- Division of Business Administration, Chosun University, Gwangju, South Kroea
We investigate the mechanisms of investors’ herding behavior using machine learning and textural data analysis from social media and the impact of sentiment in forming the herding behavior. We find that the abnormal information creation activity (AICA) for the retail investor is positive and statistically significant with the herding behavior, while informed investors with access to valuable information are negative with relation to the AICA. The herding behavior in firms traded by the retail investor is strongly related to the sentiment in social media at the cross-sectional level and has been more effective after COVID-19.
1 Introduction
The herding behavior of heterogeneous investors play an important role in the fundamental mechanism of financial markets. Consequently, much of the literature on herding behavior is associated with understanding investor characteristics and how the opinion of an informed agent might affect the herding effect in the financial market via the maintenance of their reputation [1–3] or maximize the incentive through learning from the other investors [4]. Despite the importance of this field in understanding potential channels of herding effects through social media, information studies investigating how social media information created by retail investors might affect the herding behavior in the financial market are relatively scarce [5,6].
As the number of social media users increases and activates, an enormous amount of data has been generated. In South Korea, especially, stock-related social media is very active. Naver, the largest portal site in South Korea, provides Naver Financial (http://finance.naver.com), which provides stock discussion room services that allow stock investors to share their opinions or information. The average number of messages per day is 39,504, and the average number of views is 15,608,238. Many of the writers working here are individual investors. Institutional investors obtain sophisticated information through specialized channels such as Bloomberg so that individual investors have relatively low-level information [7,8]. To overcome this, they use social media to obtain information and share opinions.
Retail investors are known as noise traders who move randomly and invest relatively little professional knowledge and advanced fundamental analysis. However, studies have shown that social media, where individual investors occupy a large proportion, is informative and not only explains the market but also affects the financial market [9]. Therefore, in this study, we analyze the effect of social media on herding behavior that plays an important role in the fundamental mechanism of financial markets. In other words, this study aims to confirm whether social media can be used as a variable to explain herding through regression analysis.
Much of the literature on herding behavior is associated with understanding investor characteristics and how the opinion of an informed agent might affect the herding effect in the financial market via the maintenance of their reputation [1–3] or maximizes the incentive through learning from the other investors [4]. Despite the importance of this field in understanding potential channels of herding effects through social media, information studies investigating how social media information created by retail investors might affect the herding behavior in the financial market are relatively scarce [5,6]. This serves as the main motivation in this study.
To test the investors’ herding effects on social media information, we adopt two key independent variables, the AICA and bullishness. Some researches argue that when the cost of an information search is low, investors obtain free information and herd [10,11]. We are motivated by the model of [7] to compute the abnormal information creation activity (AICA) index. Each listed firm’s historical search volume index (SVI) from Google Trends is stored because the Google search volume index can be the proxy for the information demand of informal retail investors. However, Google Trends has a disadvantage of including the amount of searched for people who do not invest in stocks. Therefore, in this paper, we redefined the information creation activity (ICA) as a variable that indicates information creation and supply by utilizing the number of daily posts in the Naver Financial stock discussion room where investors form their opinions directly. As individual investors have relatively lower-level information than other investors, they use the stock discussion room to obtain information and share opinions. Regression analysis is conducted to confirm that individual investors herd when the AICA is greater than the average.
Many studies explain the stock market using sentiment. Reference [12] showed that the volatility in the Chinese financial market is positively affected by B–W investor sentiment index, which is a composite index including CEFD, listing share turnover, the number and the average first-day M on IPO, the equity share in new issues and dividend premium [13]. Its sentiment has limitations that do not represent investors’ opinions directly. To overcome this problem, there are increasing studies to explain financial markets using opinions formed on social media and these studies are showing significant results [9]. The data used in this study directly represent the opinion of market participants, so we will check whether the sentiment formed on social media based on behavioral finance affects decision-making.
According to prospect theory, one of the behavioral finance theories, traders respond asymmetrically more sensitively to losses than to gains. In other words, people have a loss avoidance tendency [14]. Based on this theory, regression is conducted to check whether the bullish behavior is negatively related to herding behavior when the bullish behavior is bad. In addition, we check whether the investor sentiment is related to the herding behavior regardless of investor types.
Reference [15] discovered a significant peak of connectivity between investor sentiment and the stock market in the Chinese stock market during the COVID-19 pandemic. After COVID-19, the transaction volume of individual investors increases and the social media activation increases in South Korea. If we can confirm that the above two hypotheses are correct, we can establish the following hypothesis that bullishness and the AICA will still affect the herding of individual investors after COVID-19, that is, when the transaction volume of individual investors increases and the social media activation increases. We will try to confirm that main variables still have an effect on retail investors herding. Regression is conducted to check whether the variables still have an impact on retail investor herding behavior after COVID-19 by dividing before and after COVID-19 as of 22 March 2020, respectively, when social distancing was implemented in South Korea.
In this paper, we confirm these results. The effects of individual investor herding on the AICA are positive and statistically significant, implying that the retail investor who obtained information from social media follows into and out of the same equities. Its result supports the hypothesis stating that the retail investor’s herding is affected by the information production process in social media. The effects of individual, institutional and foreign investor herding on Bullish are negative and statistically significant. Based on prospect theory, investors react more sensitively in a bear market than in a bull market. The explanatory power of the regression analysis model is highest in the model explaining the herding of retail investors. Finally, the effect of AICA on the herding of individual investors after COVID-19 is still statistically significant based on the positive relation. AICA has a statistically significant negative relationship with the herding of institutional investors before and after COVID-19.
Our paper is meaningful to the study of the herding of individual investors, which has not been studied well in the past. Despite the importance of herding effects through social media, information studies investigating how social media information created by retail investors might affect herding behavior in the financial market are relatively scarce [5,6]. So, in this paper, the social media data that directly represent the opinions of individual investors are used to explain the herding of individual investors. So far, studies analyzing the relationship between social media and herding have looked at the overall level of the market [16]. The effect of social media on herding behavior by investor type is analyzed. By comparing the impact of variables measured using social media data before and after COVID-19, the effects of social media on herding by investor types are studied.
The remainder of this study is organized as follows. Section 2 reviews the past literature and develops the hypotheses. Section 3 offers the data and methodology. Section 4 presents the empirical results and analysis. The final section consists of the conclusion.
2 Literature review
In economics and finance, herding behavior, imitating actions mutually or following the decision-making of others, plays an important role in financial markets. Most of the literature on the herding of different types of investors explores institutional investor herding behavior. Money managers herd to maintain their reputation [1–3] or maximize the incentive through learning from other investors [4]. Few studies have concentrated on retail investor herding. Reference [5] found that retail investor herding behavior can be systematic and that buying behavior can be driven by past performance and the abnormal trading volume observed from individual investors’ trading. So in this paper, we analyze the retail investor herding using social media where they occupy a large proportion.
Several measurements are established to measure herding behavior and applied to numerous studies. The cross-sectional absolute deviation (CSAD) and the cross-sectional standard deviation of returns (CSSD) regard the overall herding existing in a specific market and do not allow researchers to distinguish between different types of investors as important [17–21]. The LSV measurement can categorize market participants into different types of investors [22,23]. Thus, we follow the LSV measurement [24] to estimate the herding behavior of each investor type.
Retail investors are known as noise traders who move randomly and invest relatively little professional knowledge in advanced fundamental analysis. However, there are studies that show that social media, where individual investors occupy a large proportion, is informative and not only explain the market but also affect financial markets [9]. Therefore, in this study, we analyze the effect of social media on herding behavior that plays an important role in the fundamental mechanism of financial markets. In other words, this study aims to confirm whether social media can be used as a variable to explain herding through regression analysis.
In the South Korea stock market where individual investors’ trading volume is numerically superior, there is a crucial need to understand individual investor herding in depth. This would also be a substantial contribution to the literature. The abnormal search volume index (ASVI) that is generated by individual investors is a better proxy for investors searching for information [7]. The ASVI, as a relevant proxy for information demand, allows us to examine the relationship between information demand and individual investor herding behavior [25]. Individual investors are more sensitive to public information than institutional investors and exhibit more significant herding of public information [26].
Along with the increase in the amount of data that directly represents the writer’s psychology and the development of technology for analysis, it is possible to analyze textual data at a low cost. Research is conducted to measure sentiment using text data. This approach can be divided into two parts.
One is to use a sentiment dictionary. The opinions in the text are divided into positive and negative words, and the text’s sentiments are measured through the sentiment dictionary. In the early days, the Harvard IV-4 dictionary, a common sentiment dictionary, was used in all fields. There are studies that analyze the relationship between stock returns and sentiment using this dictionary [27,28]. However, there is a limit in that the classification of the text using such a common sentiment dictionary can give insufficient accuracy [29]. Sentiment dictionary that specializes in the stock market was established and provided [30]. The prediction of stock returns has higher value when using a specific stock market sentiment dictionary other than the Harvard IV-4 dictionary [31]. Applying current data to existing dictionaries reduces accuracy because it is difficult to comprehend the implied meaning mainly used on the Internet and words can be used as other sentiments depending on a specific situation. Even some words do not exist in the dictionaries [32].
