- 1Dipartimento di Ingegneria Elettrica Elettronica e Informatica, University of Catania, Catania, Italy
- 2CNR-IASI, Italian National Research Council, Institute for Systems Analysis and Computer Science, Rome, Italy
In this contribution, the main guidelines that, in the opinion of the authors, will address bioinspired technologies in the next future are discussed. The topics are related to some specific subjects. The presented perspectives could be useful to remark how bioinspired technologies can be applied to solve every day problems in a low cost and sustainable way. Moreover, all the considerations reported hallmark the need of changing the paradigm to design innovative bionspired systems. Efficient and alternative bioinspired systems cannot be designed by only looking at macroscopic scale as observed in nature. The efforts of this paper are oriented towards providing a wide perspective on bioinspired technologies as complex systems where nonlinear phenomena are fundamental elements.
1 Introduction
The main guidelines for the design of bioinspired advanced systems will be presented in this paper. The approach is generally oriented to the paradigm of biomimetics [1, 2] that consists in the examination of nature, its mathematical models, the various biological processes and then in the identification of all the elements emulating or taking inspiration from nature to solve human problems. The discussion presented in this communication is mainly related to research, presenting projects developed by the authors during the last decades [3–5]. Therefore, the paper does not discuss all the problems related to bionspired approaches. This paper can be considered a platform of discussion and perspectives and aims at proposing the paradigm of complex systems as an effective point of view on Bioinspired Technologies. The ideas are fixed points that derive from laboratory experiences, continuous discussions developed in the scientific community, projects with industrial partners, interdisciplinary knowledge integrated with modern silicon technologies, coupled to the complex system science.
The contribution is organized as follows. In Section 2 some aspects and applications based on the main principles of living systems considered of great interest for potential applications are presented, with some of them that already shown their capabilities for solving real problems. Focus will be given to the Cellular Nonlinear Networks (CNN) [6]. Moreover by using analog circuits strictly related to the Central Pattern Generator (CPG), innovative systems inspired by insect locomotion are presented [7].
In Section 3, the main principles that make living systems efficient are discussed. In particular both the active role of noise [8] and of imperfection [9–11] will be remarked. In considering new design paradigms, the previous two items are particularly relevant. A further general principle that can drive the design of dynamical bioinspired systems is synchronization [12, 13]. In Section 4, the challenges and the opportunities of fully bioinspired technologies will be discussed with reference to several other aspects. Further remarks regarding the Neural Networks and innovative concepts in distributed memories will be dealt with [14, 15]. The previous topics will be related to the concept of the active matter [16, 17], both looking to the active waves and the cooperative behavior. In Section 4 of this paper several examples of real living systems that could be source of inspiration for new technologies will be outlined. In particular the case of plant revolution [18–20] will be remarked.
The conclusive remarks will summarize the discussed concepts and the main ingredients in order to conceive innovative classes of bioinspired technologies.
2 Properties of Living Organisms for Innovative Real-World Challenges
In this Section, we will focus on peculiar properties related to the locomotion of living systems and how to use them in order to actuate robotic systems. In order to face this aspect, we want to introduce a bioinspired device on which the locomotion pattern generation is based, the Cellular Nonlinear Network (CNN).
2.1 The Cellular Nonlinear Network Paradigm
In introducing the CNN paradigm, the notion of emergent properties of a complex dynamical system is particularly important. This concept gained popularity due to the actual powerful performance of computers and to the internet era, this notion has a long history being related to the evolution, the self-organization and to the interaction among population of entities that could be said cells. With this term we indicate simple units that, thanks to their cooperation, lead to an emergent behavior, that cannot be explained observing and analyzing the behavior of the single units.
The history of emergence starts from the David Hume’s Dialogue concerning Natural Religions [21] and reached a mature level with the ideas of Gell-Mann [22] and Kauffmann and Mainzer [23, 24]. Definitely, in electronic and information technologies the concept of emergence has been widely applied by Leon Chua, in his theory of CNN architecture [25]. Leon Chua conceived the CNN concept in the 1988. The idea has been inspired by living systems and in particular by the synergetic properties of elementary units said cells that are locally connected as observed in living systems cells.
After the original paper by Chua and Yang in 1988 [25], different scientific communities dedicated their efforts to CNN studies, regarding both theoretical aspects and applications in different fields. CNN studies stimulated the improvement of technologies and VLSI design. A lot of books on CNNs have been published and the topic is still nowadays a fundamental one in various conferences and scientific societies. Since the beginning, every 2 years a conference dedicated to CNN studies has been regularly organized. Moreover, the CNN universal chip represents a milestone in information technology: it is a fully programmable 128 × 128, 0.35 μm CMOS cell processor, where the main features are the digital interface of the analog core interfaced with an array of photo-sensors for direct optical image and video processing. The chip, reported in the Figure 1, has been realized during the FP4 project DICTAM—Dynamic image coding using tera-speed analog IC visual microprocessors, with the participation of the University of Catania and STMicroelectronics.
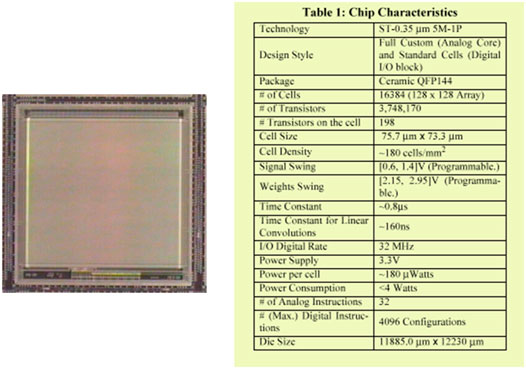
FIGURE 1. Photograph and list of chip characteristics of the 128 × 128 CNN-UM chip developed during the FP4 DICTAM project.
Even if high standard technologies lead to very sophisticated and fast devices (about 1015 operations per second), the absolutely simple and reliable concept of CNN allow scientists, researchers and practitioners to realize parallel analog architectures in their labs with low-cost components. This is the additional power of CNNs: each of us, each student can build his CNN with few components in his home lab! Our efforts in this review will be also devoted to give self-contained information and material to do this.
In its standard definition a CNN architecture consists of a rectangular array of M × N identical cells C(i, j) where (i, j) with i = 1, 2, …, M and j = 1, 2, …, N indicates the spatial coordinates of the single cell, i indexing the rows and j the columns.
A schematized representation of a CNN is reported in Figure 2A in which each cell is locally linked with the others within a connection radius equal to one. This means that each cell communicates locally with the nearest ones. In a general architecture a radius greater than one can also be considered. Indeed, the local connectivity permits the real-time propagation of the system variables to all cells. Information propagates similarly to a wave inside the network. Each cell, which is a continuous-time dynamical system (in the original CNN formalization it is a first-order system), is characterized by the following signals:
• The input signal
• The state variable signal
• The output signal
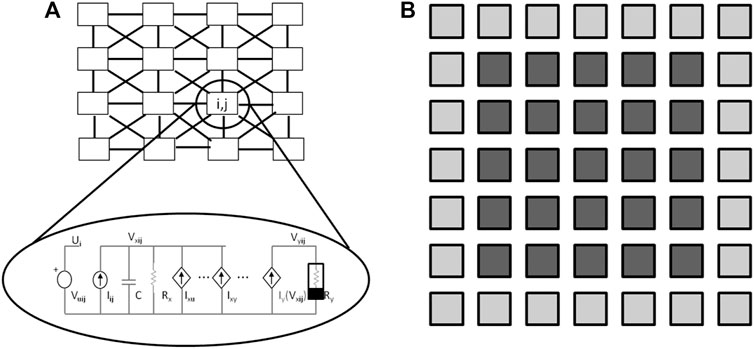
FIGURE 2. Cellular Nonlinear Networks. (A) Each cell C(i, j) of the array is connected with its neighbors and realized by the nonlinear circuit shown in the inset. (B) Additional cells (in light gray) are used to represent the boundary conditions of the cells of a 5 × 5 CNN (in dark gray).
The elementary electrical circuit of a single cell is reported in the inset of Figure 2A. It is a first-order dynamical circuit in which the state variable is the voltage across capacitor C and the output nonlinearity is realized through the nonlinear resistor Ry whose current-voltage characteristic implements the saturation function
As concerns the other elements in the considered circuit, they are voltage-controlled current generators and, in particular:
•
•
• Iij is a constant current generator.
Given these definitions, and referring to the circuit in Figure 2A, we apply the current Kirchhoff law to the generic cell C(i, j) to get:
with 1 ≤ i ≤ M and 1 ≤ j ≤ N, while Nr(i, j) represents the r-neighborhood of cell C(i, j) defined as
The operators A and B are labelled feedback and control template. Altogether A and B are referred to as the cloning templates. In practical applications space invariant templates and a 3 × 3 neighborhood (radius of influence r = 1) are usually considered.
We adopt the following notation:
• Boundary cells: those cells belonging to the first and last columns and rows;
• Corner cells: those cells located at the corners of the CNN.
The choice of appropriate boundary conditions is a fundamental point in problems of partial differential equations. In fact, the CNN represents a discrete-space, continuous-time system. Boundary conditions are applied considering a frame of additional cells around the M × N CNN, as represented in Figure 2B where the light gray cells compose the frame of a 5 × 5 CNN. The state variables of additional cells are set according to the following possible choices:
• Fixed, or Dirichlet, boundary conditions: the additional cells provide an output set to a constant value;
• Zero-flux, or Neumann, boundary conditions: the additional cells are constantly updated so that each of them provides an output which is equal to that of the proximal cell of the actual boundary;
• Periodic, or toroidal, boundary conditions: the additional cells replicate the output of the actual boundary at the opposite side of the CNN.
By using the convolution operator and normalizing with respect to the time constant RxC, the equation of each CNN cell becomes:
with 1 ≤ i ≤ M and 1 ≤ j ≤ N.
The model introduced with Eq. 4 describes the dynamical behavior of a generic M × N CNN whose cells can be considered as placed over a two-dimensional plane. This model is often indicated as single-layer CNN. Thus, in the single-layer model, each cell has a single state variable. Furthermore, it is worth to note that in Eq. 4 the cloning templates and the bias are considered as being space invariant. Due to the fact that the main applications of CNNs make use of this assumption, in the following sections cloning templates are considered space invariant. The model can be generalized to multiple state variables considering several stacked single-layer CNNs. A multi-layer CNN (or ML-CNN) can be also viewed as a single-layer CNN in which each cell has several state variables. Within the same cell any interaction between the state variables can occur, whereas the cell-to-cell interaction remains local, that is, restricted to the r-neighborhood.
