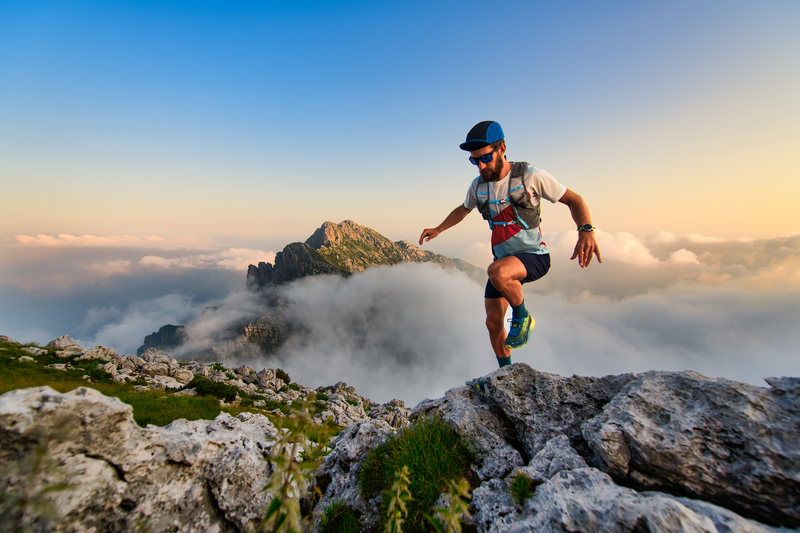
94% of researchers rate our articles as excellent or good
Learn more about the work of our research integrity team to safeguard the quality of each article we publish.
Find out more
REVIEW article
Front. Phys. , 09 June 2021
Sec. Biophysics
Volume 9 - 2021 | https://doi.org/10.3389/fphy.2021.666916
This article is part of the Research Topic Viscoelasticity: From Individual Cell Behavior to Collective Tissue Remodeling View all 11 articles
Spatiotemporal changes in viscoelasticity are a key component of the morphogenesis of living systems. Experimental and theoretical findings suggest that cellular- and tissue-scale viscoelasticity can be understood as a collective property emerging from macromolecular and cellular interactions, respectively. Linking the changes in the structural or material properties of cells and tissues, such as material phase transitions, to the microscopic interactions of their constituents, is still a challenge both at the experimental and theoretical level. In this review, we summarize work on the viscoelastic nature of cytoskeletal, extracellular and cellular networks. We then conceptualize viscoelasticity as a network theory problem and discuss its applications in several biological contexts. We propose that the statistical mechanics of networks can be used in the future as a powerful framework to uncover quantitatively the biomechanical basis of viscoelasticity across scales.
The viscoelastic or material properties of cells and tissues are key regulators of cell and tissue growth, motion, and homeostasis [1–5]. Viscoelasticity allows living systems to preserve a basic architecture due to their solid-like characteristics, but also at the same time to dynamically reorganize in different shapes and patterns due to their viscous-like characteristics [4, 6–8]. Cellular-scale viscoelasticity influences several single-cell functions such a shape, division, and motility, and it is predominantly determined by the physical properties of the underlying cytoskeletal networks [8]. Tissue-scale viscoelasticity was shown to be important in collective morphogenetic processes such as tissue folding, spreading, wound healing and migration, and it is mainly determined by the interplay of cell-cell and/or cell-extracellular space interactions [2, 4, 5]. Advances in biophysical tools measuring viscoelasticity [4, 9, 10] have revealed an essential and physiologically relevant link between material properties and morphogenesis [11–13], opening the challenge to now understand how emergent viscoelasticity is regulated by, and in turn, regulates the mechanochemistry of living systems.
A material is viscoelastic if it displays both viscous and elastic behavior [14]. Our knowledge of viscoelasticity mainly comes from material sciences, where certain physical parameters are well-defined for non-living materials such as glasses, rubbers, metals and polymers [14, 15]. Viscoelasticity of such materials is evaluated from the degree of deformation upon constant force application and release, an experimental procedure called creep and recovery test (Figure 1A). Solid-like objects deform shortly and reversibly under constant force, whereas fluid-like objects irreversibly increase their deformation as long as a force is exerted. Viscoelastic materials exhibit characteristics of both solids and fluids: at short time scales they deform elastically and, at long timescales, they behave as viscous fluids. On the theoretical level, viscoelasticity was independently modelled by Maxwell and Kelvin in the 19th century [14]. Both models abstract a viscoelastic system as a composite structure containing an elastic spring connected to a dashpot containing a viscous fluid (Figure 1B). The difference between Maxwell’s and Kelvin’s approaches comes from the disposition of the system: In Maxwell’s model, the dashpot and the spring are connected in series, whereas in Kelvin’s model, the two components are connected in parallel. As a result, when force is applied on the system, in the former, the deformation of the spring will drag the dashpot, describing the deformation of a viscoelastic fluid; whereas when force is applied in the latter, the dashpot response is restricted by the deformation of the spring, describing thus the deformation of a viscoelastic solid [16]. Although biological materials display characteristics of viscoelastic materials, due to their heterogeneous composition and dynamic nature, fitting such models and frameworks from material science, is conceptually and technically still difficult [16, 17]. For instance, during experimental measurements of cell and tissue viscoelasticity, typically an external force is applied to the system such as via a micropipette, or a magnetic field [13, 18, 19] for a certain time window, during which its deformation is monitored (Figure 1C’). From such measurements, parameters such as elastic modulus, viscosity, and yield stress can be extrapolated (Box 1) [16, 20, 21]. However, whether such measurements are relevant to the time window of morphogenesis still needs to be addressed, since such measurements show how a tissue deforms when applying an exogenous force for a certain time. How these features compare to the magnitude and duration of the endogenously exerted forces is still unclear [9, 22, 23]. In addition, during such experimental measurements, the applied force typically triggers a large deformation of the material (nonlinear regime). In contrast however, most theoretical frameworks predicting viscoelasticity upon deformation, are based on forces triggering infinitesimal deformations (linear regime) [24]. Last, similar to the viscoelasticity of non-living materials, viscoelasticity of cells and tissues is a property arising from their underlying structure, defined by the way macromolecules and cells interact [1, 8, 15, 25–29]. Several theoretical frameworks have for long been used in non-living materials to link the microscopic structure to the macroscopic viscoelastic properties (Box 1), showing that viscoelasticity may behave as an emergent property [27–32], in the same way, e.g., temperature arises non-trivially from microscopic particle motion in statistical mechanics [14]. How macroscopic viscoelasticity can be predicted by the interactions of the microscopic constituents of living cells and tissues is an open question at the interface of statistical and soft matter physics with molecular and cell biology, and the main topic of this review.
BOX 1 | Terminology.
Affine deformation: Deformation of a body in which the macroscopic strain is translated uniformly to all microscopic parts of the material.
Bulk modulus: Denoted by K, describes the material’s response to isotropic hydrostatic pressure.
Cell contact network: A network where the nodes are cells and links represent active contacts between neighbouring cells.
Contour length: In a polymer chain, the contour length, lc, is the distance between the two extremes of the filament if the polymer is fully unfolded.
Critical point: Magnitude of the control parameter that triggers a phase transition. At the critical point, a discontinuity on some macroscopic observable is expected, and specific statistical patterns, such as power-laws, often largely independent of system’s details, are observed. Formally, the functional dependence of the order parameter on the control parameter shows a singularity in some of its derivatives at the critical point.
Cytoskeleton: A network of biopolymer fibres that extends throughout a cell. It is the main determinant of the material response of the cell under deformations and stress.
ECM network: The extracellular matrix (ECM) is a non-cellular component providing the material backbone for the cellular constituents. Beyond its structural character, it plays a key, active role in morphogenesis, differentiation and homeostasis.
Elastic modulus: Also known as Young’s modulus, E, quantifies the strain response to uniaxial stress in the direction of this stress in the linear regime.
Enthalpic elasticity: In a polymer network where
Entropic elasticity: In a polymer network where
Interstitial fluid: space between the cells of a nonconfluent tissue.
Isostatic point: A system is at the isostatic point when the degrees of freedom of its constituents are absorbed by the constraints imposed by the structure in a non-redundant way. In the theory of rigidity, the isostatic point is marked by the critical value of connectivity in which the system becomes rigid, e.g., the lowest value of connectivity that leaves no degrees of freedom within the internal constituents of the system.
Jamming: Divergence of the viscosity of a material with increasing particle density
Micro/macro mapping: The mapping between microscopic dynamics, often containing a great number of degrees of freedom to a single, often scalar microscopic observable. The most common example is the microscopic motion of particles in a gas giving rise to the macroscopic observable of temperature.
Network rigidity: A topological concept where a network structure (or part of it) made of nodes and connecting links is considered to be generically rigid if no independent (geometric) movement of the nodes is possible without stretching/compressing a link.
Percolation: A network is in the percolating regime if a significant part of its nodes define a connected cluster, that is, for any pair of nodes of this cluster, there is a path that connects them. In a random network, the emergence of the percolating cluster is an abrupt event, and has all the properties of a high order phase transition as described in statistical mechanics. Many different classes of percolation transitions can be defined, depending on the attributes one expects to observe in the emerging cluster when the average connectivity increases.
Persistence length: In a polymer chain, the persistence length,
Phase transition: Macroscopic change in the properties of a system (order parameter) when a parameter crosses a certain critical value (control parameter), also called critical point.
Rigidity percolation: A high order phase transition triggered by increasing the average number of links per node in a network, leading to the sudden emergence of a rigid region that spans almost the whole network, the Giant Rigid Cluster (GRC). The term rigidity percolation comes from the fact that almost any two nodes of the graph are connected through a path that is entirely inside the same rigid cluster.
Shear modulus: Denoted by G, quantifies the material's response to shear stress.
Strain: Denoted by
Strain softening response: Non-linear response of many biological materials consisting on the decrease of the stiffness along the increase of strain.
Strain stiffening response: Non-linear response of many biological materials consisting on the increase of the stiffness along the increase of strain.
Vertex models: Tissue models represented by tilling of the space (in general, over 2D surfaces) in which the energetic contributions come from geometric considerations on the cell shape and cell-cell contact regions (vertices).