The other approach is to use machine learning to overcome this limitation. The number of studies using machine learning to classify sentiments is increasing. Reference [33] used a self-learning method to identify the relation between user reviews and bubble rating, by using TripAdvisor, well-liked American travel platform. Reference [9] investigated that the movement of the stock market was related to the sentiment of individual investors, who are the main users of postings, and the activity of the bulletin board. That is, the higher the buying opinions of the postings was, the lower the return rate the next day. Unlike foreign countries where there is a emotion dictionary specialized in the stock market, South Korea does not have a emotion dictionary specialized in the stock market. In this paper, using machine learning, we directly measure investor sentiments.
Retail investors use Google to search for companies to obtain financial information [7], and institutional investors use channels such as Bloomberg to obtain more sophisticated and advanced information than individual investors [8]. Reference [26] applied a trading volume-based herding measure and concluded that individual investors are more sensitive to public information than institutional investors and exhibit more significant herding to public information. In other words, based on previous studies, individual investors are relatively inferior to institutional investors; they use social media to obtain information or share opinions.
We are motivated by the model of [7] to compute the abnormal information creation activity (AICA) index. They stored each listed firm’s historical search volume index (SVI) from Google Trends because the Google search volume index can be the proxy for the information demand of informal retail investors. However, Google Trends has a disadvantage of including the amount of searched for people who do not invest in stocks. Therefore, in this paper, we redefine information creation activity (ICA) as a variable that indicates information creation and supply by utilizing the number of daily posts in the Naver Financial stock discussion room, where investors form their opinions directly by modifying the model of abnormal information creation activity (AICA) index. We set up the following hypotheses and conducted regression analysis for verification.
Hypothesis 1. AICA, which means the information creation activity of social media, is positively related to retail investor herding behavior.We expect there is a positive relation generated by the AICA when the dependent variable is retail investor herding behavior. Reference [7] discovered that utilizing the Google search engine is the most convenient method for individual investors to obtain considerable information. Because individual investors are relatively inferior to other investors, they will use the stock discussion room to obtain information and share opinions, and the main user of the stock discussion room may be retail investors [7,8]. Therefore, in this study, regression analysis is conducted to investigate the effect of social media information creation activity, AICA, on the herding behavior of individual investors when the activity of social media increases more than average.There are many studies that explain the stock market using the opinion formed in social media, and there are precedent studies showing significant results [34]. The data used in this study directly represents the opinion of market participants, so we check whether the opinion formed on social media affects decision-making based on behavioral finance. According to prospect theory [14], one of the behavioral finance theories, people respond asymmetrically more sensitively to losses than to gains. In other words, people have a loss avoidance tendency. Thus, we hypothesize that investors herd when negative opinions prevail.
Hypothesis 2. The bullish variable is negatively related with all types of investors’ herding.Bullish is calculated as an opinion score of bullishness, as proposed by [9]. This means that if positive opinions outweigh negative opinions, the bullish variable has a positive value. On the contrary, if negative opinions outweigh positive opinions, the bullish variable has a negative value. To examine the relationship between bullishness and investor’s herding, when negative opinions prevail, regression analysis is conducted.Figures 1A,B shows that the number of retail investors increases and that the number of posts on social media also increased after COVID-19. Reference [15] discovered a significant peak of connectivity between investor’s sentiment and stock market in Chinese stock market during the COVID-19 pandemic. In this paper, if we can confirm that the above two hypotheses are correct, we can establish the following hypothesis stating that the bullish variable and AICA still affect the herding of individual investors after COVID-19, that is, when the transaction volume of individual investors increases and the social media activation increases.
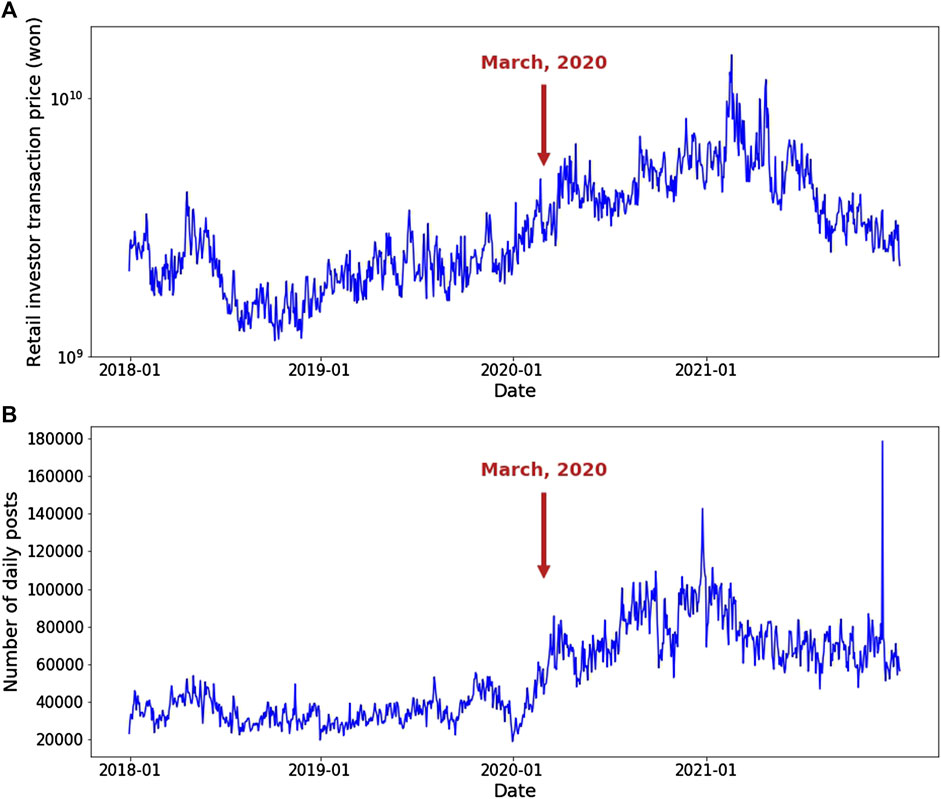
FIGURE 1. This figure shows the trading volume of retail investors and the number of daily posts of Naver Financial from 2018 to 2021. (A) Trading volume of retail investor. (B) The number of daily posts of Naver Financial.
Hypothesis 3. The relationship between variables and retail investor’s herding still has an effect after COVID-19.We try to show that the main variables still affect retail investor herding and whether the impact of AICA and Bullish on herding gets stronger or weaker after COVID-19. We conduct regression analysis as shown in Eq. 6 by dividing before and after COVID-19 as of 22 March 2020, respectively, when social distancing was implemented in South Korea.
3 Data and methodology
3.1 Data
Naver, the largest portal site in Korea, provides information on the Korean financial market through Naver Financial (http://finance.naver.com), which provides stock discussion room services that allow stock investors to share their opinions or information. The Naver Financial stock discussion room is most actively used by users and has the largest number of stock-related posts in South Korea.
This study employs the web-crawling method to download the posts of each firm from January 2018 to December 2021 in the stock discussion room provided by Naver Financial. The sample firms that have transactions in the KOSPI and KOSDAQ markets from January 2018 to December 2021 with an average of 10 or more daily posts are 971 companies.
Figure 2 shows the distribution of the average number of social media posts per day (Figure 2A) and per time (Figure 2B) used in the Naver Financial stock discussion room. Most of the posts can be seen on weekdays when stock-trading takes place (9:00 a.m.) before closing (15:30 p.m.). Therefore, this study uses only the posts posted when the stock market is open during trading days when measuring investment sentiment.
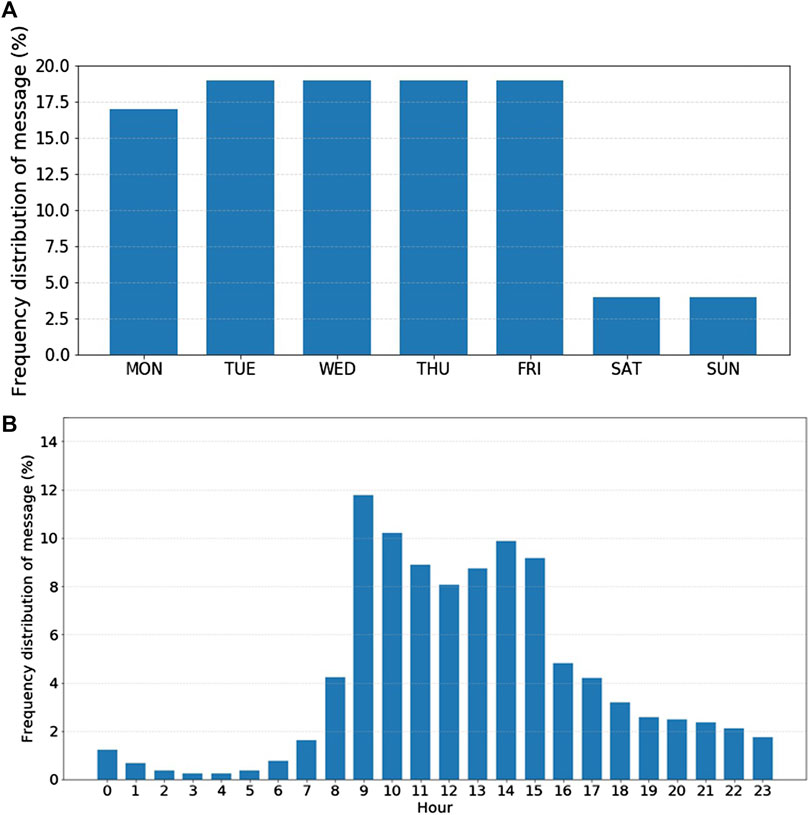
FIGURE 2. This figure shows the distribution of social media posts by day and time from 2018 to 2021. (A) Distribution of social media posts by day. (B) Distribution of posts by time.