The CNN scheme represents an analog system made by a huge number of differential equations. Let us consider, for example, a 128 × 128 CNN, its dynamics includes more than 13,000 state variables. The corresponding nonlinear dynamical system is solved in real-time in few milliseconds. This means that the steady-state stable equilibrium point, if it exists, is reached in short time, with high speed. Note also that we are considering nonlinear systems with a great number of equilibrium points so that the end point depends on the initial state and on the input signals. The previous considerations lead us to consider CNNs as very powerful analog circuits able to solve nonlinear partial differential equations. Moreover, they can constitute associative memories.
Indeed, the power of CNNs derives from their architecture and the nonlinear behavior of its dynamics. If we consider a CNN and a r-neighborhood with r = 1, the system dynamics is determined by the 9 coefficients of the template A and the 9 coefficients of the template B. Including the bias constant current I, we have 19 parameters. Hence, by considering space-invariant cloning templates, a powerful system with more than 10,000 equations depending only on 19 parameters can be obtained!
By using different cloning templates A and B we can program the CNN in order to obtain different operations. By choosing the cloning templates we build a dynamical system whose steady-state solution gives the desired processed information starting from a matrix of initial conditions and of input signals. The key point in the development of CNN systems is to create a suitable family of cloning templates that solves some basic operations. Then, executing one after the other such simple tasks, complex algorithms for image processing can be implemented.
Four aspects of CNNs must be remarked:
• They have been conceived as nonlinear image processing architecture;
• The images to process are used to initialize inputs and/or state variables, thus the size of the CNN should be fixed to reflect that (in pixels) of the considered image;
• Gray-scale images must be normalized in the range [−1, 1], where −1 maps white, 1 maps black and 0 the average gray;
• From their intrinsic structure CNNs are solvers of partial differential equations, that are discrete in space and continuous in time.
Real-time image processing is a difficult computational task such as, for instance, the times for classical segmentation and interpolation operations depend on the processor used to perform them. For their high performance in carrying out parallel processing and their high speed of execution, CNNs are a valid alternative method to the classical processing techniques. The cloning templates A and B to perform standard image processing have been derived heuristically taking into account some guidelines that have been successfully used in spatial digital filters. Furthermore, libraries of standard templates are available [26].
To apply CNNs in the field of image processing, the simple CNN model in Eq. 4 must be fed by a two-dimensional array of data, typically a gray-scale digital image that is set as the initial value (normalized in the [−1, 1] range) for the cell state variables. This image is then processed as result of the dynamical evolution of the CNN. Here for example if there is no input, then B(i, j; k, l) = 0 or uij = 0. As the evolution of the CNN dynamical system takes place, the state changes in a way that depends on the cloning templates. As soon as a steady-state is reached, then the state values (or the output) can be considered as the result of the processing task, reinterpreting them as the processed image. When B(i, j; k, l) ≠ 0 another array of data is available for processing, such that it can be used as a second operand and other tasks can be performed. In this way, the templates represent the instructions given to the machine performing the operations, while tje initial state and the input are the operands. More complex processing can be obtained by iterating this type of operations, using different templates in sequence, as it occurs for data processed by various instructions in a computer program. This duality between templates and computer instructions is the principle underlying the so-called CNN Universal Machine (CNN-UM).
One of the problems of CNNs, known as the learning and design problem, is how to find the templates that correspond to a given processing task. This problem is equivalent to build a dynamical system with the constraint of the CNN model and choose the templates in such a way that the dynamics of the designed system evolves in the desired manner. Practically, we have seen that the design problem is solved when a desired task could be transformed into a set of local dynamical rules. The dual learning problem is considered when the templates are obtained by using learning algorithms aimed at finding the coefficients so that pairs of inputs and outputs are matched.
Another interesting approach to select the cloning templates is the direct mapping between a partial differential equation and a CNN model. If an equivalence between the two models can be established, then the templates are directly derived.
Let us consider the elementary CNN cell, that can be viewed as a simple brick. Can we realize a general dynamical system by coupling more than one cell? From the coupling of dynamical cells, does the CNN generate a more complex dynamics? And what kind of nonlinear dynamics can be obtained from a CNN, taking into account that it is programmable and extremely flexible circuit? Quite importantly, answering these questions defines the class of circuits whose dynamics can be reproduced by the CNN. In this way, the cell becomes the primitive of a class of dynamical circuits. To provide the guidelines to establish what we called “procedure for the design of cells” a further definition of a new (and more complete) class of CNNs must be given.
The state controlled cellular neural network (SC-CNN) is a generalization of the standard CNN architecture obtained by introducing a further template, namely
where 1 ≤ i ≤ M and 1 ≤ j ≤ N and the operators A and B represent the feedback and control template, whereas the operator
The procedure to realize arbitrary dynamical circuits as SC-CNNs is based on the following steps:
• Define the discrete component realization of SC-CNN cells;
• Generate nonlinear dynamics by using SC-CNN by using a number of cells equal to the system order;
• Define a strategy to realize nonlinearity different from the canonical PWL one;
• Implement the nonlinear systems with circuits based on the SC-CNN cells.
These considerations lead to the conclusion that nonlinear systems with complex dynamics can be realized by CNN cells. So, although the cells are simple, that is, their uncoupled dynamics displays a trivial behavior, coupling them, as the CNN philosophy suggests, leads to the emergence of the complex dynamics. Moreover, the paradigm of CNNs can be extended by incorporating novel electronic devices which are at the same time nonlinear and memory elements, the so-called memristors [27, 28]. The use of such components allows CNN to be even more efficient and leads to a easier implementation of the integrated devices, as two key ingredients of CNNs (nonlinearity and memory) are merged in a single component opening the way to novel modeling paradigms [29].
2.2 The Reaction-Diffusion Cellular Nonlinear Network
Many nonlinear phenomena such as solitons, autowaves, and spiral waves appear in nonlinear active media and are of great interest in biology, physics, neuroscience and many other fields [30]. The model that characterizes them is the classical reaction-diffusion equation:
where
diffuses its effect in a medium thanks to the action of the diffusive term D∇2x. If the phenomenon occurs in active media there is the possibility that autowaves and active waves are generated. Reaction-diffusion equations can be solved mapping their dynamics on the CNN (or SC-CNN) architecture. We start describing the model of a universal cell that could represent the local reaction of an electronic cell.
Let us consider the following non-autonomous model:
Let us assume μ = 0.7, s = 1, ɛ = 0, i1 = −0.3, and i2 = 0.3 and integrate the system dynamics. In Figure 3A,B the temporal evolution of the two state variables x1 and x2 and the limit cycle described in the phase plane are shown. The oscillations are clearly periodic, but the waveform is far to be quasi-sinusoidal.
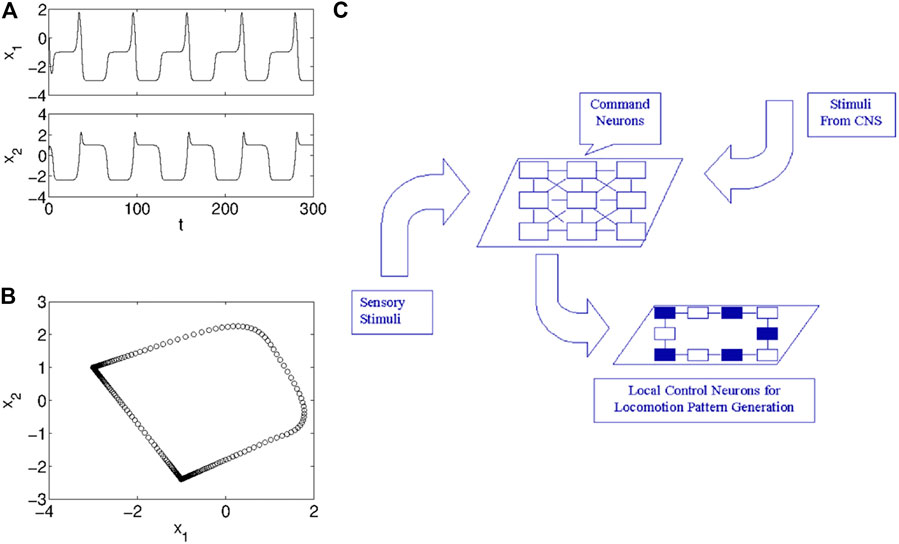
FIGURE 3. The Central Pattern Generator realized via CNNs. Dynamical behavior of the model (7): (A) temporal trend of the two state variables of a cell, and (B) trajectory of a cell in the phase plane. (C) Schematic representation of the Central Pattern Generator, which is divided into an ensemble of local neurons controlling the actuators of the locomotion pattern, and an ensemble of command neurons selecting the locomotion pattern to implement, on the basis of stimuli from higher neuronal centers or of sensor feedback.
A specific feature is observed: oscillations occurs with a slow-fast dynamics. This can be clearly seen by looking at Figure 3B where the trajectory in the phase plane is reported using markers taken with a constant sampling time. The density of markers is not constant along the cycle, meaning that portions of the cycle are fastly spanned (lower density) while other portions are slowly spanned (higher density). This is a key feature of many reaction-diffusion models and it is the reason why the model in Eq. 7, characterized by the slow-fast dynamics, is particularly suitable to realize a RD-CNN generating complex phenomena such as pattern formations and autowaves propagation.
It is here important to remark that the spiking dynamics observed in the CNN is also typical of other systems, as for example the laser with feedback recently revisited in [31]. This universal model, first proposed in 1986, has the same potentialities as the CNN model and of several other paradigmatic dynamical systems, hallmarking the importance of spiking dynamics in the implementation of control systems inspired to biological systems.
Once identified Eq. 7 as a potentially good model to mimic reaction-diffusion systems, we consider it as the dynamical system governing the M × N cells of a two-dimensional array. The equations of the global system are written as:
with 1 ≤ i ≤ M and 1 ≤ j ≤ N. The system represents a RD-CNN, and, in particular, a two-layer CNN, where the cells interact each other via two diffusive terms, each term acting in one of the two layers and associated with its own diffusion coefficient (D1 or D2). The model is reformulated in the standard two-layer CNN form as:
where
This reaction-diffusion CNN can be considered a reference for modeling, by means of circuits, different processes and phenomena. In fact, it can emulate both autowaves and Turing patterns which are the basis of the bioinspired robot locomotion system.