Viscosity: Denoted by
T1 transition: Relative movement of cells in a tissue occurring when an edge between two cells shrinks to a point and a new edge arises between two neighbouring cells. The outcome of this process is a net change of neighbouring cells and, in consequence, a relative movement, with respect to the other cells, of at least one cell within the tissue.
FIGURE 1. Emergence of cell and tissue viscoelasticity. (A) Strain plots as a function of time for different materials during constant force application (grey shaded box) and release. (B) Schematic illustrations of the Maxwell and Kelvin viscoelastic models. In the Maxwell model (top) the spring and dashpot are connected in series and account for the behaviour of viscoelastic fluid materials. In the Kelvin model (bottom) the spring and dashpot are connected in parallel and account for the behaviour of viscoelastic solid materials. Stress σ is applied along the axis of the spring triggering a strain γ. k is the spring constant and η is the viscosity of the fluid. (C) Cellular-scale viscoelasticity is defined by the cytoskeletal network and extracellular matrix. The close ups illustrate an exemplary composition of an actin and collagen network. (C’) Schematic illustration of an experimentally induced deformation of a cytoskeletal network and a paradigm of how viscoelastic properties can be computed from such experiments. (C”) Stiffness-strain plots of actin and collagen networks exhibiting a non-linear increase of their elastic modulus, or a stress-stiffening response. The plot was adapted from [71, 141]. (D) Tissue-scale viscoelasticity is determined by several cellular processes such as cell rearrangements, cell-cell adhesion and cell division. (D’) Schematic illustration of an experimentally induced deformation of an embryonic tissue using micropipette aspiration and a paradigm of how viscoelastic properties can be computed from such experiments. (D”) Plot of the nonlinear decrease of tissue viscosity as observed during zebrafish morphogenesis.
An intriguing empirical observation is that the material properties of the microscale components of cell and tissue viscoelasticity, such as the cytoskeletal elements and the cells, respectively, usually do not match the macroscale material properties of cells and tissues [15, 29, 33]. Macroscopic viscoelasticity frequently exhibits nonlinear changes that are not observed at the microscopic level. Such examples have been experimentally detected such as the strain-stiffening response of the cytoskeleton networks [26, 30, 34], phase transitions in the energetic costs of cell movements [12] or abrupt changes in tissue viscosity [13] (Box 1, Figures 1C”,D”). In the above cases, the mechanical resilience of the individual microscopic components to forces falls short in explaining the macroscopic viscoelastic changes, and thus probing the pattern of interactions between the components instead, is key.
Such nonlinear phenomena set a number of challenges to the theoretical understanding of cell and tissue dynamics. Theoretical analyses of cell and tissue material properties are typically addressed from the biopolymer or cell level, respectively. In the first theoretical approach, the microscopic basis is the mechanical properties of the biopolymer filaments building the cytoskeleton [35] and the macroscopic viscoelastic features are derived from the network geometry and local topology of the filaments [26]. Nonlinear phenomena such as strain-stiffening of cytoskeletal networks have been probed with such models. In the second theoretical approach, the microscopic basis is the tiling patterns of the constituents, such as the cells forming a tissue. In such modeling frameworks, mainly represented by the vertex models (Box 1) [25, 36, 37], rheological properties such as rigidity and fluidity are inferred from the energetic costs for cells to independently move through the tissue [27, 28, 38–41]. This viewpoint comes from the fundamental observation that material deformation can only take place through cell-cell rearrangements. Nonlinear phenomena such as jamming transitions (Box 1) have been predicted with such frameworks [27, 28, 40–42]. A third theoretical approach that is not as frequently applied in active viscoelastic systems, but has been used so far to probe material properties across scales, is network theory [29, 31, 43, 44]. In this framework, the starting point is the topology of the network, e.g., how the system’s constituents are connected between them. Of particular relevance for these approaches is the concept of percolation (Box 1). Percolation refers to a wide range of phenomena where a sudden shift in the macroscopic properties of a system made of microscopic, interacting units, is observed when a certain threshold of connectivity at the microscopic scale is overcome [45]. In material sciences, percolation transitions underlie many sudden, qualitative changes in the behavior/response of the material, including, among other, shifts in rigidity and force transmission properties [46–54]. A paradigmatic example of the role of percolation theory in explaining material properties is found in the exploration of the emergence of cracks when the material is under stress. In this context, the length and width of cracks emerging in the material increase dramatically when the system approaches the critical point of rigidity percolation [55, 56] (Box 1). Several forms of percolation theory have been applied to cytoskeletal networks, fiber networks and, recently, to “cellular” networks - tissues - to map material properties [29, 31, 43, 44]. As is the case with inanimate materials, the structure of interactions at the microscopic level --and the potential nonlinear shifts arising from small changes in them-- are supposed to underlie and, ultimately, explain, the emergence of macroscopic material properties like viscoelasticity.
In this review we summarize and discuss experimental and theoretical work probing cell and tissue viscoelasticity as an emergent property. We will first introduce experimental findings on how viscoelasticity emerges in cytoskeletal networks, extracellular matrix fiber networks and tissues. We will then summarize and classify theoretical frameworks supporting such experimental findings that address cell and tissue material properties. Finally, we will discuss the potential of applying network theory to predict viscoelasticity, and speculate how such an approach could impact our biophysical understanding of the material properties of living systems and their morphogenesis.
Changes in cellular-scale viscoelasticity are key for cell physiology [57]. Both the cytosol and cytoskeleton contribute to cellular-scale viscoelasticity, with cytoplasmic viscosity dominating processes of macromolecular movement [58] and cytoskeletal viscoelasticity influencing cell morphology, motility and division [8, 57]. Given that experimental work suggests that the cytoskeleton is the major determinant of cellular viscoelasticity [59, 60], we focus here on its viscoelastic properties. The cytoskeleton is the underlying biopolymer scaffold of living cells, and its viscoelasticity offers a balance between dynamic reorganization and maintenance of the cell body. Cytoskeletal viscoelasticity is a complex phenomenon, since it arises from the mechanical properties and interactions of at least three different biopolymers: actin, microtubules and intermediate filaments. These fiber structures further self-organize into filaments and heterogeneous networks through mechanisms of entanglement, branching, crosslinking and bundling (Figure 1C) [61–64]. Such mechanisms involve several types of linkers, such as cross-linking/bundling proteins a-actinin and fascin, and motor proteins like myosin and kinesin [65]. In general, the mechanical properties of the cytoskeleton depend on the physical properties of the individual filaments, the pattern of linkages between the filaments, and the geometry of the filament arrangement [66, 67]. Although cell viscoelasticity is a result of the heterogeneous mechanical properties of all cytoskeletal elements together, our understanding of this process comes mostly from studying each type of element separately (for extensive reviews see [1, 3, 8]).
At the single-filament level, each cytoskeletal biopolymer displays different physical properties, assessed by the ratio of its persistence length (
Besides the emergence of material properties in intracellular cytoskeletal networks, similar behaviors are observed in networks of the extracellular matrix (ECM), the non-cellular material backbone spanning cells and tissues. ECM viscoelasticity is fundamental in cell migration, tissue morphogenesis, organ development, and cancer progression [5, 79, 80]. The ECM is a heterogeneous network composed by several biopolymer filaments, such as fibronectin, laminin, and collagen, that exhibit various persistence lengths. This can range from the 4–8 nm persistence length
Similarly, tissue-scale viscoelasticity has been recently experimentally measured to undergo nonlinear changes [11–13, 86–89] resembling phase transitions [90–92]. Direct measurements of viscoelastic features such as yield stress and viscosity have been performed in embryonic tissues and spatial and/or temporal drastic changes have been observed [12, 13]. In the case of the early zebrafish blastoderm, for example, tissue viscosity was found to abruptly drop by more than an order of magnitude within a few minutes at the onset of morphogenesis [13] (Figures 1D’, D’’). In addition, comparison of the yield stress between two neighboring tissues along the zebrafish body axis, the presomitic mesoderm and the progenitor zone, has revealed the presence of solid-like and fluid-like tissues, respectively [12]. However, does cell viscoelasticity scale in such cases with tissue viscoelasticity? Although --to our knowledge-- no simultaneous analysis has been performed yet on measuring both cell and tissue scale viscoelasticity under different conditions to quantitatively assess their relationship, several lines of evidence point at the hypothesis that tissue-scale viscoelasticity critically depends on the interaction patterns between cells. Along these lines, it was reported that inhibition of myosin cytoskeletal motors in zebrafish, that is expected to decrease cell-scale viscoelasticity, had no effect on tissue viscosity [29]. Similarly, pharmacological treatments of the zebrafish tailbud with blebbistatin (a pharmacological myosin II inhibitor), which lowers cytoskeletal elasticity, had surprisingly inverse results on the tissue-scale material properties, where the tissue yield stress in treated embryos it is almost double than the control embryos [12]. In contrast, changes in cell rearrangements, cell-cell adhesion, contractility and cell division rates were shown to trigger changes in the tissue scale material properties (Figure 1D) [11–13, 86–88, 93]. This agrees with extensive theoretical work inferring tissue material phase transitions based on certain cell parameters such as cell motility and adhesion [27, 28, 32, 41], and quantitatively linking tissue rigidity to cell-cell connectivity and adhesion [29], but not directly to the rigidity of individual cells.
Overall, experimental measurements of several viscoelastic characteristics in cells and tissues have revealed nonlinear changes at the macroscale, e.g., viscosity, yield-stress, elastic modulus that do not trivially match similar changes at the microscale, e.g., individual cell and filaments material properties, strongly supporting that viscoelasticity of living systems is an emergent property.
Several theoretical frameworks have been developed to establish a micro-macro link that can explain cell and tissue viscoelasticity. Numerous models exist in describing complex viscoelastic behaviors in chemical polymers where we recommend to the reader for a more specialized relevant literature [94–96]. Here however, we will summarize experimentally-based models belonging to three categories, based on the abstraction used to represent the biological system: In cytoskeleton networks, the building blocks are the biopolymer filaments and their mechanical properties, in vertex models, the geometrical properties of individual cells tilling the tissue and finally, in the topological models, the local topological arrangements of e.g., cell-cell contacts.
