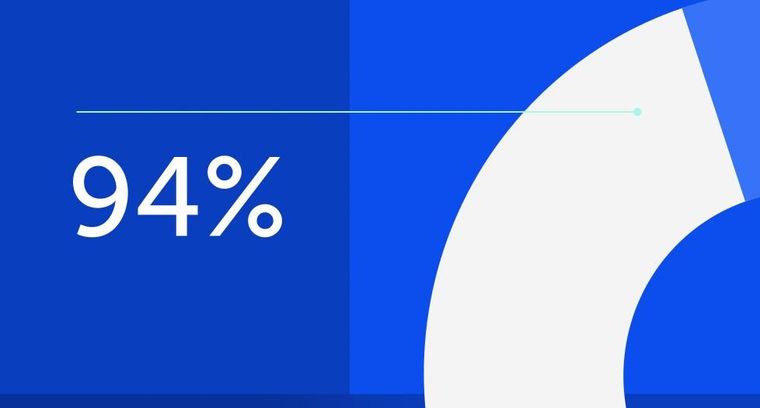
94% of researchers rate our articles as excellent or good
Learn more about the work of our research integrity team to safeguard the quality of each article we publish.
Find out more
PERSPECTIVE article
Front. Phys., 21 October 2022
Sec. Interdisciplinary Physics
Volume 10 - 2022 | https://doi.org/10.3389/fphy.2022.1050277
This article is part of the Research TopicTaking Stock in EconophysicsView all 5 articles
We discuss and analyze some recent literature that introduced pioneering methods in econophysics. In doing so, we review recent methods of estimating the volatility, volatility of volatility, and probability densities. These methods will have useful applications in econophysics and finance.
The volatility estimation is a key topic in finance and econophysics. It is an indicator of the movement in the asset price. For example, see [1,2]. Recently, the literature focused on the volatility of volatility. Examples include [3,4]. Closely related to the volatility estimation is the probability density estimation. The density estimation can be used to estimate the volatility and volatility of volatility. Needless to say, the probability density has many other applications.
In this note, we briefly discuss recent methods in the estimation of the volatility, volatility of volatility, and probability densities.
There are typically two methods of density estimation: parametric and non-parametric methods. For example, [5,6] adopted the parametric method. [7–13] used the non-parametric approach. [14–17] provided empirical estimation. [18] used copulas. [19] used histograms and numerical simulations. [20] employed orthogonal polynomials.
A limitation of the parametric method is that it requires knowing the marginal distributions [5,21]. While the bandwidth selection problems, the high computational cost, and the kernel specification are some of the limitations of the non-parametric approach.
In response to some of these limitations, [22] introduced non-parametric methods for estimating the marginal and joint probability densities. The advantage of these methods is their relative simplicity. In particular, it allows us to circumvent the bandwidth selection problem and the kernel specification. Accordingly, the joint density can be calculated as
where
Using Taylor’s expansions, [6, 23] introduced parametric methods for estimating the joint, marginal, conditional, and cumulative probability densities. In doing so, they relied on estimating regressions. For example, the joint density can be given by
where ci is a constant. The marginal density can be obtained by integrating the above equation.
The advantage of this method is its simplicity and the fact that the marginal distributions need not be known. Moreover, the estimation accuracy can be improved by increasing the order of the Taylor expansion. The limitation of this method is that we need to ensure the goodness-of-fit of the regression.
Previous literature on volatility typically considered time series, such as the generalized autoregressive conditional heteroskedasticity GARCH models. For example, see the excellent surveys by [1,2,24]. Asai and McAleer [25] adopted a Wishart stochastic volatility model. Bollerslev et al (2011) investigated asymmetry in volatility. Asai et al [26] assumed a noisy realized volatility. Muhle-Karbe et al [27] considered multivariate stochastic volatility. Sahiner [28] used the GARCH method. Mastroeni [29] considered vanishing stochastic volatility.
Alghalith [4] provided novel, parametric methods for estimating the volatility and volatility of volatility. According to this model, volatility data are needless. Also, the method can be applied to cross-sectional data. Furthermore, estimating the volatility matrix can be avoided. The limitation of this model is that we need to ensure the validity of the non-linear regression results.
