- Department of Management Science and Engineering, East China University of Science and Technology, Shanghai, China
Information diffusion in social media has attracted the wide attention of scholars from diverse disciplines. In real life, many offline events can cause online diffusion of relevant information, and the relation between the characteristics of information diffusion and offline events, as well as the diffusion differences corresponding to different phases of offline events have been studied. However, the effects of offline events on information diffusion are not well explored. In this paper, we study the influence of a popular and multi-phase talent show with elimination mechanism on relevant information diffusion. We find that elimination mechanism has significant influence on the features of information diffusion, and elimination results have a negative effect on followers’ emotional tendency. Elimination results also significantly affect the topics discussed by users. Besides elimination results have a negative effect on participants’ popularity, but do not affect the followers’ loyalty to program participants. This study not only reveals the effects of offline events on online information diffusion, but also provides approaches for studying the online diffusion of similar offline events.
1 Introduction
The emergence and development of online social networks and social media have not only changed the way people make friends, but also change the way of information acquisition and diffusion. Users are not only receivers of information, but also producers and disseminators of it. In recent years, information diffusion has attracted the attention of scholars from different fields, and significant progress has been made in empirical, modeling and prediction research [1–4].
In fact, the information posted by users on social media is often closely related to events occurring in the offline real world. Some events have far-reaching impact, and some last for a long time, thus attracting the attention of numerous users and triggering extensive discussions. In certain events, especially political ones, offline events and online discussions can influence each other, creating online-offline interactions. The correlation between offline events and corresponding online discussions provides a new scenario for information diffusion research.
Some researchers study the influence of TV series on related information diffusion. Since TV series usually update once every week, the related discussion is periodic impulsive. Fu et al proposed an impulsive susceptible-infected-removed (SIR)-like model to reproduce the periodic impulsive feature [5]. The influence of offline sports events on online user behavior has also been explored. Chung et al examined #BoycottNFL, an online connective action created to discontinue support of the National Football League, and found that associated offline trigger events affect the diversity of actors participating in connective action and fostering interactions between actors and online communities of diverse backgrounds [6].
The influence of offline vicious events on users’ online behavior has been extensively studied. For instance, Burnap et al studied the terrorist event in Woolwich, London in 2013 and built models to predict information flow size and survival using data from Twitter. They found that the number of offline press reports relating to the event published on the day the tweet is posted is a significant predictor of size [7]. Zhou mined the users’ behaviors in four emergency events from microblogs to reveal their behavior preferences, and found that users’ behaviors in emergencies are related to their own interests and economic status [8]. Some studies explored the communication dynamics in social networks/media during or after natural disasters. For example, Kim and Hastak explored patterns created by the aggregated interactions of users on Facebook during responses in the 2016 Louisiana flood [9], and Pourebrahim et al investigated the communication dynamics on Twitter during Hurricane Sandy in 2012 [10]. The studies can help emergency agencies develop better operation strategies for a disaster mitigation/relief plan.
The COVID-19 pandemic is the most influential public health event in recent years which has caused profound social and economic impacts. Shen et al analyzed posts related to COVID-19 on Weibo, a popular Twitter-like social media site in China, to predict COVID-19 case counts in mainland China [11]. During COVID-19, unreliable information or fake news spread on the Internet. Using Twitter messages, Gallotti et al assessed the risks of the spread of information of questionable quality during the early stages of COVID-19 epidemics [12]. Vaccines are an important means to contain the large-scale spread of COVID-19. Hu et al investigated public opinion and perception on COVID-19 vaccines in the United States with Twitter data and found the rising confidence and anticipation of the public towards vaccines [13]. The COVID-19 not only affects people’s physical health, but also their mental health. Through the analysis on Twitter, it was found that Australians’ mental health signals, quantified by sentiment scores, have a shift from pessimistic (early pandemic) to optimistic (middle pandemic). However, the signals progressively recess towards a more pessimistic outlook (later pandemic) [14]. Using social media data from Twitter and Weibo, Wang et al found that COVID-19 outbreaks cause steep declines in expressed sentiment globally [15]. They also found moderate to no effects of lockdown policies on expressed sentiment.
Political events have also attracted the attention of scholars because of their extensive and profound influence on people’s life. High-impact public protests [16–28] or election campaigns [29–32] and related online discussions often interact, such as the Arab Spring [23, 27], the Spanish indignados movement [17, 18, 21, 24], the Occupy Wall Street movement [21, 22, 24] and the 2016 United States Presidential Election [32]. During the Arab Spring movement, social media activity in Twitter correlates with subsequent large-scale decentralized coordination of protests [23]. For the Spanish indignados movement, social media are the main tools for informing and mobilizing [19], and there are four types of users (influentials, hidden influential, broadcasters, and common users) in Twitter and they play different roles in the growth of the protest [18]. During the Occupy Wall Street movement, Twitter users generated a loosely connected hub-and-spoke network, suggesting that information is likely to be organized by several central users in the network and that these users bridge small communities [22]. Only a very small minority of tweets refer to protest organization and coordination issues [21]. During the 2016 United States Presidential Election, individuals are more active in interacting with similar-minded Twitter users (“echo chambers” effect), and the aggressive use of Twitter bots, coupled with the fragmentation of social media and the role of sentiment, could enhance political polarization.
Recently the causal impact of offline or online events on information diffusion in social media has also been studied. Yu et al examined the effect of the online 16 Days Campaign on the changes in public discussions of the MeToo in Twitter by applying the state-space model, and found that there are significantly more discussions in MeToo after the launch of the campaign [33]. Leveraging difference-in-difference (DID) method, Balawi et al investigated the impact of the United Airlines crisis on three dimensions of customer relationship management efforts on social media, and found that the brand crisis increases informativeness efforts but reduces timeliness and attentiveness efforts [34]. Falavarjani et al studied the causal relation between real world activities and emotional expressions of users in social media based on a quasi-experimental design, and found that users’ offline activities impact their online affective expressions, both of emotions and moods [35].
