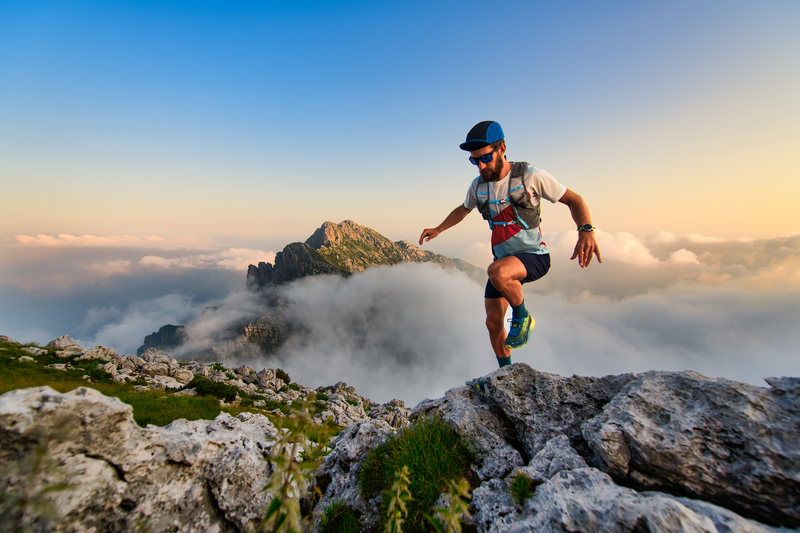
94% of researchers rate our articles as excellent or good
Learn more about the work of our research integrity team to safeguard the quality of each article we publish.
Find out more
ORIGINAL RESEARCH article
Front. Phys. , 02 November 2022
Sec. Social Physics
Volume 10 - 2022 | https://doi.org/10.3389/fphy.2022.1017015
This article is part of the Research Topic Epidemic Models on Networks View all 5 articles
Complex networks are the preferential framework to model spreading dynamics in several real-world complex systems. Complex networks can describe the contacts between infectious individuals, responsible for disease spreading in real-world systems. Understanding how the network structure affects an epidemic outbreak is therefore of great importance to evaluate the vulnerability of a network and optimize disease control. Here we argue that the best network structure indexes (NSIs) to predict the disease spreading extent in real-world networks are based on the notion of network node distance rather than on network connectivity as commonly believed. We numerically simulated, via a type-SIR model, epidemic outbreaks spreading on 50 real-world networks. We then tested which NSIs, among 40, could a priori better predict the disease fate. We found that the “average normalized node closeness” and the “average node distance” are the best predictors of the initial spreading pace, whereas indexes of “topological complexity” of the network, are the best predictors of both the value of the epidemic peak and the final extent of the spreading. Furthermore, most of the commonly used NSIs are not reliable predictors of the disease spreading extent in real-world networks.
The fundamental role of networks in epidemiology has been recognized in the last years [1–12]. The disease spreading can be modeled as a network where nodes (vertices) represent the individuals (i.e., the hosts) and links (edges) indicate the social contacts among them [1–9]. Real-world complex networks display many structural connectivity patterns, such as the heavy-tailed degree distribution, small-world effect, high clustering coefficient, self-similarity, assortativity, community structures, etc. [1, 13–18]. These network structural connectivity patterns may affect the evolution of the spreading process [1, 5, 18–21]. Knowing the relationship between network structure indexes (NSIs) and the spreading dynamics is crucial to prevent and control diseases [17].
The field measures and analyses of real-world complex networks can be extremely consuming, in terms of both money and time. It is therefore necessary to know which features of the network structure should be first measured to assess the network vulnerability to disease and consequently optimize the control [1, 18–21]. To address this issue, we gathered a dataset of 50 real-world complex systems. They represent archetypical examples of network structures in different domains of reality, ranging from social, computers, internet, transportation, biological, and ecological networks (see Supplementary Materials S1.2 for details). We explicitly simulated a disease spreading over them via a classical compartmental susceptible–infected–recovered (SIR) model [1–5].
We derived three indicators of the speed and magnitude of the disease spread: 1) the time steps needed for the disease to strike 15% of the network nodes,
We considered 40 different NSIs, and we tested them, using 4 different regression models, which were the best predictors of the epidemic vulnerability simulated by the SIR model. We considered both classic NSIs from network science literature, graph theory, chemical graph theory, and original NSIs conceived in the present work (See Table 2 in the Methods and Supplemental Material S1.1). Regarding the type of relationship between the 3 disease spread indicators
To select the best, among 40, NSI predictor, and the best, among 4, regression type, we ranked the 40*4 = 160 different models via the Akaike information criterion (AIC). AIC aims to select the model with the best goodness of fit to data while discouraging overparameterization and model complexity [31]. Eventually, for any model, we computed the fraction of variance unexplained (FVU). FVU is a measure of the goodness of fitting of the model, with FVU tending to zero for “ideal” models explaining the entire variability in the observations.
