- 1Shanghai Engineering Research Center of Molecular Therapeutics & New Drug Development, School of Chemistry and Molecular Engineering, East China Normal University, Shanghai, China
- 2Institute of Food Science and Engineering, School of Medical Instrument and Food Engineering, University of Shanghai for Science and Technology, Shanghai, China
- 3School of Food and Biological Engineering, Zhengzhou University of Light Industry, Zhengzhou, China
- 4NYU-ECNU Center for Computational Chemistry at NYU Shanghai, Shanghai, China
- 5Department of Chemistry, New York University, New York, NY, United States
Enzyme thermostability is an important parameter for estimating its industrial value. However, most naturally produced enzymes are incapable of meeting the industrial thermostability requirements. Software programs can be utilized to predict protein thermostability. Despite the fast-growing number of programs designed for this purpose; few provide reliable applicability because they do not account for thermodynamic weaknesses. Aspartic proteases are widely used in industrial processing; however, their thermostability is not able to meet the large-scale production requirements. In this study, through analyzing structural characteristics and modifying thermostability using prediction software programs, we improved the thermostability of pepsin, a representative aspartic protease. Based on the structural characteristics of pepsin and the experimental results of mutations predicted by several energy-based prediction software programs, it was found that the majority of pepsin’s thermodynamic weaknesses lie on its flexible regions on the surface. Using computational design, mutations were made based on the predicted sites of thermodynamic weakness. As a result, the half-lives of mutants D52N and S129A at 70°C were increased by 200.0 and 66.3%, respectively. Our work demonstrated that in the effort of improving protein thermostability, identification of structural weaknesses with the help of computational design, could efficiently improve the accuracy of protein rational design.
Introduction
Enzymes, as catalytic biological macromolecules, have the advantages of high efficiency and low pollution. They are competitive alternatives to chemical catalysts and are widely used in food, medicine, biofuel, and other industries [1–3]. Industrial processes usually need to be performed in extreme environments. For example, processing at high temperatures accelerates reactions, increases substrate utilization, and reduces the risk of microbial contamination [4]. However, high temperatures can easily destroy the enzyme structure, resulting in a loss of its catalytic activity. Therefore, thermostability is an important parameter for measuring the industrial value of enzymes. Traditional methods for enzyme thermostability modification include directed evolution, ancestral stabilization, and site-directed mutagenesis based on structure prediction. However, owing to their unpredictability and long-range effects, mutants with improved thermostability are not readily obtained [5, 6]. With the development of structural and computational biology, protein structures can be obtained efficiently through experimental determination and computational prediction [7–10]. In recent years, based on protein structure and energy function, the prediction of mutations stabilizing the folded protein structure has improved considerably. Several methods based on structures, sequences, and other characteristics have been developed to predict protein stability, including machine learning, and physical, statistical, and empirical potentials [11–16]. These methods provide a clear ranking of mutations, but are not widely used in the design of enzymes for enhanced thermostability. This is primarily due to the optimization of prediction software programs based on a specific potential or structural feature, i.e., if these characteristics are not the weakest points responsible for poor stability, thermostability improvement due to mutagenesis may not be significant, and may result in the destruction of protein structure and lead to contradictory results. Therefore, it is necessary to analyze the structural characteristics of enzymes and consider thermodynamic structural weaknesses as a guide for rapid screening. Here, we selected an important enzyme, pepsin, to conduct a systematic test of conventional stability prediction software programs.
Aspartic proteases are a large class of proteases widely used in food, fermentation, pharmaceutical, and other industries [17, 18]. However, the poor thermostability of most natural aspartic proteases hinders their large-scale industrial applications, making the improvement of their thermostability crucial. Traditional methods, such as site-directed mutagenesis based on comparison of the amino acid sequence with a more thermostable, homologous counterpart, have achieved good results in the modification of other proteases [19–21]. However, these methods are not suitable for aspartic proteases due to their low sequence identity across different species [22]. Improving thermostability by enhancing resistance to autocatalysis was successful in aspartic protease, but the result of single point mutation increased by only 53.0% [23]. Therefore, the feasibility of improving the thermostability of aspartic proteases using traditional methods is fairly low. Aspartic proteases have the advantages of having a clear structure and catalytic mechanism, making it more suitable to adopt a computational design strategy. However, few studies have been conducted on improving the thermostability of aspartic proteases using prediction software programs. This is primarily owing to a lack of a thermodynamic structural weakness guide, as it is difficult to predict mutations with improved thermostability quickly and accurately by relying solely on the energy function.
In this study, we first performed an in-depth analysis of the structural characteristics of pepsin, a typical aspartic protease, and predicted possible thermodynamic weaknesses. By systematically testing a variety of software programs, we found that mutations with good prediction scores were not associated with thermodynamic weakness and performed poorly during experimental verification. The major structural area of weakness in pepsin was determined to be the flexible regions on its surface. Several mutations were identified based on this structural weakness. Among them, two excellent mutants, D52N and S129A, were obtained, and their half-lives at 70°C were found to increase by 200.0 and 66.3%, respectively. Our analysis showed that the guide of thermodynamic structural weakness promotes fast and accurate thermostability design using prediction software programs.
Materials and Methods
Strains, Reagents, and Chemicals
The pPIC9K plasmid and Pichia pastoris GS115 (Invitrogen, Carlsbad, CA, United States) were used for subcloning and overexpression. Escherichia coli DH5α (Weidi Biotechnology Co., Ltd., Shanghai, China) was used for gene cloning and DNA sequencing. Plasmid pPIC9K-pepsinogen was previously constructed and stored in our laboratory. Primers for mutation construction were synthesized by Ruimian Biotech Co., Ltd. (Shanghai, China). Plasmid Extraction and Gel/PCR Purification Kits were purchased from TOROIVD (BVI, United Kingdom). The Seamless Cloning Kit was purchased from Beyotime Biotechnology (Shanghai, China). All enzymes used in the experiments were supplied by Thermo Scientific (Waltham, MA, United States). The bovine hemoglobin substrate was purchased from Maokang Biotech Co., Ltd. (Shanghai, China). All other chemicals were of analytical grade and are commercially available.
Site-Directed Mutagenesis
Site-directed mutagenesis was performed using the Seamless Cloning Kit according to the manufacturer’s instructions, with the plasmid pPIC9K-pepsinogen (with a C-terminal His-tag) as the template. The primers used for mutagenesis are listed in Supplementary Table S1. Subsequently, the seamless cloning products were transformed into E. coli DH5α cells. Recombinant plasmid construction was confirmed by DNA sequencing. They were then linearized with SalI, and transformed into P. pastoris GS115 competent cells through electroporation. Positive clones were screened using minimal dextrose (MD) plates, their activity was demonstrated using skim milk plates, and confirmed by DNA sequencing.