2.3 Bioinspired Locomotion
An important finding of neurobiological studies is the fact that coordinated motion is generated by the central nervous system (CNS) via specific patterns of motor neuron impulses. Muscle commands form a motor program that is structured before the movement begins and is sent to muscles with the proper timing assuring that a predefined motion sequence can be carried out, also in the absence of feedback from peripheral sensors [32]. The structure devoted to generate the motor oscillators in vertebrates and invertebrates is the Central Pattern Generator (CPG). This structure is capable of generating either plateau potentials or oscillations for rhythm generation, but also of driving the transition among various types of locomotion (walking, running and swimming) [33].
The CPG can be viewed as a complex of two groups of neurons. Local pattern generating neurons (LPGNs) are devoted to produce the rhythmic movements driving the mucles in animals or, more in general, e.g., in bioinspired robots, the locomotion effectors. These neurons are controlled by a higher level neural centre where the command neurons (CNs) are located. These are devoted to select a specific locomotion pattern among those possible, eventually also relying on sensorial feedback. This allows the output of the pattern generator to adapt to the environment [34, 35]. The hierarchical organization of the CPG can be schematized as in Figure 3C that shows as the CNs, as a result of sensory or central excitation, activate the LPGNs that then produce the appropriate timing signals needed by the specific locomotion pattern elicited by the CNs.
The model of the neural signal processing occurring in the CPG (in particular, the excitation and propagation of impulses in nerve membranes) is based on nonlinear RD PDEs. This observation is crucial as it enables its implementation via CNNs that, as we have seen, are arrays of programmable and easy realizable electronic analog circuits.
CNNs offer the possibility of an analog implementation of the autowaves, required in the LCPG, and the Turing patterns, used in the CNs, such that the whole control system of locomotion is based on solving nonlinear PDEs in a spatial medium. The signals obtained in the CNN are then used to control the joints of the robotic structure. The idea of using Turing patterns to model the CNs was introduced by Arena et al. [36]. Here, the core mechanism is that the Turing pattern reflects the specific locomotion pattern adopted. Instead, the LPGNs do not produce stationary patterns, but autowaves that are then used to trigger the muscle/motor system (more specifically, in the structures considered in the following the signals generated by the CNN cells are used to drive servomotors). A first example of a CNN-based CPG is shown in [37] where the CNN consists of nine cells that can generate up to eight stable patterns. These are associated to different locomotion patterns finally implemented by a one-dimensional array of cells (the LPGNs) where propagation of the autowave front takes place. This control system was applied to drive several robots, and in particular the worm-like robot illustrated in Figure 4A and the hexapod robot of Figure 5. Figure 4B,C shows the macroscopic wave-front propagation that takes place along the longitudinal robot axis, from the back to the front side, pushing the robot in the forward direction [38], in two diverse scenarios, where the neural system is intact and when it is damaged. The result is particularly striking as, even in the presence of severe fault, the hexapod is still able to move.
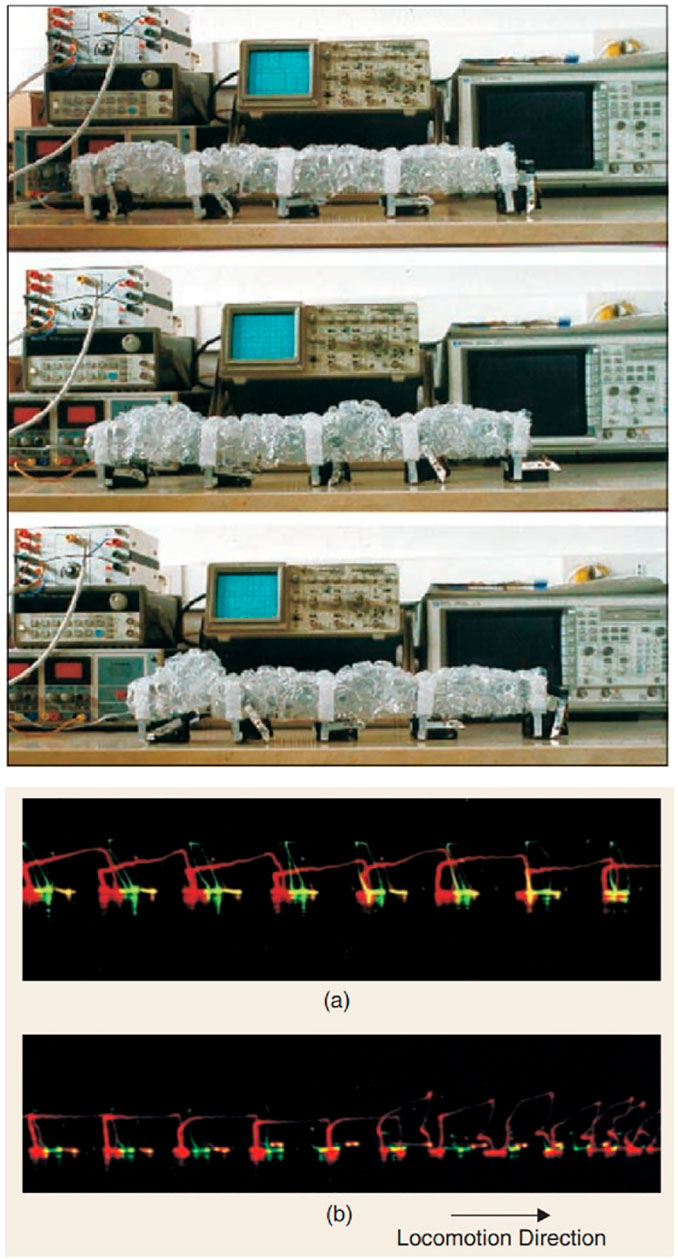
FIGURE 4. (A) A series of frames illustrating motion of the worm-like robot. (B) Regular locomotion leg cycles and (C) irregular leg cycles due to CNN ring damage in the hexapod robot. The image sequences were obtained by recording, in a long-exposure photo, the light emitted by LEDs mounted on the robot foots. The red trace is associated to the front leg trajectory, the green one to the middle leg, and the yellow to the hind leg.
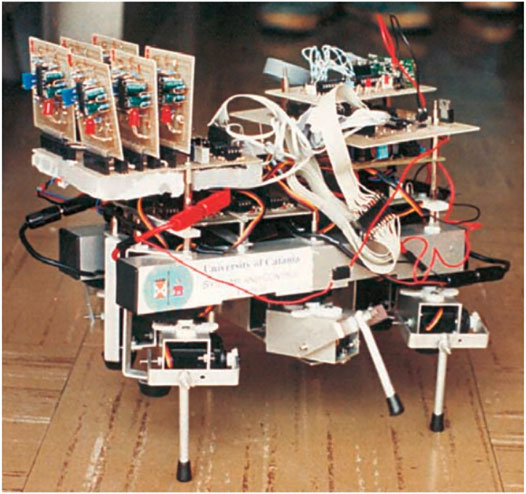
FIGURE 5. Rexabot: the Reaction-Diffusion walking hexapod. Each leg has two degrees of freedom, each actuated by a servomotor. The robot kinematics is designed so that both leg joints can be driven by the same output of a CNN cell. Motion coordination among the legs is obtained at the level of the CPG.
The case of the hexapod robot is particularly interesting also from the point of view of the implementation of diverse locomotion patterns. Insects, in fact, display extraordinary adaptation capabilities that manifest in several ways: one of them is their ability to readapt the locomotion pattern to the environment, here stereotyped via the implementation of three distinct gait patterns. Each gait is initiated by the CNs and in particular by one of the Turing patterns that can be generated. A first example is shown in Figure 6, that is obtained by setting an initial condition V0 = −0.15V on the cell A22. Via the classical mechanism of diffusion-driven instability underlying Turing patterns, this initial condition lets the other cells, initially set at zero (the equilibrium point), move from this point until, eventually, inhibition restores stability and the checkerboard pattern emerges ([37] for details). Hence, this pattern represents the equilibrium point for the six CNN cells. This Turing pattern corresponds to the fast gait, also known as alternating tripod, that is illustrated in Figure 7A. Each horizontal bar represents the time that each leg spends detached from ground (white part), or on the ground (black part) [33]. This is not the only gait implemented in the hexapod robot, on the contrary as shown in Figure 7B,C, the hexapod can move also according to the medium or slow gait. To realize all these patterns, the scheme of Figure 7D is adopted. Each cell of the 2 × 3 CNN is associated to a leg of the robot, and, for this reason, the cells have been labeled with the corresponding leg name. In the LPGNs where there is another CNN structure (the one generating autowaves), then the same signal needs to drive all the legs that belong to the same group as derived from the Turing pattern. For instance, for the fast gait, there are two groups of cells; the gait can be achieved by connecting the three legs, L1-R2-L3, to the same CNN cell (say A0), and the second set of legs, R1-L2-R3, to a CNN cell where the autowave signal has a phase shift of 180° with respect to A0. When another locomotion gait is selected by the CNs (via another Turing pattern), then in the LPGNs different pathways, involving a different number of neurons, will be activated, as shown in Figure 7D.
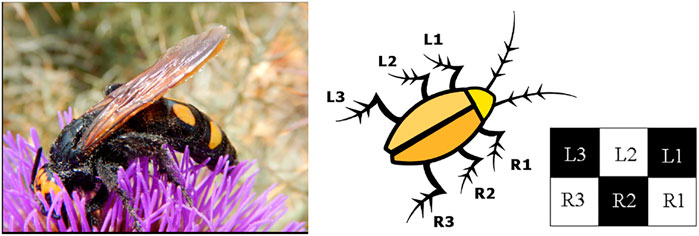
FIGURE 6. Turing pattern associated to the fast-gait. The pattern is characterized by two equilibrium values (depicted in black or white), which define the two groups of legs that have to move simultaneously: the tripod R1, L2, R3 and the tripod L1, R2, L3.