The theoretical modeling of filament networks must account for the particular rheological phenomena these systems show, such as the strain stiffening response, stiffening tunability, and recoverable network fluidization [8, 15, 26, 30, 33, 71, 77, 97–99]. These models consider, at the microscopic level, filament properties like stiffness or length, and the local geometric and topological patterns of cross-linking, which project to the macroscopic level as material properties. Given these parameters, qualitative shifts in the response of the network are expected while increasing the density of filaments: First, beyond a certain threshold of density, the phenomenon of geometric percolation is observed, usually referred to simply as percolation, purely based on the network topology (Box 1). At higher filament density, another qualitative shift in the properties of the network is observed, when the stiffness percolation threshold is overcome (Figure 2A). Beyond this filament density threshold, any stress applied at any point of the network will propagate throughout the whole system, meaning that the Young modulus,
FIGURE 2. Modelling cell and tissue viscoelasticity. (A) Phase diagram of the regimes arising in a topological model for rigidity, such as stiffness percolation, predicting a floppy to rigid transition at a critical point of average connectivity of the underlying network (grey rectangle). A random network displaying average connectivity below the isostatic point (critical point), has a small Giant Rigid Cluster (red) and the whole network is floppy, displaying Young’s modulus
A well accepted model describing the phase space of filament viscoelasticity defined by the parameters of filament density and individual filament properties is the Worm-like Chain (WLC) model [15, 102]. In the WCL model, biopolymers are considered as elastic rods or fibers with finite resistance to bending. Geometrically, such fibers are depicted by an inextensible curve with an energy penalty for bending. Let
The second derivative accounts for the local curvature of the filament. The bending modulus
which corresponds to the persistence length
Experimental work on reconstituted F-actin networks, revealed that linear and nonlinear strain-stress relationships can be explained by entropic and enthalpic models, respectively. In the absence of crosslinkers, actin networks generally form weak elastic gels mimicking the elastic nature of the filaments [106, 107]. At the entropic regime, elasticity comes from the resistance of each polymer/filament against stretching [33, 107]. At the enthalpic regime, while increasing strain, filaments first bend, reorganize along the direction of shear strain and the network deformation arises from the enthalpic stretching of the aligned filaments [108–110]. Further work showed that by decreasing the concentration of cross-linkers the network transits from affine to non-affine [74, 75]. In conclusion, the modeling of biopolymer networks within this framework has proven powerful enough to account for the special viscoelastic properties of these systems.
When modelling tissue viscoelasticity, the fundamental units, cells, are considered to exhibit certain properties [25, 111–117] (Box 1) arising from the cell cytoskeleton [118]. Parameters, such as departure from an ideal cell shape and active fluctuations condense the material response of the cell and its effect to the tissue architecture under stress. There are several abstractions, depending on where the emphasis is placed concerning the energetic cost of the tissue deformations or configurations. Here, we will briefly mention Cellular Potts models and Centroid models, and focus more on the Vertex models (Box 1) which provide a widely applicable framework to the understanding of biological tissue properties.
Cellular Potts models idealize the tissue architecture as a mesh in which each point can be in several states and, accordingly, can represent a part of the cell, a contact point, or a free space. Each state of the mesh point has a particular contribution to the overall energy of the system, and may depend critically on the state of its neighbors [116]. Cellular Potts models may be considered within the much broader family of network models. In turn, centroid models base the analysis on the assumption that cells can be represented by their centroid position within the geometry of the tissue. Energetic contributions are based on geometrical considerations between centroids, such as distance between them [117, 119, 120]. The source of energetic contributions can be considered somehow complementary or even opposed [120] to the one considered in vertex models and the energetic costs associated with different configurations can be associated with material properties of the modeled tissue.
Vertex models have recently received a lot of attention due to their potential in describing a wide range of tissue properties [25, 27, 36, 111–115, 117–119, 121–123]. Moreover, the accurate study of their mathematical properties revealed a plethora of interesting physical properties [27, 28], such as second order rigidity [121], that could play a relevant role in the modeling of biological tissues. Importantly, these properties may imply an interesting departure from the framework proposed by inanimate material science, and proposes a theoretical framework that extends to biological materials. In principle, vertex models have been postulated to model confluent tissues, with recent approaches extending the framework to non-confluent tissues [32]. The essential problem vertex models try to answer is: what are the energetic costs for cell migration within a tissue? In that framework, fluid states are those by which the movement of cells can happen at almost no cost, and rigid states will correspond to those states by which moving a cell - in particular, performing a T1 transition - implies a positive energy penalty, to be paid either in the form of external work or by the cells themselves (Figure 2C). Arguably, the response to an external stress will be, at least partially, driven by the possibility of cell rearrangements which, in turn, depend on the ease of movements of cells within the tissue. The energetic contributions that configure the overall energy of the tissue come from the resistance against compression and the departure from some preferred shape in cells which, in most cases, is introduced as the preferred relation perimeter/area in 2D projections [27, 113, 121, 122]. Having N cells, the functional accounting for the energy of the (2D) system reads:
where
If a T1 transition can be performed at no energy cost, the tissue is in a fluid configuration. Otherwise, the tissue is considered rigid. Remarkably, one can observe a well-defined phase transition from
The presence of interstitial fluid (Box 1) in non-confluent tissues, such as embryonic tissues and tumors [12, 13, 127], opens the possibility to apply even simpler theoretical frameworks to study their viscoelasticity [29]. The reason is that abandoning confluence liberates the system from a lot of implicit constraints at the structural level. Within non-confluent tissues, the range of potential structural patterns increases enormously and topological models can exploit this potential heterogeneity. Here we discuss how topological models can be connected to the rheological properties of non-confluent tissues.
Topological models consider only the structural pattern of connections as the source of the material properties of the tissues. When the system is abstracted at the topological level, its structure is represented by a network defined by nodes and connections among them (Figure 2A). It is important to stress that, at first approximation, no other component, such as link properties or geometric embedding, is considered. The topological analysis, therefore, distills the structure of the system at the level of microscopic minimal components and combinatorial relations among them. In that sense, the basic observables of these models are, for example, the number of links connecting a given node to other nodes of the network, or the existence of paths, within the graph, between a given pair of them. In general, the approximation of a random network, in which the number of connections per node fluctuates stochastically according to some general constraint, quite accurately describes the behavior of real systems --given a suitable choice of constraints [92, 128–131]. For example, in the case that a network is representing the contacts of cells in epithelial (2D) tissues, it must belong to the class of planar networks, namely, those networks that can be extended in a 2D surface without displaying any link overlap [131]. In spite of the apparent simplicity of the approach, the study of networks at the topological level displays a wide range of non-linear phenomena, such as phase transitions or self-organized criticality [92, 129–131]. Particularly relevant is the phenomenon of percolation [91, 92, 128, 129], briefly mentioned in previous sections (Box 1). We will focus on the emergence of the so-called rigid cluster percolation (Box 1), due to its important implications in cells and tissue material properties.
Rigid cluster percolation is based on generic rigidity theory. Given a graph of
A network will be rigid if the constraints absorb all the degrees of freedom for the motion of nodes (Figure 3A). Considering a triangular lattice embedded in a 2D plane, each node has, a priori,
Note that here we consider that all links are responsible for an independent constraint. We must notice that this is an important simplification [51, 132] of the problem, but has proven useful in terms of both simplicity and predictive power. Starting from
that lead us to:
which, in terms of average network connectivity, implies
FIGURE 3. Rigidity percolation in living systems. (A) Schematic illustration of rigidity. A rhombus cluster of four nodes and four links deforms in the presence of a deformation force. Just by the addition of one link that absorbs the remaining degrees of freedom of the central nodes, under the same deformation force it does not deform and it is considered rigid. (B,B’) Rigidity percolation transition in actomyosin crosslinked networks. (B) At low connectivity (left) or low crosslinker concentration the network is mostly disconnected and displays local contractions. At intermediate connectivity (middle) and intermediate crosslinker concentration the network is critically connected. At higher connectivity (right) and high crosslinker concentration the network is fully connected, the Giant Rigid Cluster (GRC, red) emerges and the network displays global contractions and signs of fracture. (B’) Plot of the size of the GRC as a function of crosslinker concentration and thus average connectivity. Depending on the absence or presence of contractility the system can be considered as passive or active respectively, and this changes the transition point. Figure adapted from [43]. (C,C’) Rigidity percolation transition in cellular networks - tissues - of the zebrafish embryonic blastoderm. (C) At low average connectivity (∼3.5 contacts per cell) the GRC (red) is small and the blastoderm is fluidized whereas at higher connectivity (>4 contacts per cell) the GRC is big, occupies almost the whole network and the tissue displays experimentally high viscosity. (C’) Plot of the size of the GRC as a function of cell connectivity. Note that the GRC is emerging sharply at the critical point of connectivity (2/3 of maximum potential connectivity). Figure adapted from [29].
In order to connect rigidity and topology with the material properties, the mechanics of the links, considered as springs, should also be considered. In that context,
In Box 2 we show how the microscopic dynamics of energy dissipation gives rise to macroscopic viscosity. In particular, if
where γ, is the strain and σ, the applied stress (Figure 1B). Eq. 4 is a constitutive equation for a Maxwellian viscoelastic material [14] (Figure 1B). The above equation enables us to identify:
as the viscosity of the system. What is relevant here is that we have a direct relation between the Young’s modulus of the system,
We sketch here, in a very simplified way, the connection between viscoelasticity and the topological property of generic rigidity. We present a version of the Maxwell’s model for viscoelasticity (see Figure 1B), where the particularity comes from the conceptualization of the dashpot, whose role is absorbed by a stochastic resetting of the rest-lengths of the springs forming a network that, in turn, models the structure of the material. This modelization enables the linking of Maxwell’s network rigidity to Maxwell’s theory of viscoelasticity.Let us consider a network of springs, each with elastic constant k. Each spring updates its rest length at random with probability
The whole network has Young’s modulus E. We consider a random lattice arranged in a rectangular form, in a way that the bottom layer is attached to the ground and the upper layer receives the stress
We first consider the elastic component, e.g.:
To compute
Using the expression for
Considering the two contributions, the overall strain evolves according to the following equation:
Which is a constitutive equation for a Maxwellian viscoelastic material [14]. The above equation enables us to identify the viscosity as:
In consequence, qualitative changes in the Young’s modulus of the network will project into qualitative changes in the viscoelastic behaviour of the system. If
since the strain is 0. The solution of the above equation is given by
The above framework is able to bridge, in spite of its simplicity, microscopic topological patterns to macro-structural properties [47, 51], without a priori reference to the mechanical properties of the constituents. These patterns, in turn, can be formally mapped to macroscopic material observables, such as viscoelasticity, hence demonstrating a potentially widely applicable framework to quantitatively link microscopic interactions to macroscopic viscoelasticity across scales.