Heterologous Expression and Purification
Positive clones were fermented as previously described [24]. Cultures were centrifuged at 8000 ×g for 10 min at 4°C and crudely purified by the slow addition of ammonium sulfate until 80% saturation was achieved. After overnight dialysis against TE buffer (20 mM Tris-HCl, 500 mM NaCl, pH 7.5) at 4°C, the crude samples were purified using a HisTrap HP affinity column (General Electric Company, Boston, MA, United States). The fractions containing pepsinogen were pooled and dialyzed against the TE buffer, then activated by adding 0.9 M HCl to adjust the pH to ∼2 and subsequently incubated at 25°C for 10 min. The prosegment was removed using Centricon filtration units with a 10 kDa molecular mass cutoff (Millipore, Boston, MA, United States). All samples were stored in 20 mM sodium acetate (pH 5.3) and analyzed using 12% sodium dodecyl sulfate-polyacrylamide gel electrophoresis (SDS-PAGE, Coomassie Brilliant Blue R250-stained). Finally, the concentration of recombinant pepsin was measured using a BCA protein quantificationkit (Yeasen Biotech Co., Ltd., Shanghai, China).
Enzymatic Activity and Kinetic Assays
The proteolytic activities of pepsin and its variants were determined by measuring the amount of exposed tyrosine residues during hydrolysis in 100 mM sodium citrate buffer (pH 2.0), with 1% bovine hemoglobin as the substrate. A mixture containing 0.5 ml of 1% bovine hemoglobin and 0.5 ml of appropriately diluted recombinant proteins was incubated at 60°C for 10 min. The reactions were terminated by adding 1.0 ml of 0.4 M trichloroacetic acid (TCA). Exposed tyrosine residues were quantified by incubating reactions containing 0.5 ml of the filtered supernatant, 2.5 ml of 0.4 M sodium carbonate solution, and 0.5 ml of Folin’s phenol reagent at 40°C for 20 min and then measuring absorbance at 680 nm. Control samples were treated in a similar manner, but TCA was added before adding the enzyme.
The initial reaction rates of recombinant proteins were also determined at 60°C and a pH of 2.0, using 0.5–10.0 mg ml−1 bovine hemoglobin as the substrate. Kinetic parameters (Km, kcat, and Vmax) were obtained by fitting the initial data to the Michaelis–Menten nonlinear regression equation in the Origin 9.0 software (Origin Lab Corporation).
Half-Life Analysis
To evaluate thermostability, the half-lives (t1/2) of pepsin and its mutants were measured at 65°C and 70°C. Recombinant proteins were appropriately diluted with 100 mM sodium citrate buffer (pH 2.0) and pre-incubated at 65°C and 70°C. Samples were taken at regular intervals, and the residual activities measured at 60°C and pH 2.0, as described in Enzymatic Activity and Kinetic Assays. Initial activity, before pre-incubation, was set to 100%.
Molecular Dynamics Simulation
The crystal structure of pepsin was obtained from the Protein Data Bank (PDB: 4PEP), and all the crystal waters were removed. The structures of the mutants were prepared using Discovery Studio 2019. The Leap module of AMBER18 was used to fill the missing atoms. The parameters were generated using the AMBER14SB force field. The protein was placed in a TIP3P water box with 12 Å buffer, and counter ions were added to neutralize the system. The steepest descent and conjugate gradient methods were used to optimize the structure. The entire system was subsequently heated to 310 K and the protein was constrained to 10 kcal/(mol Å2). The SHAKE algorithm was employed to constrain chemical bonds involving hydrogen atoms [25]. Finally, simulations were run for 100 ns.
Statistical Analyses
All statistical analyses were performed using Origin 9.0 software, with values expressed as the mean ± standard error of the mean. A one-sample Student’s t-test was used to compare the mutant and wild-type means. Statistical significance was set at p < 0.05. For comparisons between the wild-type and mutants, *represents p < 0.05, **represents p < 0.01, and ***represents p < 0.001.
Results
Analysis of Catalytic Mechanism, Structural Characteristics, and Stability Weaknesses of Pepsin
Members of the aspartic protease family have a similar catalytic mechanism. A nucleophile is formed from a water general-base activated by Asp215, which subsequently attacks the carbonyl oxygen of the scissile bond to form a gem diol of a tetrahedral intermediate with Asp32. The proton transfer from Asp215 to the leaving group is coupled with the regeneration of the proton of Asp32 [26]. Finally, the peptide bond is broken. Thus, the catalytic dyad composed of Asp32 and Asp215 is crucial for the catalysis of pepsin. Two key points need to be avoided in the thermostability design.
Many aspartic proteases have been reported to have similar three-dimensional structures. For simplicity, the pepsin structure can be divided into three regions: N-lobe, C-lobe, and connecting domain (Figure 1). The N-lobe is made up of a series of ß-strands that are folded into three layers of orthogonal packing: sheets IV, VI, andII.The C-lobe has two orthogonal packing sheets, VII and III, and a rather separate sheet V [27]. Several a-helices and loops are also present in both lobes. The connecting domain is comprised mainly of a six-stranded antiparallel interdomain ß-sheet connecting the N-lobe and the C-lobe to form the catalytic dyad.
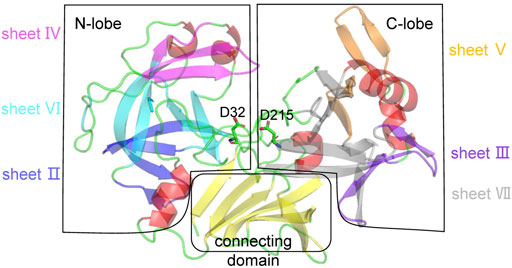
FIGURE 1. Structural analysis of pepsin. Pepsin is divided into three regions: N-lobe, C-lobe, and connecting domain. In the N-lobe, ß-strands can be divided into three layers: sheets IV (magenta), VI (cyan), and II (blue) that are orthogonally packed. In the C-lobe, ß-strands can be divided into two orthogonal packing sheets, VII (grey) and III (purpleblue), and a rather separate sheet V (orange). The connecting domain is composed of a six-stranded antiparallel interdomain ß-sheet (yellow). Other secondary structures, a-helices and loops are shown in red and green. The catalytic dyad is represented by sticks.
Structural analysis revealed that the three ß-sheets in the N-lobe form a continuous hydrophobic region, and the a-helix near the connecting domain and adjacent loops cap the hydrophobic core, further stabilizing the structure. Similarly, a hydrophobic core is formed in the C-lobe, and the connecting domain simultaneously interacts internally with the N- and C- lobes [27]. The loops in pepsin are mainly ß-turns or ß-hairpins that connect ß-strands. We speculated that pepsin may possess two thermodynamic structural weaknesses: the relatively loose internal hydrophobic packing and the high flexibility of the surface a-helices and loops that are not part of the hydrophobic cores. Therefore, there are two strategies for improving the thermostability of pepsin: 1) designing ß-strands that directly improve the interaction between ß-sheets or ß-strands, and 2) designing a-helices or loops on the surface to reduce local disorder.
