GC. Environmental and sustainability indicators and the concept of situational indicators. A systems approach. Environ Model Assess (1996) 1(3):101–17. doi:10.1007/bf01874899
60. Poncela P, Nardo M, Pericoli FM. A review of international risk sharing for policy analysis. East Asian Econ Rev (2019) 23(3):227–60. doi:10.11644/kiep.eaer.2019.23.3.362
61. Turnhout E, Hisschemöller M, Eijsackers H. Ecological indicators: Between the two fires of science and policy. Ecol Indicators (2007) 7(2):215–28. doi:10.1016/j.ecolind.2005.12.003
62. Santos ME, Villatoro P. The importance of reliability in the multidimensional poverty index for Latin America (MPI-LA). J Develop Stud (2019) 56(9):1784–9. doi:10.1080/00220388.2019.1663177
63. Beccari B. A comparative analysis of disaster risk, vulnerability and resilience composite indicators. Plos Curr (2016) 8:453df025e34b682e9737f95070f9b970. doi:10.1371/currents.dis.453df025e34b682e9737f95070f9b970
64. Sachs JD, Schmidt-Traub G, Durand-Delacre D. Preliminary sustainable development goal (SDG) index and dashboard. Sust Develop Solutions Netw (2016) 15:24–7.
65. Nardo M, Saisana M, Saltelli A, Tarantola S, Hoffmann A, Giovannini E. Handbook on constructing composite indicators: Methodology and user guide. Paris: Organization for Economic Co-operation and Development (2008). p. 162. JRC (JRC), editor. Paris: Organisation for Economic Co-operation and Development (OECD)Available from: https://www.oecd.org/els/soc/handbookonconstructingcompositeindicatorsmethodologyanduserguide.htm (Accessed March 18, 2021).
66. Becker W, Benavente D, Dominguez Torreiro M, Moura C, Neves A, Saisana M, et al. COIN tool user guide [internet]. Luxemburgo: Publications Office of the European Union (2019). p. 118407. [cited 2022 Sep 30]. p. Available from: https://op.europa.eu/en/publication-detail/-/publication/310f6210-fba8-11e9-8c1f-01aa75ed71a1/language-en (Accessed September 30, 2022).
67.Ipea. Índice de Desenvolvimento humano municipal brasileiro. Brasília: Instituto de Pesquisa Econômica Aplicada (2013).
68. Hunke P, Mueller EN, Schröder B, Zeilhofer P. The Brazilian Cerrado: Assessment of water and soil degradation in catchments under intensive agricultural use. Ecohydrol (2015) 8(6):1154–80. doi:10.1002/eco.1573
69. Vieira RRS, Ribeiro BR, Resende FM, Brum FT, Machado N, Sales LP, et al. Compliance to Brazil’s Forest Code will not protect biodiversity and ecosystem services. Divers Distrib (2018) 24(4):434–8. doi:10.1111/ddi.12700
70. Assis LFFG, Ferreira KR, Vinhas L, Maurano L, Almeida C, Carvalho A, et al. TerraBrasilis: A spatial data analytics infrastructure for large-scale thematic mapping. ISPRS Int J Geo-Information (2019) 8 (513):1–27. Available from: https://www.mdpi.com/2220-9964/8/11/513
72. Yumkella KK, Yillia PT. Framing the water-energy nexus for the post-2015 development agenda. Aquat Proced (2015) 5:8–12. doi:10.1016/j.aqpro.2015.10.003
73. Weitz N, Nilsson M, Davis M. A nexus approach to the post-2015 agenda: Formulating integrated water, energy, and food SDGs. SAIS Rev Int Aff (2014) 34(2):37–50. doi:10.1353/sais.2014.0022
74. Terrapon-Pfaff J, Ortiz W, Dienst C, Gröne MC. Energising the WEF nexus to enhance sustainable development at local level. J Environ Manage (2018) 223:409–16. doi:10.1016/j.jenvman.2018.06.037
75. Barros PHB, Stege AL. Socioeconomic development and deforestation in the Cerrado biome, Brazil: Spatial interactions and heterogeneity. Revista Brasileira de Estudos Regionais e Urbanos (2022) 15(3):393–415. doi:10.54766/rberu.v15i3.792
76. Martinelli LA, Naylor R, Vitousek PM, Moutinho P. Agriculture in Brazil: Impacts, costs, and opportunities for a sustainable future. Curr Opin Environ Sustainability (2010) 2(5–6):431–8. doi:10.1016/j.cosust.2010.09.008
77.BRASIL. Plano de Desenvolvimento Agropecuário do Matopiba e a criação de seu Comitê Gestor. Decreto no 8.447. [Internet]. Brasil, 2015 1. Available at: https://www.planalto.gov.br/ccivil_03/_ato2015-2018/2015/decreto/d8447.htm.