Recent experimental work indicates that analysis at the purely topological level has the potential to indeed probe viscoelastic properties across scales. These models have been applied to several forms of biological networks, such as the cytoskeleton, ECM and cellular networks–tissues, to probe their material properties [29, 31, 44].
In the case of the cytoskeleton, both experimental and theoretical studies have shown that network connectivity is an essential parameter for cytoskeletal network mechanics [26]. If the actomyosin cytoskeleton is considered as a passive system, then rigidity percolation can predict the elastic modulus of the system based solely on its connectivity. In fact, a phase transition is proposed to occur in such networks, where mechanical rigidity emerges at the isostatic point --see Eq. 3 [31] (Figures 3B,B’). Such a framework can be expanded to active systems, where network connectivity together with motor activity can be further used as parameters to predict the contractile behavior of the actin cytoskeleton. Experiments on actin gels, where connectivity is regulated by the density of fascin crosslinkers and the motor activity by the density of myosin, showed characteristics of a rigidity percolation transition [43]. Briefly, weakly crosslinked networks (low connectivity) showed local contractions, medially crosslinked networks (higher connectivity) formed distinct contractile clusters within the network with a certain rigid cluster size distribution and, strongly crosslinked networks (highest connectivity) exhibited global network contraction associated with network fracture [43] (Figure 3B). The authors further propose that the motors have the ability to reduce connectivity via forcing the crosslinkers to unbind, in order to avoid network fracture and thus the interplay of motor activity and crosslinking drives active gels to a critically connected state that can balance between local and global contractions [43].
Rigidity properties of ECM networks and, in particular, type I collagen fiber networks, have also been well-described by rigidity percolation theory [134]. Studies combining experiments and theory suggest that the shear modulus of collagen fibers shows a strong correlation with the collagen volume fraction, and that these networks display connectivity near the percolation threshold [134–138]. Further experimental work however, has revealed that collagen networks with connectivity slightly below the isostatic threshold, can also become rigid in the presence of large deformation instead, thus in such cases passive rigidity percolation may not be sufficient to explain ECM rigidity [44, 85]. In particular, increasing shear deformation in sub-isostatic networks leads to nonlinear increase of the elastic modulus of such networks along different connectivity values, an observation highlighting the possibility of incorporating the active nature of such systems when applying rigidity percolation theory [139].
Recently, the concept of rigidity percolation has been applied in non-confluent embryonic tissues to map tissue rigidity/viscosity (Box 2). Although tissues do not form physically crosslinked networks as the cytoskeleton or ECM, they can be approached as “cellular networks”, where the nodes are the cells and the connecting links the adherens-junctions (Figure 3C) [140]. This theory was applied to the zebrafish blastoderm which undergoes an abrupt and dramatic loss in viscosity at the onset of morphogenesis [13, 29]. These changes in blastoderm viscosity were probed via rigidity percolation analysis over cell contact networks of the blastoderm. The size of the GRC was analyzed as a function of connectivity, and it was found that the GRC size correlates with the experimentally observed changes in tissue viscosity [29] (Figure 3C). The emergence and disappearance of the GRC around the critical point matched the empirical observations where embryos whose cell contact network displays an average connectivity below the critical point, display a small GRC and are fluidized, whereas embryos whose network displays an average connectivity above the critical point display a big GRC and are rigid (Figure 3C). This work further traced hallmarks of phase transitions, such as the diverge of macroscopic observables and its critical exponents at criticality, showing that rigidity percolation theory can be applied in embryonic tissues in vivo to link macroscopic tissue rigidity to the microscopic cell connectivity of these tissues [13, 29].
Living cells and tissues behave like viscoelastic materials [20, 141], a long-standing observation that has only recently been linked to cell and tissue physiology [2–5]. Spatiotemporal regulation of viscoelasticity has been shown to influence essential biological processes, such as cell motility, proliferation, wound healing and the morphogenetic processes of body axis elongation and tissue spreading during embryonic development [11–13, 86, 88, 142–144]. Tracing the microscopic regulators of viscoelasticity is, however, a challenging task: typically, the mechanical properties of these microscopic building blocks do not match trivially the emerging viscoelastic behavior of cells and tissues at the macroscopic scale. Among many examples, we find the nonlinear increase of the elastic modulus of cytoskeletal and fiber networks in response to strain, or abrupt drastic changes in tissue viscosity without associated mechanical changes at the cellular level [12, 29, 30, 33]. Given that the mechanical properties of tissues are regulated at the microscopic level, e.g., from the properties of the microscopic building blocks and their interactions, quantitatively bridging the microscale to macroscale is fundamental in order to understand the emergence of viscoelasticity [145]. Several theoretical approaches shed light on the biological mechanisms by which viscoelasticity can emerge in a system. However, the application of such theories is still far from comprehensive, given several challenges --such as the active, non-equilibrium nature of living systems.
At the cellular scale, the viscoelasticity of networked biopolymer filaments forming the cytoskeleton and ECM is most frequently modeled based on the mechanics, geometric alignment and local topology of the biopolymer fibers. It is worth mentioning that most of the research activity was performed in networks composed of actin or microtubules or intermediate filaments. In fact, biopolymer networks show a much wider heterogeneity, since the cytoskeleton is a dynamic mixture of all the cytoskeletal elements, interacting with each other, and these interactions were shown to affect their mechanical properties. Percolating networks of actin and vimentin for example, display synergistic effects in the elastic modulus, which becomes much greater than the sum of the elastic moduli of the two networks alone [146]. Another synergistic effect on actin and IFs networks is the recently reported phenomenon of super-elasticity, observed during epithelial morphogenesis [147]. Similarly, actin and MTs composite networks were shown to exhibit reinforcement against compression and mechanical enhancement [148–150], and a similar phenomenon was also observed when compressing composite ECM networks of collagen and hyaluronan [151, 152]. Future experimental and theoretical work on composite networks is expected to provide a more complete understanding of cell and ECM viscoelasticity.
At the tissue scale, the vertex models are widely used to represent tissue viscoelasticity and have the special advantage of collapsing the emergence of macroscopic properties on a single cellular parameter, such as cell shape, and its associated scalar parameters, such as compressibility or departure from preferred area/perimeter [27, 28]. Since, in a tilling of cells, deformation can only arise through cell-cell rearrangements, vertex models mostly focus the analysis on the study of the energy penalties associated with these cell-cell rearrangements. Such rearrangements can for example be induced by differences in cell-cell adhesion and cortical tension or active tension fluctuations [27, 28, 32, 125]. It is implicitly postulated that the results at that level of abstraction will project into the material properties of the system. Even though numerical approaches are coherent with the predictions of the models, a direct bridge between micro and macro scales in tissue viscoelasticity has yet to be clearly described. The recent application of rigidity percolation in tissues however, provides a quantitative link between the topological patterns of cell-cell contacts and tissue rigidity regime (quantified by the size of the GRC). In the case of the zebrafish blastoderm, the topological approach of rigidity percolation was sufficient to capture the floppy and rigid regimes of the tissue by one single (microscopic) measurable parameter, the average number of cell-cell contacts in different cell types and, as a result, match the observed (macroscopic) changes in viscoelasticity [29]. Cell connectivity was further shown to be defined by the biophysics of cell-cell contacts and specifically the cell-cell interfacial tension at the contact. In this biological context, experimental testing of the phase transition parameters revealed that changes in connectivity and cell-cell adhesion were driven by cell division, and not by cell rearrangements, cell shape or active tension fluctuations [29]. Since cell-cell adhesion is key in tissue rigidity theoretical frameworks so far, we speculate that some parameters may be common, such as cell-cell adhesion strength [29, 32, 118, 123], and some others may be context-specific such as cell division and rearrangements. Future experimental work has the potential to disentangle the physiological role of several cellular parameters in rigidity transitions.
In all of the above models, incorporating detailed dynamic analyses of the microscopic parameters that can account for local heterogeneities, such as in adhesion strength (between the cells and with their environment), shape differences or heterogeneous motility patterns, will increase their potential to model absolute viscosity values and provide a more accurate and representative image of tissue viscoelasticity. A key challenge is the choice of the viscoelastic model relevant to the biological system. Here, we have extensively described how Maxwell viscoelasticity has the potential to be linked to Maxwell rigidity. However, other biological systems may be better described with different models. For example, the Kelvin model was recently used to describe the phenomenon of arrested coalescence in multicellular aggregates from the adherent and contractile protrusion interactions between the cells [153]. In addition, both Maxwell and Kelvin viscoelastic models can describe different viscoelastic regimes during density/packing dependent collective cell migration [154]. Another important challenge is to understand if and how the timescale of a biological process taking place at the microscale is relevant to the timescale of a biological process taking place at the macroscale when bridging scales in viscoelasticity. For example, how macromolecular motion within the cytoskeleton (milliseconds to seconds) influences cell shape changes driven by the mechanics of the cytoskeleton (seconds to minutes), or how cellular motion within a tissue (minutes) influences tissue-scale fluidization (minutes) and spreading (hours)? Several theoretical and experimental frameworks should be developed do bridge scales in time and space [142, 155].