Selection of Thermostability Predicting Software Programs and Mutation Screening Principles
To investigate correlations between computational design software programs and structural characteristics, we selected several thermostability prediction software programs with successful cases and with varying principles. PoPMuSiC is a web server that predicts folding free energy changes (
We chose the above-mentioned representative and unique software programs. By analyzing the successful cases of these software programs, mutations were found in a-helices, ß-strands, and loops distributed in the interior and surface of proteins, thus meeting the requirements of our analysis of thermodynamic weaknesses. Among these software programs, PoPMuSiC, HoTMuSiC, ETSS, and DeepDDG provided mutation rankings. Based on the highest-ranking batch and the principle of scattered mutation points, we selected four mutations predicted by each software program for follow-up experimental verification. The top 20 mutations predicted by PoPMuSiC, HoTMuSiC, ETSS, and DeepDDG are shown in Supplementary Tables S2–S5 to assist with explaining the screening principles. The number of mutations given by Fireprot was limited; thus all mutations were selected for further experiments.
Thermostability Detection of Mutations Designed Based on Energy From Multiple Software Programs
Based on the screening described above, 34 single-site mutants were constructed and transformed into P. pastoris for expression, using the pPIC9K-pepsinogen plasmid as the template. The mutants predicted based on energy (from PoPMuSiC, HoTMuSiC, ETSS, DeepDDG, and Fireprot-Energy) were tested as a set. To rapidly screen the mutants, we used crude enzyme to measure thermostability. One unit of enzyme activity was defined as the amount of enzyme required to hydrolyze bovine hemoglobin toproduce 1 μg tyrosine per minute at 60°C and pH 2.0. HCl (0.9 M) was added to the fermentation broth supernatants and the pH was adjusted to 2.0, to convert pepsinogen into pepsin. The acidified fermentation broths were incubated at 65°C for 3 min and then cooled on ice. Changes in enzyme activity before and after incubation were measured to reflect the thermostability of recombinant proteins. Enzyme activity before incubation was plotted at 100%. The results are shown in Figure 2A. Apart from the mutations selected by energy-based Fireprot, some mutants predicted by PoPMuSiC, HoTMuSiC, ETSS, and DeepDDG resulted in complete loss of enzyme activity before incubation. These occurred mainly at Gly85, Asp87, Gly102, and Arg315. The remaining mutants did not improve pepsin thermostability. These results indicate that the energy-based mutants were not associated with the thermodynamic weakness of pepsin, and some were located on critical pepsin residues, resulting in the collapse of the hydrophobic cores.
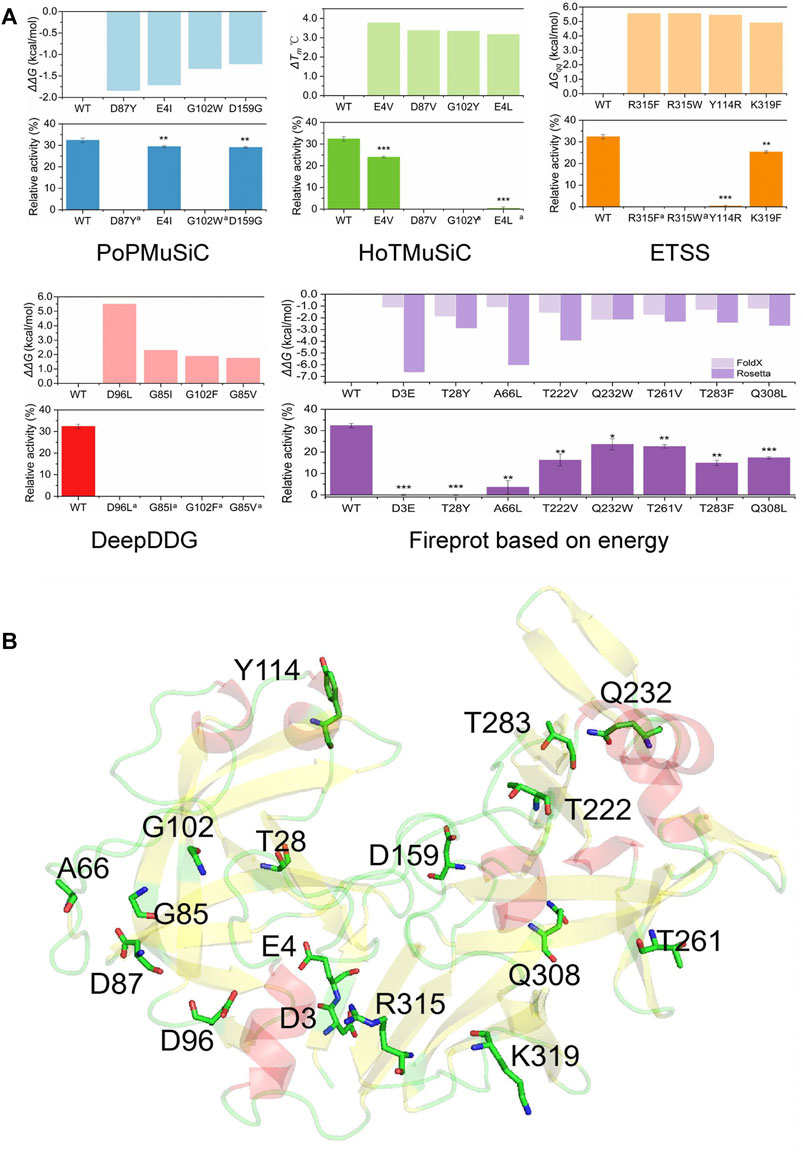
FIGURE 2. Thermostability results and analyses of positions of the set of mutations predicted using various energy-based software programs. (A) Software programs’ parameters and relative activities of single-point mutations after incubation at 65°C for 3 min. Data are mean ± s.e.m. ***p < 0.001, **p < 0.01, *p < 0.05. Data are from three independent experiments. (B) Positions of mutations on the pepsin structure. a indicates that the activity of the original crude enzyme is too low to be measured.