The researches on the correlation between offline events and online discussions rely on event details and social media data, and vital conclusions have been obtained. However, the effects [36, 37] of offline events on online discussions as well as the underlying mechanisms still have not been well explored. Besides in real life, some offline events can last for a long time and show significant multiple stages over time. Few studies have explored the impact of such events on online discussions, of which results will deepen our understanding of how offline events affect social media. In this paper, take an influential and multistage entertainment program for example, we will study the effects of offline events on related information diffusion in social media by collecting publicly available data, and try to fill the research gaps.
2 Data set
The public pays much attention to entertainment programs in their leisure time. Compared with the social news and political events, the influence of entertainment programs is long-term and moderate [38]. The TV talent show “Produce 101” (first aired on 21 April 2018, and last aired on 23 June 2018) is one of the most influential entertainment programs in the Chinese mainland in recent years. The 101 contestants participated in the training and assessment of singing and dancing. According to the results of multiple rounds of voting and elimination, the final winners were determined.
Specifically, in the first elimination round, 101 contestants competed based on the audience’s votes in the official voting channel, with 55 contestants advancing and the rest eliminated. In the second round, 36 were promoted and 19 eliminated. In the third round, 22 were promoted and 14 eliminated. In the fourth round, i.e. the final round, the top 11 votes won.
The talent show was presented in the form of live TV. In addition to watching the program, the audience also published relevant posts on social media, which makes it a typical event combining online and offline. We collected publicly available posts with the topic of “Produce 101” from Sina Weibo, Chinese largest online microblogging platform, including the original posts and reposts. The time span of the data set is from 1 April 2018 to 30 June 2018 and it includes the information of microblog release time, content, poster’s encrypted ID number, gender, and city. After data cleaning, there are 33,522,289 original posts and 56,267,334 reposts in the data set.
We choose this program for research for two reasons. First, this program has a large popularity, a wide range of audience, and is representative. Second, the program has a relatively long time span, with four rounds of elimination, making it possible to study the information diffusion in different phases of the program.
3 Program progress
As shown in Figure 1, we divide the data set according to the four rounds of elimination. The phase before the first elimination can be that for media promotion. In the early stage of the program preparation and broadcast, the publicity of the official account is crucial to ensure the authority and credibility of the program information. In addition, some audiences learned about the program from various channels in the early stage and spontaneously became the propagandists of the program. Official account and spontaneous users published and spread program information online, built initial nodes of information diffusion network, and introduced and connected more users by virtue of their own social networks and open platforms.
The phase between the first and second elimination can be the guidance stage of opinion leaders. Opinion leaders played an important role in the diffusion process. By releasing relevant highlights in the program and by virtue of their social capital and position advantages in social networks, they could reach more users at a faster speed and in a wider range, and finally expand the audience.
The phase between the second and third elimination can be the stage of contestants’ self-growth. With the broadcast of the program, the audience increased, ordinary users gradually became fans, contestants accumulated their own fan groups, and the increase of audience also made the information diffusion scale expand again. At this phase, the increase of users could not be all from the influence of the program, but more from the attractiveness of the contestants.
The phase between the third and fourth elimination can be the stabilization stage for the contestants’ fans. In the previous stages, the fans kept increasing, and in this stage, the fan groups could tend to be stable. Some users gradually lost interest in the program and no longer paid attention to it, while the remaining ones could be more loyal.
The phase after the program can be the recession one. The popularity of talent shows decreased with its end. For the audience, the novelty of and the enthusiasm for the program is limited. Without the real-time discussion, the related posts in social media could gradually decrease.
4 Characterizing information diffusion
Elimination mechanism is commonly used in competitive programs, which means that the discussion of users on Weibo may change with elimination results, and the indicators of posts related to contestants with different results after eliminations can also change. In preliminary research we have found that for the two groups of promoted and eliminated contestants, there exists interaction between information on them, and different interactions can occur at different stages. From the three perspectives of posts, users and contestants, we study the influence of elimination mechanism on the indicators involved in each perspective.
4.1 Posts
According to the repost relation in microblogs, each original post and its reposts can form an information diffusion tree, which represents the diffusion path of the original post and can be viewed as directed networks where the nodes without in-degree are root nodes or seed nodes, the nodes without out-degree are leaf nodes or passive nodes, and the nodes with both in-degree and out-degree are viral nodes.
In the paper, at the post level, we utilize four basic indicators to measure the diffusion capacity of original microblogs from different perspectives [39]. Let the numbers of seed nodes, viral nodes and passive nodes be Ns, Nv, and Np respectively, and the total number of nodes is N = Ns + Nv + Np which represents the diffusion scale and reflects the overall influence. The branches of diffusion trees refer to the forwarding chains, with one branch starting from the root node and ending with a leaf node. According to this definition, the number of branches Nb is equal to leaf node number Np. Let the length of each branch be di (i = 1, 2, … , Nb), and the maximum length D =
4.2 Users
At the user level, microblogs posted by users usually have specific emotions and belong to specific topics. Thus, we use two indicators, emotional tendency and topic category, to describe the content of microblogs.
The emotional tendency of microblogs refers to the degree of positive or negative emotion expressed in posts, which is usually measured by a single value. The positive values indicate positive emotion, while negative values negative emotion, and the absolute values indicate the degree of tendency. In this study, we utilize an authoritative simplified Chinese affective lexicon ontology to obtain emotional tendency of microblogs [40, 41]. The lexicon divides emotions into seven categories (happy, good, surprise, anger, sad, fear, and disgust) and 21 subcategories, which are labeled with polarity, part of speech, and emotional intensity for each emotional vocabulary. The emotional intensity label has levels 1, 3, 5, 7, and 9. Level 1 has the lowest intensity, and level 9 indicates the highest intensity. To better assess the emotions of microblogs, we comprehensively consider the combination and order of emotional words, adverbs of degree and negative words. The detailed calculation methods are shown in the Supplementary Material. The accuracy of emotion analysis for the lexicon ontology is 0.79, and its effectiveness has been demonstrated in many studies [42, 43] and it has been extensively applied in the emotion analysis of short texts [44, 45].