Alghalith et al [30] introduced a simple, non-parametric method to estimate both the volatility and volatility of volatility. Accordingly, the volatility of the asset returns and volatility of volatility can be estimated, respectively, as
where St is the price of the asset (typically a stock) at time t and vt is the estimated volatility at time t.
where γt is the estimated volatility of volatility at time t.
Also, [30] explored the possibility that the volatility of volatility is not constant. The advantage of this approach is its simplicity. Its limitation is that it requires high-frequency data for a high level of accuracy.
In sum, these methods introduced by Alghalith and co-authors were reasonably accurate when they were applied to practical examples. In general, they were at least as accurate as other methods. However, the accuracy can be improved by increasing the frequency of the data or the order of the Taylor expansion.
We introduced simpler and less restrictive methods for estimating the volatility, volatility of volatility, and probability densities. In general, the other methods are more technical. Future research can utilize these methods of density estimation to estimate the volatility and volatility of volatility. Moreover, future research can apply these methods to other areas of econophysics.
The original contributions presented in the study are included in the article/supplementary material, further inquiries can be directed to the corresponding author.
The author confirms being the sole contributor of this work and has approved it for publication.
I’m very grateful to Editor SS and the reviewers for their excellent and fast comments.
The author declares that the research was conducted in the absence of any commercial or financial relationships that could be construed as a potential conflict of interest.
All claims expressed in this article are solely those of the authors and do not necessarily represent those of their affiliated organizations, or those of the publisher, the editors and the reviewers. Any product that may be evaluated in this article, or claim that may be made by its manufacturer, is not guaranteed or endorsed by the publisher.
1. Asai M, McAleer M. Alternative asymmetric stochastic volatility models. Econometric Rev (2011) 30:548–64. doi:10.1080/07474938.2011.553156
2. Caporin M, McAleer M. Do we really need both BEKK and DCC? A tale of two multivariate GARCH models. J Econ Surv (2012) 26:736–51. doi:10.1111/j.1467-6419.2011.00683.x
3. Corsi F, Mittnik S, Pigorsch C, Pigorsch U. The volatility of realized volatility. Econometric Rev (2008) 27:46–78. doi:10.1080/07474930701853616
4. Alghalith M. Estimating the stock/portfolio volatility and the volatility of volatility: A new simple method. Econometric Rev (2016) 35:257–62. doi:10.1080/07474938.2014.932144
5. Morimura T, Sugiyama M, Kashima H, Hashiya H, Tanaka T. Parametric return density estimation for reinforcement learning (2012). arXiv:1203.3497.
6. Alghalith M. A new parametric method of estimating the joint probability density: Revisited. Physica A: Stat Mech its Appl (2019) 527:121455. doi:10.1016/j.physa.2019.121455
7. Chen S. Optimal bandwidth selection for kernel density functionals estimation. J Probab Stat (2015) 2015:1–21. doi:10.1155/2015/242683
8. Zhang J. Generalized least squares cross-validation in kernel density estimation. Stat Neerlandica (2015) 69:315–28. doi:10.1111/stan.12061
9. Scricciolo C. Adaptive bayesian density estimation in lp-metrics with pitman-yor or normalized inverse-Gaussian process kernel mixtures. Bayesian Anal (2014) 9:475–520. doi:10.1214/14-ba863
10. Shen W, Tokdar ST, Ghosal S. Adaptive Bayesian multivariate density estimation with Dirichlet mixtures. Biometrika (2013) 100:623–40. doi:10.1093/biomet/ast015
11. Durante F, Okhren O. Estimation procedures for exchangeable Marshall copulas with hydrological application (2014). SFB 649 Discussion Paper 2014-014.
12. Hazlett C. Kernel balancing: A balancing method to equalize multivariate densities and reduce bias without a specification search (2014). MIT working paper. Available from: www.mit.edu/hazlett.