To guide the follow-up design, we analyzed the positions of the above mutations to confirm the structural weakness of pepsin. As shown in Figure 2B, Ala66 and Asp159 are on loops, Tyr114 and Gln232 are on a-helices, and the remaining mutation sites are on ß-strands. Thr28, Gly85, Asp87, Asp96, and Gly102 are located on the three ß-sheets of the N-lobe, which are stacked orthogonally to form hydrophobic cores; Asp3, Glu4, Arg315, and Lys319 are located on the closely packed ß-sheet in the connecting domain; Thr222, Thr261, Thr283, and Tyr309 are located in the orthogonally packed ß-sheets in the C-lobe. The a-helices where Tyr114 and Gln232 are located are also close to hydrophobic cores; the Gln232 side chain is oriented towards the protein, and Tyr114 is an aromatic amino acid. Thus, most of the mutations are located on or near hydrophobic cores, and changes in hydrophobic cores can easily cause enzyme inactivation [37]. The results showed that pepsin’s hydrophobic cores were tightly packed, and were not the major thermodynamic structural weakness of the enzyme. Mutations in the compact region can have long-range effects. Therefore, when further strengthening the internal packing, mutations in the ß-strands and a-helices involving hydrophobic cores lead to enzyme inactivation. Although the computational design results showed that the overall energy of these mutants was greatly reduced, the probability of protein structure destruction was very high. Thus, mutations on ß-strands or inside a-helices are not suitable and mutations guided by the real structural weaknesses—flexible regions on the surface of pepsin—need to be selected.
Detection of Thermostable Mutants Predicted by Fireprot Based on Evolution
Fireprot also identified 10 mutations based on evolution (Table 1). As mentioned above, mutations on tightly packed sheets and a-helices are less feasible, and surface a-helices and loops are the main thermodynamic weakness points of pepsin. Thus, we initially analyzed the positions of these 10 mutations. As shown in Figure 3A, E65Q is on the orthogonally packed ß-sheet of the N-lobe, L167F is on the tightly packed ß-sheet of the connecting domain, D200N is on the ß-turn of the C-lobe, L140M and W141M are on the a-helix, which is part of the hydrophobic core, and the other five mutations are on the loops or a-helices on the surface of pepsin. Thus, A24P, D52N, Q55R, S129A, and S270P mutants were set as the experimental group, and E65Q, L140M, W141M, L167F, and D200N were set as the control group. As described above, we measured the thermostability of the crude enzymes, the results of which are shown in Figure 3B. The wild-type pepsin retained 32.4% of its original activity after incubation for 3 min at 65°C. Among the mutants in the experimental group, the thermostabilities of A24P, D52N, E65Q, and S129A were significantly improved, with D52N and S129A retaining 75.4 and 63.5% of their initial activity, respectively. Only S270P showed a slight decrease in thermostability. The thermostability of mutants in the control group decreased, particularly L140M and W141M. The results indicate that the above strategy of being guided by the thermodynamic weakness of pepsin can rapidly and effortlessly guide mutant design.
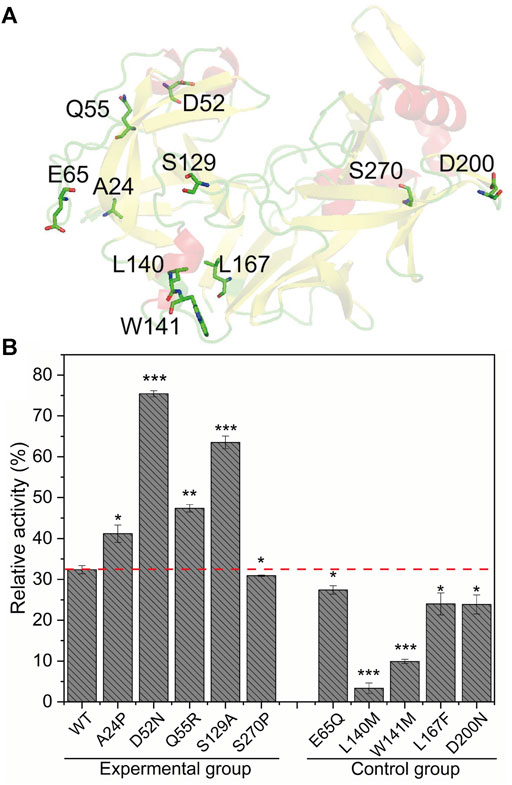
FIGURE 3. Analysis of the positions and thermostability results of mutations predicted using Fireprot based on evolution. (A) Positions of mutations on the pepsin structure. (B) Relative activities of mutants after incubation at 65°C for 3 min. Data are mean ± s.e.m. ***p < 0.001, **p < 0.01, *p < 0.05. Data are from three independent experiments.
Mutants A24P, D52N, E65Q, and S129A, which had improved thermostability, were purified (Supplementary Figure S1). The thick band around 35 kDa is unglycosylated pepsin, and the fuzzy fine band above it is O-glycosylated pepsin, both of which are consistent with previous reports. Glycosylation is used to enhance the thermostability of proteins, and Yoshimasu et al. confirmed that the thermostability of O-glycosylated pepsin was improved [24]. Therefore, it was retained in the experiment. The half-lives (t1/2) at 65°C and 70°C were measured and 1% bovine hemoglobin was used as the substrate to determine changes in thermostability (Table 2). The t1/2 values of A24P and Q55R at 65°C and 70°C were slightly improved. The t1/2 of S129A at 65°C and 70°C increased by 55.6 and 66.3%, respectively, while that of D52N at 65°C and 70°C increased by 308.1 and 200.0%, respectively.
The activities of the mutants D52N and S129A were determined at pH 2.0 and 60°C, using 1% bovine hemoglobin as the substrate. The kinetic parameters were also measured under these conditions using gradient concentrations of bovine hemoglobin (Supplementary Figure S3). As shown in Table 3, compared with the wild-type, the activities of the D52N and S129A mutants decreased by 31.8 and 23.3%, respectively. The Km values of D52N and S129A were 2.12 ± 0.05 and 1.40 ± 0.09 mg ml−1, 130.4 and 52.2% lower than that of the wild-type, respectively. Thus, although kcat values improved, the kcat and kcat/Km values of D52N and S129A mutants decreased by 37.0 and 27.0%, respectively.
Structural and Mechanistic Analysis of Mutations
Dynamic simulation analysis was performed to explore the mechanism of the effect of mutations on the thermostability of pepsin and its mutants. Single-point mutations D52N and S129A were introduced into the crystal structure of pepsin using Discovery Studio 2019, and a stable structure was obtained through a 100 ns MD simulation. The root mean squared deviations (RMSDs) of the protein backbones are shown in Supplementary Figure S2. The RMSD of the wild-type and the D52N and S129A substituted structures were approximately 2.5 Å, 1.5 Å, and 1.5 Å, respectively, indicating that the three systemsare stable, and that D52N and S129A are more stable than the wild-type. A snapshot was extracted from each of the three stable structures for structural analysis (Figure 4). The D52N substitution was located on the a-helix on the protein surface, and formed hydrogen bonds with Leu48 and Ala49, making the a-helix more stable and improving structural rigidity. The S129A substitution was located in a loop on the protein surface. The replacement of Ser with Ala promoted the hydrophobic packing of Pro126, Gly132, Ala133, and Pro135, making this region more stable.