We obtain the emotional tendency of microblogs and find that the mean tendency value of all original posts is 1.85, the mean value of original posts with positive emotion is 3.27, and the mean value of original posts with negative emotion is -1.79. Overall the sentiment of microblogs in the data set is positive.
We use Biterm Topic Model (BTM) [46], a topic classification model suitable for short texts, to evaluate the topic of each original microblog. BTM integrates word co-occurrence information into latent Dirichlet allocation (LDA) to solve the problem of inferring topics from large-scale short texts. For the classification performance of Chinese short texts, the accuracy of BTM is close to 0.7. BTM has many advantages over some previous topic classification methods, and recently has been widely used in (Chinese) short text topic classification [47, 48].
We first set the number of topics to 50, and except the topics that cannot be specifically identified, we manually classify the others into six ones by topic merging according to the characteristics of entertainment programs. The posts with endorsement topic are on followers’ behaviors. Contestants’ fans or other users could increase the popularity of their supporters through microblogs, and increase ranking by soliciting votes, inviting clicks and other behaviors. The posts with stage performance topic are on the stage performance of contestants in the program. The posts with praise and encouragement topic are to praise the participants to achieve the purpose of publicity. The posts with contestant activities topic are on the activities of the participants outside the program. The posts with program publicity topic are on the publicity released by program producer to improve the popularity of the program. The posts with program criticism topic are on users’ negative evaluation of the program, including doubts about or objections to program editing, competition fairness, elimination results, etc.
4.3 Contestants
We defined two indicators to characterize contestants, i.e., the mean number of mentions < cntd,s> of contestant s on some day d and the losing rate outRatiod+1,s of contestant s’s active fans on the next day d+1.
Specifically, if a user posts original microblogs that only mention a certain contestant on some day, the user is considered to be the active follower of that contestant on that day. For each contestant we obtain the number of daily active followers daud,s and the number of next day active followers daud+1,s. According to the posts published by each user every day, we obtain the number of times cntd,s,u that user u mentions contestant s on day d (similarly, only the microblogs that mention only one contestant will be considered). Then, the mean number of daily mentions < cntd,s> of each contestant can be obtained from the ratio of the total number of daily mentions of each contestant to the number of daily active followers of each contestant, i.e.,
We define the retention number rd+1,s of followers of contestant s on the next day d+1 as the number of users who post microblogs that only mention that contestant on day d and d+1, namely the intersection of the active followers of day d and d+1. For each contestant, we define the number of followers who lose activity on day d+1 as the number of users who post microblogs that only mention that contestant on day d but not on day d+1, i.e. the difference
5 Effect estimation
5.1 Methods
In competitive programs, different participants will face different results after elimination. Some participants will be eliminated and lose the qualification to continue to participate in the following program, while others will be promoted and continue to participate in the program. Since the offline process is closely related to the online discussion, we will discuss the influence of elimination mechanism on the information diffusion related to the two types of contestants.
There are two dimensions for the elimination competitions, one is before and after eliminations, and the other is the promoted and eliminated contestants. If elimination matches are considered as an intervention, the significance of treatment effect after intervention can determine the existence of causality. We use the DID method to estimate the treatment effect of elimination matches.
According to the counterfactual reasoning, a group of samples similar to the treatment group is selected as the control group to obtain the results without treatment, and the difference between them is the treatment effect of the event. In the paper, the observation value of the treatment group is the diffusion performance of the relevant microblogs of the eliminated contestants in an elimination match, while the observation value of the control group is that of the promoted contestants in the same elimination match.
In the DID method, for the treatment and control groups, the first difference is the difference between them before elimination and the difference between them after elimination. The mean difference between the treatment group and the control group before elimination is
The DID method estimates the treatment effect by constructing a regression model with interaction terms, and the basic model is shown in Eq. 1:
Let
5.2 Data partitioning
As shown in Figure 2, there are four elimination matches for the talent show, and the dates are May 13, June 2, June 16, and June 23. The four elimination matches divide the entire data set into five parts, and the treatment effect of each elimination match is discussed separately. The DID method is usually used in policy research, and the time span of the data studied is often very long. Considering some periodic fluctuations, data are usually studied in periods of a year or a month. There are often multiple periods of data before the implementation of policies. However, the time span of the entertainment program studied is less than 2 months, and the duration of each phase is shorter, even only a week. Thus, in the paper, only the two phases before and after the elimination match are considered in each analysis. For example, for the first elimination match, only the microblogs in p1 and p2 phases are considered, where p1 is the phase before the elimination match, i.e.,
To ensure the reasonability of the DID regression model, we put forward two assumptions. The first is stable unit treatment value assumption (SUTVA). It is difficult to rigorously test this hypothesis, and we adopt the interpretation method used by Weiler et al. to give reasons that SUTVA can be true in our study [49]. SUTVA consists of three aspects. 1) Individual independence assumption. According to the rules of the program, all contestants participate as individuals, not as multi-person teams, thus the results of the program will only affect individuals and their related posts, i.e., the elimination result of a contestant only affects the contestant’s related posts, and there is no interaction between contestants. 2) The assumption of single treatment. There are only two results of elimination and promotion. The eliminated contestants, regardless of their specific ranking in the eliminated group, will leave the stage. The promoted contestants, regardless of their specific ranking in the promoted group, will compete on the stage until the next elimination. In other words, the treatment effect of elimination matches on eliminated contestants is the same, and the difference of contestants’ rankings has no additional effect on the characteristics of online information diffusion associated with them. 3) The assumption of no interference in posts. The program studied has a wide range of audience. Users only discussed the status of the contestants they followed in their posts, and there may be no correlation between posts published by different users, i.e., different microblogs do not affect each other.
The second is parallel trend assumption, i.e., other factors have the same influence on the information diffusion characteristics of microblogs associated with eliminated and promoted contestants. That is, in the absence of elimination, the trend of the mean characteristics of the treatment group and the control group is parallel over time. As explained above, in the paper, we set one period before and after the elimination match in each analysis, and the DID method for one period of data may not be able to carry out the parallel trend test. However, this assumption can be satisfied with propensity score matching (PSM) which will be discussed in section 6.1.