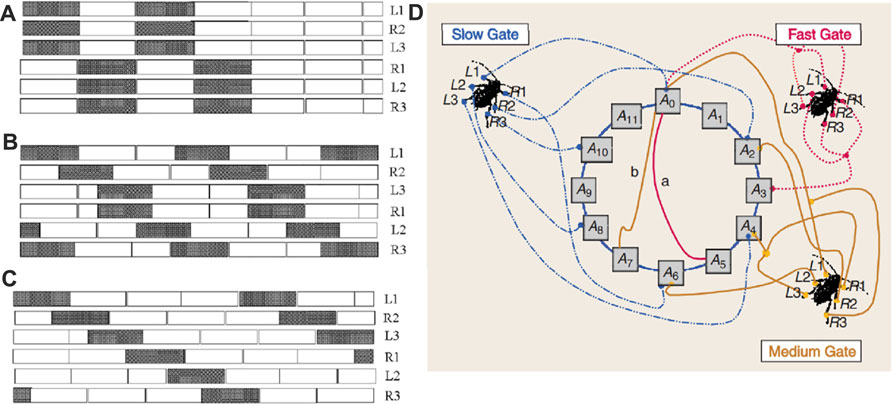
FIGURE 7. The three gaits implemented in the hexapod robot: (A) fast, (B) medium, and (C) slow. (D) Scheme of the locomotion pattern generator network implementing the three gaits. Each gait is realized implementing rings of different sizes in the CNN structure, the smallest one associated to the fast gait, and the largest one associated to the slow gait.
In more detail, the key elements of the control system are: a ring of neurons (realized by a CNN) where an autowave front propagates, a set of connections between given neurons and the motion effectors (in this particular case, a leg movement is elicited by firing in these neurons), and the possibility of changing the number of neurons within the loop, without affecting the cell structure. Since the cell dynamics is not changed, the speed of propagation of the wavefront is determined by the number of neurons within the loop, being the speed faster for a lower number of cells. Therefore, implementing the fast gait requires a chain of neurons in a number smaller than those required for the medium gait, that in turn requires fewer neurons than the slower gait. As shown in Figure 7, the fast gait can be obtained by connecting L1-R2-L3 to the cell A0 and the other legs to the cell A3 which has a phase shift of 180°. The ring consists of six neurons as the cells A5 and A0 are connected. For the medium gait, instead, the ring includes the cells from A0 to A9 such that the speed of the autowave front will be smaller and each leg will move at a slower frequency. Finally, in the slow gait the loop includes neurons from A0 to A11. In this simple way, exploiting the adaptivity of a reaction-diffusion CNN in modulating the speed of the wavefront as a function of the number of neurons, all three gaits are efficiently implemented.
The intrinsic robustness that derives from the use of spatio-temporal phenomena in the control system and the adoption of experimental analog setup pave the way to unexpected emergent properties. To illustrate them, we consider the fast gait and show that it proves to be particularly robust to faults in the cell circuits. The type of faults that is here considered is damaging one cell such that the ring is no more intact. Different behaviors are observed according to which cells are damaged. In particular, moving from simulations, where the initial conditions have to be properly selected to observe the autowave propagation, to real circuits, we notice that setting the initial conditions is no longer required as typically we find a dominant cell that triggers the onset of wave propagation. The dominant cell discriminates the different behavior that can be obtained. Indeed, if the ring is split in correspondence of the dominant cell, the locomotion rhythm is lost and legs are moved in irregular periods, due to the fact that the leading cell will start the oscillation at irregular times. Alternatively, different spurious wavefronts can also be observed. This behavior is reflected into complex locomotion patterns where the robot generally succeeds in the locomotion, but with a different sequence of leg movements with respect to that with the intact ring. We think that the results of these experiments carried out in our laboratory provide a clear evidence of emergent properties of the self-organizing dynamics of spatially-distributed systems.
It is interesting also to discuss the strategy to turn the robot. To change the robot direction (e.g., turning towards right), it is possible to let the leg R1 (belonging to the tripod R1-L2-R3) follow a longer trajectory with respect to the others. Using the CNN framework, this is straighforward if, rather than moving this leg at the same time of the other two legs by connecting it to the CNN cell A3, it is connected also to the cell A2. Doing so, R1 will move earlier than the others, such that to implement the desired change of direction.
Our attention has been mainly devoted to illustrate the CNN implementation of LPGNs and how they are connected to the robot legs so as to realize different types of gait. The CNs are only used to generate specific Turing patterns under the feedback from higher neuronal centers or from the environment. Here, for CNs we used a 2 × 3 CNN, which, as demonstrated in [39], can generate up to three stable patterns. If a larger number of patterns is required, then the size of the CNN should be increased. For instance, a 3 × 3 CNN can generate up to eight different configurations, a 4 × 4 CNN up to 16, a 5 × 5 CNN up to 32, and so on. The number of stable configurations grows as 2d where d is the size of the array employed. Once again, local connectivity and modularity are key to obtain the desired dynamics.
Another interesting feature of the CNN implementing the CNs is that the transition from one pattern to another can be elicited by acting only on a small number of cells. For example, to elicit the checkerboard pattern it is sufficient to set the initial conditions of only one state variable, despite the whole structure has a larger number of state variables (twelve). Similar results hold in larger structures, thus constituting another evidence of the synergetic effects where the so-called regulative variables enable the whole complex system to reach a given order [40]. These considerations are particularly relevant as a given stable configuration can be attained by choosing one or more regulative variables. Consequently, the greater the number of regulative variables employed concurrently, the shorter the time to reach the steady-state configuration. In our case, each regulative variable can be connected to a different sensor, so that the robot may change the type of locomotion in a faster way when many stimuli concurrently arrive at the CNs.
We notice here that using CNNs to generate and control locomotion of biologically inspired robots contrasts with the approach often adopted where control is performed in a highly sequential, digital and algorithmic way. With the CNN, the locomotion problem is solved in real-time, via the use of circuit emulator of nonlinear PDE solvers. In this sense, the CNN approach resembles biological locomotion that is a complex spatio-temporal phenomenon.
Thanks to the possibility of programming the templates, the proposed architecture is particularly flexible and can be adapted to other robotic structures. The same idea of switching the templates also enables other approaches where the cells/neurons are always linked to the same legs and the locomotion patterns are changed by reconfiguring the connections among cells in complex topologies [7, 41, 42] and the units composing the distributed control system can be generalized to consider other dynamics [43, 44]. Finally, it is worth mentioning that not only biological locomotion schemes can be reproduced with this approach, but also low cost analog and programmable circuits that could pave the way to a new control technology can be designed and realized [45].
From another point of view, robot locomotion can be controlled by using innovative brain-computer interfaces [46]. These technologies merge the use of nonlinear time-series analysis with efficient control algorithms, thus remarking the possibility to link the complex system of the human brain with the complexity of robot locomotion.
3 Guidelines and Principles That Make Living Systems Efficient
In this Section, two concepts will be emphasized: the crucial role that noise plays in living systems and the effects of imperfections will be discussed. Both noise and imperfections are considered negative effects in ideal linear systems [47, 48], but both are necessary in the evolution and in the biological development of the living system. Moreover, it will be shown that the efficiency of several biological systems are positively affected by both noise and imperfections.
3.1 The Effect of Noise
Could noise in biological systems lead to new classes of bioinspired devices? Noise is considered an undesired effect in several systems. Examples are the acoustic noise, the thermic noise in electronic devices, the effect of noise in telecommunication, the effect of the noise in linear control systems. The efforts of engineers are directed towards limiting the drawbacks of the noise. The tremendous efforts that is generally done in order to increase the measurement systems precision is due to the effect of the noise [49].
Consider now what happens in the living systems. Generally in the last century, biologists looked at the noise from a qualitative point of view taking conclusions only from observations. Therefore, in order to get more information, more experiments had been done and the statistics help a lot to get the correct interpretation of data. The recent studies [9, 50], both in nonlinear dynamics and in the emergent behavior of nonlinear distributed systems, lead to consider fluctuation and noise in biology in a different way. The noise is therefore considered not a drawback.
Many biological functions take advantages from stochastic fluctuations: consider for example the positive effect of noise in the evolution. Therefore, noise in biology is considered now in a positive way [8], addressing also information science in the perspectives to consider it an added values in some applications [51]. In biological systems and, in particular, in the cell dynamics, even if the noise is considered due to the increasing entropy that limits the channel information capabilities, noise has a surprising constructive role: evolution acceleration, increasing the fitness of the population and the information capabilities of the signal pathways.
The route from qualitative biology to quantitative biology, made by models and networks, informed us with many important general concepts that could be useful when transferred in systems engineering. Why do not consider the possibility of exploiting noise in nonlinear systems, looking at the positive effects that from it could derive? In order to reinforce the fundamental role of noise in biology, we have to look at the gene expression mechanism and in the transcription and translation mechanisms where the chemical master stochastic equation [51] addresses the biology, both in the large area in model based biology and in the specific area where innovative processing information systems could be conceived.
Moreover, the role of noise both in morphogenesis and in the population fluctuations is an intriguing theme that leads to reconsider positively the noise in real-world specific applications. The living cell is a small world of intense and continuous signals transmission. Even if from a macroscopic point of view the noise does appear to have a negligible aspect, being the cell a complex network made by the connections of nonlinear units, noise plays a fundamental role in its hidden communication paths [8]. A particular consideration arises today in evaluating the key role that spatiotemporal fluctuations play in several biological systems [52], allowing to conceive new approaches in order to open an intriguing area of application in network analysis and control.
Therefore the quantitative approach of biology stimulates the evaluation of the emergent aspects of noise and makes the biology models examples of impressive challenges to inspire new approaches and new systems, in the electronics and information technologies. The key aspect in this discussion is based on the research efforts made in biology considering today essential the nonlinear dynamics behavior and the self-organization principles. Moreover let us consider the case of unicellular organisms, such as the bacteria, that switch from normal state to spores. This switching phenomenon is classical in nonlinear dynamical systems in the presence of noise. Could the switching simple organism be considered like electronic memories? Or could be the classical electronic memories realized by living organisms working in the presence of noise? Considering again the developments of multicellular organisms, this is illustrated in modern morphogenesis as a symmetry breaking phenomenon, as explained by Turing [53]. It is the key principle of pattern formation. This is enabled by noise that triggers various switching phenomena in nonlinear dynamical systems. All these phenomena belong to the class of Reaction-Diffusion CNN, discussed in the previous section [54, 55].