The financial market data, including adjusted stock price, the number of issued stocks, PER, the trading volume and the transaction price according to the investor type, are downloaded from FnGuide. Market capitalization is calculated as the product of the adjusted price and the number of common stock shares and is taken as the natural logarithm to reduce scale. Trading volume is also taken as the natural logarithm for the same reason. Daily return is calculated by adjusted stock price. The STD is the standard deviation of daily returns from the previous 3 months.
3.2 Methodology
3.2.1 AICA measure
We are motivated by the model of [7] to compute the abnormal information creation activity (AICA) index. They downloaded each listed firm’s historical search volume index (SVI) from Google Trends because the Google search volume index can be the proxy for the information demand of informal individual investors. However, Google Trends has an disadvantage of including the amount of searched for people who do not invest in stocks. Therefore, in this paper, we redefine information creation activity (ICA) as a variable that indicates information creation and supply by utilizing the number of daily posts in the Naver Financial stock discussion room where most of the writers are presumed to be stock investors. We adjust the ICA variable to control for time trends. AICA is defined as the log of ICA during the day minus the log of the median ICA during the previous 8 days. The AICA measure is as follows:
Where log (ICAi,t) is the logarithm of ICA for each stock (i) in that time (t) and log [Med (ICAi,t−1, … … , ICAi,t−8)] is the logarithm of the median ICA value for each stock (i) during the previous 8 days. If the activity of social media is greater than the average value, it is a positive value, and if it is smaller than the average value, it is a negative value.
3.2.2 Opinion labeling process
The purpose of this paper is to analyze the relationship between the investment sentiment indicator formed with social media and the herding value of three different investors (foreigner, institution, and retail investor types). The general method of assigning a sentiment is to use sentiment dictionaries. There are sentiment dictionaries in Korea, but they are not specialized in the financial market. Financial markets are sensitive to economic conditions, and the sentiments of words vary with context. Thus, ordinary sentiment dictionaries have difficulty comprehending the implied meaning depending on a specific situation, and some words do not exist in the dictionaries [32]. As there are so many limitations, we do not use an existing sentimental dictionary. Because there is no sentiment dictionary that specializes in the Korean financial market, we use the machine learning method to classify text data according to the context and conditions. Machine learning can be established when the sentiment dictionary specialized in financial markets does not exist.
3.2.3 Bullish measure
In this study, we use the bullish measurement presented by [9] as an investment sentiment indicator. The bullish measurement is as follows:
Bullishi,t, the measurement of the opinion score of bullishness, is calculated by the daily (t) number of buying opinion posts
3.2.4 Herding measure
The cross-sectional absolute deviation (CSAD) and the cross-sectional standard deviation of returns (CSSD) regard the overall herding existing in market [17–21]. The authors measured the relationship between herding behavior and investor attention at the market-wide level. Reference [16] identified relations between herding behavior calculated by CSAD measurement and Google search volume index in 21 international equity markets. Reference [35] analyzed United States and United Kingdom financial markets for herding behavior. The LSV measurement can categorize market participants into different types of investors [22,23]. Reference [25] studied the effect of social media on herding by investor type in the Taiwan stock market. They used Google search volume index (ASVI) representing investor attention was used as a major variable. So, to estimate the herding behavior of each investor type. We follow the LSV measurement [24]. In LSV measurement, each letter is the daily (t) trading herding index for each stock (i) and each type of investor, including retail, institutional and foreign investors [22,23]. The herding measure is as follows:
Where Bi,j,t is the transaction price of buying for each stock (i) and investor type (j) in a given day (t) and Si,j,t is the transaction price of selling for each stock (i) and investor type (j) in a given day (t). Hi,j,t is the transaction price of buying to the total number of trades of each stock (i) for investor type (j) in a given day (t). pj,t is the average value of Hi,j,t for all stocks for each investor type (j) in a given day (t). AFi,j,t is the adjustment factor for the herding measure that adjusts the scale difference for trading volume. AFi,j,t accounts for the fact that for the null hypothesis of no herding, which is when the probability of an investor being a net buyer of any stock (i) is pj,t; the absolute value of Hi,j,t − pj,t is greater than or equal to zero. Thus, AFi,j,t is defined as the expected value of |Hi,j,t − pj,t| assuming the null hypothesis of no herding. Bi,j,t follows a binomial distribution with a probability of success pj,t. Thus, AFi,j,t is easily calculated given pj,t and the number of investors active in stock (i) in that time (t). For any stock (i), the number of active investors, AFi,j,t, decreases.
3.2.5 Regression model
We set up the hypotheses and conduct regression analysis for verifications. We followed studies by [26], who argued that investor herding may be affected by beliefs about other types of investors when consensus occurs. Thus, we added a control variable when the dependent variable Herdi,j,t is retail investor herding behavior. Herdi,k,t and Herdi,l,t are the control variables when the dependent variable is institutional and foreign investor herding behavior and vice versa.
We use the following variables: Herdi,j,t is the specific type (j) of investor herding. Herdi,k,t and Herdi,l,t are the other investor herding types except for the type (j) of investor herding. AICAi,t is abnormal ICA for stock (i) in time (t). lnSIZEi,t is the value taking the natural log of market capitalization. RETi,t−1 is the past return of stock (i) during the previous day. PERi,t is the P/E ratio for stock (i). lnVOLi,t is the value taking the natural log of trading volume for stock (i) in time (j). STDi,t is the standard deviation of daily returns for stock (i) during the previous 3 months. ϵi,j,t is the disturbance term [36,37]. The variables should be controlled because they are related to the characteristics of the company. Companies can be affected by industry and year, so the industrial fixed effect and yearly fixed effect are considered.
To confirm Hypothesis 1, “AICA, abnormal information creation activity of social media, is positively related to retail investor herding behavior,” we regress. We expect there is a positive significant coefficient generated by β1 in Eq. 5 when the dependent variable Herdi,j,t is retail investor herding behavior [7,8]. As individual investors have relatively lower-level information than other investors, they use the stock discussion room to obtain information and share opinions. Regression analysis is conducted to confirm that individual investors herd when the abnormal information creation activity, AICA, is greater than the average.
To confirm Hypothesis 2, “the bullish variable is negatively related to all types of investors’ herding,” we conduct regression. We expect there to be a negative significant coefficient generated by α1 in Eq. 6 when the dependent variable Herdi,j,t is in all types of investors’ cases. According to behavioral finance, when the direction of utility is the loss not the profit, i.e., it more sensitive, it reacts [14]. In other words, there is greater pain when it falls, decreases or is bad. Based on this theory, regression is conducted to check whether the bullish variable is negatively related to herding behavior when bullishness is bad. In addition, we verify that it is applied to all types of investor herding using Eq. 6.
To confirm Hypothesis 3, “the relationship between variables and retail investor’s herding still has an effect after COVID-19,” we estimate the regression as shown in Eq. 6 by dividing before and after COVID-19 as of 22 March 2020, respectively, when social distancing was implemented in South Korea. After COVID-19, the number of retail investors increased, and social media activity also increased. Therefore, regression analysis is conducted to check whether the variables influence investor’s herding behavior after COVID-19.
4 Empirical results
4.1 Summary statistics
For the empirical analysis, we used the 971 companies with an average of 10 or more daily posts from January 2018 to December 2021. In this study, the posts of the Naver Financial stock discussion room during the sample period are collected by the web crawling method using Python. The financial market data, including adjusted stock price, the number of issued stocks, PER, trading volume and transaction price according to the investor type, are acquire from FnGuide.
Table 1 shows the summary statistics of the variables used in regression analysis, and it represents summary statistics of whole sample firms calculated with the average value of daily data. AICA is the abnormal information creation activity calculated by Eq. 1. If the activity of social media is greater than the average value, it is a positive value, and if it is smaller than the average value, it is a negative value. Bullish is an investment sentiment indicator that represents opinion score. If the bullish variable is a negative value for a specific firm, investors in social media think the firm’s market value is negative. If it is a positive value, the firm’s market value is positive. Herd_Retail, Herd_Fore. and Herd_Insti. represent retail, foreign and institutional herding behavior measures, respectively, and are calculated Eqs 3, 4. lnSIZE is the value taking the natural log of market capitalization. PER is the P/E ratio. lnVOL is the value taking the natural log of the trading volume. STD is the standard deviation of daily returns for the previous 3 months. Panel A represents summary statistics of regression variables, and Panel B represents the correlation between regression variables.