The best results of the model selection procedures and the best model performances are reported in Table 1. The forms and fitting of the best regression models, for different spreading indicators and values of transmissibility, are reported in Figure 1. The spreading indicators vs. NSI scatterplots are in Supplementary Figures S3–S7. All the results of the model selection procedures and performances are in Supplementary Tables S2–S5.
FIGURE 1. The best regression models of the Network Structural indexes (NSI) vs. Spreading Indicators (SI). Left column: the best regression models for SIR parameters β = 0.03 and γ = 0.04. Right column: the best regression models for SIR parameters β = 0.06 and γ = 0.04. Best for
When considering the initial pace of disease (
The node closeness (or closeness centrality) is a measure of centrality in a network, calculated as the reciprocal of the sum of the distances (shortest paths length) between the node and all other nodes in the network [32]. Usually, the node closeness centrality may be normalized by dividing it by the term
When considering the maximum number of concurrently infected nodes (
When considering the overall number of nodes that have been infected during an epidemic (
For high epidemic transmission (β = 0.06) the best predictor is the average node coreness
Our results show that to predict network spreading to consider the distance among nodes is more important than focusing on their connectivity level. The most usual NSI evaluating the connectivity level of the network, i.e. the average node degree
TABLE 2. Network structural indexes (NSI) list. For the NSIs from the literature is indicated the reference between square brackets; for the new NSIs is indicated “new” and the NSI number from they are derived.
This seems counter-intuitive, since higher connectivity levels correlate, on average, with lower node distance in the network [1–13].
Focusing
Both
FIGURE 2. Model network examples of increasing complexity following Bonchev and Buck [16] theory of network complexity. When evaluating the complexity of the network with the rationale of the network structural indexes
In particular, the higher spreading of the star network with respect to the chain network, hence these ideal-types of network show similar network connectivity, they present very different node distance, allows to explain how the network connectivity alone may not be a reliable predictor of the spreading entity, and networks of similar connectivity level may present very different spreading entity. On the other hand, our outcomes show that the magnitude of the de-correlation between connectivity and node distance of the real-world networks may be higher enough to make the network connectivity alone a scarce predictor of the epidemic spreading.
This outcome is particularly important in the context of the epidemic spreading, such as the SARS-Cov2 research. Important and recent research by Thurner and colleagues [11] focusing SIR epidemic spreading on networks showed that classic epidemiological models formulated as differential equations, and based on the mean-field approximation (assuming that every node/individual in principle can infect any other), can produce a misleading prediction of the real epidemic spreading extent. Consequently, Thurner et al. [11] questioned the applicability of standard compartmental models, which neglect the network structure, to describe the real epidemic spreading and the SARS-Cov2 containment phase.
From one side, the outcomes of our research strongly support the Thurner et al. [11] main statement showing how neglecting the network structure may perform erroneous predictions of the real epidemic spreading. On the other side, our results go further and extend the Thurner et al. [11] research outcomes. Here, we show that the network epidemic models investigating the SARS-Cov2 epidemic spreading focus on the network connectivity density as a main structural feature to parameterize network epidemic spreading, as done by Thurner et al. [11] and many recent network epidemic models [6, 8], may perform incomplete or even unrealistic spreading predictions.
Further, most of the non-pharmaceutical interventions (NPIs) implemented to curb the SARS-Cov2 epidemic follow the rationale to reduce social interactions [33, 34], that is to decrease the number of the network links. Our analyses suggest that implementing NPIs with the aim to space out the nodes, i.e., increasing the node distance in the network, would be a more effective strategy to halt the epidemic. This would translate into a reduced peak of infected individuals (
Last, we outline that many of the NSIs conceived in complex network science to encompass important network features that may potentially be leading to differently spreading entities, are not able to perform reliable predictions of the SIR epidemic spreading in real-world networks. The modularity (
On the other hand, our results show that the node distance is the most important factor affecting the network spreading. The aforementioned NSIs may not correlate with node distance, and, as explained above for the relationship between average node degree and node distance (Figure 2), real-world networks with the same value for these NSIs may present different node distance
In Table 2 we list the network structural indexes (NSIs) used in this study, a short definition, and their reference. For the NSIs coming from literature, we indicate the literature reference. For the new ones formulated in the present study by modifying or combining notions or indicators from literature, we list the indicators from which the new ones are derived. In the Supplementary Material S1.1, we furnish the extended definition of each network structural indicator.