Disentangling the connection between microscale behavior and emerging, macroscopic properties is not a novel goal: it has been the long-sought target of statistical mechanics in order to connect thermodynamics to a solid microscopic basis [156]. Moving towards a broader conception of statistical mechanics encompassing the living phenomena requires the introduction of the microscopic role of the biological building blocks - which are far more complex than gas particles, for example. Nevertheless, establishing the critical point in the microscopic dynamics of the building blocks that would trigger a macroscopic phase transition would create a rich toolbox for biology, regardless of the theoretical approach being used. Phase diagrams or morphospaces, accounting for what is possible in the relations between tissue organization and material properties, can be defined that will allow further exploration for the different regimes the system can occupy and their grounding. In the case of the zebrafish blastoderm, for example, it was experimentally possible to position the system in the vicinity of criticality. Hallmarks of criticality, such as divergence of macroscopic observables with associated power laws, were determined experimentally in the living embryo, showing that tissue morphogenesis in vertebrates may start close to a critical point of a rigidity transition. This indicates that embryonic tissues may be at optimal fitness [157] since they are able to easily switch between rigid and floppy regimes by slightly changing their connectivity at almost zero energetic cost [29]. Through this lens, one can explore fundamental questions concerning morphogenesis. How do local heterogeneities in the microscopic parameters influence the emergence of macroscopic viscoelasticity? How do noisy biological systems [158–160] guarantee stability during development when they are poised at criticality?
Networked systems and criticality have been for long used to understand an extremely rich palette of macroscopic phenomena occurring in the natural world [92, 131, 161, 162], that now include the viscoelastic characteristics of biological systems, from the nanometer scale of the cytoskeleton to the micrometer scale of tissues and embryos. Such observations indicate that an efficient organizing strategy of complex biological systems may be to behave as networked systems close to criticality.
BC-M and NP designed, drafted, revised and finalised the work.
The authors declare that the research was conducted in the absence of any commercial or financial relationships that could be construed as a potential conflict of interest.
We thank Preeti Sahu and Edouard Hannezo for critical discussion on the topic and Anna Erzberger and Gautam Dey for feedback on the manuscript.
1. Huber F, Schnauß J, Rönicke S, Rauch P, Müller K, Fütterer C. Emergent Complexity of the Cytoskeleton: from Single Filaments to Tissue. Adv Phys (2013) 62(1):1–112. doi:10.1080/00018732.2013.771509
2. Barriga EH, Mayor R. Adjustable Viscoelasticity Allows for Efficient Collective Cell Migration. Semin Cell Dev Biol (2019) 93:55–68. doi:10.1016/j.semcdb.2018.05.027
3. Burla F, Mulla Y, Vos BE, Aufderhorst-Roberts A, Koenderink GH. From Mechanical Resilience to Active Material Properties in Biopolymer Networks. Nat Rev Phys (2019) 1(4):249–63. doi:10.1038/s42254-019-0036-4
4. Petridou NI, Heisenberg CP. Tissue Rheology in Embryonic Organization. EMBO J (2019) 38(20):e102497. doi:10.15252/embj.2019102497
5. Chaudhuri O, Cooper-White J, Janmey PA, Mooney DJ, Shenoy VB. Effects of Extracellular Matrix Viscoelasticity on Cellular Behaviour. Nature (2020) 584(7822):535–46. doi:10.1038/s41586-020-2612-2
6. Lecuit T, Lenne P-F, Munro E. Force Generation, Transmission, and Integration during Cell and Tissue Morphogenesis. Annu Rev Cell Dev Biol (2011) 27:157–84. doi:10.1146/annurev-cellbio-100109-104027
7. Clément R, Dehapiot B, Collinet C, Lecuit T, Lenne P-F. Viscoelastic Dissipation Stabilizes Cell Shape Changes during Tissue Morphogenesis. Curr Biol (2017) 27(20):3132–42.e3134. doi:10.1016/j.cub.2017.09.005
8. Pegoraro AF, Janmey P, Weitz DA. Mechanical Properties of the Cytoskeleton and Cells. Cold Spring Harb Perspect Biol (2017) 9(11):a022038. doi:10.1101/cshperspect.a022038
9. Campàs O. A Toolbox to Explore the Mechanics of Living Embryonic Tissues. Semin Cell Dev Biol (2016) 55:119–30. doi:10.1016/j.semcdb.2016.03.011
10. Gómez-González M, Latorre E, Arroyo M, Trepat X. Measuring Mechanical Stress in Living Tissues. Nat Rev Phys (2020) 2(6):300–17. doi:10.1038/s42254-020-0184-6
11. Barriga EH, Franze K, Charras G, Mayor R. Tissue Stiffening Coordinates Morphogenesis by Triggering Collective Cell Migration In Vivo. Nature (2018) 554(7693):523–7. doi:10.1038/nature25742
12. Mongera A, Rowghanian P, Gustafson HJ, Shelton E, Kealhofer DA, Carn EK. A Fluid-To-Solid Jamming Transition Underlies Vertebrate Body axis Elongation. Nature (2018) 561(7723):401–5. doi:10.1038/s41586-018-0479-2
13. Petridou NI, Grigolon S, Salbreux G, Hannezo E, Heisenberg C-P. Fluidization-mediated Tissue Spreading by Mitotic Cell Rounding and Non-canonical Wnt Signalling. Nat Cell Biol (2019) 21(2):169–78. doi:10.1038/s41556-018-0247-4
14. Findley WN, Lai JS, Onaran K. Creep and Relaxation of Nonlinear Viscoelastic Materials. Amsterdam: Elsevier (1989).
15. Broedersz CP, MacKintosh FC. Modeling Semiflexible Polymer Networks. Rev Mod Phys (2014) 86(3):995–1036. doi:10.1103/RevModPhys.86.995
16. Özkaya N, Nordin M. Mechanical Properties of Biological Tissues. Editor Nordin M. In: Fundamentals of Biomechanics. New York: Springer (1999).
17. Gutierrez-Lemini D. Engineering Viscoelasticity. New York: Springer (2014). doi:10.1007/978-1-4614-8139-3
18. Serwane F, Mongera A, Rowghanian P, Kealhofer DA, Lucio AA, Hockenbery ZM. In Vivo quantification of Spatially Varying Mechanical Properties in Developing Tissues. Nat Methods (2017) 14(2):181–6. doi:10.1038/nmeth.4101
19. D'Angelo A, Dierkes K, Carolis C, Salbreux G, Solon J. In Vivo Force Application Reveals a Fast Tissue Softening and External Friction Increase during Early Embryogenesis. Curr Biol (2019) 29(9):1564–71.e6. doi:10.1016/j.cub.2019.04.010
20. Forgacs G, Foty RA, Shafrir Y, Steinberg MS. Viscoelastic Properties of Living Embryonic Tissues: a Quantitative Study. Biophysical J (1998) 74(5):2227–34. doi:10.1016/S0006-3495(98)77932-9
21. Bonn D, Denn MM, Berthier L, Divoux T, Manneville S. Yield Stress Materials in Soft Condensed Matter. Rev Mod Phys (2017) 89(3):035005. doi:10.1103/RevModPhys.89.035005
22. Davidson LA. Embryo Mechanics. Curr Top Dev Biol (2011) 95:215–41. doi:10.1016/b978-0-12-385065-2.00007-4
23. Mongera A, Pochitaloff M, Gustafson HJ, Stooke-Vaughan GA, Rowghanian P, Campàs O. Mechanics of the Cellular Microenvironment as Perceived by Cells <em>In Vivo</em>. bioRxiv (2021). 2021.2001.2004.425259. doi:10.1101/2021.01.04.425259
24. Kollmannsberger P, Fabry B. Linear and Nonlinear Rheology of Living Cells. Annu Rev Mater Res (2011) 41(1):75–97. doi:10.1146/annurev-matsci-062910-100351
25. Farhadifar R, Röper J-C, Aigouy B, Eaton S, Jülicher F. The Influence of Cell Mechanics, Cell-Cell Interactions, and Proliferation on Epithelial Packing. Curr Biol (2007) 17(24):2095–104. doi:10.1016/j.cub.2007.11.049
26. Pritchard RH, Shery Huang YY, Terentjev EM. Mechanics of Biological Networks: from the Cell Cytoskeleton to Connective Tissue. Soft Matter (2014) 10(12):1864–84. doi:10.1039/C3SM52769G
27. Bi D, Lopez JH, Schwarz JM, Manning ML. A Density-independent Rigidity Transition in Biological Tissues. Nat Phys (2015) 11(12):1074–9. doi:10.1038/nphys3471
28. Bi D, Yang X, Marchetti MC, Manning ML. Motility-driven Glass and Jamming Transitions in Biological Tissues. Phys Rev X (2016) 6(2). doi:10.1103/PhysRevX.6.021011
29. Petridou NI, Corominas-Murtra B, Heisenberg C-P, Hannezo E. Rigidity Percolation Uncovers a Structural Basis for Embryonic Tissue Phase Transitions. Cell (2021) 184(7):1914–28.e19. doi:10.1016/j.cell.2021.02.017
30. Gardel ML, Shin JH, MacKintosh FC, Mahadevan L, Matsudaira P, Weitz DA. Elastic Behavior of Cross-Linked and Bundled Actin Networks. Science (2004) 304(5675):1301–5. doi:10.1126/science.1095087
31. Alvarado J, Sheinman M, Sharma A, MacKintosh FC, Koenderink GH. Force Percolation of Contractile Active Gels. Soft Matter (2017) 13(34):5624–44. doi:10.1039/c7sm00834a
32. Kim S, Pochitaloff M, Stooke-Vaughan GA, Campàs O. Embryonic Tissues as Active Foams. Nat Phys (2021). doi:10.1038/s41567-021-01215-1
33. MacKintosh FC, Käs J, Janmey PA. Elasticity of Semiflexible Biopolymer Networks. Phys Rev Lett (1995) 75(24):4425–8. doi:10.1103/PhysRevLett.75.4425
34. Gardel ML, Nakamura F, Hartwig JH, Crocker JC, Stossel TP, Weitz DA. Prestressed F-Actin Networks Cross-Linked by Hinged Filamins Replicate Mechanical Properties of Cells. Proc Natl Acad Sci (2006) 103(6):1762–7. doi:10.1073/pnas.0504777103
35. Wen Q, Janmey PA. Polymer Physics of the Cytoskeleton. Curr Opin Solid State Mater Sci (2011) 15(5):177–82. doi:10.1016/j.cossms.2011.05.002
36. Fletcher AG, Osterfield M, Baker RE, Shvartsman SY. Vertex Models of Epithelial Morphogenesis. Biophysical J (2014) 106(11):2291–304. doi:10.1016/j.bpj.2013.11.4498
37. Alt S, Ganguly P, Salbreux G. Vertex Models: from Cell Mechanics to Tissue Morphogenesis. Phil Trans R Soc B (2017) 372:20150520. doi:10.1098/rstb.2015.0520