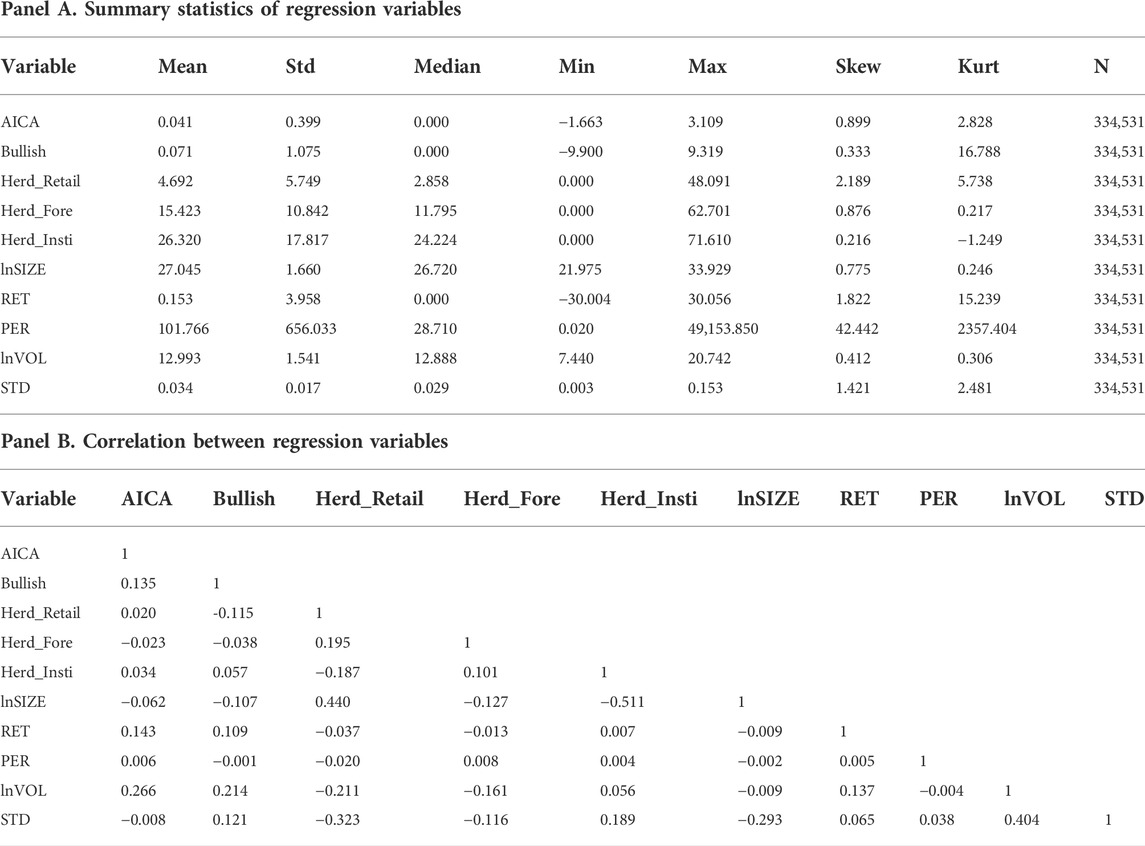
TABLE 1. Summary statistics. Table reports the summary statistics of regression variables used in regression analysis. AICA is abnormal information creation activity. Bullish is an investment sentiment indicator that represents an opinion score. Herd_Retail, Herd_Fore. and Herd_Insti. are retail, foreign and institutional herding behavior measures, respectively. lnSIZE is the value taking the natural log of market capitalization. PER is the P/E ratio. lnVOL is the value taking the natural log of the trading volume. STD is the standard deviation of daily returns from the previous 3 months. Panel A represents summary statistics of regression variables, and Panel B represents the correlation between regression variables.
Table 1 (Panel A) reports the average herding value of three different investors (foreigner, institution, and retail investor types) measured by Eqs 3, 4 in our sample period (approximately 4 years). The institution and foreigner investors, as informed investors, show a significantly higher herding behavior than those for retail investors, as noise investors. This result was obtained because informative investors who refer to fundamental sources have a relatively large herding effect, and noise investors who move randomly have relatively small herding effects. The kurtosis of the bullish variable, 16.788, is heavy tailed because it has a larger value than the normal distribution. The large bullish value means there are many positive opinions, and therefore, the return is also high. As the relationship between Bullish and the return move in the same direction, the kurtosis of Bullish and that of the return (16.788 and 15.239, respectively) have similar values. The results in Table 1 (Panel A) reveal a different herding behavior according to the investor types and show heterogeneous features in the social media sentiment, primarily driven by investors who are passionate about social media. Panel B reports the correlation between the main variables used in regression analysis. On average, there are 971 firms and 334,531 data points with at least one social media activity each day. The correlation between Bullish and AICA, which are the main variables in this paper, is 0.135, which is low. This indicates that there is no multicollinearity, so it is possible to use these two variables for regression analysis at the same time.
4.2 Herding behavior regression estimates
If heterogeneous investors used similar information set to trade a specific stock, then the investors show a herding behavior. To test the investors’ herding effects on social media information, we adopt the two key independent variables, the AICA and bullishness. The novel information created from the social media activity is used as a valuable information channel to explain the stock market movement [38]. Despite results about the positive relationship between social media and the stock market, the study of the investors’ herding behavior through social media information is insufficient. Therefore, this study aims to confirm whether social media can be used as a variable to explain herding through regression analysis.
Here, we present an empirical analysis of the hypotheses described in Section 2. First, we present evidence that AICA, as the information created from the social media activity, influences the herding behavior of the retail investor. We employ the regression analysis about the relation between the retail investors’ herding behavior and social media information. As a rigorous test of this relationship, we measure the herding effect for heterogeneous investors of a certain firm on day t by the LSV measurement [24]. Naver Finance, as an information source, is social media optimized for stocks. The data created from the Naver Finance website is different from Google data and includes the number of searches of people who do not invest in stocks. Therefore, we define abnormal information creation activity (AICA) as social media information and supply it by utilizing the number of daily posts in social media where investors form their opinions directly by modifying the model of the abnormal search volume index provided by [7]. We regress the investors’ herding behavior on the novel information in social media using Eq. 5. In this equation, the dependent variable is the investor’s herding behavior. The explanatory variables of interest are AICA, the information created from the social media activity to measure the abnormal behavior, and Herdi,k,t, the herding behavior of other investors. The coefficients β1 and β2 test the social media effect on the investors’ herding behavior predicted in Section 2. Other independent variables contain firm control variables [36,37]. We include yearly and industrial fixed effects to remove time trends and industry features.
Table 2 reports the regression estimates. The AICA coefficients are positive and statistically significant, implying that the retail investor who obtained the information from social media follows in and out of the same equities. On the other hand, the correlation coefficients of the effect of institution and foreign investor herding behavior on the AICA are negative and statistically significant, indicating that the informed traders of social media activity follow in and out of the different stocks. This result supports the hypothesis that the retail investor’s herding is affected by the information production process in social media. Individual investors use Google to gather valuable information for the investor, and institutional investors use channels such as Bloomberg to obtain more sophisticated and advanced information than individual investors [7,8]. Therefore, retail investors with relatively deficient information levels use social media information to obtain and share information and confirm that individual investors herd when the activity is greater than the average. Institutional and foreign investors show the opposite pattern with respect to individual investors in the Korean stock market [39]. The explanatory power of the regression analysis model is highest in the model explaining the herding of individual investors because the users in social media are retail investors. As a result, Hypothesis 1 suggesting that AICA is related to the herding of individual investors is confirmed.