Therefore biologically inspired CNN architecture in silicon, and noise opens another point of view in biology and suggest the idea of incorporating in the CNN circuits the principle of wave computation and wave memory based on the effect of noise in the CNN circuits. The stochastic resonance is a phenomenon of certain nonlinear systems that permits to detect the systems response thanks to the presence of suitable level of noise in the input. This characteristic is completely in agreement with the role of noise in biological mechanisms that encourage to design new systems for communication and to design signal detection equipments based on added noise systems [56, 57].
Let us consider how noise can have a positive effect in control theory by considering the simple system reported in Figure 8A. The feedback loop includes a dead-zone nonlinearity that prevents subthreshold inputs to be retrieved at the output of the system. Including a feedback noise signal with a suitable level, the stochastic resonance scenario that is triggered by the insertion of noise allows the subthreshold input signal to be reconstructed at the output, as shown in Figure 8B.
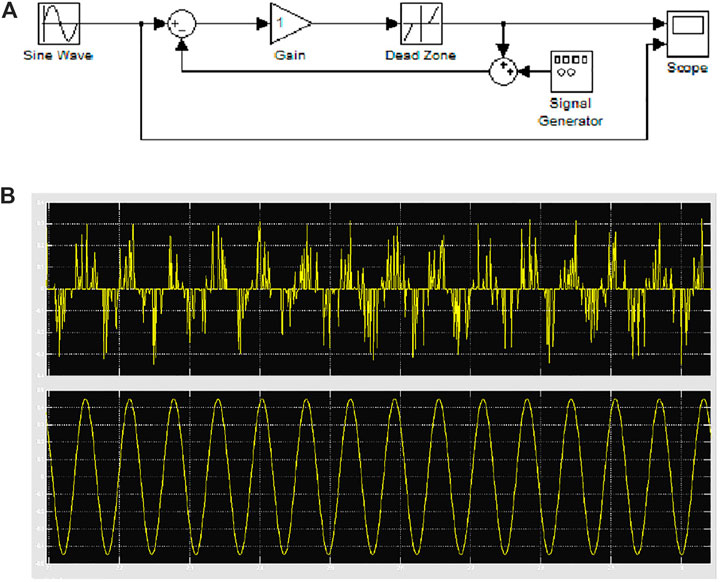
FIGURE 8. Stochastic resonance in nonlinear systems. (A) Closed-loop system with the inclusion of feedback noise. (B) The subthreshold input signal (lower panel) can be retrieved at the output of the closed-loop system (higher panel) when a suitable amount of noise is included.
Stochastic resonance is a revolutionary approach from an engineering point of view, since leads to design new devices that improve their behavior thanks to the noise, also in agreement with the message given by the biological world. In the next subsection this concept is further explored.
3.2 Imperfections
Rita Levi Montalcini wrote one of her more important books on imperfection [58]. In such contribution, she wrote this statement on imperfection:
“Imperfection has always allowed continuous mutations of that wonderful and very imperfect mechanism that is the human brain. I believe that imperfection is more in keeping with human nature than perfection.”
Imperfection is therefore a fundamental component of evolution. From amphibians to Homo Sapiens, the vertebrates brain has always let itself to an improvement, to a change, while in invertebrates it was born so perfect that it did not enter the game of mutations, so much so that the trilobites, which lived hundreds of millions of years ago, are essentially not different from insects, or from today’s arthropods. This is why imperfection “deserves praise”.
The philosopher Telmo Pievani in his book [59] remarks the main importance of the imperfection:
We are the result of a series of imperfections that have been successful. Our brains and our genome, two of the most complex systems that nature has produced, are full of imperfections.
The imperfect structures let us understand how evolution works. Nature does not work as engineers, who systematically optimize their inventions, but as a craftsman who does what he can with the material available, transforming it with imagination, arranging and reworking it. Even the natural history, that has led us this far, is a catalog of imperfections that have worked, starting from that infinitesimal deviation into the primordial quantum vacuum from which the universe was born. The philosopher of science and evolutionist Telmo Pievani, one of the most prominent Italian writers of science, has recently written a surprising essay where Lucretius and XXI century science go arm in arm. Tracing the history of imperfection is of great importance.
Taking into account the above considerations, the emerging concept of imperfect systems opens a new challenge in the area of bioinspired ideas. With this term, we do not refer to uncertain systems, but rather to systems, as it typically occur to real ones, that differ from their abstract mathematical model for any source of non-ideality. A few examples can be used to clarify this aspect. Friction-less and conservative systems are useful theoretical models in mechanics, however in real system it is friction that allows wheels to move. Analogously, junctions and transistors are not realized with “perfect” semiconductors, but on the contrary their working principle requires the presence of impurities. More in general, the imperfect dynamics are the source of the fluctuations that in turn allow the systems to properly operate. These imperfect dynamics can also be exploited to regularize the system behavior, for example inducing synchronization in imperfect systems coupled each other. Imperfect systems appear in biology, physics, engineering but also in art and many other fields. The importance of imperfections and imperfect systems is today subject of intense debate, as the efforts generally made to obtain perfect systems are often destined to fail, whereas often a system is working thanks to the presence of some level of imperfection.
A remarkable example are the patterns shown in Figure 9. The three cases are related to systems from different scientific fields: the oscillations in E. Coli populations [52], the oscillations of coupled pendulums [60] and the rotation of coils coupled in a magnetic field [61]. In the three cases synchronization occurs thanks to imperfections.
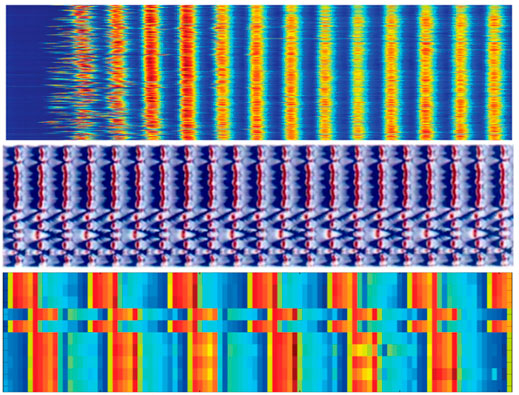
FIGURE 9. Synchronization induced by imperfections. Three examples of arrays of nonlinear systems in which organized behavior arises thanks to diversity and imperfections in the model. From top to down: coherent oscillations in the dimension of populations of E. Coli, organized collective motion of forced pendulums, and synchronized behavior of imperfect rotating coils.
4 The Active Matter
The theme of active matters is widely studied [62] and the term is essentially referred to the collective motion of animals, to their collective behavior, to the collective phenomenon of cell migration.
This concept is strictly linked to the paradigm of what has been discussed when CNN architectures has been introduced. In fact, CNNs are conceived to exploit the cooperative behavior of many simple elements, that are electronic cells. They can be considered as classical bioinspired high performance distributed electronic analog processors. Often today the swarm of simple robots is considered as a particular active matter. Active matter in living systems includes the concepts of large numbers of active agents, each consuming energy but that cooperating each other to achieve a double aim; the first one is the emergent behavior, that allows the agent swarm to perform tasks that the single agent cannot achieve as single unit; the second one is that cooperation allows the agent to save energy. Intrinsically, the swarm does work out of the thermal equilibrium.
Of course, we have many relevant examples of active matter in biology. The following examples reported here are only two different cases where the role of active matter is impressive, with not exactly known mechanisms so that the research on this subject is fascinating and can inspire future inventions of inspired biosystems and devices.
The discussion will be referred to three cases of collective behavior that emphasize another key concept, important in bioinspired systems: synchronization. Moreover, the case of the effects of turbulence in fluids may inspire artificial swimming systems where the recovery of force leads to something similar to the active matter.
4.1 Case 1: Catopsilla
The observations of C. B. Williams [63] referring to the swarms that cross each other without any collision is astonishing. After about one century, even if a mathematical model can be derived, the real conditions under which the swarm phenomenon occurs is still unknown. There is a swarm of butterflies that fly together and a swarm of locusts that penetrates inside the first without any collision. The distance among the insects are only few centimeters. Is a phenomenon that occurs because of the induced turbulence of the flight of each agent of the swarms? It appears that is what occurs in more immiscible fluids. If it is true, such type of conditions can be created in the swarm of flying robots without local control.
Moreover, in swarms of insects another unknown question does appear. They cover some thousand miles, they reach the target following light signals, but the target is reached even if this signal is lost. This is one example of active matter from which future bioinspired systems may arise. It is a very important problem. A possible change of paradigm would lead to an increase of knowledge and the peculiarities of flying swarms can stimulate this new paradigm.
4.2 Case 2: Fireflies
The Borneo fireflies collective behavior has been described many times. It is a fascinating show that exists thanks to the phenomenon of bioluminescence. Millions of fireflies flash synchronously. The precision of light synchronization is about few milliseconds [64].
The implementation of networks of decentralized elementary electronic circuits, further biological findings, advanced models, and new discoveries in the field of active matter could pave the way to realize innovative large-scale systems of synchronous oscillators. May these systems be also useful for brain understanding?
4.3 Case 3: Dolphins
Let us consider the dolphins swimming speed [65]. It is computed on the basis of studies on their metabolic balance, that indicate that the speed that dolphins should achieve based on these considerations is smaller than that really achieved by them. Is the turbulence in the water (which reduces the viscous dissipation) the reason for which dolphins may recover some energy?
Looking at these three open problems an area of intense activity emerges. Moreover even if the computer models today could help the scientist, only experimental observations and really new types of non invasive measurements could lead to reliable answers. An example of such experiment is the lamprey swimming robot [66] reported in Figure 10A, equipped with a CPG based on the CNN chip shown in Figure 10B.
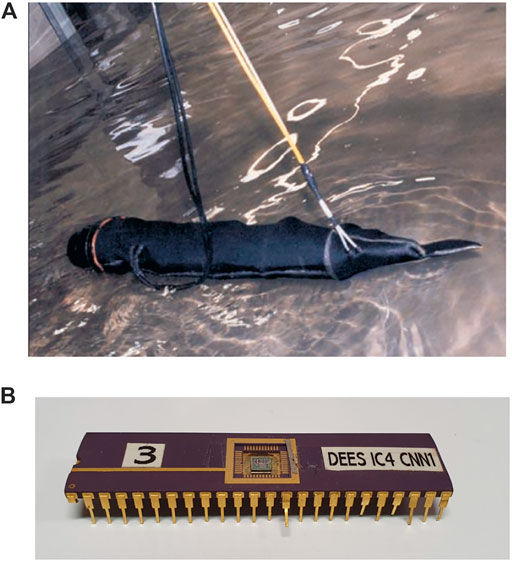
FIGURE 10. Lamprey-like swimming robot: (A) the robot in operation and (B) CNN chip implementing the lamprey robot CPG.