6 Results
Previous studies have revealed the correlation between the performance of information diffusion and information topics [50], emotions expressed in texts [51], and user attributes [52]. In fact, in some cases, user attributes are not related to diffusion performance [27]. This study focuses on online interactions in an entertainment program context, and traditional cues such as user gender, or location can become irrelevant to information diffusion on contestants.
Recently textual data have been applied to causal inference studies, such as the influence of collective sentiment expressed in social media on stock market or cryptocurrency prices [53] or the causal effects of brevity of tweets on their success by controlled experiments [54]. Text characteristics can also be dependent variables. For example, Egami et al. studied how awareness about an individual’s criminal history affects attitudes toward immigration using a survey experiment [55]. Besides texts can be confounders in causal analyses and Roberts et al. used text analysis to control for this type of confounding [56]. In this section we use microblog features as dependent variables or confounders to study the effect from elimination mechanism on information diffusion.
It is noteworthy that the entertainment program studied was presented in the form of live TV. Some users can watch the program on TV and then published relevant posts on social media. There are also some users who were informed of the program’s progress through other channels rather than TV, and it is hard to obtain channel information from post texts. In this paper, offline means the program was presented on TV, we focus on the influence of elimination mechanism on the information diffusion, and may not focus on the channels through which users got information about the program. Besides since the user ID numbers in the data set have been encrypted, some of their important attributes, including centrality indices characterizing user influence, are unavailable, and we have applied the PSM method to try to address the endogeneity issue caused by user influence and inertia.
6.1 Posts
We estimate the treatment effect of elimination on the structural features of information diffusion trees in different phases by taking each elimination match as a time point. The original microblog content corresponding to each diffusion tree is analyzed, the microblogs related to eliminated contestants in each time period are taken as the treatment group, and the ones related to promoted contestants are taken as the control group. Eq. 1 can be further written as
where Yit is the characteristics of information diffusion trees, such as diffusion scale (ln (size)), depth, width (ln (width)) and active time, and Days is the fixed effect refined to every day. We use also Chinese affective lexicon ontology to obtain the fine-grained emotions of each microblog which include happy, good, surprise, anger, sad, fear, and disgust, and the detailed calculation methods are also presented in the Supplementary Material. Control variable Xit contains content features of microblogs, such as emotional tendency, fine-grained emotions and topics.
Selection bias and the endogeneity problem among microblogs on the eliminated contestants may exist. The microblogs on eliminated and promoted contestants can differ substantially, meaning that they may not be directly comparable. Besides there are three confounding variables, i.e. post emotional tendency, fine-grained emotions and discussion topics. To study the influence of the results of elimination matches on the characteristics of information diffusion, we use PSM to match the control variables which is performed before the DID regression.
PSM converts multidimensional confounders according to the corresponding function (for example, logistic regression) to a one-dimensional propensity score pscore, which means the probability of the sample being treated, i.e.
PSM can guarantee the homogeneity between control group and treatment group and the establishment of the assumption of long-term trend consistency to a certain extent. Then the matched samples are used for DID to ensure the applicability of DID method.
Table 1 shows the results of the DID regression model (please see Supplementary Material for summary statistics and distributions of variables, correlation matrices and VIF tests for independent variables, PSM results, and complete regression results). The difference column before elimination is the estimation for β1, the difference column after elimination is the estimation for β1+β3, and finally the difference column corresponding to DID is the estimation for β3. Figure 3 shows the values of β3 with significance level indicated. We find that the first and fourth elimination matches have a significant effect on diffusion depth, the first, third and fourth elimination ones have a significant effect on active time, and the first, second and fourth elimination ones have a significant effect on diffusion width and scale.
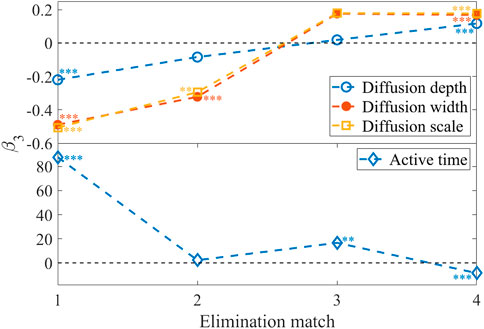
FIGURE 3. The β3 values with significance level for DID regressions for diffusion tree characteristics.
The first and last elimination matches have a significant effect on the metrics of information diffusion trees. In the first elimination match, the audience does not have a deep understanding of the contestants, the eliminated contestants do not have enough time to show themselves and gain high popularity, and they leave the stage in a hurry. The microblogs related to the eliminated contestants may also gradually become silent, and the attention of ordinary audiences will be shifted to the promoted contestants. Elimination results have a negative effect on diffusion depth, width and scale of posts on eliminated contestants (β3 = -0.220 for depth, β3 = -0.489 for width, and β3 = -0.506 for scale). Even in the second elimination match, elimination results still have a negative effect on diffusion width and scale. On the contrary, the elimination results have a positive effect on the active time of posts on eliminated contestants (β3 = 87.729). One possible reason is that the promoted contestants are still active on the stage, and the original microblogs related to them emerge every day. Users tend to forward the latest related microblogs, and the forwarding frequency of past posts can reduce, thus the active time of the original microblogs is short. While the eliminated contestants lose the opportunity to perform on stage, the number of new posts related to the contestants can decrease, and some users choose to forward the past posts related to them, which may increase the mean active time of the posts.
At different stages of the program, the elimination matches result in different treatment effects, which may be related to the external factors associated with contestants in offline events. In the final elimination, both the eliminated and the final winners have accumulated a large number of followers over the course of the program. The treatment effect of elimination on diffusion depth, width and scale of the microblogs on eliminated contestants is positive (β3 = 0.117 for depth, β3 = 0.168 for width, and β3 = 0.177 for scale). The possible reason is that the eliminated contestants leave the stage earlier, have access to industry resources faster than the winning contestants, and are known to users outside the program. After the final round, users can forward more new posts on eliminated contestants than the old ones, the active time of posts decreases, and finally the final match has a negative effect on it (β3 = -8.493).