We analyzed a set of 50 high-quality real-world networks from different fields of science (see Supplementary Material S1.2). The number of real-world networks for different areas of science is: road transportation 6, airports transportation 2, cargo-ship transportation 1, biological 4, ecological 2, social 13, citation 2, phone 2, internet 5, financial 1, computers 9, email 3. The complete list of real-world networks with network type and reference is in Supplementary Table S1.
We used a susceptible-infected-recovered (SIR) model to numerically simulate the spreading entity over real-world networks. Type SIR models can successfully predict the dynamics of many infectious diseases. See Keeling and Rohani [35] for an overview. When considering SIR models over a network, at any time, a node can be in one of three possible compartments: susceptible (S), infected (I), and recovered (R). If a node/individual is infected, it will infect susceptible nodes linked to it with a transmission rate, β. An infected node/individual stays infectious on average for γ−1 consecutive days, i.e., recovers with a rate equal to γ. Recovered node/individual can no longer infect others and its state will no longer change, which is equivalent to assume that immunization does not vanish in the considered time horizon. We initialized the system by fixing all nodes/individuals as susceptible except one, randomly chosen, whose state is set as infected. The system dynamics can then be solved and permit to model the epidemics evolution over time. To simulate the SIR spreading on a network we used the NDlib Python library presented in Rossetti et al. [36]. We fix the SIR parameters β equals 0.03 or 0.06, and γ = 4. We adopt two different transmission rate values of parameter β to describe low and high epidemic transmission. Higher values of β represent epidemics with higher transmissibility. We chose relatively small values for β, according to Kitsak et al. [5], so that the infected percentage of the population in the network remains small and the simulation can outline the role of the network structure for the spreading. In the case of larger β values, where spreading can reach a large fraction of the population in a few steps, the spreading would cover almost all the network in a few time steps thus hiding the role of topological structure to affect the pace of the spreading. For each real-world network, we implemented 103 independent SIR simulations each with a different node/individual initially infected.
The pace of the epidemic spreading can also be evaluated by the time to infect a given part of the population [37]. We define the time to reach the 15% of infected nodes in the network.
Then, we assessed the pace of the epidemic spreading by the total number of individuals that have been infected (
The list of the spreading indicators with their definition is in Table 3.
To estimate the goodness of the relationship between the spreading indicator value (response variable Y) and the network structural indicator value (independent variable or predictor X) we performed four types of regression models: linear, quadratic, exponential, and monomolecular.
Linear:
Exponential:
Quadratic:
Monomolecular (also known as Brody or Mitscherlich function):
We selected the best model using the Akaike information criterion (AIC) [31].
where k is the number of estimated parameters in the regression model (2 or 3 according to the regression model), and
Eventually, to provide an easily interpretable measure of the goodness of the fitting model performances over network structural indexes (predictors), we computed the fraction of variance unexplained (FVU), calculated as:
where
Publicly available datasets were analyzed in this study. This data can be found here: The datasets analysed during the current study are available in the “Netzschleuder” repository [https://networks.skewed.de/], in the “Stanford Large Network Dataset Collection” repository [https://snap.stanford.edu/data/index.html], and in “The Colorado Index of Complex Networks (ICON)” repository [https://icon.colorado.edu/#!/].
BM, CD, AR, and BD conceived the research. BM, AR, and TM performed the analyses. All the authors wrote the manuscript.
This research is funded by a grant from the Italian Ministry of Foreign Affairs and International Cooperation. This project has received funding from the European Research Council (ERC) under the European Union’s Horizon 2020 research and innovation programme [grant agreement No. (816313)]. This work is supported by the Vietnam’s Ministry of Science and Technology (MOST) under the Vietnam-Italy scientific and technological cooperation program for the period 2021–2023. This work is supported by the Vietnam National University Ho Chi Minh City (VNU-HCM), Ho Chi Minh city, Vietnam under grant number B2018-42-01.
BM, TM, CD, and AR acknowledge the Italian Ministry of Foreign Affairs and International Cooperation. We are greatly thankful to Van Lang University, Vietnam for providing the budget for this study. We thank F. Sartori for helpful discussions.
The authors declare that the research was conducted in the absence of any commercial or financial relationships that could be construed as a potential conflict of interest.
All claims expressed in this article are solely those of the authors and do not necessarily represent those of their affiliated organizations, or those of the publisher, the editors and the reviewers. Any product that may be evaluated in this article, or claim that may be made by its manufacturer, is not guaranteed or endorsed by the publisher.