78. Favareto A, Nakagawa L, Kleeb S, Seifer P, Pó M. Há mais pobreza E desigualdade do que bem estar E riqueza nos municípios do matopiba/there is more poverty and inequality than well-being and wealth in the municipalities of matopiba/hay más pobreza Y desigualdad que bienestar Y riqueza en los municipios de Matopiba. Rev NERA (2019) 47:348–81. doi:10.47946/rnera.v0i47.6275
80. Chiquito Gesualdo G, Sone JS, Galvão Cde O, Martins ES, Montenegro SMGL, Tomasella J, et al. Unveiling water security in Brazil: Current challenges and future perspectives. Hydrological Sci J (2021) 66(5):759–68. doi:10.1080/02626667.2021.1899182
81. Cunha APMA, Zeri M, Deusdará Leal K, Costa L, Cuartas LA, Marengo JA, et al. Extreme drought events over Brazil from 2011 to 2019. Atmosphere (Basel) (2019) 10(11):642. doi:10.3390/atmos10110642
82. Marengo JA, Bernasconi M. Regional differences in aridity/drought conditions over northeast Brazil: Present state and future projections. Climatic Change (2015) 129(1–2):103–15. doi:10.1007/s10584-014-1310-1
83. Cunha Libanio PA. Water reforms in Brazil: Challenges and opportunities for promoting water security in a continental-sized country. World Water Policy (2020) 6(2):230–45. doi:10.1002/wwp2.12042
85.Empresa de Pesquisa Energética (EPE). Anuário estatístico de Energia elétrica (2022). [Internet]. Rio de Janeiro, RJ; 2022. Available from: http://shinyepe.brazilsouth.cloudapp.azure.com:3838/anuario-livro/. (Accessed October 19, 2022)
86. Castro NJ, Dantas G, Brandão R, Moszkowicz M, Rosental R. Tdse 67: Perspectives and challenges of the diffusion of micro and mini generation photovoltaic solar in Brazil. Rio de Janeiro, RJ: GESEL (2016). Report No.: 67.
87. Diniz CC. Celso Furtado e o desenvolvimento regional. Nova Econ (2009) 19(2):227–49. doi:10.1590/s0103-63512009000200001
89. Pinto IV, Andrade SSde A, Rodrigues LL, Santos MAS, Marinho MMA, Benício LA, et al. Profile of notification of violence against lesbian, gay, bisexual, transvestite and transsexual people recorded in the national information system on notifiable diseases, Brazil, 2015-2017. Rev Bras Epidemiol (2020) 23. doi:10.1590/1980-549720200006.supl.1
90. Reddy VR, Cunha DGF, Kurian M. A water-energy-food nexus perspective on the challenge of eutrophication. Water (2018) 10 (101):1–13. (Switzerland). MDPI AG.
91. Aquino JR, Alves MO. Agricultura familiar No nordeste do brasil: Um retrato atualizado A partir dos dados do censo agropecuário 2017 family farming in Northeast of Brazil: An updated portrait from the data of the 2017 agricultural census. Rev Econ NE (2020) 51:31–54.
92. Schneider S, Cassol A. Diversidade E heterogeneidade da agricultura familiar No brasil E algumas implicações para políticas públicas. Brasília: Cadernos de Ciência & Tecnologia (2014). p. 227–63.