6.2 Users
Elimination matches not only have a significant impact on the diffusion tree characteristics of the original microblogs, but have a certain impact on the emotional tendency of users to contestants and the topics they talk about on contestants. We study the effect of elimination matches at the user level. Since information diffusion features cannot affect content features, it is unnecessary to match them when constructing the regression models. First, we explore the influence of elimination matches on users’ emotional tendency, and Eq. 3 gives the DID regression model:
where Topicit is the topic classification of microblogs which is the control variable and is represented by a dummy variable. In this context PSM is used to match topic categories.
Table 2 shows the impact of elimination matches on users’ emotional tendency (please see Supplementary Material for more information on variables, PSM results and complete regression results), and Figure 4 shows the values of β3 with significance level indicated. In the first two matches, elimination results have no significant effect on users’ emotional tendency, but in the latter two, elimination results have significant negative effect on emotional tendency. The possible reason is that in the early stage, users do not have a deep understanding of the contestants and take a wait-and-see attitude. They might just be ordinary audience of the program, rather than fans of the contestants. Therefore, there could be less of a gap in expectations and less emotional volatility when the elimination results were announced. As can be seen from the p-value, there is almost no difference between the treatment group and the control group in the first elimination match, no matter before or after the competition, and the treatment effect of the elimination match is almost zero. In the second elimination match, some users began to support their favorite contestants, and the emotional tendency to eliminated contestants increases (p < 0.05), but the difference before and after the match is not significant.
The topics discussed by users in social media are also closely related to the program, and the elimination results may also affect the topics discussed by users. Eq. 4 shows the DID logistic regression model for the effect:
where Yit is the topic category which is a binary classification variable and is represented by a dummy variable. As control variables, Sentimentit includes emotional tendency and fine-grained sentiment. PSM is also used to match the control variables.
Table 3 gives the regression results, with the values in each column representing estimates of the differences for each topic (please see Supplementary Material for more information on variables, PSM results and complete regression results), and Figure 5 shows the values of β3 with significance level indicated. We find that the early elimination results have a significant impact on the topics of endorsement and stage performance. The elimination results of the middle stage have significant influence on the topics of endorsement, stage performance, praise and encouragement and contestant activities, and the late stage has significant influence on all topics.
From the perspective of post topics, the discussion on the endorsement topic decreases significantly after several eliminations. The results of the elimination matches are closely related to the audience’s vote, and Weibo is one of the important platforms to support and attract users to vote. After the elimination matches, the result has been decided, and thus the discussion on eliminated contestants on the topic of calling for vote and support decreases. However, the discussion on the topic of stage performance increases significantly after the elimination matches. The stage performance in the elimination competition attracts much attention. After the elimination, users could discuss the performance of each contestant in combination with the result of the competition. By contrast, users may pay more attention to the performance of the eliminated contestants to analyze the reasons for elimination. The program criticism and propaganda topics are related to the program itself, and there is no significant relationship with most elimination results. In the last elimination match, the posts published by users on program criticism and propaganda topics for eliminated contestants decrease significantly.
About the eliminated contestants, posts on praise and encouragement topic in the last three elimination matches drop significantly. The possible reasons are, on the one hand, because some contestants are eliminated, users can have negative emotions and the posts on praise and encouragement topic reduce. On the other hand, after each elimination, the competition between the contestants weakens, reducing the discussion on praise and encouragement topic to some extent. Users’ discussion on the topic of contestant activities increases significantly in the last three elimination rounds. After each elimination, the eliminated contestants quit the program and could carry out personal activities without following the relevant regulations of the program. As a result, the discussion on contestants’ activities increases.
6.3 Contestants
The impact of elimination matches on information diffusion in social media is not only reflected at the levels of posts and users, but also at the level of contestants. Elimination matches may change the behavior pattern of contestants’ followers when they posted microblogs related to the contestants. Based on the original microblogs, we obtain the losing rate outRatiod+1,s of each contestant’s active followers on day d+1 and the mean number of daily mentions < cntd,s> of each contestant, and Eq. 5 gives the DID regression model:
where Yit is outRatiod+1,s or < cntd,s>. At the contestant level, the behavioral characteristics of users are concerned with individual contestant rather than individual post, thus the information diffusion characteristics and content characteristics cannot be quantified, and the PSM is not used.
Table 4 shows the regression results (please see Supplementary Material for more information on variables and complete regression results), and Figure 6 shows the values of β3 with significance level indicated. We find that for all four elimination matches, the results of elimination have a significant positive impact on the eliminated contestants’ outRatiod+1,s, i.e., weakening their popularity, but have no significant impact on their < cntd,s>, that is, they do not affect the loyalty of contestants’ followers.
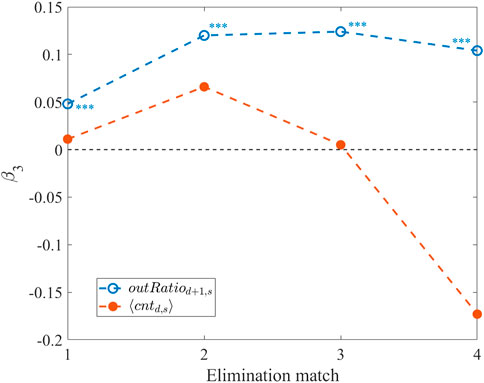
FIGURE 6. The β3 values with significance level for DID regressions for user behavioral characteristics.
Elimination matches increase the outRatiod+1,s of the eliminated contestants. According to regression results, the influence of the first elimination (β3 = 0.048) is lower than those of the subsequent eliminations, and the contestants’ follower group may form in the second elimination. In the first elimination, contestants could have less followers and the change of the losing rate is small. In the fourth elimination competition, although < cntd,s> of eliminated contestants is significantly lower than that of promoted contestants after elimination, this trend has appeared before the competition, and finally, the elimination match has no significant effect on < cntd,s> of eliminated contestants.