The Supplementary Material for this article can be found online at: https://www.frontiersin.org/articles/10.3389/fphy.2022.1017015/full#supplementary-material
1. Pastor-Satorras R, Castellano C, Van Mieghem P, Vespignani A. Epidemic processes in complex networks. Rev Mod Phys (2015) 87:925–79. doi:10.1103/RevModPhys.87.925
2. Pastor-Satorras R, Vespignani A. Immunization of complex networks. Phys Rev E (2002) 65:036104. doi:10.1103/PhysRevE.65.036104
3. Newman M. Spread of epidemic disease on networks. Phys Rev E (2002) 66:016128. doi:10.1103/PhysRevE.66.016128
4. Chen Y, Paul G, Havlin S, Liljeros F, Stanley H. Finding a better immunization strategy. Phys Rev Lett (2008) 101:058701. doi:10.1103/PhysRevLett.101.058701
5. Kitsak M, Gallos LK, Havlin S, Liljeros F, Muchnik L, Stanley HE, et al. Identification of influential spreaders in complex networks. Nat Phys (2010) 6:888–93. doi:10.1038/nphys1746
6. Firth JA, Hellewell J, Klepac P, Kissler S, Jit M, Atkins KE, et al. Using a real-world network to model localized COVID-19 control strategies. Nat Med (2020) 26:1616–22. doi:10.1038/s41591-020-1036-8
7. Amaral MA, Oliveira MMd., Javarone MA. An epidemiological model with voluntary quarantine strategies governed by evolutionary game dynamics. Chaos Solitons Fractals (2021) 143:110616. doi:10.1016/j.chaos.2020.110616
8. Nishi A, Dewey G, Endo A, Neman S, Iwamoto SK, Ni MY, et al. Network interventions for managing the COVID-19 pandemic and sustaining economy. Proc Natl Acad Sci U S A (2020) 117:30285–94. doi:10.1073/pnas.2014297117
9. Hu Y, Ji S, Jin Y, Feng L, Eugene Stanley H, Havlin S. Local structure can identify and quantify influential global spreaders in large scale social networks. Proc Natl Acad Sci U S A (2018) 115:7468–72. doi:10.1073/pnas.1710547115
10. Pei S, Makse HA. Spreading dynamics in complex networks. J Stat Mech (2013) 2013:P12002. doi:10.1088/1742-5468/2013/12/P12002
11. Thurner S, Klimek P, Hanel R. A network-based explanation of why most COVID-19 infection curves are linear. Proc Natl Acad Sci U S A (2020) 117:22684–9. doi:10.1073/pnas.2010398117
12. Bellingeri M, Turchetto M, Bevacqua D, Scotognella F, Alfieri R, Nguyen Q, et al. Modeling the consequences of social distancing over epidemics spreading in complex social networks: From link removal analysis to SARS-CoV-2 prevention. Front Phys (2021) 9:1–7. doi:10.3389/fphy.2021.681343
13. Boccaletti S, Vito L, Moreno Y, Chavez M, Hwang D. Complex networks: Structure and dynamics. Phys Rep (2006) 424:175–308. doi:10.1016/j.physrep.2005.10.009
14. Buckley F, Harary F. Distance in graphs. Redwood City, CA: Addison-Wesley Publishing Company (1990). doi:10.1201/b16132-64
15. Bonchev D, Buck GA. Quantitative measures of network complexity. Complex Chem Biol Ecol (2005) 2005:191–235. doi:10.1007/0-387-25871-X_5
16. De Domenico M, Granell C, Porter MA, Arenas A. The physics of spreading processes in multilayer networks. Nat Phys (2016) 12:901–6. doi:10.1038/nphys3865
17. Volz EM, Miller JC, Galvani A, Meyers L. Effects of heterogeneous and clustered contact patterns on infectious disease dynamics. Plos Comput Biol (2011) 7:e1002042. doi:10.1371/journal.pcbi.1002042
18. Noldus R, Mieghem PV. Assortativity in complex networks. J Complex Netw (2015) 3:507–42. doi:10.1093/comnet/cnv005
19. Miller JC. Spread of infectious disease through clustered populations. J R Soc Interf (2009) 6:1121–34. doi:10.1098/rsif.2008.0524
20. Salathe M, James J. Dynamics and control of diseases in networks with community structure. Plos Comput Biol (2010) 6:e1000736. doi:10.1371/journal.pcbi.1000736
21. Badham J, Stocker R. The impact of network clustering and assortativity on epidemic behaviour. Theor Popul Biol (2010) 77:71–5. doi:10.1016/j.tpb.2009.11.003
22. Bellingeri M, Vincenzi S. Robustness of empirical food webs with varying consumer’s sensitivities to loss of resources. J Theor Biol (2013) 333:18–26. doi:10.1016/j.jtbi.2013.04.033