93. Gomes Pereira MC. Água E convivência com O semiárido: Múltiplas águas, distribuições E realidades (2016) [Internet] [Doctor]. [São Paulo]: FUNDAÇÃO GETULIO VARGAS; 2016 [cited 2022 Sep 28]. Available from: https://bibliotecadigital.fgv.br/dspace/handle/10438/17062. (Accessed September 28, 2022)
94. de Castro CN. Avaliação do Programa Nacional de Apoio à Captação de Água de Chuva e Outras Tecnologias Sociais (Programa Cisternas), à luz dos objetivos de Desenvolvimento Sustentável. Brasília: IPEA (2021). Report No.: N o 2722. doi:10.38116/td2722
95. Pahl-Wostl C, Knieper C. The capacity of water governance to deal with the climate change adaptation challenge: Using fuzzy set Qualitative Comparative Analysis to distinguish between polycentric, fragmented and centralized regimes. Glob Environ Change (2014) 29:139–54. doi:10.1016/j.gloenvcha.2014.09.003
96. Pahl-Wostl C, Gorris P, Jager N, Koch L, Lebel L, Stein C, et al. Scale-related governance challenges in the water–energy–food nexus: Toward a diagnostic approach. Sustain Sci (2021) 16(2):615–29. doi:10.1007/s11625-020-00888-6
97. Lebel L, Nikitina E, Pahl-Wostl C, Knieper C. Institutional fit and river basin governance: A new approach using multiple composite measures. E&S (2013) 18(1):art1. doi:10.5751/es-05097-180101
98. Ferreira AL, Silva FB. Universalização do acesso ao serviço público de energia elétrica no brasil: Evolução recente e desafios para a amazônia legal. Rev. Bras. Energ (2021) 27(3):135–54.
99. Kunz A, Otenio MH, Leitão RC, Gambetta R. Energia limpa e acessível: Contribuições da embrapa (2018). [Internet]. 2018 [cited 2022 Sep 27]. 4–56 p. Available from: https://ainfo.cnptia.embrapa.br/digital/bitstream/item/184236/1/ODS-7-energia-limpa-e-acessivel.pdf (Accessed September 27, 2022).
100. Geller H, Schaeffer R, Szklo A, Tolmasquim M. Policies for advancing energy efficiency and renewable energy use in Brazil. Energy Policy (2004) 32(12):1437–50. doi:10.1016/s0301-4215(03)00122-8
101. Lazaro LLB, Giatti LL, Bermann C, Giarolla A, Ometto J. Policy and governance dynamics in the water-energy-food-land nexus of biofuels: Proposing a qualitative analysis model. Renew Sust Energ Rev (2021) 149:111384. doi:10.1016/j.rser.2021.111384
102.CAISAN - Câmara Interministerial de Segurança Alimentar e Nutricional. Estudo Técnico CAISAN - mapeamento da Insegurança Alimentar e Nutricional com foco na Desnutrição a partir da análise do Cadastro Único, do Sistema Nacional de Vigilância Alimentar e Nutricional (SISVAN) e do Sistema de Informação da Atenção à Saúde Indígena (SIASI). Brasília: CAISAN (2016).
103. Kronemberger DMP. Os desafios da construção dos indicadores ODS globais. Ciência e cultura (2019). [Internet]. 2019 [cited 2022 Sep 27];71(1):40–5. Available from: http://cienciaecultura.bvs.br/scielo.php?pid=S0009-67252019000100012&script=sci_arttext&tln (Accessed September 27, 2022).
Keywords: sustainable development, composite indicators, socio-ecological system, biomes, governance, complexity
Citation: Arcoverde GFB, Menezes JA, Paz MGA, Barros JD, Guidolini JF, Branco EA, De Andrade PR, Pulice SMP and Ometto JPHB (2023) Sustainability assessment of Cerrado and Caatinga biomes in Brazil: A proposal for collaborative index construction in the context of the 2030 Agenda and the Water-Energy-Food Nexus. Front. Phys. 10:1060182. doi: 10.3389/fphy.2022.1060182
Received: 02 October 2022; Accepted: 05 December 2022;
Published: 09 January 2023.
Edited by:
Leonardo B. L. Santos, Cemaden, BrazilReviewed by:
Meiry Sayuri Sakamoto, Fundação Cearense de Meteorologia e Recursos Hídricos, BrazilArthur Valencio, North Carolina State University, United States
Copyright © 2023 Arcoverde, Menezes, Paz, Barros, Guidolini, Branco, De Andrade, Pulice and Ometto. This is an open-access article distributed under the terms of the Creative Commons Attribution License (CC BY). The use, distribution or reproduction in other forums is permitted, provided the original author(s) and the copyright owner(s) are credited and that the original publication in this journal is cited, in accordance with accepted academic practice. No use, distribution or reproduction is permitted which does not comply with these terms.
*Correspondence: Gustavo Felipe Balué Arcoverde, Z3VzdGF2by5hcmNvdmVyZGVAaW5wZS5icg==; Evandro Albiach Branco, ZXZhbmRyby5hbGJpYWNoQGlucGUuYnI=
†These authors have contributed equally to this work