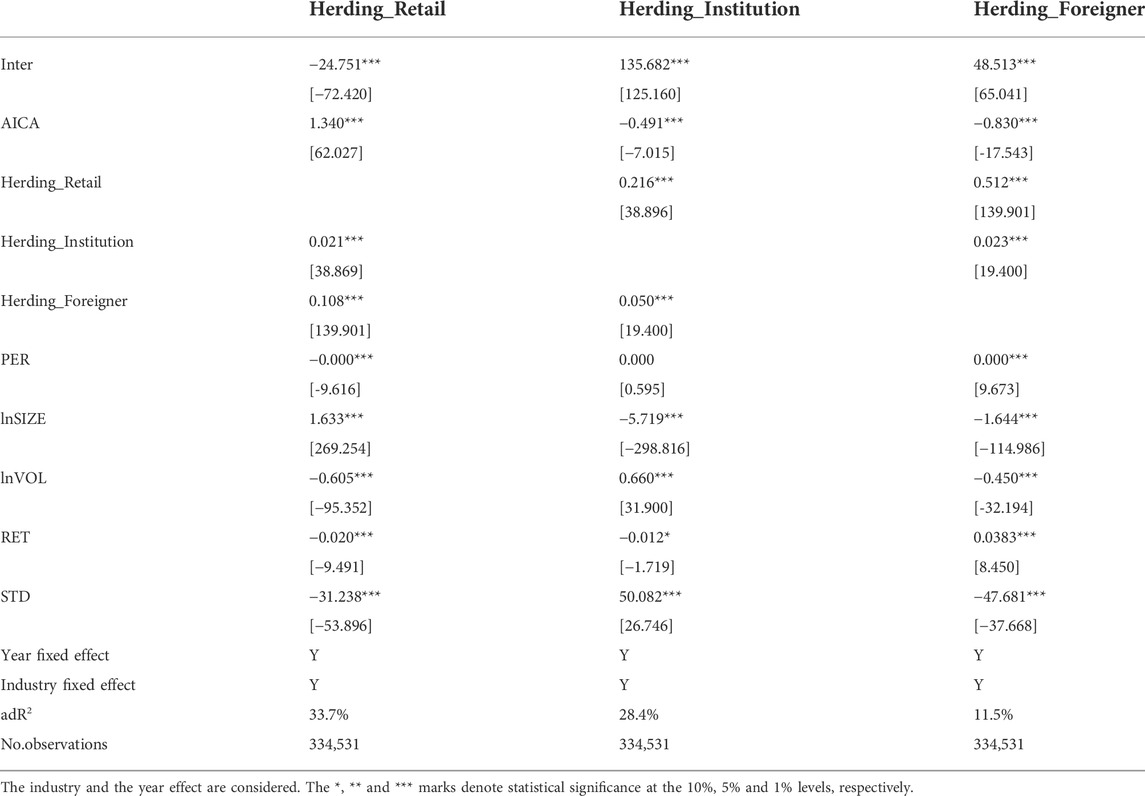
TABLE 2. Relationship between investor herding behavior and AICA. Table reports the impact of AICA on the retail, foreign and institutional investor herding measures. This table presents the parameter in Eq. 5. Herd_Retail, Herd_Foreigner and Herd_Institution are retail, foreign and institutional herding behavior measures, respectively. AICA is the abnormal ICA. lnSIZE is the value taking the natural log of market capitalization. PER is the P/E ratio. lnVOL is the value taking the natural log of the trading volume. STD is the standard deviation of daily returns from the previous 3 months.
The coefficients of interest in Table 2 have the opposite sign according to investor types. This increases the possibility that factors for investor types correlated with investor’s herding behavior are behind, indicating whether the investor sentiment is related to the herding behavior regardless of investor types. Next, we attempt to rule out this factor by estimating opinions in social media messages. Specifically, we examine how the sentiment and activity in social media are associated with herding behavior. If investors use valuable information from the opinion formed from social media to buy (or sell) specific securities, then the sentiment in social media and investors’ herding behavior should be closely related. There are many studies that explain the stock market using the opinion formed in social media, and precedent studies are showing significant results. The data used in this study directly represent the opinion of market participants, so we will check whether the sentiment formed on social media based on behavioral finance affects decision-making. According to prospect theory, one of the behavioral finance theories, traders respond asymmetrically more sensitively to losses than to gains. In other words, people have a loss avoidance tendency [14].
We define the bullish variable calculated by Eq. 2 as an opinion score in social media activity, as proposed by [9]. All bullish variable are real numbers. If the bullish variable is a negative value for a specific firm, investors in social media think the firm’s market value is negative. If it is a positive value, the firm’s market value is positive. To examine the relationship between bullishness and investor herding, we perform the regression analysis defined in Eqs 3, 4. We also establish the control variables to remove the firm’s characteristics [36,37]. In addition, each company can be affected by industry and year, so the industrial fixed effect and yearly fixed effect are considered. In the regression of Eq. 6, the dependent variable is investor herding, and the independent variable is bullishness. Table 3 shows the regression analysis result of Eq. 6.
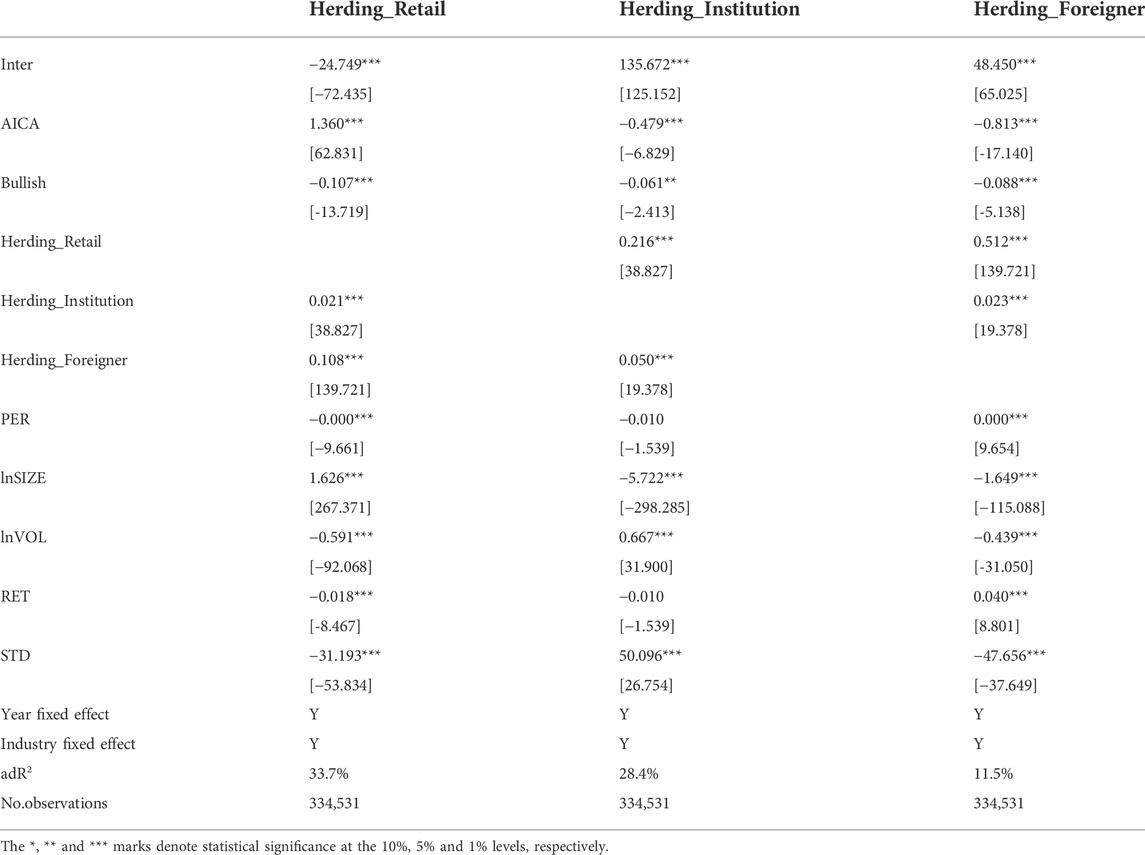
TABLE 3. Relationship between investor herding behavior and Bullish. Table reports the impact on Bullish on the retail, foreign and institutional investor herding measures. This table presents the parameter in Eq. 6. Herd_Retail, Herd_Foreigner and Herd_Institution are retail, foreign and institutional herding behavior measures, respectively. Bullish is an investment sentiment indicator that represents the opinion score. AICA is the abnormal ICA. lnSIZE is the value taking the natural log of market capitalization. PER is the P/E ratio. lnVOL is the value taking the natural log of the trading volume. STD is the standard deviation of daily returns from the previous 3 months. The industry and the year effect are considered.
Table 3 reports an estimate of the regression in Eq. 6 after including bullishness and AICA in the sample. We measure the bullishness of social media activity for specific firms. The finding in Table 3 shows the role of sentiment in social media. The bullish coefficient is negatively related to all types of investor herding, suggesting that based on prospect theory, investors react more sensitively in a bear market than in a bull market. The explanatory power of the regression analysis model is highest in the model explaining the herding of retail investors. As a result, the bullish variable being negatively related to the herding of individual investors can be confirmed as described in Hypothesis 2.
4.3 Impact of COVID-19 on the association between AICA, bullishness and herding behavior
We found that the AICA and bullish variables have a statistically significant effect on the herding behavior of investors in Tables 2, 3. Figures A,B shows that the number of retail investors has increased and that the number of posts on social media has also increased after COVID-19. Therefore, we try to show that the main variables still affect retail investor herding and whether the AICA and Bullish in social media can explain the investor’s herding behavior after the COVID-19 event as an economic crisis. We examine the association between AICA and Bullish variables and herding behavior before and after COVID-19. We conduct regression analysis as shown in Eq. 6 by dividing before and after COVID-19 as of 22 March 2020, when the social distancing policy started in South Korea, respectively.