7 Heterogeneity analysis and robustness test
Users of different characteristics may have different reactions to the program studied. Given that demographic information on users is often unavailable on Weibo platform due to specific profile settings or not filling in information, we perform the heterogeneity analysis to examine the variation of effects for users of different genders (the missing rate of gender attribute is low) and obtain the DID regression results (due to space limitation, we present the results in Supplementary Material).
We find that for the effect of elimination results on diffusion tree characteristics, overall the DID regression results for female users are qualitatively consistent with those in Table 1. For male users, elimination results never have a positive effect on diffusion depth, width and scale, which means that elimination results have a negative effect or no significant effect on the metrics of posts by male users on eliminated contestants.
For the effect of elimination results on sentiment tendency, we find that the DID regression results are qualitatively consistent with those in Table 2 both for female and male users. In the last two elimination matches, elimination results have a negative effect on followers’ emotional tendency. For the effect of elimination results on post topics, we find that overall the DID regression results for female users are also qualitatively consistent with those in Table 3. For male users, most regression results are insignificant, which means that elimination results have no significant effect on the topics of posts by male users on eliminated contestants. However, the signs of significant results are consistent with those in Table 3.
We also perform robustness test by supplementing variables. Specifically, we added two control variables on user characteristics to the regression equations, i.e., users’ gender and region (province level) where they are located. The DID regression results are also presented in the Supplementary Material, and we find that the conclusions in the paper still hold which indicates the robustness of the conclusions.
8 Conclusion
In this paper, we study the effect of an influential and multi-phase entertainment program on related information diffusion and explore the underlying mechanisms. We find that elimination mechanism significantly influences the features of information diffusion trees, and elimination results negatively affect followers’ emotional tendency. Elimination results also negatively affect the topics on endorsement and praise and encouragement discussed by users, and positively affect the topics on stage performance and contestant activities. Besides elimination results negatively affect participants’ popularity, but do not affect the followers’ loyalty to participants. The methods of this study are generalizable to some extent. Except entertainment events with multiple rounds of elimination, the approach in this paper could apply to research on information diffusion of offline events in different domains, for instance, sports events with multiple rounds of elimination or multi-phase political events.
There are several limitations for the paper. We divide the contestants into the promoted and eliminated groups to estimate the treatment effect of elimination match. In fact, even for contestants in the same group, elimination matches may have different effects. Besides, the conclusions of this paper may lack universality. Considering the different participants, audiences, and contexts of events, different conclusions may emerge, and we need to study more events or scenarios in detail and comparatively. Further there can be mutual influence between online discussion and offline events, which can cause the problem of reverse causality. Reverse causality can cause endogeneity problems which are mainly caused by the four reasons: omitted variables, sample selection bias/self-selection bias, reverse causality, and measurement error, and the problems can be dealt with in a number of ways, such as instrumental variable (IV), Heckman model, fixed effects model, DID, regression discontinuity, and PSM. In the paper we utilize PSM to address the issue. However, PSM only controls the influence of measurable confounders, does not fundamentally solve the endogeneity problem caused by selection bias or omitted variables, and also can not solve the problem of reverse causality. Generally, IV method can address the four endogeneity problems, and for the reverse causality IV method is an option. All of these give potential directions for future further research.
Data availability statement
The original contributions presented in the study are included in the article/supplementary material, further inquiries can be directed to the corresponding author.
Author contributions
Conceptualization, HH, YL, and NX. Methodology, QL, NX, HH, and YL. Software, QL and NX. Visualization, NX. Formal analysis, NX, QL, and HH. Writing, NX, QL, and HH.
Funding
The study was partially supported by the National Natural Science Foundation of China (Grant Nos. 61973121 and 71971082).
Conflict of interest
The authors declare that the research was conducted in the absence of any commercial or financial relationships that could be construed as a potential conflict of interest.
Publisher’s note
All claims expressed in this article are solely those of the authors and do not necessarily represent those of their affiliated organizations, or those of the publisher, the editors and the reviewers. Any product that may be evaluated in this article, or claim that may be made by its manufacturer, is not guaranteed or endorsed by the publisher.
Supplementary material
The Supplementary Material for this article can be found online at: https://www.frontiersin.org/articles/10.3389/fphy.2022.1032913/full#supplementary-material
References
1. Zhang ZK, Liu C, Zhan XX, Lu X, Zhang CX, Zhang YC. Dynamics of information diffusion and its applications on complex networks. Phys Rep (2016) 651:1–34. doi:10.1016/j.physrep.2016.07.002
2. Wang X, Lan Y, Xiao J. Anomalous structure and dynamics in news diffusion among heterogeneous individuals. Nat Hum Behav (2019) 3:709–18. doi:10.1038/s41562-019-0605-7