38. Angelini TE, Hannezo E, Trepat X, Marquez M, Fredberg JJ, Weitz DA. Glass-like Dynamics of Collective Cell Migration. Proc Natl Acad Sci (2011) 108(12):4714–9. doi:10.1073/pnas.1010059108
39. Sadati M, Taheri Qazvini N, Krishnan R, Park CY, Fredberg JJ. Collective Migration and Cell Jamming. Differentiation (2013) 86(3):121–5. doi:10.1016/j.diff.2013.02.005
40. Park J-A, Kim JH, Bi D, Mitchel JA, Qazvini NT, Tantisira K. Unjamming and Cell Shape in the Asthmatic Airway Epithelium. Nat Mater (2015) 14(10):1040–8. doi:10.1038/nmat4357
41. Merkel M, Manning ML. A Geometrically Controlled Rigidity Transition in a Model for Confluent 3D Tissues. New J Phys (2018) 20(2):022002. doi:10.1088/1367-2630/aaaa13
42. Atia L, Bi D, Sharma Y, Mitchel JA, Gweon B, A. Koehler S. Geometric Constraints during Epithelial Jamming. Nat Phys (2018) 14:613–20. doi:10.1038/s41567-018-0089-9
43. Alvarado J, Sheinman M, Sharma A, MacKintosh FC, Koenderink GH. Molecular Motors Robustly Drive Active Gels to a Critically Connected State. Nat Phys (2013) 9(9):591–7. doi:10.1038/nphys2715
44. Sharma A, Licup AJ, Jansen KA, Rens R, Sheinman M, Koenderink GH. Strain-controlled Criticality Governs the Nonlinear Mechanics of Fibre Networks. Nat Phys (2016) 12(6):584–7. doi:10.1038/nphys3628
45. Hunt A, Ewing R, Ghanbarian B. Percolation Theory: Topology and Structure. Cham: Springer (2014). doi:10.1007/978-3-319-03771-4
46. Thorpe MF. Continuous Deformations in Random Networks. J Non-Crystalline Sol (1983) 57(3):355–70. doi:10.1016/0022-3093(83)90424-6
47. Jacobs DJ, Thorpe MF. Generic Rigidity Percolation: The Pebble Game. Phys Rev Lett (1995) 75(22):4051–4. doi:10.1103/PhysRevLett.75.4051
48. Jacobs DJ, Thorpe MF. Generic Rigidity Percolation in Two Dimensions. Phys Rev E (1996) 53(4):3682–93. doi:10.1103/PhysRevE.53.3682
49. Alexander S. Amorphous Solids: Their Structure, Lattice Dynamics and Elasticity. Phys Rep (1998) 296(2):65–236. doi:10.1016/S0370-1573(97)00069-0
50. Thorpe MF, Jacobs DJ, Chubynsky MV, Phillips JC. Self-organization in Network Glasses. J Non-Crystalline Sol (2000) 266-269:859–66. doi:10.1016/S0022-3093(99)00856-X
51. Chubynsky MV, Thorpe MF. Algorithms for Three-Dimensional Rigidity Analysis and a First-Order Percolation Transition. Phys Rev E (2007) 76(4). doi:10.1103/PhysRevE.76.041135
52. Wyart M, Liang H, Kabla A, Mahadevan L. Elasticity of Floppy and Stiff Random Networks. Phys Rev Lett (2008) 101(21):215501. doi:10.1103/PhysRevLett.101.215501
53. Broedersz CP, Depken M, Yao NY, Pollak MR, Weitz DA, MacKintosh FC. Cross-link-governed Dynamics of Biopolymer Networks. Phys Rev Lett (2010) 105(23):238101. doi:10.1103/PhysRevLett.105.238101
54. Tighe BP. Relaxations and Rheology Near Jamming. Phys Rev Lett (2011) 107(15):158303. doi:10.1103/PhysRevLett.107.158303
55. Driscoll MM, Chen BG-g., Beuman TH, Ulrich S, Nagel SR, Vitelli V. The Role of Rigidity in Controlling Material Failure. Proc Natl Acad Sci USA (2016) 113(39):10813–7. doi:10.1073/pnas.1501169113
56. Bouzid M, Del Gado E. Network Topology in Soft Gels: Hardening and Softening Materials. Langmuir (2018) 34(3):773–81. doi:10.1021/acs.langmuir.7b02944
57. Fletcher DA, Mullins RD. Cell Mechanics and the Cytoskeleton. Nature (2010) 463(7280):485–92. doi:10.1038/nature08908
58. Shamipour S, Caballero-Mancebo S, Heisenberg C-P. Cytoplasm's Got Moves. Dev Cell (2021) 56(2):213–26. doi:10.1016/j.devcel.2020.12.002
59. Trickey WR, Vail TP, Guilak F. The Role of the Cytoskeleton in the Viscoelastic Properties of Human Articular Chondrocytes. J Orthop Res (2004) 22(1):131–9. doi:10.1016/s0736-0266(03)00150-510.1016/s0736-0266(03)0150-5
60. Bonakdar N, Gerum R, Kuhn M, Spörrer M, Lippert A, Schneider W. Mechanical Plasticity of Cells. Nat Mater (2016) 15(10):1090–4. doi:10.1038/nmat4689
61. de Gennes PG. Reptation of a Polymer Chain in the Presence of Fixed Obstacles. J Chem Phys (1971) 55(2):572–9. doi:10.1063/1.1675789
62. Lin Y-C, Broedersz CP, Rowat AC, Wedig T, Herrmann H, MacKintosh FC. Divalent Cations Crosslink Vimentin Intermediate Filament Tail Domains to Regulate Network Mechanics. J Mol Biol (2010) 399(4):637–44. doi:10.1016/j.jmb.2010.04.054
63. Blanchoin L, Boujemaa-Paterski R, Sykes C, Plastino J. Actin Dynamics, Architecture, and Mechanics in Cell Motility. Physiol Rev (2014) 94(1):235–63. doi:10.1152/physrev.00018.2013
64. Basnet N, Nedozralova H, Crevenna AH, Bodakuntla S, Schlichthaerle T, Taschner M. Direct Induction of Microtubule Branching by Microtubule Nucleation Factor SSNA1. Nat Cell Biol (2018) 20(10):1172–80. doi:10.1038/s41556-018-0199-8
65. Lieleg O, Claessens MMAE, Bausch AR. Structure and Dynamics of Cross-Linked Actin Networks. Soft Matter (2010) 6(2):218–25. doi:10.1039/B912163N
66. Fabry B, Maksym GN, Butler JP, Glogauer M, Navajas D, Fredberg JJ. Scaling the Microrheology of Living Cells. Phys Rev Lett (2001) 87(14):148102. doi:10.1103/PhysRevLett.87.148102
67. Chen DTN, Wen Q, Janmey PA, Crocker JC, Yodh AG. Rheology of Soft Materials. Annu Rev Condens Matter Phys (2010) 1(1):301–22. doi:10.1146/annurev-conmatphys-070909-104120
68. Schopferer M, Bär H, Hochstein B, Sharma S, Mücke N, Herrmann H. Desmin and Vimentin Intermediate Filament Networks: Their Viscoelastic Properties Investigated by Mechanical Rheometry. J Mol Biol (2009) 388(1):133–43. doi:10.1016/j.jmb.2009.03.005
69. Beck R, Deek J, Choi MC, Ikawa T, Watanabe O, Frey E. Unconventional Salt Trend from Soft to Stiff in Single Neurofilament Biopolymers. Langmuir (2010) 26(24):18595–9. doi:10.1021/la103655x
70. Gittes F, Mickey B, Nettleton J, Howard J. Flexural Rigidity of Microtubules and Actin Filaments Measured from thermal Fluctuations in Shape. J Cell Biol (1993) 120(4):923–34. doi:10.1083/jcb.120.4.923
71. Storm C, Pastore JJ, MacKintosh FC, Lubensky TC, Janmey PA. Nonlinear Elasticity in Biological Gels. Nature (2005) 435(7039):191–4. doi:10.1038/nature03521
72. van Oosten ASG, Vahabi M, Licup AJ, Sharma A, Galie PA, MacKintosh FC. Uncoupling Shear and Uniaxial Elastic Moduli of Semiflexible Biopolymer Networks: Compression-Softening and Stretch-Stiffening. Sci Rep (2016) 6(1):19270. doi:10.1038/srep19270
73. Wen Q, Janmey PA. Effects of Non-linearity on Cell-ECM Interactions. Exp Cell Res (2013) 319(16):2481–9. doi:10.1016/j.yexcr.2013.05.017
74. Mizuno D, Tardin C, Schmidt CF, MacKintosh FC. Nonequilibrium Mechanics of Active Cytoskeletal Networks. Science (2007) 315(5810):370–3. doi:10.1126/science.1134404
75. Koenderink GH, Dogic Z, Nakamura F, Bendix PM, MacKintosh FC, Hartwig JH. An Active Biopolymer Network Controlled by Molecular Motors. Proc Natl Acad Sci (2009) 106(36):15192–7. doi:10.1073/pnas.0903974106
76. Xu S, W. Reinhardt H. Crack Extension Resistance and Fracture Properties of Quasi-Brittle Softening Materials like Concrete Based on the Complete Process of Fracture. Int J Fracture (1998) 92(1):71–99. doi:10.1023/A:1007553012684
77. Chaudhuri O, Parekh SH, Fletcher DA. Reversible Stress Softening of Actin Networks. Nature (2007) 445(7125):295–8. doi:10.1038/nature05459
78. Lin Y-C, Koenderink GH, MacKintosh FC, Weitz DA. Viscoelastic Properties of Microtubule Networks. Macromolecules (2007) 40(21):7714–20. doi:10.1021/ma070862l
79. Rozario T, Dzamba B, Weber GF, Davidson LA, DeSimone DW. The Physical State of Fibronectin Matrix Differentially Regulates Morphogenetic Movements In Vivo. Dev Biol (2009) 327(2):386–98. doi:10.1016/j.ydbio.2008.12.025
80. Rozario T, DeSimone DW. The Extracellular Matrix in Development and Morphogenesis: a Dynamic View. Dev Biol (2010) 341(1):126–40. doi:10.1016/j.ydbio.2009.10.026
81. Licup AJ, Münster S, Sharma A, Sheinman M, Jawerth LM, Fabry B. Stress Controls the Mechanics of Collagen Networks. Proc Natl Acad Sci USA (2015) 112(31):9573–8. doi:10.1073/pnas.1504258112
82. Berezney JP, Saleh OA. Electrostatic Effects on the Conformation and Elasticity of Hyaluronic Acid, a Moderately Flexible Polyelectrolyte. Macromolecules (2017) 50(3):1085–9. doi:10.1021/acs.macromol.6b02166
83. Muiznieks LD, Keeley FW. Molecular Assembly and Mechanical Properties of the Extracellular Matrix: A Fibrous Protein Perspective. Biochim Biophys Acta (Bba) - Mol Basis Dis (2013) 1832(7):866–75. doi:10.1016/j.bbadis.2012.11.022
84. Han YL, Ronceray P, Xu G, Malandrino A, Kamm RD, Lenz M. Cell Contraction Induces Long-Ranged Stress Stiffening in the Extracellular Matrix. Proc Natl Acad Sci USA (2018) 115(16):4075–80. doi:10.1073/pnas.1722619115
85. Jansen KA, Licup AJ, Sharma A, Rens R, MacKintosh FC, Koenderink GH. The Role of Network Architecture in Collagen Mechanics. Biophysical J (2018) 114(11):2665–78. doi:10.1016/j.bpj.2018.04.043
86. Iyer KV, Piscitello-Gómez R, Paijmans J, Jülicher F, Eaton S. Epithelial Viscoelasticity Is Regulated by Mechanosensitive E-Cadherin Turnover. Curr Biol (2019) 29(4):578–91.e575. doi:10.1016/j.cub.2019.01.021
87. Khalilgharibi N, Fouchard J, Asadipour N, Barrientos R, Duda M, Bonfanti A. Stress Relaxation in Epithelial Monolayers Is Controlled by the Actomyosin Cortex. Nat Phys (2019) 15(8):839–47. doi:10.1038/s41567-019-0516-6
88. Tetley RJ, Staddon MF, Heller D, Hoppe A, Banerjee S, Mao Y. Tissue Fluidity Promotes Epithelial Wound Healing. Nat Phys (2019) 15(11):1195–203. doi:10.1038/s41567-019-0618-1
89. Cavanaugh KE, Staddon MF, Banerjee S, Gardel ML. Adaptive Viscoelasticity of Epithelial Cell Junctions: from Models to Methods. Curr Opin Genet Dev (2020) 63:86–94. doi:10.1016/j.gde.2020.05.018