In Table 4, we compare the result of herding behavior for three investor types, such as retail, institution, and foreign investors using Eq. 6 between before (Panel A) and after (Panel B) COVID-19. The impact of AICA and Bullish on the herding behavior of the retail investor is still significant but the impacts are weaker (AICA: 1.634 vs. 0.9748, Bullish: 0.1046 vs. -0.0816), after COVID-19. AICA and Bullish variables are statistically meaningless to institutional investor herding after COVID-19. The effect of AICA on herding behavior of the foreign investor is stronger (−0.066 vs. −1.032) and the impact of Bullish on herding of foreign investor herding is weaker (−0.113 vs. −0.055), after COVID-19.
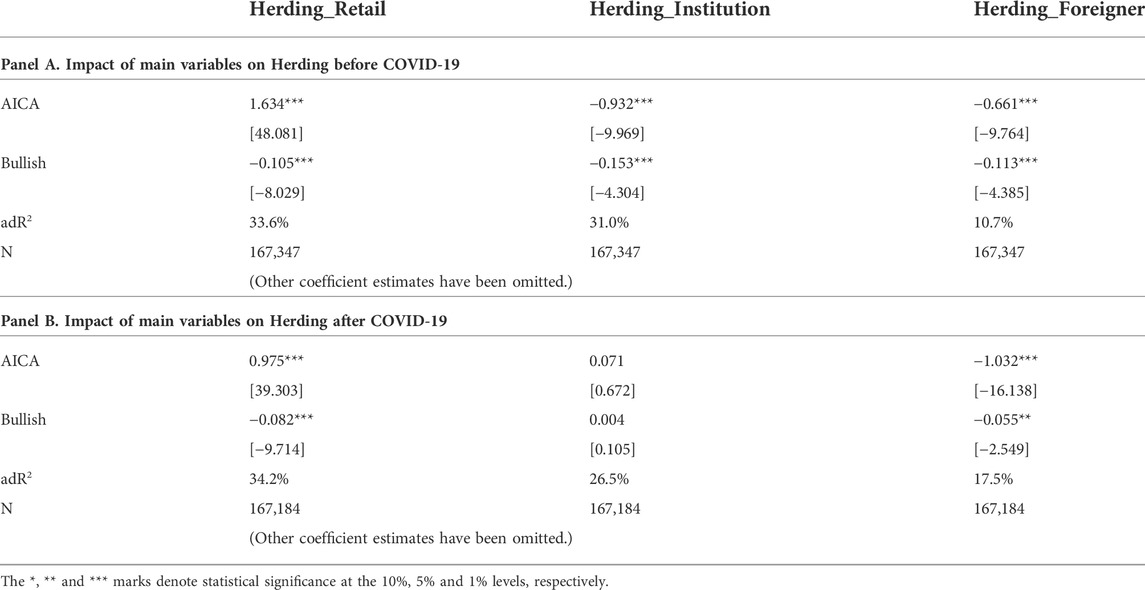
TABLE 4. Impact of COVID-19 on the association between AICA, Bullish and Herding. Table reports impact of COVID-19 on the association between AICA, Bullish and investor’s Herding. Regression analysis is performed by dividing COVID-19 into before and after with 22 March 2020 as the reference date and this table represents regression results using Eq. 6. AICA is the abnormal ICA. Bullish is an investment sentiment indicator that represents the opinion score. For brevity, We only show the results of the main variables in the table and the coefficient estimates of controlled variables are suppressed.
Overall, empirical results are statistically significant relation between main variables, such as AICA and Bullish and herding behavior even after COVID-19. The results shown in Table 4 confirm Hypothesis 3, which argues that the relationship between main varaibles and herding behavior still shows statistically significant after the COVID-19 crisis. However, overall, the COVID-19 event weakens the effect of AICA and Bullish on herding behavior.
4.4 Robustness test
The significant issue in our analysis above is that the measure for social media activity is possibly endogeneous variable. It is true for make information that the capability to extract valuable information on the firm within social media activity is limited because of small social media activity. We face a bias because it is difficult to get valuable information in firms with low social media activity. Here, we test whether the degree of social media activity is associated with the relationship between AICA and Bullish and herding behavior. We replicate the empirical analysis of Table 3 and show the result of robustness tests by constructing subsample groups composed of sample firms with an average of 20 or more posts per day (Panel A) and sample firms with an average of 30 or more posts per day (Panel B) using Eq. 6. In Panel A and B of Table 5, the relationship between AICA and the herding behavior for three types shows a statistically significant negative, while the association between AICA and individual investor herding is a positive value with statistically significant. These findings imply that herding behavior is not affected by the degree of social media activity. The results shown in Table 5 are consistent with the observation in Table 3 and confirm Hypotheses 1 and 2.
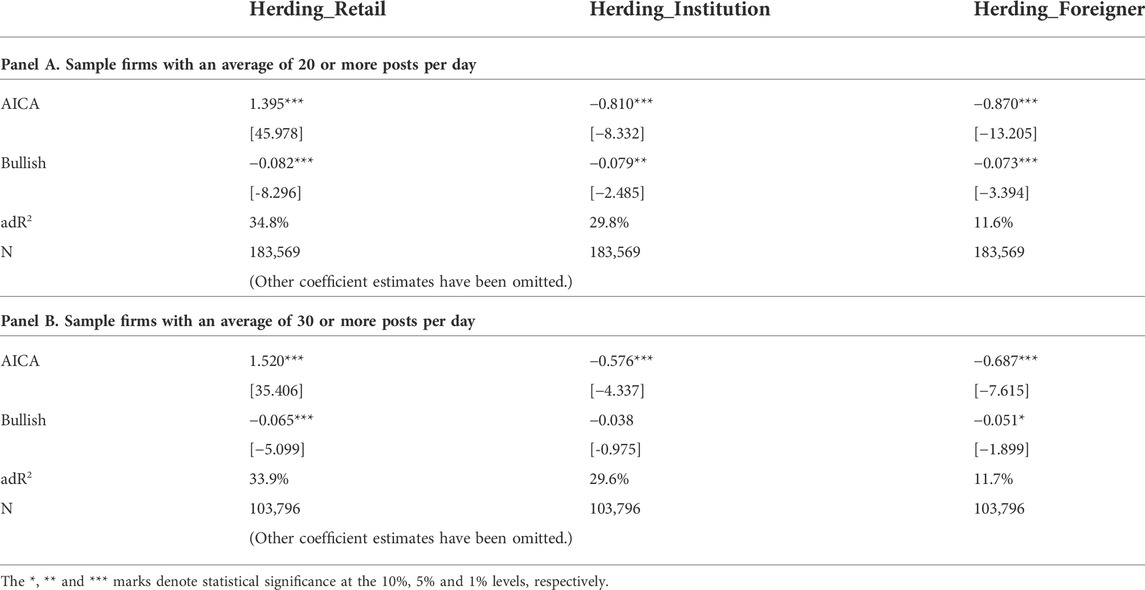
TABLE 5. Robustness tests of subsample groups. Table reports robustness tests of sub samples, applying sample firms with an average of 20 or more posts per day (Panel A) and sample firms with an average of 30 or more posts per day (Panel B). This table presents the parameter when we apply subsample groups using Eq. 6. AICA is the abnormal ICA. Bullish is an investment sentiment indicator that represents the opinion score. For brevity, We only show the results of the main variables in the table and the coefficient estimates of controlled variables are suppressed.
Another potential issue is the portion of the retail investor. Firms with higher retail investor activity may have more relationships than those with small retail investor activity. Because companies with small retail trading volume tend to be firms with lower social media activity, these would be the companies that might the week influence by information created from social media. We analyze whether the degree of retail investor activity affects the observed outcome in Tables 2, 3. We estimate the relationship between AICA and Bullish and herding behavior according to the retail investor ratio. We divided the whole sample into two subsamples with respective to the degree of retail investor activity and estimate the relationships using Eg (6). In Panel A of Table 6, we analyze the regression analysis with a subsample constructed above the media retail investor ratio. We find that the Bullish coefficient is negatively related to herding behavior regardless of investor type. However, the AICA is negatively related to the herding behavior of both institutions and foreign investors, whereas the retail herding behavior shows a positive relationship. The results from Panel B in Table 6 are consistent with Table 3. It is because the dominant users of social media are retail investors, so overall, we find that the subsample with a higher retail investor ratio is associated with more significance related to the results in Tables 2, 3. In Panel B of Table 6, the group below the median retail investor ratio shows that the relations between two investors, such as retail and institution herding behavior, and AICA are statistically significant positive whereas the relationship between foreigner herding behavior and AICA is negative with statistically significant. We find that Bullish as an investor’s sentiment is a statistically negative relation with the herding behavior of both the retail and foreign investors while showing a positive relationship with the herding behavior of institutional investor.