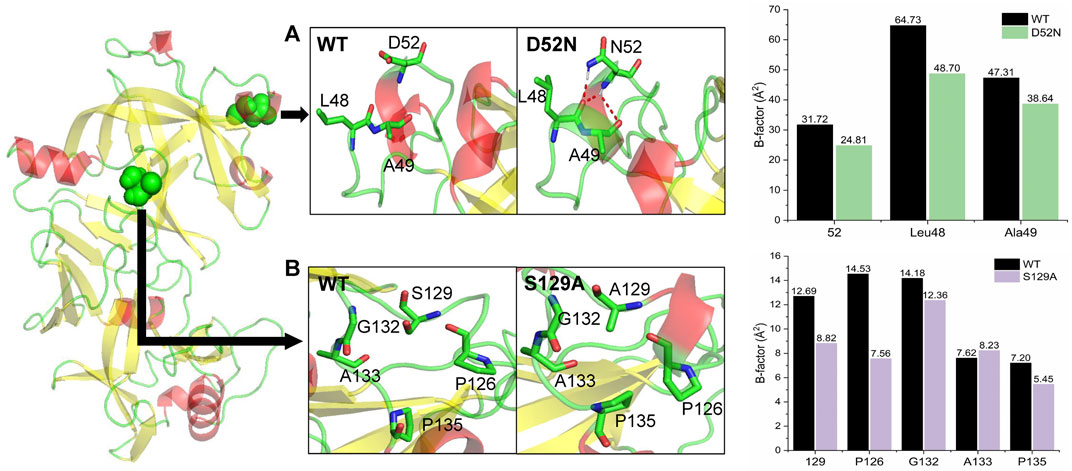
FIGURE 4. Conformational analysis of wild-type (WT) and mutant pepsin. (A) Mutant D52N formed new hydrogen bonds with Leu48 and Ala49. The B-factors of these residues decreased compared with the wild-type. (B) Mutant S129A promoted hydrophobic packing in the loop. The B-factors of most these residues decreased compared with the wild-type.
Furthermore, isotropic temperature factors (B-factors) of the three systems in the last 10 ns of the simulation were calculated to measure the residue fluctuations relative to their mean positions. As shown in Figure 4, we found that the D52N substitution reduced the flexibility of position 52, and the flexibility of Leu48 and Ala49 also decreased, but to varying degrees. After S129A substitution, the flexibility of position 129 decreased, and the flexibility of Pro126, Gly132, and Pro135 of the residues forming the hydrophobic packing decreased by different degrees, with the biggest decrease being noted in Pro126 and Pro135.
Discussion
Numerous breakthroughs have recently been made in the study of enzyme thermostability. The structural rigidity of proteins has been associated with their thermostability through the comparison of mesophilic and thermophilic variants [38]. Protein engineering, which includes the introduction of hydrogen bonds, salt bridges, and disulfide bonds to enhance local rigidity and improve enzyme thermostability, further confirmed this finding [39–41]. Rigidifying flexible sites is a common strategy that seems to be similar to that employed in our study, which involved improving the thermostability of proteins by analyzing the flexible regions of proteins and then rigidifying these sites. However, this strategy is associated with some difficulties when it comes to determining the flexible regions, for example, the crystal structure does not perfectly reflect the flexibility of the protein in the solution, the molecular dynamics is complex and time-consuming, and choosing possible positions to be rigidified [42]. Therefore, as a non-regular secondary structure with high flexibility, loops are often chosen as potential sites for rigidification. Rigidifying loops combined with saturation mutagenesis, proline substitutions, and
The conformational fluctuation of a protein increases when it is exposed to high temperatures. High fluctuation yields reversible or irreversible protein unfolding, leading to loss of function [48]. Therefore, regions that have fewer contacts with the surrounding residues fluctuate significantly and can easily trigger protein unfolding. This is why loops are often chosen as mutation sites [45]. However, the results of agarase AgWH50C from Agarivorans gilvus WH0801 and glucose oxidase from Aspergillus niger showed that modification of other secondary structures within the protein can significantly enhance thermostability, and modification of loops is not the only option [33, 49]. Therefore, based on its high-resolution crystal structure, pepsin is divided into hydrophobic cores and surface covering areas. At high temperatures, the solvation of hydrophobic cores caused by surface fluctuations and the collapse of hydrophobic cores can result in thermodynamic weaknesses. The surface flexible regions were confirmed as the major points of thermodynamic weakness and subsequently used in computational design. The results also showed that mutants on the surface flexible regions enhanced the thermostability of pepsin by reducing local fluctuation. We also found that the enzyme’s reaction temperature can reflect its structural rigidity, to a certain extent, providing some guidance for follow-up mutation design. Pepsin is mainly composed of orthogonally packed antiparallel ß-sheets, which are stable enough to form hydrophobic cores, while most of the flexible regions are located on the protein surface. The optimum reaction temperature for pepsin was determined to be 60°C, and after incubation at 60°C for 30 min, the enzyme still retained more than 50% of its peak activity, indicating the rigidity of the pepsin structure. The results of the set of mutations provided by energy-based prediction software programs confirmed this result. Therefore, pepsin’s rigidity implies that the flexible regions on the surface are the major thermodynamic weakness point, and its design is more feasible. Compared with our results, Guo et al. generated a mutation on the surface ß-strand to increase the thermostability of BsAPA at an optimum temperature of 75°C [23]. Chen et al. generated a mutation on the surface a-helix to improve the thermostability of L-rhamnose isomerase at an optimum temperature of 85°C [50]. Mu et al. generated an interior mutant to improve the thermostability of glucoseoxidase via enhancing internal hydrophobic packing at an optimum temperature of 40°C [51]. The results of these studies are consistent with our finding that the reaction temperature reflects structural rigidity, to a certain extent, which assists in modifications.