90. Stanley HE. Introduction to Phase Transitions and Critical Phenomena. New York: Oxford University Press (1971).
92. Solé R. Phase Transitions. Princeton, NJ: Princeton University Press (2011). doi:10.1515/9781400838929
93. Jain A, Ulman V, Mukherjee A, Prakash M, Cuenca MB, Pimpale LG. Regionalized Tissue Fluidization Is Required for Epithelial gap Closure during Insect Gastrulation. Nat Commun (2020) 11(1):5604. doi:10.1038/s41467-020-19356-x
94. Rubinstein M, Colby RH, Dobrynin AV, Joanny J-F. Elastic Modulus and Equilibrium Swelling of Polyelectrolyte Gels. Macromolecules (1996) 29(1):398–406. doi:10.1021/ma9511917
95. Gurtovenko AA, Gotlib YY. Dynamics of Inhomogeneous Cross-Linked Polymers Consisting of Domains of Different Sizes. J Chem Phys (2001) 115(14):6785–93. doi:10.1063/1.1401819
96. Müller P. Critical Behaviour of the Rouse Model for Gelling Polymers. J Phys A: Math Gen (2003) 36(42):10443–50. doi:10.1088/0305-4470/36/42/002
97. Janmey PA, McCormick ME, Rammensee S, Leight JL, Georges PC, MacKintosh FC. Negative normal Stress in Semiflexible Biopolymer Gels. Nat Mater (2007) 6(1):48–51. doi:10.1038/nmat1810
98. Lieleg O, Bausch AR. Cross-Linker Unbinding and Self-Similarity in Bundled Cytoskeletal Networks. Phys Rev Lett (2007) 99(15):158105. doi:10.1103/PhysRevLett.99.158105
99. Trepat X, Deng L, An SS, Navajas D, Tschumperlin DJ, Gerthoffer WT. Universal Physical Responses to Stretch in the Living Cell. Nature (2007) 447(7144):592–5. doi:10.1038/nature05824
100. Zaccone A, Scossa-Romano E. Approximate Analytical Description of the Nonaffine Response of Amorphous Solids. Phys Rev B (2011) 83(18):184205. doi:10.1103/PhysRevB.83.184205
101. Laman G. On Graphs and Rigidity of Plane Skeletal Structures. J Eng Math (1970) 4(4):331–40. doi:10.1007/BF01534980
102. Kratky O, Porod G. Röntgenuntersuchung Gelöster Fadenmoleküle. Recl Trav Chim Pays-bas (1949) 68(12):1106–22. doi:10.1002/recl.19490681203
104. Tharmann R, Claessens MMAE, Bausch AR. Viscoelasticity of Isotropically Cross-Linked Actin Networks. Phys Rev Lett (2007) 98(8):088103. doi:10.1103/PhysRevLett.98.088103
105. Wagner B, Tharmann R, Haase I, Fischer M, Bausch AR. Cytoskeletal Polymer Networks: The Molecular Structure of Cross-Linkers Determines Macroscopic Properties. Proc Natl Acad Sci (2006) 103(38):13974–8. doi:10.1073/pnas.0510190103
106. Janmey PA, Peetermans J, Zaner KS, Stossel TP, Tanaka T. Structure and Mobility of Actin Filaments as Measured by Quasielastic Light Scattering, Viscometry, and Electron Microscopy. J Biol Chem (1986) 261(18):8357–62. doi:10.1016/s0021-9258(19)83920-0
107. Hinner B, Tempel M, Sackmann E, Kroy K, Frey E. Entanglement, Elasticity, and Viscous Relaxation of Actin Solutions. Phys Rev Lett (1998) 81(12):2614–7. doi:10.1103/PhysRevLett.81.2614
108. Onck PR, Koeman T, van Dillen T, van der Giessen E. Alternative Explanation of Stiffening in Cross-Linked Semiflexible Networks. Phys Rev Lett (2005) 95(17):178102. doi:10.1103/PhysRevLett.95.178102
109. van Dillen T, Onck PR, Van der Giessen E. Models for Stiffening in Cross-Linked Biopolymer Networks: A Comparative Study. J Mech Phys Sol (2008) 56(6):2240–64. doi:10.1016/j.jmps.2008.01.007
110. Žagar G, Onck PR, Van der Giessen E. Elasticity of Rigidly Cross-Linked Networks of Athermal Filaments. Macromolecules (2011) 44(17):7026–33. doi:10.1021/ma201257v
111. Nagai T, Honda H. A Dynamic Cell Model for the Formation of Epithelial Tissues. Philosophical Mag B (2001) 81(7):699–719. doi:10.1080/13642810108205772
112. Hilgenfeldt S, Erisken S, Carthew RW. Physical Modeling of Cell Geometric Order in an Epithelial Tissue. Proc Natl Acad Sci (2008) 105(3):907–11. doi:10.1073/pnas.0711077105
113. Staple DB, Farhadifar R, Röper J-C, Aigouy B, Eaton S, Jülicher F. Mechanics and Remodelling of Cell Packings in Epithelia. Eur Phys J E (2010) 33(2):117–27. doi:10.1140/epje/i2010-10677-0
114. Chiou KK, Hufnagel L, Shraiman BI. Mechanical Stress Inference for Two Dimensional Cell Arrays. PLoS Comput Biol (2012) 8(5):e1002512. doi:10.1371/journal.pcbi.1002512
115. Wang G, Manning ML, Amack JD. Regional Cell Shape Changes Control Form and Function of Kupffer's Vesicle in the Zebrafish Embryo. Dev Biol (2012) 370(1):52–62. doi:10.1016/j.ydbio.2012.07.019
116. Vroomans RMA, Hogeweg P, ten Tusscher KHWJ. Segment-Specific Adhesion as a Driver of Convergent Extension. PLoS Comput Biol (2015) 11(2):e1004092. doi:10.1371/journal.pcbi.1004092
117. Marin-Riera M, Brun-Usan M, Zimm R, Välikangas T, Salazar-Ciudad I. Computational Modeling of Development by Epithelia, Mesenchyme and Their Interactions: a Unified Model. Bioinformatics (2016) 32(2):219–25. doi:10.1093/bioinformatics/btv527
118. Manning ML, Foty RA, Steinberg MS, Schoetz E-M. Coaction of Intercellular Adhesion and Cortical Tension Specifies Tissue Surface Tension. Proc Natl Acad Sci USA (2010) 107(28):12517–22. doi:10.1073/pnas.1003743107
119. Delile J, Herrmann M, Peyriéras N, Doursat R. A Cell-Based Computational Model of Early Embryogenesis Coupling Mechanical Behaviour and Gene Regulation. Nat Commun (2017) 8(1):13929. doi:10.1038/ncomms13929
120. Sharpe J. Computer Modeling in Developmental Biology: Growing Today, Essential Tomorrow. Development (2017) 144(23):4214–25. doi:10.1242/dev.151274
121. Sahu P, Sussman DM, Rübsam M, Mertz AF, Horsley V, Dufresne ER. Small-scale Demixing in Confluent Biological Tissues. Soft Matter (2020) 16(13):3325–37. doi:10.1039/C9SM01084J
122. Wang X, Merkel M, Sutter LB, Erdemci-Tandogan G, Manning ML, Kasza KE. Anisotropy Links Cell Shapes to Tissue Flow during Convergent Extension. Proc Natl Acad Sci USA (2020) 117(24):13541–51. doi:10.1073/pnas.1916418117