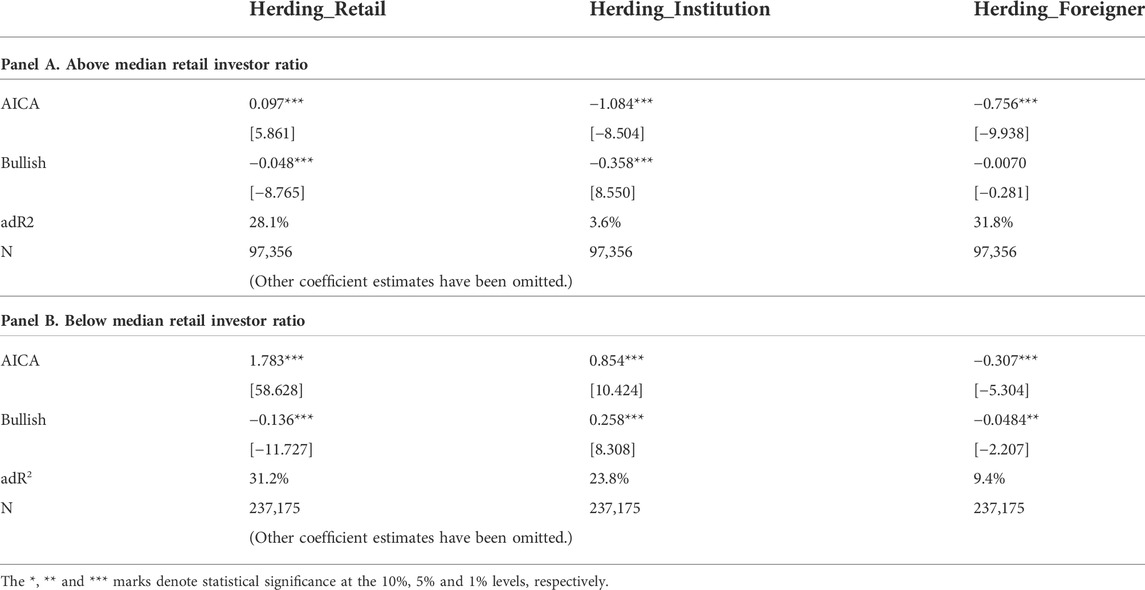
TABLE 6. Robustness tests of retail investor ratio groups. Table reports impact of key variables on herding separated into different retail investor ratio groups. This table presents the parameter in Eq. 6. Regression analysis is performed by dividing into two groups, above and below median retail investor ratio underlying trading volume. AICA is the abnormal ICA. Bullish is an investment sentiment indicator that represents the opinion score. For brevity, We only show the results of the main variables in the table and the coefficient estimates of controlled variables are suppressed.
We further expand our analysis on the impact of AICA and Bullish on herding behavior in terms of industry characteristics. We estimate the regression by grouping industry to enhance the robustness of the empirical results. To fix statistical significance, we use industry exceeding 30 companies, including Manufacturing, Wholesale and retail trade, Information and communication, and Professional, scientific and technical activities. In Table 7, we collected subsample data according to the above condition. We conduct a test of data on whether the relationship between the social media information and herding behavior is related to the industry characteristics, overall there is a significant relation between the AICA and Bullish and herding behavior. The results show the positive relationship between the AICA and individual investor herding behavior, while for the institution and foreign investors, there is a negative relationship with statistically significant, regardless of industries. We also find a similar result in Table 3 for Bullish variables. In sum, we extend our robustness tests by analyzing a subsample that reflected specific industry characteristics. These findings show that statistically, significant coefficients are consistent with the results in Table 3.
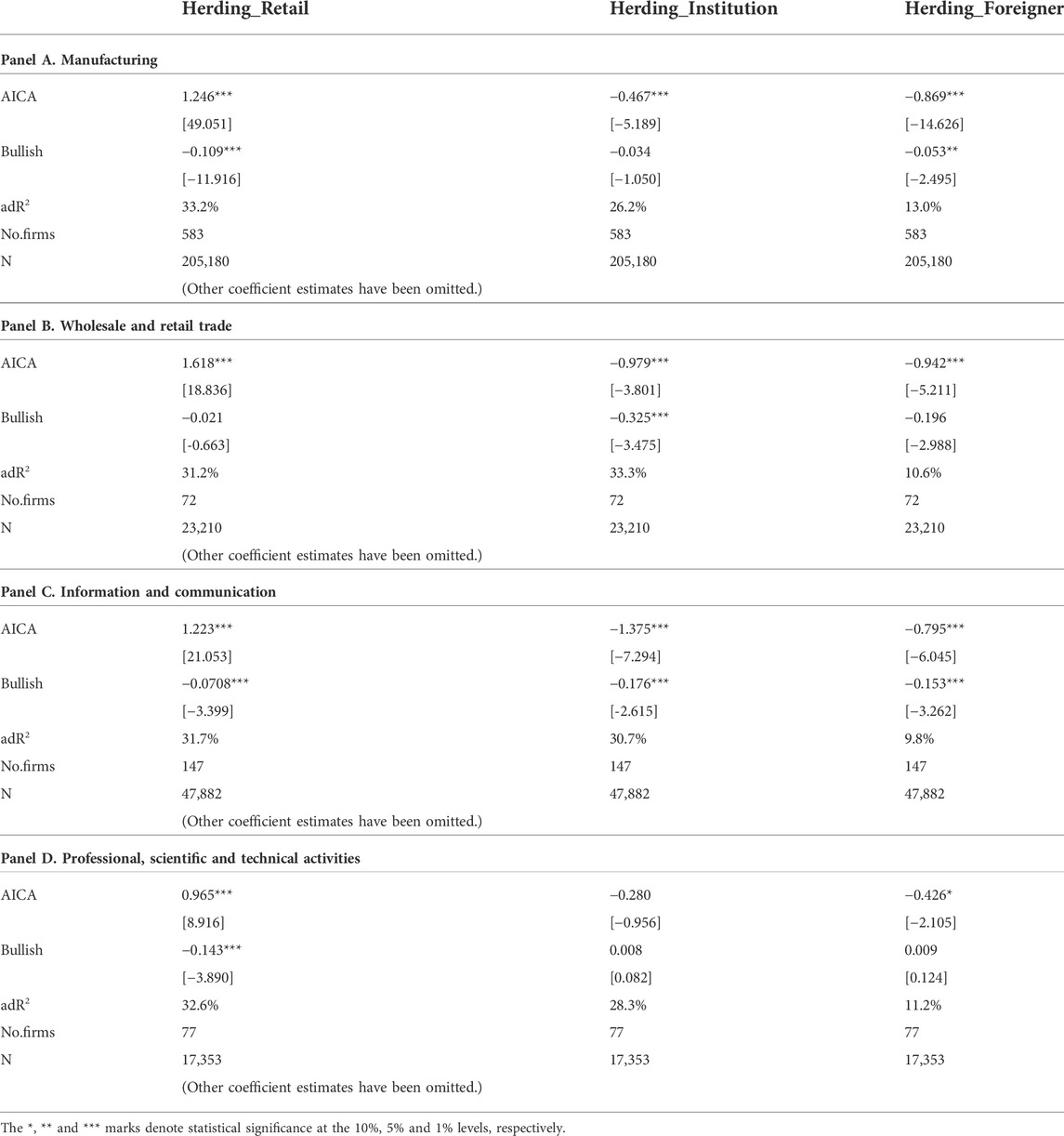
TABLE 7. Robustness tests of industry group. Table shows the coefficients of the results of regression analysis by industry are shown using Eq. 6 excluding industry fixed effect. Panel A is Manufacturing industry, Panel B is Wholesale and retail trade industry, Panel C is Information and communication and Panel D is Professional, scientific and technical activities industry. AICA is the abnormal ICA. Bullish is an investment sentiment indicator that represents the opinion score. For brevity, We only show the results of the main variables in the table and the coefficient estimates of controlled variables are suppressed.
5 Conclusion
In this paper, we present novel results on the social media information responsible for investors’ herding behavior and implications of the usefulness of social media information for the herding behavior. We show that abnormal information creation activity (AICA) is positively related to the herding behavior of retail investors, while institution and foreign investors are negatively affected by the AICA. The evidence suggests that social media activity is the key factor driving investor herding behavior.
With the sentiment in social media activity focused on a specific firm, this paper uses a bullish measure in social media messages, which are the opinions of investors in social media. In this study, abnormal information creation activity (AICA) and Bullish are calculated using data from social media to examine the impact of these variables on investor herding from 2018 to 2021. It is confirmed that the AICA variable has a statistically significant positive value for the herding of individual investors. The AICA variable has a statistically significant negative value for the herding of institutional and foreign investors. In other words, as its sign is opposite the sign for the effect on the herding of individual investors, it seems that the characteristics of the Korean stock market, which are opposite the trading patterns of foreigners and institutions, of individual investors are reflected from the perspective of herding. It is confirmed that the bullish variable is a statistically significant negative value for the herding of all investor types. It seems to reflect the theory of behavioral finance, which is more sensitive to losses than to gains.
Finally, we confirm that the effect of key variables on the herding of individual investors is statistically significant, even after COVID-19. This seems to reflect the fact that social media became more active and that the number of individual investors increased after COVID-19. It is meaningful that the study of the herding of individual investors, which has not been studied well in the past, is conducted. The social media data that directly represent the opinions of individual investors are used to explain the herding of individual investors. By comparing the effects of variables measured using social media data before and after COVID-19, the effects of social media on herding by investors types is improved.