4.4 Towards Further Opportunities Looking at Nature
In order to get ideas for the realization of innovative biosystems, researchers must take into accounts that continuous observations and appropriate considerations must be done. Indeed, infinite sources exist that could help scientists. First of all, the full knowledge of the simplest organisms is the main source of appealing artificial realizations.
It is general opinion that in order to understand the real capabilities of each bioinspired system, scientists and engineers must look at the evolution of the biological organism taken as example for the artificial application. If we are interested in building intelligent motion control system, it is fundamental to look at the number of neurons of some set of organisms that realize the desired motion patterns. Therefore it is useful to keep in mind the Table 1 that allow us to derive some useful information. It reflects in part the animals evolution.
The number of neurons is generally referred as the quantity of information that the nervous system can process. The case of spiders is amazing. They have a limited numbers of neurons with respect to the autonomous capabilities they show. Even if the fruit fly and ants have about the same neurons of the spider, they are social insects. The spiders have extraordinary movements capabilities, therefore showing an excellent locomotion nervous systems, it has been recently proved that they have learning capabilities both in predation and in building [67], as shown by their capabilities to realize impressive cobwebs, like that reported in Figure 11A. It is absolutely important to consider in artificial systems and, in particular, in the design of artificial networks, that an high number of neurons do not guarantee an optimal learning. Few neurons but suitable patterns on interaction may lead to an efficient learning. This is the spider lesson.
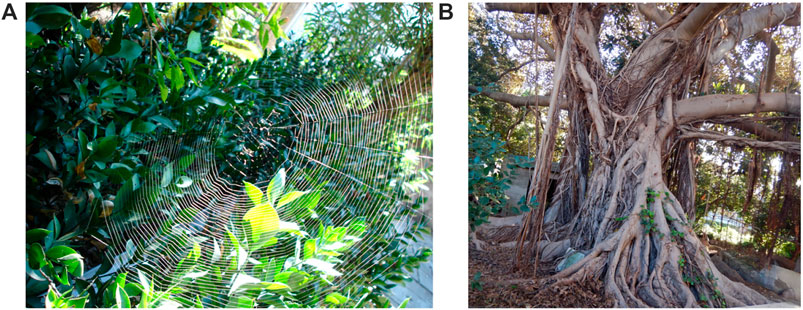
FIGURE 11. Structures in nature: (A) a cobweb showing accurate regularity and (B) root system of a ficus.
The following brief list of spiders could encourage the interested people to get ideas for further bioinspired systems:
• Segestria: this is a genus of tube dwelling spiders, the tubes bay branch and are often built in tree bark fissures.
• Argiobe basilica: their networks are similar in the shape to a church dome.
• Argyrometa spider: underwater spider that organizes its life underwater.
• Dolomedes fimbriatus: it establishes its living site near the rivers.
• Atrax spider: is one of the most dangerous spiders. Only recently the structure of its poison has been decoded in terms of the tridimensional sites of its peptides.
• Epeira foemina also identified as Avenus Diadematus: its characteristics is the elegant pattern in the body, nowadays the structure of her web is still unknown, it produces 7 types of threads.
• Deinopis also known as gladiator spider: it spins a small uprights rectangular crivellate web on the prey.
The number of spiders and the diversity of distinguishing actions that each genus shows is astonishing. The study of this animal allows to get intriguing bioinspired ideas.
Moreover the following consideration related to the number of neurons in the honey bee is interesting. It is a social insect, like the ant, moreover the higher number of neurons indicate something else. Researchers of the Queen Mary College proved that the honey bee has the capability to create a mental image. Have honey bee some type of consciousness?
As previously discussed in the case of distributed memories without neurons, the plants could in future be source of quite interesting bioinspired systems. This is due to the fact that the distributed functions of the plants lead to the understanding of new mechanisms of memory and to create the mathematical models of the plant behavior. Plants memory is based on biochemical mechanisms, they have robust distributed networks both to process information and to suitably use the energy, as the giant ficus reported in Figure 11B. Moreover the capabilities of the network of the plant roots inspire us to conceive new families of distributed sensors-actuators devices with high capabilities of self-regeneration. Do you imagine a type of robots that depending on the environment decides to improve its performance creating copies of itself, or to extend continuously its number of legs or manipulators? The mechanism of tropism, indicating grow and timing movements with a biological automatism in response to the environmental stimulus, makes the plant a reference in conceiving a new class of systems. In fact the main tropisms, like the phototropism, the gravimetric tropism, the contact tropism, the hydropic tropism, the oxigenon tropism, the electrical tropism, and, why not, the electromagnetic tropism, could be source of innovative bioinspired systems and new signal processing devices.
These ideas lead to new class of robots referred to with the term of plantoid [68]. This class of robots that can be displaced in the soil like seeds, works like a plant root, being able to investigate the soil and their characteristics. This is only an example, moreover the studies of Stefano Mancuso [69] could be stimulating in more areas and are intriguing in more applications.
5 Conclusion
In this contribution, some ideas and bioinspired applications, realized during EU funded projects, have been reported. The attention has been devoted essentially to the complex systems point of view, while the wide area of bioinspired materials has not been discussed in this work. Moreover, the main guidelines in order to conceive new classes of bioinspired systems based on nonlinear phenomena have been outlined.
This study is aimed at stimulating the interest in an analog green transition based on analog and bioinspired processing units, in order to conceive new devices and systems that improve their behavior based on nature principles. Moreover, the review is intended to investigate on the possibility of applying complex dynamical systems in the realization of efficient and green systems in a circular economy framework.
Much attention in the future must be devoted to the energy transfer and we are looking forward to have new flexible solar cells and silicon low voltage technologies, since they could be the premise to realize innovative artificial bioinspired systems, like the microrobot based on piezoelectric materials [70, 71] shown in Figure 12. Light is fundamental for any bioinspired device [72].
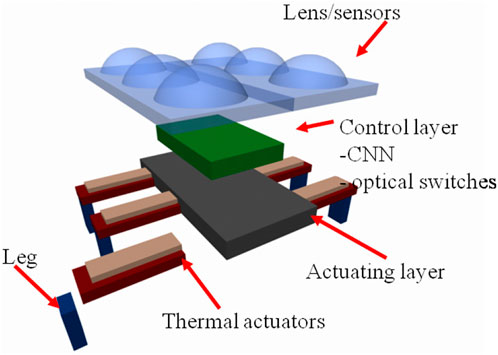
FIGURE 12. Schematic representation of a nanorobot based on piezoelectric materials for the actuation of the legs. The control system is based on a CPG implemented via a CNN chip. The top layer provides both a medium for harvesting solar energy and for collecting sensorial stimuli.
The key points that we would like to remark is that a new class of bioinspired systems must be supported by a complete nonlinear dynamics point of view. In fact many of the bioinspired systems of the last generation had been founded on using simple nonlinear system approaches. Looking at the new age of dynamical networks, the problems of collective behavior agent based systems can be an appealing topic in order to create innovative classes of bioinspired systems. The ideas of using the active role of the noise and to look at imperfections in view of what nature does, encourage us to conceive new classes of bioinspired systems, also taking into account these aspects. Distributed systems can be an even more appealing subject for the new age of bioinspired systems.
Moreover, for low cost green applications in the area of sensors and actuators, efforts must be devoted in understanding natural structures. As an emerging example, let us consider the conifer cone reported in Figure 13A. When the conifer cone is submerged in water, the increase of the internal humidity implies that the woody scales tends to close the cone, in order to preserve the seeds. Therefore, conifer cones can be used as natural actuators, for example actuating a switch, as in Figure 13B,C, to turn off the diode.
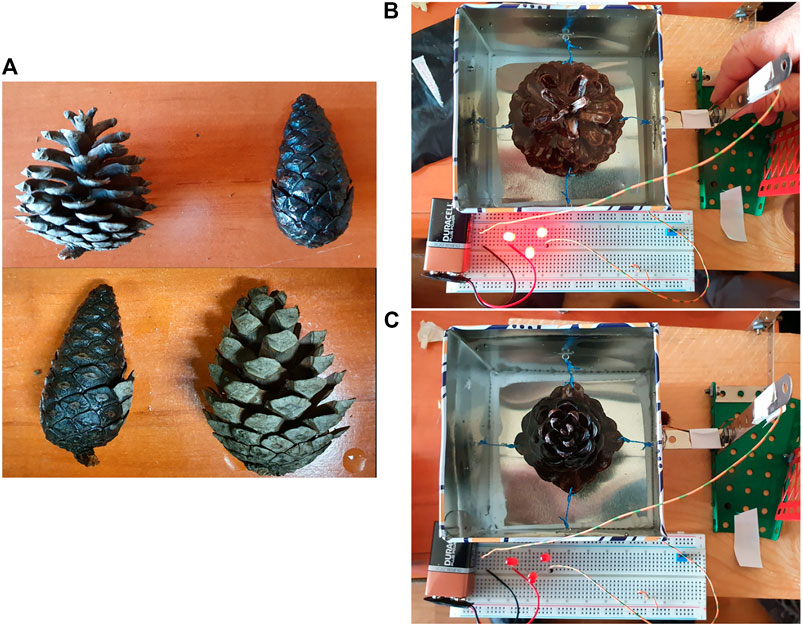
FIGURE 13. A green actuator. (A) Opening and closing of conifer cones scales due to humidity, (B–C) actuation of an electric switch by means of a closing conifer pine.
The structure of fluids circulation both in plants and in animals is a further appealing subject to take into account in the conception of new bioinspired systems. The key problem is to conceive innovative mechanical supports for the realization of the vessels and in this direction an appealing source of inspiration cames from plants and animals. Figure 14 reports astonishing examples of microchannels in living systems in comparison with a microfluidic device realized in our laboratories by using specific techniques [73–77]. The microfluidic devices should also be considered in future for the conception of artificial organs, such as the pancreas [78].