The prediction software programs utilized in this study also have a cross-linked application in enzyme thermostability. In a study on improving the thermostability of agarase AgWH50C, Zhang et al. used a cross-linked protocol combining PoPMuSiC, HoTMuSiC, and ETSS, and successfully screened three mutations with improved thermostability from seven potential mutations [49]. This showed that combining multiple software programs can effectively reduce the scope of mutations for experimentation. However, in our study, the four mutations with improved thermostability performed poorly in PoPMuSiC, HoTMuSiC, and ETSS, only exhibiting positive performance in DeepDDG, and the best mutations (D52N and S129A) were not at the top of the list (Table 4). The linear combination of the statistical potentials used by PoPMuSiC depends on the volume of the wild-type and mutant amino acids [13]. The volume terms were also considered in the standard and temperature-dependent statistical potentials of HoTMuSiC [30]. Therefore, partial consistency in the results is expected. However, PoPMuSiC only considers the torsion angles defining the backbone conformation, whereas HoTMuSiC does not consider local structural rearrangements upon residue substitutions in the hydrophobic cores. ETSS does not consider the pH of the enzymatic reaction. Under the optimal pH for pepsin (2.0), the charge of the residue changes significantly, possibly causing errors [34]. Thus, each software program has its own limitations, and considering the structural weaknesses of the enzyme will be a highly appropriate choice.
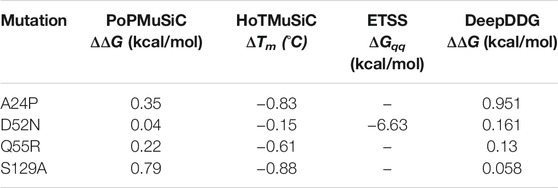
TABLE 4. Mutations with improved thermostability predicted using PoPMuSiC, HoTMuSiC, ETSS and DeepDDG software programs.
Thermostability modification is an important method for improving the industrial value of enzymes. Apart from quite blind and inefficient directed evolution, there have been many successful cases in the past decade involving local minor modifications such as introducing non-covalent or covalent interactions, increasing proline levels, or deleting glycine through protein engineering [52]. However, it is still difficult to accurately determine the regions and residues to be modified. Although thermostability prediction software programs can identify a clear mutation, their accuracy and effectiveness are poor and they therefore have low applicability [53]. By improving the thermostability of pepsin, we showed that thermodynamic weakness combined with rational design guided by prediction software programs can solve the difficulties involved in identifying the regions and the replacement residues, thus improving the accuracy of rational thermostable protein design. However, we must recognize that while we effectively and accurately obtained mutants with improved thermostability, assisted by the thermodynamic weakness of pepsin, in-depth structural analysis was based on high-resolution protein structure, which is a challenge for structure determination and prediction. The types and structures of enzymes vary, and the identification of thermodynamic weaknesses in different enzyme families requires comprehensive research. It is also necessary to develop systematic testing methods and trial-and-error experiments. Decreased activity of mutants with improved thermostability is the common activity-stability trade-off in enzyme design [54]. This needs to be addressed through additional research. Nevertheless, our research provides an effective method for modifying enzyme thermostability.
Data Availability Statement
The original contributions presented in the study are included in the article/Supplementary Material, further inquiries can be directed to the corresponding authors.
Author Contributions
YZ: performed the experiments, processed and analysed the data, and wrote the article; YM: performed the virtual calculation and dynamics simulation and interpreted the results; FZ: performed part of the experiments; YP: reviewed the paper and provided technical assistance; JZ and XY: reviewed the paper; JZ and LZ: acquired funding and provided project supervision, conceived and designed the study, and reviewed the article. All authors approved the final version of the article.
Funding
This work was supported by the National Key R&D Program of China (grant no. 2019YFA0905200), the National Natural Science Foundation of China (grant nos. U1805235, 31571786), and the NYU-ECNU Center for Computational Chemistry at NYU, Shanghai.
Conflict of Interest
The authors declare that the research was conducted in the absence of any commercial or financial relationships that could be construed as a potential conflict of interest.
Publisher’s Note
All claims expressed in this article are solely those of the authors and do not necessarily represent those of their affiliated organizations, or those of the publisher, the editors and the reviewers. Any product that may be evaluated in this article, or claim that may be made by its manufacturer, is not guaranteed or endorsed by the publisher.
Supplementary Material
The Supplementary Material for this article can be found online at: https://www.frontiersin.org/articles/10.3389/fphy.2021.755253/full#supplementary-material
References
1. Guo Y, Tu T, Yuan P, Wang Y, Ren Y, Yao B, et al. High-level Expression and Characterization of a Novel Aspartic Protease from Talaromyces leycettanus JCM12802 and its Potential Application in Juice Clarification. Food Chem (2019) 281:197–203. doi:10.1016/j.foodchem.2018.12.096
2. Li X, Cong Y, Ma M, You Z-N, Gao B, Zhang JZH, et al. An Energy Optimization Strategy Based on the Perfect Conformation of Prolyl Endopeptidase for Improving Catalytic Efficiency. J Agric Food Chem (2020) 68(18):5129–37. doi:10.1021/acs.jafc.0c00731
3. Polgár L. The Catalytic Triad of Serine Peptidases. Cell. Mol Life Sci (2005) 62(19-20):2161–72. doi:10.1007/s00018-005-5160-x