Data availability statement
The raw data supporting the conclusion of this article will be made available by the authors, without undue reservation.
Author contributions
JY wrote the main manuscript text. GO directed and revised the manuscript and contributed to all aspects of this work. JY and GO conducted the experiment and analyzed the data. All authors reviewed the manuscript.
Funding
This work has been supported by NRF (National Research Foundation of Korea) Grant funded by the Korean Government (NRF-2022R1F1A1068796).
Conflict of interest
The authors declare that the research was conducted in the absence of any commercial or financial relationships that could be construed as a potential conflict of interest.
Publisher’s note
All claims expressed in this article are solely those of the authors and do not necessarily represent those of their affiliated organizations, or those of the publisher, the editors and the reviewers. Any product that may be evaluated in this article, or claim that may be made by its manufacturer, is not guaranteed or endorsed by the publisher.
References
1. Scharfstein DS, Stein JC. Herd behavior and investment. The American economic review (1990). p. 465–79.
2. Trueman B. Analyst forecasts and herding behavior. Rev Financ Stud (1994) 7:97–124. doi:10.1093/rfs/7.1.97
3. Graham JR. Herding among investment newsletters: Theory and evidence. J Finance (1999) 54:237–68. doi:10.1111/0022-1082.00103
4. Chevalier J, Ellison G. Career concerns of mutual fund managers. Q J Econ (1999) 114:389–432. doi:10.1162/003355399556034
5. Barber BM, Odean T, Zhu N. Systematic noise. J Financial Markets (2009) 12:547–69. doi:10.1016/j.finmar.2009.03.003
6. Blasco N, Corredor P, Ferreruela S. Market sentiment: A key factor of investors’ imitative behaviour. Account Finance (2012) 52:663–89. doi:10.1111/j.1467-629x.2011.00412.x
7. Da Z, Engelberg J, Gao P. In search of attention. J Finance (2011) 66:1461–99. doi:10.1111/j.1540-6261.2011.01679.x
8. Da Z, Engelberg J, Gao P. The sum of all fears investor sentiment and asset prices. Rev Financ Stud (2015) 28:1–32. doi:10.1093/rfs/hhu072
9. Antweiler W, Frank MZ. Is all that talk just noise? The information content of internet stock message boards. J Finance (2004) 59:1259–94. doi:10.1111/j.1540-6261.2004.00662.x
10. Bikhchandani S, Hirshleifer D, Welch I. A theory of fads, fashion, custom, and cultural change as informational cascades. J Polit Economy (1992) 100:992–1026. doi:10.1086/261849
11. Sgroi D. Optimizing information in the herd: Guinea pigs, profits, and welfare. Games Econ Behav (2002) 39:137–66. doi:10.1006/game.2001.0881
12. Xie D, Cui Y, Liu Y. How does investor sentiment impact stock volatility? New evidence from shanghai a-shares market. China Finance Rev Int (2021). doi:10.1108/cfri-01-2021-0007
13. Baker M, Wurgler J. Investor sentiment and the cross-section of stock returns. J Finance (2006) 61:1645–80. doi:10.1111/j.1540-6261.2006.00885.x
14. Kahneman D, Tversky A. Prospect theory: An analysis of decision under risk. Econometrica (1979) 47:263. doi:10.2307/1914185
15. Mezghani T, Boujelbène M, Elbayar M. Impact of Covid-19 pandemic on risk transmission between googling investor’s sentiment, the Chinese stock and bond markets. China Finance Rev Int (2021) 11:322–48. doi:10.1108/cfri-08-2020-0120
16. Wanidwaranan P, Padungsaksawasdi C. Unintentional herd behavior via the Google search volume index in international equity markets. J Int Financial Markets, Institutions Money (2022) 77:101503. doi:10.1016/j.intfin.2021.101503
17. Christie WG, Huang RD. Following the pied piper: Do individual returns herd around the market? Financial Analysts J (1995) 51:31–7. doi:10.2469/faj.v51.n4.1918
18. Demirer R, Kutan AM. Does herding behavior exist in Chinese stock markets? J Int Financial markets, institutions money (2006) 16:123–42. doi:10.1016/j.intfin.2005.01.002
19. Lao P, Singh H. Herding behaviour in the Chinese and indian stock markets. J Asian Econ (2011) 22:495–506. doi:10.1016/j.asieco.2011.08.001
20. Chang EC, Cheng JW, Khorana A. An examination of herd behavior in equity markets: An international perspective. J Banking Finance (2000) 24:1651–79. doi:10.1016/s0378-4266(99)00096-5
21. Chiang TC, Zheng D. An empirical analysis of herd behavior in global stock markets. J Banking Finance (2010) 34:1911–21. doi:10.1016/j.jbankfin.2009.12.014
22. Wermers R. Mutual fund herding and the impact on stock prices. J Finance (1999) 54:581–622. doi:10.1111/0022-1082.00118
23. Choi N, Skiba H. Institutional herding in international markets. J Banking Finance (2015) 55:246–59. doi:10.1016/j.jbankfin.2015.02.002
24. Lakonishok J, Shleifer A, Vishny RW. The impact of institutional trading on stock prices. J financial Econ (1992) 32:23–43. doi:10.1016/0304-405x(92)90023-q
25. Hsieh S-F, Chan C-Y, Wang M-C. Retail investor attention and herding behavior. J Empirical Finance (2020) 59:109–32. doi:10.1016/j.jempfin.2020.09.005
26. Li W, Rhee G, Wang SS. Differences in herding: Individual vs. institutional investors. Pacific-Basin Finance J (2017) 45:174–85. doi:10.1016/j.pacfin.2016.11.005
27. Tetlock PC. Giving content to investor sentiment: The role of media in the stock market. J Finance (2007) 62:1139–68. doi:10.1111/j.1540-6261.2007.01232.x
28. Kothari SP, Li X, Short JE. The effect of disclosures by management, analysts, and business press on cost of capital, return volatility, and analyst forecasts: A study using content analysis. Account Rev (2009) 84:1639–70. doi:10.2308/accr.2009.84.5.1639
29. Li F. Textual analysis of corporate disclosures: A survey of the literature. J Account Lit (2010) 29:143–65.
30. Loughran T, McDonald B. When is a liability not a liability? Textual analysis, dictionaries, and 10-ks. J Finance (2011) 66:35–65. doi:10.1111/j.1540-6261.2010.01625.x
31. Henry E, Leone AJ. Measuring qualitative information in capital markets research: Comparison of alternative methodologies to measure disclosure tone. Account Rev (2016) 91:153–78. doi:10.2308/accr-51161
32. Kraus M, Feuerriegel S. Decision support from financial disclosures with deep neural networks and transfer learning. Decis Support Syst (2017) 104:38–48. doi:10.1016/j.dss.2017.10.001
33. Abeysinghe P, Bandara T. A novel self-learning approach to overcome incompatibility on tripadvisor reviews. Data Sci Management (2022) 5:1–10. doi:10.1016/j.dsm.2022.02.001
34. Kim W, Wei S-J. Foreign portfolio investors before and during a crisis. J Int Econ (2002) 56:77–96. doi:10.1016/s0022-1996(01)00109-x
35. Galariotis EC, Rong W, Spyrou SI. Herding on fundamental information: A comparative study. J Banking Finance (2015) 50:589–98. doi:10.1016/j.jbankfin.2014.03.014
36. Zhou RT, Lai RN. Herding and information based trading. J Empirical Finance (2009) 16:388–93. doi:10.1016/j.jempfin.2009.01.004
37. Vlastakis N, Markellos RN. Information demand and stock market volatility. J Banking Finance (2012) 36:1808–21. doi:10.1016/j.jbankfin.2012.02.007
38. Das SR, Chen MY. Yahoo! for amazon: Sentiment extraction from small talk on the web. Management Sci (2007) 53:1375–88. doi:10.1287/mnsc.1070.0704
Keywords: herding, social media, sentiment, support vector machine, COVID-19
Citation: Yoon J and Oh G (2022) Investor herding behavior in social media sentiment. Front. Phys. 10:1023071. doi: 10.3389/fphy.2022.1023071
Received: 19 August 2022; Accepted: 10 October 2022;
Published: 24 October 2022.
Edited by:
Can-Zhong Yao, South China University of Technology, ChinaReviewed by:
Peng-Fei Dai, East China University of Science and Technology, ChinaXiang Gao, Shanghai Business School, China
Copyright © 2022 Yoon and Oh. This is an open-access article distributed under the terms of the Creative Commons Attribution License (CC BY). The use, distribution or reproduction in other forums is permitted, provided the original author(s) and the copyright owner(s) are credited and that the original publication in this journal is cited, in accordance with accepted academic practice. No use, distribution or reproduction is permitted which does not comply with these terms.
*Correspondence: Gabjin Oh, phecogjoh@chosun.ac.kr