24. Estrada E. Quantifying network heterogeneity. Phys Rev E (2010) 82:066102–8. doi:10.1103/PhysRevE.82.066102
25. Spellerberg IF, Fedor P. A tribute to Claude Shannon (1916–2001) and a plea for more rigorous use of species richness, species diversity and the ‘Shannon–Wiener’ Index. Glob Ecol Biogeogr (2003) 12:177–9. doi:10.1046/j.1466-822x.2003.00015.x
26. Rouvray D. The rich legacy of half a century of the wiener index. Topology Chem (2002) 2002:16–37. doi:10.1533/9780857099617.16
27. Latora V, Marchiori M. Efficient behavior of small-world networks. Phys Rev Lett (2001) 87:198701. doi:10.1103/PhysRevLett.87.198701
28. Estrada E, Hatano N. Communicability in complex networks. Phys Rev E (2008) 77:036111. doi:10.1103/physreve.77.036111
29. Freeman HE. A set of measures of centrality based on betweenness. Sociometry (1977) 40:35–41. doi:10.2307/3033543
30. Clauset C, Newman MJ, Moore C. Finding community structure in very large networks. Phys Rev E (2004) 70:066111. doi:10.1103/physreve.70.066111
31. Burnham KP, Anderson DR. Multimodel inference: Understanding AIC and BIC in model selection. Sociol Methods Res (2004) 33:261–304. doi:10.1177/0049124104268644
32. Marchiori M, Latora V. Harmony in the small-world. Physica A: Stat Mech its Appl (2000) 285:539–46. doi:10.1016/s0378-4371(00)00311-3
33. Flaxman S, Mishra S, Gandy A, Unwin HJT, Mellan TA, Coupland H, et al. Estimating the effects of non-pharmaceutical interventions on COVID-19 in Europe. Nature (2020) 584:257–61. doi:10.1038/s41586-020-2405-7
34. Perra N. Non-pharmaceutical interventions during the COVID-19 pandemic: A review. Phys Rep (2021) 913:1–52. doi:10.1016/j.physrep.2021.02.001
35. Matt K, Pejman R. Modeling infectious diseases in humans and animals. New Jersey, United States: Princeton University Press (2008).
36. Rossetti G, Milli L, Rinzivillo S, Sîrbu A, Pedreschi D, Giannotti F. NDlib: A python library to model and analyze diffusion processes over complex networks. Int J Data Sci Anal (2018) 5:61–79. doi:10.1007/s41060-017-0086-6
37. Chen D, Lü L, Shang MS, Zhang YC, Zhou T. Identifying influential nodes in complex networks. Physica A: Stat Mech its Appl (2012) 391:1777–87. doi:10.1016/j.physa.2011.09.017
Keywords: complex networks, network spreading, network epidemics, network structural characteristics, SIR (susceptible infected recovered) model
Citation: Bellingeri M, Bevacqua D, Turchetto M, Scotognella F, Alfieri R, Nguyen N-K-K, Le TT, Nguyen Q and Cassi D (2022) Network structure indexes to forecast epidemic spreading in real-world complex networks. Front. Phys. 10:1017015. doi: 10.3389/fphy.2022.1017015
Received: 11 August 2022; Accepted: 19 October 2022;
Published: 02 November 2022.
Edited by:
Ayse Peker-Dobie, Istanbul Technical University, TurkeyReviewed by:
Divya Sindhu Lekha, Indian Institute of Information Technology, Kottayam, IndiaCopyright © 2022 Bellingeri, Bevacqua, Turchetto, Scotognella, Alfieri, Nguyen, Le, Nguyen and Cassi. This is an open-access article distributed under the terms of the Creative Commons Attribution License (CC BY). The use, distribution or reproduction in other forums is permitted, provided the original author(s) and the copyright owner(s) are credited and that the original publication in this journal is cited, in accordance with accepted academic practice. No use, distribution or reproduction is permitted which does not comply with these terms.
*Correspondence: Michele Bellingeri, bWljaGVsZS5iZWxsaW5nZXJpQHBvbGltaS5pdA==
Disclaimer: All claims expressed in this article are solely those of the authors and do not necessarily represent those of their affiliated organizations, or those of the publisher, the editors and the reviewers. Any product that may be evaluated in this article or claim that may be made by its manufacturer is not guaranteed or endorsed by the publisher.
Research integrity at Frontiers
Learn more about the work of our research integrity team to safeguard the quality of each article we publish.