4. Gomes J, Steiner W. The Biocatalytic Potential of Extremophiles and Extremozymes. Food Technol Biotechnol (2004) 42(4):223–35. doi:10.1016/j.fm.2003.12.003
5. Lehmann M, Pasamontes L, Lassen SF, Wyss M. The Consensus Concept for Thermostability Engineering of Proteins. Biochim Biophys Acta (Bba) - Protein Struct Mol Enzymol (2000) 1543(2):408–15. doi:10.1016/S0167-4838(00)00238-7
6. Wijma HJ, Floor RJ, Janssen DB. Structure- and Sequence-Analysis Inspired Engineering of Proteins for Enhanced Thermostability. Curr Opin Struct Biol (2013) 23(4):588–94. doi:10.1016/j.sbi.2013.04.008
7. Baek M, DiMaio F, Anishchenko I, Dauparas J, Ovchinnikov S, Lee GR, et al. Accurate Prediction of Protein Structures and Interactions Using a Three-Track Neural Network. Science (2021) 373:871–6. doi:10.1126/science.abj8754
8. Hao L, Zhang R, Lohman TM. Heterogeneity in E. coli RecBCD Helicase-DNA Binding and Base Pair Melting. J Mol Biol (2021) 433(18):167147. doi:10.1016/j.jmb.2021.167147
9. Jones JC, Banerjee R, Shi K, Semonis MM, Aihara H, Pomerantz WCK, et al. Soluble Methane Monooxygenase Component Interactions Monitored by 19F NMR. Biochemistry (2021) 60(25):1995–2010. doi:10.1021/acs.biochem.1c00293
10. Jumper J, Evans R, Pritzel A, Green T, Figurnov M, Ronneberger O, et al. Highly Accurate Protein Structure Prediction with AlphaFold. Nature (2021) 596(7873):583–9. doi:10.1038/s41586-021-03819-2
11. Adzhubei IA, Schmidt S, Peshkin L, Ramensky VE, Gerasimova A, Bork P, et al. A Method and Server for Predicting Damaging Missense Mutations. Nat Methods (2010) 7(4):248–9. doi:10.1038/nmeth0410-248
12. Cheng J, Randall A, Baldi P. Prediction of Protein Stability Changes for Single-Site Mutations Using Support Vector Machines. Proteins (2006) 62(4):1125–32. doi:10.1002/prot.20810
13. Dehouck Y, Kwasigroch JM, Gilis D, Rooman M. PoPMuSiC 2.1: a Web Server for the Estimation of Protein Stability Changes upon Mutation and Sequence Optimality. BMC Bioinformatics (2011) 12(1):151. doi:10.1186/1471-2105-12-151
14. Masso M, Vaisman II. AUTO-MUTE 2.0: A Portable Framework with Enhanced Capabilities for Predicting Protein Functional Consequences upon Mutation. Adv Bioinformatics (2014) 2014:1–7. doi:10.1155/2014/278385
15. Parthiban V, Gromiha MM, Schomburg D. CUPSAT: Prediction of Protein Stability upon point Mutations. Nucleic Acids Res (2006) 34:W239–W242. doi:10.1093/nar/gkl190
16. Topham CM, Srinivasan N, Blundell TL. Prediction of the Stability of Protein Mutants Based on Structural Environment-dependent Amino Acid Substitution and Propensity Tables. Protein Eng Des Selection (1997) 10(1):7–21. doi:10.1093/protein/10.1.7
17. Landbo A-KR, Pinelo M, Vikbjerg AF, Let MB, Meyer AS. Protease-Assisted Clarification of Black Currant Juice: Synergy with Other Clarifying Agents and Effects on the Phenol Content. J Agric Food Chem (2006) 54(18):6554–63. doi:10.1021/jf060008d
18. Quintieri L, Caputo L, Monaci L, Deserio D, Morea M, Baruzzi F. Antimicrobial Efficacy of Pepsin-Digested Bovine Lactoferrin on Spoilage Bacteria Contaminating Traditional Mozzarella Cheese. Food Microbiol (2012) 31(1):64–71. doi:10.1016/j.fm.2012.02.015
19. Osire T, Yang T, Xu M, Zhang X, Li X, Niyomukiza S, et al. Lys-Arg Mutation Improved the Thermostability of Bacillus Cereus Neutral Protease through Increased Residue Interactions. World J Microbiol Biotechnol (2019) 35(11):173. doi:10.1007/s11274-019-2751-5
20. Xu B-L, Dai M, Chen Y, Meng D, Wang Y, Fang N, et al. Improving the Thermostability and Activity of a Thermophilic Subtilase by Incorporating Structural Elements of its Psychrophilic Counterpart. Appl Environ Microbiol (2015) 81(18):6302–13. doi:10.1128/AEM.01478-15
21. Zhao H-Y, Wu L-Y, Liu G, Feng H. Single-site Substitutions Improve Cold Activity and Increase Thermostability of the Dehairing Alkaline Protease (DHAP). Biosci Biotechnol Biochem (2016) 80(12):2480–5. doi:10.1080/09168451.2016.1230005
22. Rawlings ND, Barrett AJ. Introduction. In: ND Rawlings, and S Guy, editors. Handbook of Proteolytic Enzymes. Cambridge: Academic Press (2013) p. 3–19. doi:10.1016/b978-0-12-382219-2.00001-6
23. Guo Y, Tu T, Zheng J, Bai Y, Huang H, Su X, et al. Improvement of BsAPA Aspartic Protease Thermostability via Autocatalysis-Resistant Mutation. J Agric Food Chem (2019) 67(37):10505–12. doi:10.1021/acs.jafc.9b03959
24. Yoshimasu MA, Ahn J-K, Tanaka T, Yada RY. Soluble Expression and Purification of Porcine Pepsinogen from Pichia pastoris. Protein Expr Purif (2002) 25(2):229–36. doi:10.1016/s1046-5928(02)00003-1
25. Ryckaert J-P, Ciccotti G, Berendsen HJC. Numerical Integration of the Cartesian Equations of Motion of a System with Constraints: Molecular Dynamics of N-Alkanes. J Comput Phys (1977) 23(3):327–41. doi:10.1016/0021-9991(77)90098-5
26. Bizzozero SA, Dutler H. Stereochemical Aspects of Peptide Hydrolysis Catalyzed by Serine Proteases of the Chymotrypsin Type. Bioorg Chem (1981) 10(1):46–62. doi:10.1016/0045-2068(81)90042-0
27. Sielecki AR, Fedorov AA, Boodhoo A, Andreeva NS, James MNG. Molecular and crystal Structures of Monoclinic Porcine Pepsin Refined at 1.8A˚resolution. J Mol Biol (1990) 214(1):143–70. doi:10.1016/0022-2836(90)90153-d
28. Guo J, Rao Z, Yang T, Man Z, Xu M, Zhang X, et al. Enhancement of the Thermostability of Streptomyces Kathirae SC-1 Tyrosinase by Rational Design and Empirical Mutation. Enzyme Microb Tech (2015) 77:54–60. doi:10.1016/j.enzmictec.2015.06.002
29. Liao H, Gong JY, Yang Y, Jiang ZD, Zhu YB, Li LJ, et al. Enhancement of the Thermostability of Aspergillus niger α‐ L ‐rhamnosidase Based on PoPMuSiC Algorithm. J Food Biochem (2019) 43(8):e12945. doi:10.1111/jfbc.12945
30. Pucci F, Bourgeas R, Rooman M. Predicting Protein thermal Stability Changes upon point Mutations Using Statistical Potentials: Introducing HoTMuSiC. Sci Rep (2016) 6:23257. doi:10.1038/srep23257
31. Richmond TJ. Solvent Accessible Surface Area and Excluded Volume in Proteins. J Mol Biol (1984) 178(1):63–89. doi:10.1016/0022-2836(84)90231-6