3. Zhou B, Pei S, Muchnik L, Meng X, Xu X, Sela A, et al. Realistic modelling of information spread using peer-to-peer diffusion patterns. Nat Hum Behav (2020) 4:1198–207. doi:10.1038/s41562-020-00945-1
4. Xie J, Meng F, Sun J, Ma X, Yan G, Hu Y. Detecting and modelling real percolation and phase transitions of information on social media. Nat Hum Behav (2021) 5:1161–8. doi:10.1038/s41562-021-01090-z
5. Fu P, Zhu A, Fang Q, Wang X. Modeling periodic impulsive effects on online TV series diffusion. PLoS ONE (2016) 11(9):e0163432. doi:10.1371/journal.pone.0163432
6. Chung TL, Johnson O, Hall-Phillips A, Kim K. The effects of offline events on online connective actions: An examination of #BoycottNFL using social network analysis. Comput Hum Behav (2021) 115:106623. doi:10.1016/j.chb.2020.106623
7. Burnap P, Williams ML, Sloan L, Rana O, Housley W, Edwards A, et al. Tweeting the terror: Modelling the social media reaction to the Woolwich terrorist attack. Soc Netw Anal Min (2014) 4:206. doi:10.1007/s13278-014-0206-4
8. Zhou Q. Detecting the public’s information behaviour preferences in multiple emergency events. J Inf Sci (2022) 016555152110277. doi:10.1177/01655515211027789
9. Kim J, Hastak M. Social network analysis: Characteristics of online social networks after a disaster. Int J Inf Manage (2018) 38:86–96. doi:10.1016/j.ijinfomgt.2017.08.003
10. Pourebrahim N, Sultana S, Edwards J, Gochanour A, Mohanty S. Understanding communication dynamics on twitter during natural disasters: A case study of Hurricane Sandy. Int J Disaster Risk Reduction (2019) 37:101176. doi:10.1016/j.ijdrr.2019.101176
11. Shen C, Chen A, Luo C, Zhang J, Feng B, Liao W. Using reports of symptoms and diagnoses on social media to predict COVID-19 case counts in mainland China: Observational infoveillance study. J Med Internet Res (2020) 22(5):e19421. doi:10.2196/19421
12. Gallotti R, Valle F, Castaldo N, Sacco P, De Domenico M. Assessing the risks of ‘infodemics’ in response to COVID-19 epidemics. Nat Hum Behav (2020) 4:1285–93. doi:10.1038/s41562-020-00994-6
13. Hu T, Wang S, Luo W, Zhang M, Huang X, Yan Y, et al. Revealing public opinion towards COVID-19 vaccines with Twitter data in the United States: Spatiotemporal perspective. J Med Internet Res (2021) 23(9):e30854. doi:10.2196/30854
14. Wang S, Huang X, Hu T, Zhang M, Li Z, Ning H, et al. The times, they are a-changin’: Tracking shifts in mental health signals from early phase to later phase of the COVID-19 pandemic in Australia. BMJ Glob Health (2022) 7:e007081. doi:10.1136/bmjgh-2021-007081
15. Wang J, Fan Y, Palacios J, Chai Y, Guetta-Jeanrenaud N, Obradovich N, et al. Global evidence of expressed sentiment alterations during the COVID-19 pandemic. Nat Hum Behav (2022) 6:349–58. doi:10.1038/s41562-022-01312-y
16. Van Laer J. Activists online and offline: The Internet as an information channel for protest demonstrations. Mobilization: Int Q (2010) 15(3):347–66. doi:10.17813/maiq.15.3.8028585100245801
17. González-Bailón S, Borge-Holthoefer J, Rivero A, Moreno Y. The dynamics of protest recruitment through an online network. Sci Rep (2011) 1:197. doi:10.1038/srep00197
18. González-Bailón S, Borge-Holthoefer J, Moreno Y. Broadcasters and hidden influentials in online protest diffusion. Am Behav Scientist (2013) 57(7):943–65. doi:10.1177/0002764213479371
19. Fernandez-Planells A, Figueras-Maz M, Pàmpols CF. Communication among young people in the #spanishrevolution: Uses of online-offline tools to obtain information about the #acampadabcn. New Media Soc (2014) 16:1287–308. doi:10.1177/1461444814530097
20. Varol O, Ferrara E, Ogan CL, Menczer F, Flammini A. Evolution of online user behavior during a social upheaval. In: Proceedings of the 2014 ACM conference on web science. New York: ACM Press (2014). p. 81–90.
21. Theocharis Y, Lowe W, van Deth JW, García-Albacete G. Using twitter to mobilize protest action: Online mobilization patterns and action repertoires in the Occupy Wall Street, Indignados, and Aganaktismenoi movements. Inf Commun Soc (2015) 18:202–20. doi:10.1080/1369118X.2014.948035
22. Park SJ, Lim YS, Park HW. Comparing twitter and YouTube networks in information diffusion: The case of the “Occupy Wall Street” movement. Technol Forecast Soc Change (2015) 95:208–17. doi:10.1016/j.techfore.2015.02.003
23. Steinert-Threlkeld ZC, Mocanu D, Vespignani A, Fowler J. Online social networks and offline protest. EPJ Data Sci (2015) 4:19. doi:10.1140/epjds/s13688-015-0056-y
24. González-Bailón S, Wang N. Networked discontent: The anatomy of protest campaigns in social media. Social Networks (2016) 44:95–104. doi:10.1016/j.socnet.2015.07.003
25. Vasi IB, Suh CS. Online activities, spatial proximity, and the diffusion of the Occupy Wall Street movement in the United States. Mobilization: Int Q (2016) 21(2):139–54. doi:10.17813/1086-671x-22-2-139
26. Ahmed S, Jaidka K, Cho J. Tweeting India’s nirbhaya protest: A study of emotional dynamics in an online social movement. Soc Move Stud (2017) 16:447–65. doi:10.1080/14742837.2016.1192457
27. Venkatesan S, Valecha R, Yaraghi N, Oh O, Rao HR. Influence in social media: An investigation of tweets spanning the 2011 Egyptian revolution. MIS Q (2021) 45:1679–714. doi:10.25300/misq/2021/15297
28. Morales PR, Cointet JP, Froio C. Posters and protesters. J Comput Soc Sci (2022). doi:10.1007/s42001-022-00163-x
29. Aragón P, Kappler KE, Kaltenbrunner A, Laniado D, Volkovich Y. Communication dynamics in twitter during political campaigns: The case of the 2011 Spanish national election. Policy Internet (2013) 5:183–206. doi:10.1002/1944-2866.poi327
30. Xu WW, Sang Y, Blasiola S, Park HW. Predicting opinion leaders in Twitter activism networks: The case of the Wisconsin recall election. Am Behav Scientist (2014) 58(10):1278–93. doi:10.1177/0002764214527091
31. Segesten AD, Bossetta M. A typology of political participation online: How citizens used Twitter to mobilize during the 2015 British general elections. Inf Commun Soc (2017) 20:1625–43. doi:10.1080/1369118X.2016.1252413
32. Gorodnichenko Y, Pham T, Talavera O. Social media, sentiment and public opinions: Evidence from #Brexit and #USElection. Eur Econ Rev (2021) 136:103772. doi:10.1016/j.euroecorev.2021.103772
33. Yu X, Mashhadi A, Boy J, Nielsen RC, Hong L. Causal impact model to evaluate the diffusion effect of social media campaigns. In: Proceedings of the 20th European conference on computer-supported cooperative work (2022).