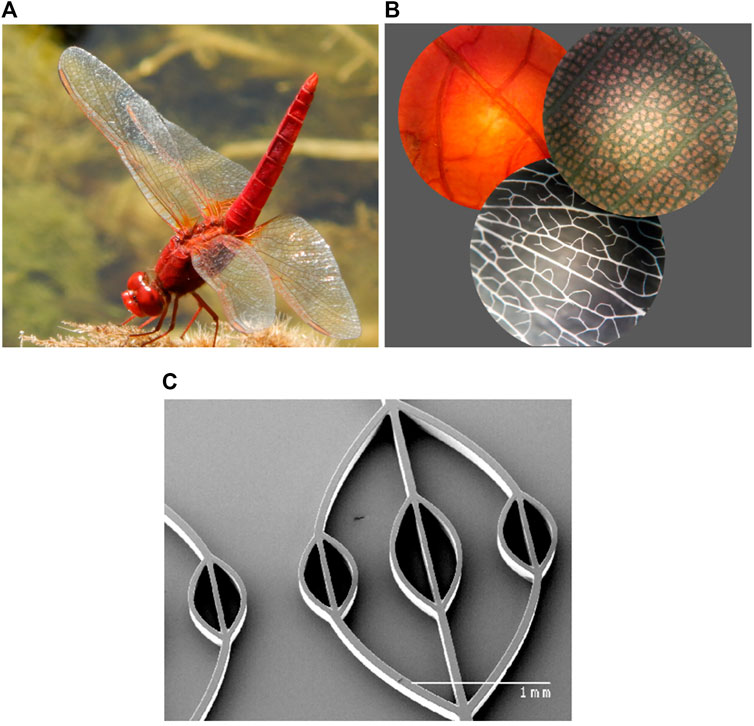
FIGURE 14. Patterns of microvessels: (A) wings of a dragonfly, (B) leafs of Poinsettia, ficus and ruscus hypoglossum, (C) microfluidic artificial device.
The main idea is that the revolution core of the information science and electronic technology has been given by the Cellular Nonlinear Networks paradigm. At the time of their invention (1988), internet was a dream and a global connection network had not been perceived. Moreover, the concept of local connectivity only gave at that time the revolutionary idea of wave-based communication. We remark that the original paradigm was from biology. It is therefore the CNN paradigm the best performance challenge bioinspired system. The realization of the hardware architecture based on the analog paradigm, as nature is, proved that the emergent behavior of CNNs is also due to the technological imperfections and to the threshold effects inside the cells, making the system suitably addressed also by noise [79].
Finally, we want to stress the concept that we must conceive new systems based on the fact that a change in the design paradigm must be done. Biosystems must be designed not copying nature but interpreting nature philosophy [80].
Author Contributions
All authors listed have made a substantial, direct, and intellectual contribution to the work and approved it for publication.
Funding
The paper is partially supported by the University of Catania under the framework of the plan PIACERI—Linea di Intervento 4 “Open Access”.
Conflict of Interest
The authors declare that the research was conducted in the absence of any commercial or financial relationships that could be construed as a potential conflict of interest.
Publisher’s Note
All claims expressed in this article are solely those of the authors and do not necessarily represent those of their affiliated organizations, or those of the publisher, the editors and the reviewers. Any product that may be evaluated in this article, or claim that may be made by its manufacturer, is not guaranteed or endorsed by the publisher.
Acknowledgments
The CNN chip ACE 128 × 128 has been developed under the project FP4 DICTAM involving as partner the University of Catania and as project leader STMicroelectronics. The locomotion devices have been developed thanks to the EU Projects SPARK I and SPARK II coordinated by the University of Catania. All the images and pictures are property of the Authors.
References
1. Balakrishnan P, Sreekala M S, Thomas S. Fundamental Biomaterials: Metals. Sawston: Woodhead Publishing (2018).
3. Manganaro G, Arena P, Fortuna L. volume 1. Cellular Neural Networks: Chaos, Complexity and VLSI Processing. Berlin: Springer Science & Business Media (2012).
4. Arena P, Patanè L. volume 1. Spatial Temporal Patterns for Action-Oriented Perception in Roving Robots. Berlin: Springer Science & Business Media (2008).
5. Buscarino A, Fortuna L, Frasca M. Essentials of Nonlinear Circuit Dynamics with MATLAB® and Laboratory Experiments. Boca Raton: CRC Press (2017).
6. Arena P, Fortuna L. Collective Behaviour in Cellular Neural Networks to Model the central Pattern Generator. Int J Syst Sci (2000) 31(7):827–41. doi:10.1080/002077200406561
7. Frasca M, Arena P, Fortuna L. volume 48. Bio-inspired Emergent Control of Locomotion Systems. Singapore: World Scientific (2004).
8. Tsimring LS. Noise in Biology. Rep Prog Phys (2014) 77(2):026601. doi:10.1088/0034-4885/77/2/026601
9. Bucolo M, Buscarino A, Famoso C, Fortuna L, Frasca M. Control of Imperfect Dynamical Systems. Nonlinear Dyn (2019) 98(4):2989–99. doi:10.1007/s11071-019-05077-4
10. Galli L, Maffei L. Spontaneous Impulse Activity of Rat Retinal Ganglion Cells in Prenatal Life. Science (1988) 242(4875):90–1. doi:10.1126/science.3175637
11. Bucolo M, Buscarino A, Famoso C, Fortuna L, Frasca M. Smart Control of Imperfect Electromechanical Systems. In: 2019 IEEE International Conference on Systems, Man and Cybernetics (SMC), Bari (Italy), October 6th-9th, 2019. IEEE (2019). p. 1882–6. doi:10.1109/smc.2019.8914052
12. Pikovsky A, Kurths J, Rosenblum M, Kurths J. Synchronization: A Universal Concept in Nonlinear Sciences. Number 12. Cambridge: Cambridge University Press (2003).
13. Cairone F, Anandan P, Bucolo M. Nonlinear Systems Synchronization for Modeling Two-phase Microfluidics Flows. Nonlinear Dyn (2018) 92(1):75–84. doi:10.1007/s11071-017-3819-0
14. Babaoglu O, Canright G, Deutsch A, Caro GAD, Ducatelle F, Gambardella LM, et al. Design Patterns from Biology for Distributed Computing. ACM Trans Auton Adapt Syst (2006) 1(1):26–66. doi:10.1145/1152934.1152937
15. Mancuso S. Plant Revolution. Le Piante Hanno Già Inventato Il Nostro Futuro. Firenze, Milano: GIUNTI EDITORE (2017).
16. Czirók A, Vicsek T. Collective Behavior of Interacting Self-Propelled Particles. Physica A: Stat Mech its Appl (2000) 281(1-4):17–29. doi:10.1016/s0378-4371(00)00013-3
17. Trepat X, Wasserman MR, Angelini TE, Millet E, Weitz DA, Butler JP, et al. Physical Forces during Collective Cell Migration. Nat Phys (2009) 5(6):426–30. doi:10.1038/nphys1269
18. Gagliano M, Vyazovskiy VV, Borbély AA, Grimonprez M, Depczynski M. Learning by Association in Plants. Sci Rep (2016) 6(1):38427–9. doi:10.1038/srep38427
19. Thellier M, Lüttge U. Plant Memory: a Tentative Model. Plant Biol (2013) 15(1):1–12. doi:10.1111/j.1438-8677.2012.00674.x
20. Gagliano M, Renton M, Depczynski M, Mancuso S. Experience Teaches Plants to Learn Faster and Forget Slower in Environments where it Matters. Oecologia (2014) 175(1):63–72. doi:10.1007/s00442-013-2873-7
22. Gell-Mann M. The Quark and the Jaguar: Adventures in the Simple and the Complex. New York: Macmillan (1995).
23. Kauffman SA. The Origins of Order: Self-Organization and Selection in Evolution. USA: Oxford University Press (1993).
24. Mainzer K. Thinking in Complexity: The Computational Dynamics of Matter, Mind, and Mankind. Berlin: Springer Science & Business Media (2007).
25. Chua LO, Yang L. Cellular Neural Networks: Theory. IEEE Trans Circuits Syst (1988) 35(10):1257–72. doi:10.1109/31.7600
26. Karacs K, Cserey GY, Zarandy A, Szolgay P, Rekeczky CS, Kek L, et al. Software Library for Cellular Wave Computing Engines (2010). Available at: http://cnn-technology.itk.ppke.hu/Template_library_v3.1.pdf (accessed October 12, 2016).
27. Buscarino A, Corradino C, Fortuna L, Chua LO. Taming Spatiotemporal Chaos in Forced Memristive Arrays. IEEE Trans VLSI Syst (2018) 26(12):2947–54. doi:10.1109/tvlsi.2018.2833291
28. Frasca M, Gambuzza LV, Buscarino A, Fortuna L. Implementation of Adaptive Coupling through Memristor. physica status solidi (c) (2015) 12(1-2):206–10. doi:10.1002/pssc.201400097
29. Bucolo M, Buscarino A, Fortuna L, Gagliano S, Stella G. Microfluidic Sensors Based on Memristive Circuits Synchronization. In: 2020 European Conference on Circuit Theory and Design (ECCTD), Sofia (Bulgaria), September 7th-10th, 2020. IEEE (2020). p. 1–4. doi:10.1109/ecctd49232.2020.9218414
30. Murray JD. Mathematical Biology: I. An Introduction. Interdisciplinary Applied Mathematics. Mathematical Biology. New York: Springer (2002).