123. Lawson-Keister E, Manning ML. Jamming and Arrest of Cell Motion in Biological Tissues arXiv (2021).
125. Bi D, Lopez JH, Schwarz JM, Manning ML. Energy Barriers and Cell Migration in Densely Packed Tissues. Soft Matter (2014) 10(12):1885–90. doi:10.1039/C3SM52893F
126. Moshe M, Bowick MJ, Marchetti MC. Geometric Frustration and Solid-Solid Transitions in Model 2D Tissue. Phys Rev Lett (2018) 120(26):268105. doi:10.1103/PhysRevLett.120.268105
127. Douezan S, Guevorkian K, Naouar R, Dufour S, Cuvelier D, Brochard-Wyart F. Spreading Dynamics and Wetting Transition of Cellular Aggregates. Proc Natl Acad Sci (2011) 108(18):7315–20. doi:10.1073/pnas.1018057108
129. Newman MEJ, Strogatz SH, Watts DJ. Random Graphs with Arbitrary Degree Distributions and Their Applications. Phys Rev E (2001) 64(2):026118. doi:10.1103/PhysRevE.64.026118
130. Dorogovtsev SN, Mendes JFF. Evolution of Networks: From Biological Nets to the Internet and WWW. Oxford: Oxford University Press (2003). doi:10.1093/acprof:oso/9780198515906.001.0001
131. Newman M. Networks: An Introduction. Oxford: Oxford University Press (2010). doi:10.1093/acprof:oso/9780199206650.001.0001
132. Maxwell JC. I.-On Reciprocal Figures, Frames, and Diagrams of Forces. Trans R Soc Edinb (1870) 26(1):1–40. doi:10.1017/S0080456800026351
133. Muñoz JJ, Albo S. Physiology-based Model of Cell Viscoelasticity. Phys Rev E (2013) 88(1):012708. doi:10.1103/PhysRevE.88.012708
134. Silverberg JL, Barrett AR, Das M, Petersen PB, Bonassar LJ, Cohen I. Structure-Function Relations and Rigidity Percolation in the Shear Properties of Articular Cartilage. Biophysical J (2014) 107(7):1721–30. doi:10.1016/j.bpj.2014.08.011
135. Poole AR, Pidoux I, Rosenberg L. Role of Proteoglycans in Endochondral Ossification: Immunofluorescent Localization of Link Protein and Proteoglycan Monomer in Bovine Fetal Epiphyseal Growth Plate. J Cell Biol (1982) 92(2):249–60. doi:10.1083/jcb.92.2.249
136. Forgacs G, Newman SA, Hinner B, Maier CW, Sackmann E. Assembly of Collagen Matrices as a Phase Transition Revealed by Structural and Rheologic Studies. Biophysical J (2003) 84(2 Pt 1):1272–80. doi:10.1016/s0006-3495(03)74942-x
137. Yadavalli VK, Svintradze DV, Pidaparti RM. Nanoscale Measurements of the Assembly of Collagen to Fibrils. Int J Biol Macromolecules (2010) 46(4):458–64. doi:10.1016/j.ijbiomac.2010.02.012
138. Shivers JL, Arzash S, Sharma A, MacKintosh FC. Scaling Theory for Mechanical Critical Behavior in Fiber Networks. Phys Rev Lett (2019) 122(18):188003. doi:10.1103/PhysRevLett.122.188003
139. Iravani A, Thambyah A, Burrowes KS. A Viscoelastic Two-Dimensional Network Model of the Lung Extracellular Matrix. Biomech Model Mechanobiol (2020) 19(6):2241–53. doi:10.1007/s10237-020-01336-1
140. Pinheiro D, Bellaïche Y. Mechanical Force-Driven Adherens Junction Remodeling and Epithelial Dynamics. Dev Cell (2018) 47(1):3–19. doi:10.1016/j.devcel.2018.09.014
141. Kasza KE, Rowat AC, Liu J, Angelini TE, Brangwynne CP, Koenderink GH. The Cell as a Material. Curr Opin Cell Biol (2007) 19(1):101–7. doi:10.1016/j.ceb.2006.12.002
142. Ranft J, Basan M, Elgeti J, Joanny J-F, Prost J, Jülicher F. Fluidization of Tissues by Cell Division and Apoptosis. Proc Natl Acad Sci (2010) 107(49):20863–8. doi:10.1073/pnas.1011086107
143. Saadaoui M, Rocancourt D, Roussel J, Corson F, Gros J. A Tensile Ring Drives Tissue Flows to Shape the Gastrulating Amniote Embryo. Science (2020) 367(6476):453–8. doi:10.1126/science.aaw1965
144. Zhu M, Tao H, Samani M, Luo M, Wang X, Hopyan S. Spatial Mapping of Tissue Properties In Vivo Reveals a 3D Stiffness Gradient in the Mouse Limb Bud. Proc Natl Acad Sci USA (2020) 117(9):4781–91. doi:10.1073/pnas.1912656117
145. Barber DL, Trepat X. Editorial Overview: Cell Dynamics: Integrating Cell Dynamics across Scales. Curr Opin Cell Biol (2020) 66:130–2. doi:10.1016/j.ceb.2020.06.003
146. van Doorn JM, Lageschaar L, Sprakel J, van der Gucht J. Criticality and Mechanical Enhancement in Composite Fiber Networks. Phys Rev E (2017) 95(4):042503. doi:10.1103/PhysRevE.95.042503
147. Latorre E, Kale S, Casares L, Gómez-González M, Uroz M, Valon L. Active Superelasticity in Three-Dimensional Epithelia of Controlled Shape. Nature (2018) 563(7730):203–8. doi:10.1038/s41586-018-0671-4
148. Brangwynne CP, MacKintosh FC, Kumar S, Geisse NA, Talbot J, Mahadevan L. Microtubules Can bear Enhanced Compressive Loads in Living Cells Because of Lateral Reinforcement. J Cell Biol (2006) 173(5):733–41. doi:10.1083/jcb.200601060
149. Das M, MacKintosh FC. Poisson's Ratio in Composite Elastic Media with Rigid Rods. Phys Rev Lett (2010) 105(13):138102. doi:10.1103/PhysRevLett.105.138102
150. Huisman EM, Heussinger C, Storm C, Barkema GT. Semiflexible Filamentous Composites. Phys Rev Lett (2010) 105(11):118101. doi:10.1103/PhysRevLett.105.118101
151. Lai VK, Nedrelow DS, Lake SP, Kim B, Weiss EM, Tranquillo RT. Swelling of Collagen-Hyaluronic Acid Co-gels: An In Vitro Residual Stress Model. Ann Biomed Eng (2016) 44(10):2984–93. doi:10.1007/s10439-016-1636-0
152. Burla F, Tauber J, Dussi S, van der Gucht J, Koenderink GH. Stress Management in Composite Biopolymer Networks. Nat Phys (2019) 15(6):549–53. doi:10.1038/s41567-019-0443-6
153. Oriola D, Marin-Riera M, Aalderink G, Anlas K, Gritti N, Sharpe J (2020). Arrested Coalescence of Multicellular Aggregates. arXiv.2012.01455.
154. Pajic-Lijakovic I, Milivojevic M. Viscoelasticity and Cell Jamming State Transition. bioRxiv (2021) 436195, 2021. 2021.2003.2019. doi:10.1101/2021.03.19.436195
155. Pajic-Lijakovic I, Milivojevic M. Multiscale Nature of Cell Rearrangement Caused by Collective Cell Migration. Eur Biophys J (2021) 50(1):1–14. doi:10.1007/s00249-021-01496-7
156. Sklar L. Physics and Chance: Philosophical Issues in the Foundations of Statistical Mechanics. Cambridge: Cambridge University Press (1993). doi:10.1017/cbo9780511624933
157. Hidalgo J, Grilli J, Suweis S, Muñoz MA, Banavar JR, Maritan A. Information-based Fitness and the Emergence of Criticality in Living Systems. Proc Natl Acad Sci (2014) 111(28):10095–100. doi:10.1073/pnas.1319166111
158. Arias AM, Hayward P. Filtering Transcriptional Noise during Development: Concepts and Mechanisms. Nat Rev Genet (2006) 7(1):34–44. doi:10.1038/nrg1750
159. Mateus AM, Gorfinkiel N, Arias AM. Origin and Function of Fluctuations in Cell Behaviour and the Emergence of Patterns. Semin Cell Dev Biol (2009) 20(7):877–84. doi:10.1016/j.semcdb.2009.07.009
160. Garcia-Ojalvo J, Martinez Arias A. Towards a Statistical Mechanics of Cell Fate Decisions. Curr Opin Genet Dev (2012) 22(6):619–26. doi:10.1016/j.gde.2012.10.004
161. Newman M, Barabasi A-L, Watts DJ. The Structure and Dynamics of Networks. Princeton: Princeton University Press (2006).
Keywords: viscoelasticity, percolation theory, network, morphogenesis, rigidity
Citation: Corominas-Murtra B and Petridou NI (2021) Viscoelastic Networks: Forming Cells and Tissues. Front. Phys. 9:666916. doi: 10.3389/fphy.2021.666916
Received: 11 February 2021; Accepted: 19 May 2021;
Published: 09 June 2021.
Edited by:
Elias H. Barriga, Gulbenkian Institute of Science (IGC), PortugalReviewed by:
Melissa Turan, University College London, United KingdomCopyright © 2021 Corominas-Murtra and Petridou. This is an open-access article distributed under the terms of the Creative Commons Attribution License (CC BY). The use, distribution or reproduction in other forums is permitted, provided the original author(s) and the copyright owner(s) are credited and that the original publication in this journal is cited, in accordance with accepted academic practice. No use, distribution or reproduction is permitted which does not comply with these terms.
*Correspondence: Nicoletta I. Petridou, bmljb2xldHRhLnBldHJpZG91QGVtYmwuZGU=
Disclaimer: All claims expressed in this article are solely those of the authors and do not necessarily represent those of their affiliated organizations, or those of the publisher, the editors and the reviewers. Any product that may be evaluated in this article or claim that may be made by its manufacturer is not guaranteed or endorsed by the publisher.
Research integrity at Frontiers
Learn more about the work of our research integrity team to safeguard the quality of each article we publish.