34. Balawi RA, Hu Y, Qiu L. Brand crisis and customer relationship management on social media: Evidence from a natural experiment from the airline industry. Inf Syst Res (2022). doi:10.1287/isre.2022.1159
35. Falavarjani SAM, Jovanovic J, Fani H, Ghorbani AA, Noorian Z, Bagheri E. On the causal relation between real world activities and emotional expressions of social media users. J Assoc Inf Sci Technol (2021) 72:723–43. doi:10.1002/asi.24440
36. Pearl J. Causal inference in statistics: An overview. Stat Surv (2009) 3:96–146. doi:10.1214/09-ss057
37. Yao L, Chu Z, Li S, Li Y, Gao J, Zhang A. A survey on causal inference. ACM Trans Knowl Discov Data (2021) 15(5):1–46. Article 74. doi:10.1145/3444944
38. Si M, Cui L, Guo W, Li Q, Liu L, Lu X, et al. A comparative analysis for spatio-temporal spreading patterns of emergency news. Sci Rep (2020) 10:19472. doi:10.1038/s41598-020-76162-7
39. Li H, Xia C, Wang T, Wen S, Chen C, Xiang Y. Capturing dynamics of information diffusion in SNS: A survey of methodology and techniques. ACM Comput Surv (2023) 55(1):1–51. Article No.: 22. doi:10.1145/3485273
40. Xu L, Lin H, Pan Y, Ren H, Chen J. Constructing the affective lexicon ontology. J China Soc Scientific Tech Inf (2008) 27(2):180–5.
41. Chen J, Lin H. Constructing the affective commonsense knowledgebase. J China Soc Scientific Tech Inf (2009) 28(4):492–8.
42. Ren G, Hong T. Investigating online destination images using a topic-based sentiment analysis approach. Sustainability (2017) 9:1765. doi:10.3390/su9101765
43. Liu SM, Chen JH. A multi-label classification based approach for sentiment classification. Expert Syst Appl (2015) 42:1083–93. doi:10.1016/j.eswa.2014.08.036
44. Ye Y, Long T, Liu C, Xu D. The effect of emotion on prosocial tendency: The moderating effect of epidemic severity under the outbreak of COVID-19. Front Psychol (2020) 11:588701. doi:10.3389/fpsyg.2020.588701
45. Qiu J, Xu L, Wang J, Gu W. Mutual influences between message volume and emotion intensity on emerging infectious diseases: An investigation with microblog data. Inf Manage (2020) 57:103217. doi:10.1016/j.im.2019.103217
46. Cheng X, Yan X, Lan Y, Guo J. Btm: Topic modeling over short texts. IEEE Trans Knowl Data Eng (2014) 26:2928–41. doi:10.1109/tkde.2014.2313872
47. Shi L, Song G, Cheng G, Liu X. A user-based aggregation topic model for understanding user’s preference and intention in social network. Neurocomputing (2020) 413:1–13. doi:10.1016/j.neucom.2020.06.099
48. Li X, Wang Y, Zhang A, Li C, Chi J, Ouyang J. Filtering out the noise in short text topic modeling. Inf Sci (2018) 456:83–96. doi:10.1016/j.ins.2018.04.071
49. Weiler M, Stolz S, Lanz A, Schlereth C, Hinz O. Social capital accumulation through social media networks: Evidence from a randomized field experiment and individual-level panel data. MIS Q (2022) 46:771–812. doi:10.25300/misq/2022/16451
50. Romero DM, Meeder B, Kleinberg J. Differences in the mechanics of information diffusion across topics: Idioms, political hashtags, and complex contagion on Twitter. In: Proceedings of the 20th international conference on world wide web. New York: ACM Press (2011). p. 695–704.
51. Fan R, Zhao J, Chen Y, Xu K. Anger is more influential than joy: Sentiment correlation in Weibo. PLoS ONE (2014) 9(10):e110184. doi:10.1371/journal.pone.0110184
52. Choudhury MD, Sundaram H, John A, Seligmann DD, Kelliher A. Birds of a Feather”: Does user homophily impact information diffusion in social media? (2010). Available at: https://arxiv.org/abs/1006.1702 (Accessed October 6, 2022).
53. Keskin Z, Aste T. Information-theoretic measures for non-linear causality detection: Application to social media sentiment and cryptocurrency prices (2019). Available at: https://arxiv.org/abs/1906.05740 (Accessed October 18, 2022).
54. Gligorić K, Anderson A, West R. Causal effects of brevity on style and success in social media. Proc ACM Hum Comput Interact (2019) 3:1–23. Article No.: 45. doi:10.1145/3359147
55. Egami N, Fong CJ, Grimmer J, Roberts ME, Stewart BM. How to make causal inferences using texts (2018). Available at: https://arxiv.org/abs/1802.02163 (Accessed October 20, 2022).
56. Roberts ME, Stewart BM, Nielsen RA. Adjusting for confounding with text matching. Am J Polit Sci (2020) 64:887–903. doi:10.1111/ajps.12526
Keywords: information diffusion, social media, talent show, elimination mechanism, effect estimation
Citation: Xu N, Lin Q, Hu H and Li Y (2022) The effect from elimination mechanism on information diffusion on entertainment programs in Weibo. Front. Phys. 10:1032913. doi: 10.3389/fphy.2022.1032913
Received: 31 August 2022; Accepted: 27 October 2022;
Published: 15 November 2022.
Edited by:
Jianguo LIU, Shanghai University of Finance and Economics, ChinaCopyright © 2022 Xu, Lin, Hu and Li. This is an open-access article distributed under the terms of the Creative Commons Attribution License (CC BY). The use, distribution or reproduction in other forums is permitted, provided the original author(s) and the copyright owner(s) are credited and that the original publication in this journal is cited, in accordance with accepted academic practice. No use, distribution or reproduction is permitted which does not comply with these terms.
*Correspondence: Haibo Hu, hbhu@ecust.edu.cn
†These authors have contributed equally to this work and share first authorship