13. Weib G. Copula parameter estimation by maximum-likelihood and minimum-distance estimators: A simulation study. Comput Stat (2011) 26(1):31–54. doi:10.1007/s00180-010-0203-7
14. Sheikhpour R, Sarram MA, Sheikhpour R. Particle swarm optimization for bandwidth determination and feature selection of kernel density estimation based classifiers in diagnosis of breast cancer. Appl Soft Comput (2016) 40:113–31. doi:10.1016/j.asoc.2015.10.005
15. Talamakrouni M, Keilegom I, Ghouch A. Parametrically guided nonparametric density and hazard estimation with censored data. Comput Stat Data Anal (2016) 93:308–23. doi:10.1016/j.csda.2015.01.009
16. Siddharth A, Taylor JW. Forecasting electricity smart meter data using conditional kernel density estimation. Omega (2016) 59:47–59. doi:10.1016/j.omega.2014.08.008
17. Xu X, Yan Z, Xu S. Estimating wind speed probability distribution by diffusion-based kernel density method. Electric Power Syst Res (2015) 121:28–37. doi:10.1016/j.epsr.2014.11.029
18. Allen D, Ashraf M, McAleer M, Powell R, Singh A. Financial dependence analysis: Applications of vine copulas. Stat Neerlandica (2013) 67(4):403–35. doi:10.1111/stan.12015
19. Artyushenko VM, Volovach VI. Methods of estimating the form of the probability distribution density in tasks of processing measurement results. J Phys Conf Ser (2021) 2096(1):012136.
20. Artyushenko VM, Volovach VI, Lyapina KV, Kutukova AI. Mathematical methods for describing the non-Gaussian random variables and processes. In: 2022 Moscow Workshop on Electronic and Networking Technologies (MWENT). Moscow, Russian Federation: IEEE (2022). p. 1–6.
21. Pitt D, Giullen M, Bolance C (2011). Estimation of parametric and nonparametric models for univariate claim severity distributions - an approach using R. SSRN Electron J. doi:10.2139/ssrn.1856982
22. Alghalith M. Novel and simple non-parametric methods of estimating the joint and marginal densities. Physica A: Stat Mech its Appl (2016) 454:94–8. doi:10.1016/j.physa.2016.02.034
23. Alghalith M. A new parametric method of estimating the joint probability density. Physica A: Stat Mech its Appl (2018) 471:799–803. doi:10.1016/j.physa.2016.12.043
24. Maasoumi E, McAleer M. Realized volatility and long memory: An overview. Econometric Rev (2008) 27:1–9. doi:10.1080/07474930701853459
25. Asai M, McAleer M. A fractionally integrated Wishart stochastic volatility model. Econometric Rev (2013) 36. doi:10.1080/07474938.2015.1114235
26. Asai M, McAleer M, Medeiros M. Modelling and forecasting noisy realized volatility. Comput Stat Data Anal (2012) 56:217–30. doi:10.1016/j.csda.2011.06.024
27. Muhle-Karbe J, Pfa el O, Stelzer R. Option pricing in multivariate StochasticVolatility models of OU type. SIAM J Finan Math (2012) 3:66–94. doi:10.1137/100803687
28. Sahiner M. Forecasting volatility in asian financial markets: Evidence from recursive and rolling window methods. SN Bus Econ (2022) 2:157. doi:10.1007/s43546-022-00329-9
29. Mastroeni L. Pricing options with vanishing stochastic volatility. Risks (2022) 10:175. doi:10.3390/risks10090175
Keywords: volatility, volatility of volatility, probability density, econophyisics, finance
Citation: Alghalith M (2022) Methods in econophysics: Estimating the probability density and volatility. Front. Phys. 10:1050277. doi: 10.3389/fphy.2022.1050277
Received: 21 September 2022; Accepted: 10 October 2022;
Published: 21 October 2022.
Edited by:
Sergio Da Silva, Federal University of Santa Catarina, BrazilReviewed by:
Joao Plinio Juchem Neto, Federal University of Rio Grande do Sul, BrazilCopyright © 2022 Alghalith. This is an open-access article distributed under the terms of the Creative Commons Attribution License (CC BY). The use, distribution or reproduction in other forums is permitted, provided the original author(s) and the copyright owner(s) are credited and that the original publication in this journal is cited, in accordance with accepted academic practice. No use, distribution or reproduction is permitted which does not comply with these terms.
*Correspondence: Moawia Alghalith, bW9hd2lhLmFsZ2hhbGl0aEBzdGEudXdpLmVkdQ==
Disclaimer: All claims expressed in this article are solely those of the authors and do not necessarily represent those of their affiliated organizations, or those of the publisher, the editors and the reviewers. Any product that may be evaluated in this article or claim that may be made by its manufacturer is not guaranteed or endorsed by the publisher.
Research integrity at Frontiers
Learn more about the work of our research integrity team to safeguard the quality of each article we publish.