31. Meucci R, Euzzor S, Tito Arecchi F, Ginoux J-M. Minimal Universal Model for Chaos in Laser with Feedback. Int J Bifurcation Chaos (2021) 31(04):2130013. doi:10.1142/s0218127421300135
32. Marsden CD, Rothwell JC, Day BL. The Use of Peripheral Feedback in the Control of Movement. Trends Neurosciences (1984) 7(7):253–7. doi:10.1016/s0166-2236(84)80218-0
33. Pearson K. The Control of Walking. Sci Am (1976) 235(6):72–86. doi:10.1038/scientificamerican1276-72
34. Stein PSG. Motor Systems, with Specific Reference to the Control of Locomotion. Annu Rev Neurosci (1978) 1(1):61–81. doi:10.1146/annurev.ne.01.030178.000425
35. Calabrese RL. Oscillation in Motor Pattern-Generating Networks. Curr Opin Neurobiol (1995) 5(6):816–23. doi:10.1016/0959-4388(95)80111-1
36. Arena P, Fortuna L, Branciforte M. Reaction-diffusion CNN Algorithms to Generate and Control Artificial Locomotion. IEEE Trans Circuits Syst (1999) 46(2):253–60. doi:10.1109/81.747195
37. Arena P, Branciforte M, Fortuna L. A CNN-Based Experimental Frame for Patterns and Autowaves. Int J Circ Theor Appl (1998) 26(6):635–50. doi:10.1002/(sici)1097-007x(199811/12)26:6<635:aid-cta37>3.0.co;2-u
38. Arena P, Fortuna L. Analog Cellular Locomotion Control of Hexapod Robots. IEEE Control Syst (2002) 22(6):21–36. doi:10.1109/mcs.2002.1077783
39. Arena P, Caponetto R, Fortuna L, Manganaro G. Cellular Neural Networks to Explore Complexity. Soft Comput - A Fusion Foundations, Methodologies Appl (1997) 1(3):120–36. doi:10.1007/s005000050013
40. Haken H. Erfolgsgeheimnisse der Natur: Synergetik: die Lehre vom Zusammenwirken. Stuttgart: Deutsche Verlags-Anstalt (1981).
41. Arena P, Fortuna L, Frasca M. Multi-template Approach to Realize central Pattern Generators for Artificial Locomotion Control. Int J Circ Theor Appl (2002) 30(4):441–58. doi:10.1002/cta.186
42. Arena P, Fortuna L, Frasca M, Sicurella G. An Adaptive, Self-Organizing Dynamical System for Hierarchical Control of Bio-Inspired Locomotion. IEEE Trans Syst Man Cybern B (2004) 34(4):1823–37. doi:10.1109/tsmcb.2004.828593
43. Minati L, Frasca M, Yoshimura N, Koike Y. Versatile Locomotion Control of a Hexapod Robot Using a Hierarchical Network of Nonlinear Oscillator Circuits. IEEE Access (2018) 6:8042–65. doi:10.1109/access.2018.2799145
44. Kitsunai S, Cho W, Sano C, Saetia S, Qin Z, Koike Y, et al. Generation of Diverse Insect-like Gait Patterns Using Networks of Coupled Rössler Systems. Chaos (2020) 30(12):123132. doi:10.1063/5.0021694
45. Arena P, Fortuna L, Frasca M, Patané L. A CNN-Based Chip for Robot Locomotion Control. IEEE Trans Circuits Syst (2005) 52(9):1862–71. doi:10.1109/tcsi.2005.852211
46. Belluomo P, Bucolo M, Fortuna L, Frasca M. Robot Control through Brain Computer Interface for Patterns Generation. volume 1389. In: ICNAAM 2011: International Conference on Numerical Analysis and Applied Mathematics, 19-5 September 2011, Halkidiki (Greece). American Institute of Physics (2011). p. 1031–4. doi:10.1063/1.3637786
47. Bucolo M, Buscarino A, Fortuna L, Frasca M. Nyquist Plots for MIMO Systems under Frequency Transformations. IEEE Control Syst Lett (2021) 6:169–174. doi:10.1109/LCSYS.2021.3053660
48. Bucolo M, Buscarino A, Fortuna L, Frasca M. Forward Action to Make Time-Delay Systems Positive-Real or Negative-Imaginary. Syst Control Lett (2019) 131:104495. doi:10.1016/j.sysconle.2019.104495
50. Bucolo M, Buscarino A, Famoso C, Fortuna L, Gagliano S. Imperfections in Integrated Devices Allow the Emergence of Unexpected Strange Attractors in Electronic Circuits. IEEE Access (2021) 9:29573–83. doi:10.1109/access.2021.3058506
51. Van Kampen NG. volume 1. Stochastic Processes in Physics and Chemistry. Amsterdam: Elsevier (1992).
52. Prindle A, Samayoa P, Razinkov I, Danino T, Tsimring LS, Hasty J. A Sensing Array of Radically Coupled Genetic 'biopixels'. Nature (2012) 481(7379):39–44. doi:10.1038/nature10722
53. Turing AM. The Chemical Basis of Morphogenesis. 1953. Bull Math Biol (1990) 52(1):153–2. doi:10.1007/BF02459572
54. Lefever R, Nicolis G, Borckmans P. The Brusselator: it Does Oscillate All the Same. J Chem Soc Faraday Trans 1physical Chem Condensed Phases (1988) 8484(4):1013–23. doi:10.1039/f19888401013
55. Dogaru R, Chua LO. Edge of Chaos and Local Activity Domain of the Brusselator CNN. Int J Bifurcation Chaos (1998) 08(06):1107–30. doi:10.1142/s0218127498000899
56. Gammaitoni L, Hänggi P, Jung P, Marchesoni F. Stochastic Resonance. Rev Mod Phys (1998) 70(1):223–87. doi:10.1103/revmodphys.70.223
57. Bucolo M, Buscarino A, Fortuna L, Gagliano S. Stochastic Resonance in Electromechanical Vibrating Systems. J Phys Soc Jpn (2020) 89(11):115001. doi:10.7566/jpsj.89.115001
58. Levi-Montalcini R. Praise of Imperfection: My Life and Work. Alfred P. New York: Sloan Foundation series (1988).
60. Braiman Y, Lindner JF, Ditto WL. Taming Spatiotemporal Chaos with Disorder. Nature (1995) 378(6556):465–7. doi:10.1038/378465a0
61. Fortuna L, Buscarino A, Frasca M, Famoso C. volume 91. Control of Imperfect Nonlinear Electromechanical Large Scale Systems: From Dynamics to Hardware Implementation. Singapore: World Scientific (2017).
62. Ramaswamy S. The Mechanics and Statistics of Active Matter. Annu Rev Condens Matter Phys (2010) 1(1):323–45. doi:10.1146/annurev-conmatphys-070909-104101
63. Williams C. A Migration of Yellow Butterflies (Catopsilia Statira) in trinidad. Trans R Entomol Soc Lond (1919) 67(1-2):76–88.
64. Sync SHS. How Order Emerges from Chaos in the Universe, Nature, and Daily Life. New York: Hachette UK (2012).
65. Nagamine H, Yamahata K, Hagiwara Y, Matsubara R. Turbulence Modification by Compliant Skin and Strata-Corneas Desquamation of a Swimming Dolphin. J Turbulence (2004) 5(18):1–2. doi:10.1088/1468-5248/5/1/018
66. Arena P, Fortuna L, Frasca M, Vagliasindi G. A Wave-Based CNN Generator for the Control and Actuation of a Lamprey-like Robot. Int J Bifurcation Chaos (2006) 16(01):39–46. doi:10.1142/s0218127406014563
67. Chapman RF, Chapman RF. The Insects: Structure and Function. Cambridge: Cambridge University Press (1998).
68. Mazzolai B, Salvini P. On Robots and Plants. In: Plant Ethics. London: Routledge (2018). p. 221–30. doi:10.4324/9781315114392-20
69. Mazzolai B, Laschi C, Dario P, Mugnai S, Mancuso S. The Plant as a Biomechatronic System. Plant Signaling Behav (2010) 5(2):90–3. doi:10.4161/psb.5.2.10457
70. Baglio S, Castorina S, Fortuna L, Savalli N. Modeling and Design of Novel Photo-Thermo-Mechanical Microactuators. Sensors Actuators A: Phys (2002) 101(1-2):185–93. doi:10.1016/s0924-4247(02)00200-5
73. Schembri F, Sapuppo F, Bucolo M. Experimental Classification of Nonlinear Dynamics in Microfluidic Bubbles' Flow. Nonlinear Dyn (2012) 67(4):2807–19. doi:10.1007/s11071-011-0190-4
74. Sapuppo F, Schembri F, Fortuna L, Bucolo M. Microfluidic Circuits and Systems. IEEE Circuits Syst Mag (2009) 9(3):6–19. doi:10.1109/mcas.2009.933853
75. Anandan P, Gagliano S, Bucolo M. Computational Models in Microfluidic Bubble Logic. Microfluid Nanofluid (2015) 18(2):305–21. doi:10.1007/s10404-014-1434-7
76. Sapuppo F, Schembri F, Fortuna L, Llobera A, Bucolo M. A Polymeric Micro-optical System for the Spatial Monitoring in Two-phase Microfluidics. Microfluidics and nanofluidics (2012) 12(1):165–74. doi:10.1007/s10404-011-0860-z
77. Cairone F, Gagliano S, Carbone DC, Recca G, Bucolo M. Micro-optofluidic Switch Realized by 3D Printing Technology. Microfluid Nanofluid (2016) 20(4):61. doi:10.1007/s10404-016-1727-0
78. Al-Hussein A-BA, Rahma F, Fortuna L, Bucolo M, Frasca M, Buscarino A. A New Time-Delay Model for Chaotic Glucose-Insulin Regulatory System. Int J Bifurcation Chaos (2020) 30(12):2050178. doi:10.1142/s0218127420501783
79. Bucolo M, Buscarino A, Famoso C, Fortuna L, Gagliano S. Automation of the Leonardo da Vinci Machines. Machines (2020) 8(3):53. doi:10.3390/machines8030053
Keywords: bioinspired technologies, analog computing, analog green transition, hybrid analog processors, active nonlinear dynamics, complex systems
Citation: Arena P, Bucolo M, Buscarino A, Fortuna L and Frasca M (2021) Reviewing Bioinspired Technologies for Future Trends: A Complex Systems Point of View. Front. Phys. 9:750090. doi: 10.3389/fphy.2021.750090
Received: 30 July 2021; Accepted: 23 September 2021;
Published: 13 October 2021.
Edited by:
Matjaž Perc, University of Maribor, SloveniaReviewed by:
Chunbiao Li, Nanjing University of Information Science and Technology, ChinaRiccardo Meucci, Istituto Nazionale di Ottica (CNR), Italy
Copyright © 2021 Arena, Bucolo, Buscarino, Fortuna and Frasca. This is an open-access article distributed under the terms of the Creative Commons Attribution License (CC BY). The use, distribution or reproduction in other forums is permitted, provided the original author(s) and the copyright owner(s) are credited and that the original publication in this journal is cited, in accordance with accepted academic practice. No use, distribution or reproduction is permitted which does not comply with these terms.
*Correspondence: Arturo Buscarino, YXJ0dXJvLmJ1c2Nhcmlub0B1bmljdC5pdA==