32. Tanford C, Kirkwood JG. Theory of Protein Titration Curves. I. General Equations for Impenetrable Spheres. J Am Chem Soc (1957) 79(20):5333–9. doi:10.1021/ja01577a001
33. Tu T, Wang Y, Huang H, Wang Y, Jiang X, Wang Z, et al. Improving the Thermostability and Catalytic Efficiency of Glucose Oxidase from Aspergillus niger by Molecular Evolution. Food Chem (2019) 281:163–70. doi:10.1016/j.foodchem.2018.12.099
34. Zhang L, Tang X, Cui D, Yao Z, Gao B, Jiang S, et al. A Method to Rationally Increase Protein Stability Based on the Charge-Charge Interaction, with Application to Lipase LipK107. Protein Sci (2014) 23(1):110–6. doi:10.1002/pro.2388
35. Cao H, Wang J, He L, Qi Y, Zhang JZ. DeepDDG: Predicting the Stability Change of Protein Point Mutations Using Neural Networks. J Chem Inf Model (2019) 59(4):1508–14. doi:10.1021/acs.jcim.8b00697
36. Musil M, Stourac J, Bendl J, Brezovsky J, Prokop Z, Zendulka J, et al. FireProt: Web Server for Automated Design of Thermostable Proteins. Nucleic Acids Res (2017) 45(W1):W393–W399. doi:10.1093/nar/gkx285
37. Banach M, Fabian P, Stapor K, Konieczny L, Roterman a. I. Structure of the Hydrophobic Core Determines the 3D Protein Structure-Verification by Single Mutation Proteins. Biomolecules (2020) 10(5):767. doi:10.3390/biom10050767
38. Paul M, Hazra M, Barman A, Hazra S. Comparative Molecular Dynamics Simulation Studies for Determining Factors Contributing to the Thermostability of Chemotaxis Protein "CheY". J Biomol Struct Dyn (2014) 32(6):928–49. doi:10.1080/07391102.2013.799438
39. Akbulut N, Tuzlakoğlu Öztürk M, Pijning T, İşsever Öztürk S, Gümüşel F. Improved Activity and Thermostability of Bacillus Pumilus Lipase by Directed Evolution. J Biotechnol (2013) 164(1):123–9. doi:10.1016/j.jbiotec.2012.12.016
40. Veno J, Ahmad Kamarudin N, Mohamad Ali M, Masomian M, Raja Abd. Rahman R. Directed Evolution of Recombinant C-Terminal Truncated Staphylococcus Epidermidis Lipase AT2 for the Enhancement of Thermostability. Ijms (2017) 18(11):2202. doi:10.3390/ijms18112202
41. Xie Y, An J, Yang G, Wu G, Zhang Y, Cui L, et al. Enhanced Enzyme Kinetic Stability by Increasing Rigidity within the Active Site. J Biol Chem (2014) 289(11):7994–8006. doi:10.1074/jbc.M113.536045
42. Yu H, Huang H. Engineering Proteins for Thermostability through Rigidifying Flexible Sites. Biotechnol Adv (2014) 32(2):308–15. doi:10.1016/j.biotechadv.2013.10.012
43. Yedavalli P, Madhusudhana Rao N. Engineering the Loops in a Lipase for Stability in DMSO. Protein Eng Des Sel (2013) 26(4):317–24. doi:10.1093/protein/gzt002
44. Yu H, Zhao Y, Guo C, Gan Y, Huang H. The Role of Proline Substitutions within Flexible Regions on Thermostability of Luciferase. Biochim Biophys Acta (Bba) - Proteins Proteomics (2015) 1854(1):65–72. doi:10.1016/j.bbapap.2014.10.017
45. Yu H, Yan Y, Zhang C, Dalby PA. Two Strategies to Engineer Flexible Loops for Improved Enzyme Thermostability. Sci Rep (2017) 7:41212. doi:10.1038/srep41212
46. Okoniewska M, Tanaka T, Yada RY. The Pepsin Residue Glycine-76 Contributes to Active-Site Loop Flexibility and Participates in Catalysis. Biochem J (2000) 349(Pt 1):169–77. doi:10.1042/0264-6021:3490169
47. Malabanan MM, Amyes TL, Richard JP. A Role for Flexible Loops in Enzyme Catalysis. Curr Opin Struct Biol (2010) 20(6):702–10. doi:10.1016/j.sbi.2010.09.005
48. Wintrode PL, Zhang D, Vaidehi N, Arnold FH, Goddard WA. Protein Dynamics in a Family of Laboratory Evolved Thermophilic Enzymes. J Mol Biol (2003) 327(3):745–57. doi:10.1016/S0022-2836(03)00147-5
49. Zhang P, Zhang J, Zhang L, Sun J, Li Y, Wu L, et al. Structure-based Design of Agarase AgWH50C from Agarivorans Gilvus WH0801 to Enhance Thermostability. Appl Microbiol Biotechnol (2019) 103(3):1289–98. doi:10.1007/s00253-018-9540-1
50. Chen Z, Chen J, Zhang W, Zhang T, Guang C, Mu W. Improving Thermostability and Catalytic Behavior of L-Rhamnose Isomerase from Caldicellulosiruptor Obsidiansis OB47 toward D-Allulose by Site-Directed Mutagenesis. J Agric Food Chem (2018) 66(45):12017–24. doi:10.1021/acs.jafc.8b05107
51. Mu Q, Cui Y, Tian Ye., Hu M, Tao Y, Wu B. Thermostability Improvement of the Glucose Oxidase from Aspergillus niger for Efficient Gluconic Acid Production via Computational Design. Int J Biol Macromolecules (2019) 136:1060–8. doi:10.1016/j.ijbiomac.2019.06.094
52. Xu Z, Cen Y-K, Zou S-P, Xue Y-P, Zheng Y-G. Recent Advances in the Improvement of Enzyme Thermostability by Structure Modification. Crit Rev Biotechnol (2020) 40(1):83–98. doi:10.1080/07388551.2019.1682963
53. Pucci F, Bernaerts KV, Kwasigroch JM, Rooman M. Quantification of Biases in Predictions of Protein Stability Changes upon Mutations. Bioinformatics (2018) 34(21):3659–65. doi:10.1093/bioinformatics/bty348
Keywords: thermostability, aspartic protease, prediction software programs, structural weakness, site-specific mutagenesis
Citation: Zhao Y, Miao Y, Zhi F, Pan Y, Zhang J, Yang X, Zhang JZH and Zhang L (2021) Rational Design of Pepsin for Enhanced Thermostability via Exploiting the Guide of Structural Weakness on Stability. Front. Phys. 9:755253. doi: 10.3389/fphy.2021.755253
Received: 08 August 2021; Accepted: 20 September 2021;
Published: 30 September 2021.
Edited by:
Jinjin Li, Shanghai Jiao Tong University, ChinaReviewed by:
Zhi Yang, California Institute of Technology, United StatesXinqing Zhao, Shanghai Jiao Tong University, China
Copyright © 2021 Zhao, Miao, Zhi, Pan, Zhang, Yang, Zhang and Zhang. This is an open-access article distributed under the terms of the Creative Commons Attribution License (CC BY). The use, distribution or reproduction in other forums is permitted, provided the original author(s) and the copyright owner(s) are credited and that the original publication in this journal is cited, in accordance with accepted academic practice. No use, distribution or reproduction is permitted which does not comply with these terms.
*Correspondence: John Z. H. Zhang, Sm9obi56aGFuZ0BueXUuZWR1; Lujia Zhang, bGp6aGFuZ0BjaGVtLmVjbnUuZWR1LmNu